Artificial Intelligence Playbook for the UK Government (HTML)
Published 10 February 2025
Acknowledgements
The publication of this playbook has been made possible by the support from a large number of stakeholders.
Central government departments
Crown Commercial Service (CCS); Cabinet Office (CO), including the Number 10 Data Science (No.10 DS) and i.AI teams; Department for Business and Trade (DBT); Department for Education (DfE); Department for Environment, Food and Rural Affairs (DEFRA); Department for Energy Security and Net Zero (DESNZ); Department of Health and Social Care (DHSC); Department for Levelling Up, Housing and Communities (DLUHC); Department for Science, Innovation and Technology (DSIT), including the Government Office for Science (GO-Science) and the Responsible Technology Adoption Unit (RTA); Department for Transport (DfT); Department for Work and Pensions (DWP); Foreign, Commonwealth and Development Office (FCDO), including FCDO Services; Government Legal Department (GLD); HM Land Registry (HMLR); HM Revenue and Customs (HMRC); HM Treasury (HMT); Home Office (HO); Ministry of Defence (MoD); and Ministry of Justice (MoJ).
Arm’s length bodies, devolved administrations and public sector bodies
Driver and Vehicle Licensing Agency (DVLA); Government Communications Headquarters (GCHQ); Government Internal Audit Agency (GIAA); HM Courts and Tribunals Service (HMCTS); Information Commissioner’s Office (ICO); Met Office; National Health Service (NHS); Office for National Statistics (ONS); and the Scottish Government.
Industry
Amazon (AWS), Google, IBM and Microsoft.
Academic institutes
Alan Turing Institute; BCS, The Chartered Institute for IT; Oxford Internet Institute; Manchester Metropolitan University; and the University of Surrey.
User research
User research participants have come from a wide range of departments and have been very generous with their time.
Update log
We have made the following core updates since the previous edition:
- we have reviewed and updated the 10 principles and the guidance within the Generative AI Framework to cater for a wider range of AI technologies beyond generative AI
- we have added new sections on topics such as What is AI?, Fields of AI (including a new diagram), User research for AI, AI business cases, Societal wellbeing and public good, Security opportunities, Governance structures for teams, AI quality assurance and Managing risk. We have also included an Appendix with case studies on recent AI projects developed by public sector organisations
- we have reorganised and expanded the Working collaboratively, Using AI safely and responsibly and Ethics sections, to better articulate the importance of engaging with academia, industry and the broader civil society
- we have moved guidance and training on building AI solutions into separate AI insights articles and e-learning courses to allow for a more in-depth discussion
Foreword
I am pleased to introduce the AI Playbook for the UK Government, which updates and expands on the Generative AI Framework for HMG. This updated guidance will help government departments and public sector organisations harness the power of a wider range of AI technologies safely, effectively, and responsibly.
In January 2025 the government published the AI Opportunities Action Plan laying out a bold roadmap for maximising AI’s potential to drive growth and deliver real benefits to people across the UK.
The publication of the AI Playbook highlights the competence and extraordinary work already being done in the AI space across the public sector. Developed collaboratively, with input from many government departments, public sector institutions, academia, and industry, this guidance reflects our commitment to continuously engaging with and learning from wider civil society.
The AI Playbook will support the public sector in better understanding what AI can and cannot do, and how to mitigate the risks it brings. It will help ensure that AI technologies are deployed in responsible and beneficial ways, safeguarding the security, wellbeing, and trust of the public we serve.
The potential of AI to transform public services is enormous, giving us an unparalleled opportunity to do things differently and deliver more with less. AI is already helping civil servants spend less time on repetitive tasks, enabling teachers to personalise lessons, and can allow doctors to access life-saving insights faster, through AI-assisted diagnostics.
However, our journey with AI is just beginning. The AI Playbook is a launchpad that we will continuously revise and improve to help the UK public sector become a leading responsible user of AI technologies. As technology evolves, so too will our approach, ensuring we remain at the forefront of responsible innovation - always guided by the principle that technology must serve people.
I want to extend my sincere thanks to everyone who contributed their expertise to this AI Playbook, both within and beyond government. I look forward to ongoing collaboration as we continue to learn how to use AI safely, responsibly, and effectively to deliver solutions that are smarter, faster, and more responsive to the collective needs of our society.
Feryal Clark MP
Parliamentary Under-Secretary of State for AI and Digital Government
Department for Science, Innovation and Technology (DSIT)
Preface
When we published the Generative AI Framework for HMG in January 2024, we described it as ‘incomplete’ and ‘dynamic’. We didn’t pretend to have all of the answers in such a fast-moving field, but did aim to provide helpful, practical guidance to public servants on how to put generative AI to work confidently, responsibly and where it matters most.
The pace of change since we published that first version has not slowed, and interest in generative AI and other forms of AI has grown. The UK government continues to believe that AI has the power to drive productivity, innovation and economic growth. In 2021, the National AI Strategy set out a 10-year vision for AI, while the 2023 white paper A pro-innovation approach to AI regulation set out the government’s proposals for implementing a proportionate, future-proof and pro-innovation framework for regulating AI. In 2025, the AI Opportunities Action Plan highlighted how the government can leverage AI to boost productivity and improve services.
This updated version of the framework has been expanded to cover new developments, and we’ve retitled it to encompass all current forms of AI. As well as a general overview, it includes primers on various AI fields for the curious, and links to learning resources for those who want to dive deeper. The AI Playbook now covers the important and emerging discipline of conducting research with the users of AI systems. It addresses emerging cyber threats to those systems, and the ways that attackers are using AI to create new threats.
I would like to echo Minister Clark’s thanks to everyone who has contributed to this playbook. It has been a collective effort of experts from government departments, arm’s length bodies, other public sector organisations, academic institutions and industry partners. As we continue to advance together in the safe, responsible, and effective use of AI, I look forward to even broader collaboration and further contributions from an expanding community.
David Knott
Government Chief Technology Officer
Department for Science, Innovation and Technology (DSIT)
Principles
We have defined 10 common principles to guide the safe, responsible and effective use of artificial intelligence (AI) in government organisations. The white paper A pro-innovation approach to AI regulation sets out 5 principles for regulators to inform AI development in all sectors. This playbook builds on those principles and defines 10 core principles for AI use in government and public sector organisations.
- Principle 1: You know what AI is and what its limitations are
- Principle 2: You use AI lawfully, ethically and responsibly
- Principle 3: You know how to use AI securely
- Principle 4: You have meaningful human control at the right stage
- Principle 5: You understand how to manage the AI life cycle
- Principle 6: You use the right tool for the job
- Principle 7: You are open and collaborative
- Principle 8: You work with commercial colleagues from the start
- Principle 9: You have the skills and expertise needed to implement and use AI
- Principle 10: You use these principles alongside your organisation’s policies and have the right assurance in place
You can find posters on each of the 10 principles for you to display in your government organisation.
Principle 1: You know what AI is and what its limitations are
AI is a broad field subject to rapid research and innovation, and many claims have been made about both its promise and risks. You should learn about AI technology to understand what it can and cannot do, and the potential risks it poses.
AI systems currently lack reasoning and contextual awareness and their limitations vary depending on the tools you use and the context in which they operate. AI systems are also not guaranteed to be accurate. You should understand how to use AI tools safely and responsibly, employ techniques to increase the accuracy and correctness of their outputs, and have a process in place to test them.
You can find more about what AI is and its capabilities in the Understanding AI section.
Principle 2: You use AI lawfully, ethically and responsibly
AI solutions bring specific legal and ethical considerations. Your use of AI tools must be lawful and responsible. You should seek legal advice on the development and use of AI and engage with compliance, legal and data protection experts in your organisation early in your journey, including during product development. You may, for example, seek advice on equalities implications, fairness, intellectual property and other legal issues.
You should seek data protection advice on your use of AI. This may be from your lawyers or your data protection officer. AI systems can process personal data, so you need to consider how you protect this personal data, be compliant with data protection legislation, and minimise the risk of privacy intrusion from the outset.
You should establish and communicate how you will address ethical concerns throughout your project, from design to deployment, so that diverse and inclusive participation is built into the project life cycle. You should also consider the ethical principles presented in this playbook, and establish robust measures to suit your technological and deployment context.
AI models are trained on data which may include biased or harmful materials. As a result, AI systems may display biases and produce harmful outputs, such as unfair, prejudicial or derogatory representations of groups or individuals. You should consider all potential sources of bias throughout the development life cycle, including unrepresentative data sets and deployment scenarios that have unfair or undesirable impacts.
You must ensure that AI systems generate a positive impact on stakeholders and civil society at large while minimising potential harms as much as possible. When defining and deploying AI systems, you must understand people’s needs and priorities by conducting user research and engaging with the public as appropriate, including civil society groups, underrepresented individuals, those most likely to experience harm, NGOs, academia and industry.
You should understand and manage the environmental impact of the AI systems you are planning to use. You should also use AI technologies only when relevant, appropriate and proportionate. Choose the most suitable and sustainable option for your organisation’s needs.
You can find out more in the Using AI safely and responsibly section.
Principle 3: You know how to use AI securely
When building and deploying AI services, you must make sure that they are secure to use and resilient to cyber attacks, as laid out in the Government Cyber Security Strategy. Your service must comply with the Secure by Design principles, which were developed by the Central Digital and Data Office (CDDO), and the government’s Cyber Security Standard.
Different types of AI are susceptible to different security risks. Some threats – such as data poisoning, perturbation attacks, prompt injections and hallucinations – are specific to AI. However, AI systems can also amplify generic risks such as phishing and cyber attacks. You must understand the risks associated with your use of AI and of adversaries potentially using AI against you.
To minimise these risks you should build in safeguards and put technical controls in place. These include security testing and, in the case of generative AI, content filtering to detect malicious activity, as well as validation checks to ensure responses are accurate and do not leak data.
You can find out more in the Security and Data protection and privacy sections.
Principle 4: You have meaningful human control at the right stages
You need to monitor the AI’s behaviour and have plans in place to prevent any harmful effects on users. This includes ensuring that humans validate any high-risk decisions influenced by AI and that you have strategies for meaningful intervention.
For applications where instant responses are required and human review is not possible in real time, such as chatbots, it’s important that you ensure human control at other stages of the AI’s development and deployment.
You should fully test the product before deployment, and have robust assurance and regular checks of the live tool in place. Since AI models can sometimes produce unwanted or inaccurate results, incorporating feedback from users is crucial. You should have systems in place that allow users to report issues and prompt a human review.
You can find out more in the Human oversight section.
Principle 5: You understand how to manage the full AI life cycle
AI solutions, like other technology deployments, have a full product life cycle that you need to understand. You should know how to choose the right tool for the job, be able to set it up and have the right resource in place to support day-to-day maintenance of it. You should also know how to update the system and how to securely close it down at the end of its useful life.
You should understand how to monitor and mitigate for potential drift, bias, and, in the case of generative AI, hallucinations. You should also have a robust testing and monitoring process in place to catch these problems. You should use the Technology code of practice to build a clear understanding of technology deployment life cycles, and understand and use the NCSC cloud security principles.
You should understand the benefits, use cases and other applications that your solution could support across government and the wider public sector. The Rose Book provides guidance on government-wide knowledge assets and The Government Office for Technology Transfer can provide support and funding to help develop government-wide solutions. If you develop a service, you must use the government Service Standard.
You can find out more about development best practices for AI in the Building AI solutions section.
Principle 6: You use the right tool for the job
You should select the most appropriate technology to meet your needs. AI is good at many tasks, but there are a wide range of models and products. You should be open to solutions involving AI because they can allow organisations to develop new or faster approaches to the delivery of public services, can provide a springboard for more creative and innovative thinking about policy and public sector problems, and help your team with time-consuming tasks. However, you should also be open to the conclusion that, sometimes, AI is not the best solution for your problem: it may be more easily solved with more established technologies.
When implementing AI solutions, you should select the most appropriate deployment patterns and choose the most suitable model for your use case. You can learn about how to choose the right technologies for your task or project in the Identifying use cases for AI and Use cases to avoid sections.
Principle 7: You are open and collaborative
There are many teams across government and the wider public sector using or exploring AI tools in their work. You should make use of existing cross-government communities where there is a space to solve problems collaboratively, such as the AI community of practice. You should also engage with other government departments that are trying to address similar issues and reuse ideas, code and infrastructure.
Where possible, you should engage with the wider civil society including groups, communities, and non-governmental, academic and public representative organisations that have an interest in your project. Collaborating with people both inside and outside government will help you ensure we use AI to deliver tangible benefits to individuals and society as a whole. Make sure you have a clear plan for engaging and communicating with these stakeholders at the start of your work.
You should be open with the public about where and how algorithms and AI systems are being used in official duties. If you’re a central government department or an arm’s length body within scope, you’re required to use the Algorithmic Transparency Recording Standard (ATRS). This means you must document information about any algorithmic tools you use in decision-making processes and make it clearly accessible to the public. The ATRS is not a requirement for all arm’s length bodies and other public sector institutions yet, but we still encourage you to use it. You should also clearly identify any automated response to the public. For example, a response generated via a chatbot interface should include something like ‘this response has been written by an automated AI chatbot’.
You can find out more in the Ethics section.
Principle 8: You work with commercial colleagues from the start
AI is a rapidly developing market, and you should get specific advice from commercial colleagues on the implications for your project. Reach out to them early in your journey to understand how to use AI in line with commercial requirements.
You should also work with commercial colleagues to ensure that the expectations around the responsible and ethical use of AI are the same between AI systems developed in-house and those procured from a third party. For example, contracts between the public sector and third parties can be drafted to require transparency from the supplier on the different information categories, as set out in the ATRS. You can find out more in the Buying AI section.
Principle 9: You have the skills and expertise needed to implement and use AI solutions
You should understand the technical and ethical requirements for using AI tools and have them in place within your team.
You and your team should gain the skills needed to use, design, build and maintain AI solutions, keeping in mind that developing bespoke AI solutions and training your own models require different specialist skills to using pre-trained models accessible through application programming interfaces (APIs).
Decision makers, policy professionals and senior responsible owners (SROs) should gain the skills they need to understand the risks and opportunities of AI, including its potential impact on organisational culture, governance, ethics and strategy.
You should take the free AI courses on Civil Service Learning and proactively keep track of developments in the field. You can find out more in our Acquiring skills and talent section.
Principle 10: You use these principles alongside your organisation’s policies and have the right assurance in place
These principles and this playbook set out a consistent approach for the UK government to use AI tools. While you should use these principles when working with AI, many government organisations have their own governance structures and policies in place. You should follow any organisation-specific policies, especially ones about security and data handling.
You should understand, monitor and mitigate the risks that using AI tools can bring. Connect with the right assurance teams in your organisation early in the project life cycle for your AI solutions. You should have clearly documented review and escalation processes in place, and have an AI review board or programme-level board.
You can find out more in the Governance section.
Understanding AI
This section explains what AI is, what its main fields are, the applications of AI and generative AI in government and their limitations. It supports Principle 1: You know what AI is and what its limitations are.
What is AI?
AI is not new. The term ‘artificial intelligence’ was coined in 1956 during the Dartmouth workshop, a gathering of scientists intent on exploring the potential of computing to emulate human reasoning. Since then, there have been recurring waves of progress and excitement, followed by periods of waning interest and investment referred to as ‘AI winters’. We use the definition of AI adopted by OECD countries:
An AI system is a machine-based system that, for explicit or implicit objectives, infers, from the input it receives, how to generate outputs such as predictions, content, recommendations, or decisions that can influence physical or virtual environments. Different AI systems vary in their levels of autonomy and adaptiveness after deployment.
The UK government’s paper Establishing a pro-innovation approach to regulating AI suggests that these systems are ‘adaptable’ because they can find new ways to meet the objectives set by humans, and ‘autonomous’ because, once programmed, they can operate with varying levels of autonomy, including without human control.
Fields of AI
AI comprises a complex and evolving set of fields. These include a broad, interconnected range of algorithms, models and processes. Advances in one area typically propagate throughout these networks of technologies, conferring novel behaviours and increases in accuracy and capability to ancestor models.
The following diagram illustrates the interdependencies between some existing and emerging fields of AI. As you can see, capturing the evolving complexity of AI is a challenging task.
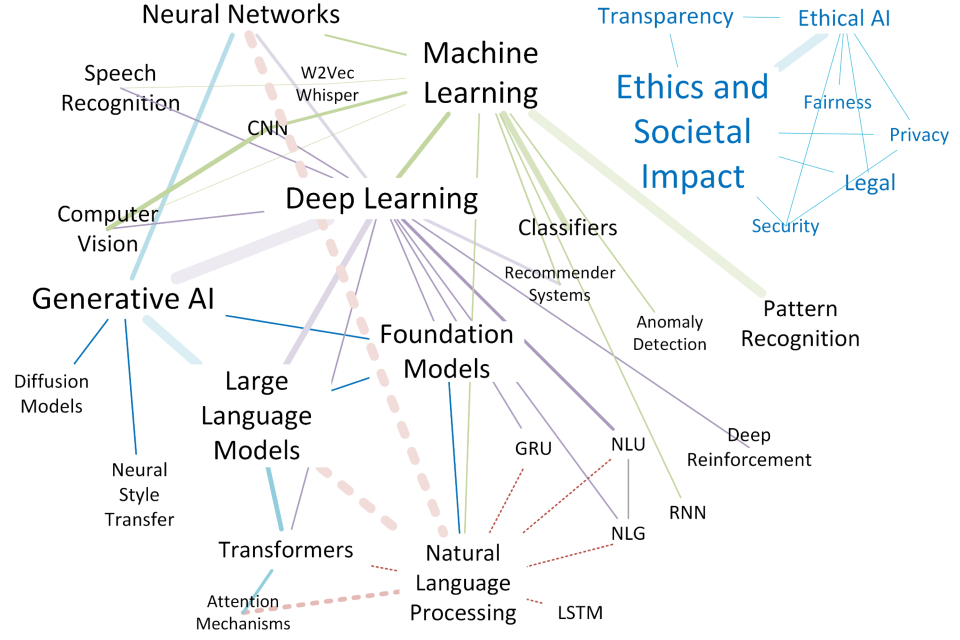
The complexity of the AI space continues to increase: as AI technologies evolve they often branch out, advancing some research areas while leaving others unchanged. For example, in the field of computer vision (CV) some legacy systems still thrive today due to the simplicity of their behaviours, their low computational and memory requirements, and their stability and well-proven performance. These legacy CV systems fall within the domain of machine learning (ML). However, more recent and capable CV systems, such as those used in self-driving or medical imaging systems, are considerably more complex and belong to CV as part of deep learning (DL).
As software and algorithms are advancing, so too is hardware infrastructure. Traditional computer processing units (CPUs) can struggle with the large amounts of data and calculations AI requires. New types of hardware have been implemented to train and run AI models faster and more efficiently. For example, the largest modern AI systems (ranging in the hundreds of billions to trillions of parameters) are trained on networks of thousands of graphics processing units (GPUs), while Tensor Processing Units (TPUs) and Neural Processing Units (NPUs) are increasingly used to optimise ML training.
Below we provide an overview of the main fields of AI. For a deeper understanding of these fields you can:
- take the free e-learning courses available on Civil Service Learning
- use the other training opportunities mentioned in the Acquiring skills and talent section
- refer to the AI insights articles
Neural networks
Neural networks (NNs), or artificial neural networks (ANNs), are a computational model inspired by biological NNs in the human brain. They were initially created as an aspect of ML in the 1940s but they may now be considered as components of more elaborate DL systems.
How do NNs work?
An NN learns through exposure to data in training cycles. During these cycles, the model gradually adjusts the connections between different parts of the network, setting the weights between nodes of adjacent layers. When the model makes an error, the network calculates it as data and checks how far off it was. The error is then adjusted backwards through the network by updating the weights by a very small amount (the learning rate) in the direction that will serve to minimise that error. Repeated executions of this process during the training phase tune the network so that it can generalise faithfully on related data it has never seen before.
Applications of NNs
NNs have a wide range of applications due to their ability to recognise patterns, process new data, make predictions and improve over time. NNs are often deployed in image recognition applications, medical imaging, speech recognition models, autonomous systems, and in many other examples.
Machine learning
ML is the branch of AI that learns from data. It does this by extracting features from data and learning the relationships between those features. Anywhere there is data, there is an opportunity to learn from it. ML can provide the public sector with unique and quantifiable insights into data that were previously impossible, expensive, or of limited or short-term utility.
How does ML work?
ML uses algorithms to analyse data, learn patterns, and then make predictions or decisions based on new data. To work effectively, ML systems must be trained using carefully selected information. This training helps create an optimised model that identifies pertinent features in the data, and weights their relationships to understand how they relate to each other.
ML systems can be trained using either ‘labelled’ or ‘unlabelled’ data, or a combination of both. Labelled data is data that has been tagged or categorised in advance. This form of training is usually called ‘supervised learning’ because the model is trained under the supervision of labelled data. Each example in the training data set comes with a correct answer, or label, from which the model is supposed to learn. When unlabelled data is used, the model is programmed to identify patterns, groupings or structures on its own. This is often referred to as ‘unsupervised learning’.
Applications of ML
The ability of ML models to learn from data, identify changes, and update based on changes in underlying data enables a host of capabilities that would simply not be possible through traditional linear, deterministic systems. ML models can be used as elements of much larger DL models, or as data processing participants in a sequence of operations, and they underpin many applications including fraud detection, feedback analysis, image processing, summarisation, and more. Modern large language models (LLMs) are also examples of ML systems.
Deep learning
DL is a subset of ML that involves more complex model structures and architectures, including sophisticated NNs, to learn complex patterns and representations from large amounts of data. DL has complemented and expanded upon ML, but ML remains the first-choice candidate for simpler tasks and can be easier to implement with less data and lower computational power. DL, while more resource-intensive, excels in handling complex tasks and larger data sets.
How does DL work?
DL models initially detect simple features – edges in an image, for example – and gradually combine them to recognise more complex patterns, such as identifying a face or understanding speech.
Applications of DL
DL is used in advanced applications like biomedical research and autonomous driving, but also in image and speech recognition (SR), natural language processing (NLP), personalised recommendations, and more.
Speech recognition
SR, sometimes referred to as automatic speech recognition (ASR), is a field of ML dedicated to processing speech. SR includes both systems that convert speech into text (STT) and new speech-to-speech (S2S) systems.
How does SR work?
Computers only understand numbers, so the challenge with SR is to turn spoken words into numbers while keeping their meaning and context. To do so, SR models first convert speech into numeric representations called spectrograms, which show sound frequencies over time. DL models then analyse these spectrograms to identify sounds, words or sentences. In STT, this process results in written text. In S2S, the recognised words are further processed by another model that translates the text into a different language, which is then turned back into spoken words.
Applications of SR
SR has expanded considerably over recent years, especially in terms of voice recognition. This technology is now used in banking, personal agents like Siri and Alexa, and general SR functionality built into phones and cars, among other things. Modern SR applications include call analytics, emergency services, meeting summarisation and media subtitling, and more.
Computer vision
CV is a field of AI and ML that enables computers to interpret, analyse and understand visual information (images and videos) to perform tasks such as object recognition, facial recognition and scene understanding. Although CV has been researched since the 1960s, it has progressed considerably in recent decades due to a combination of developments in model architecture, hardware performance, and data quality and volumes.
How does CV work?
CV systems are intended to make image data comprehensible and interpretable so that it can be consumed and evaluated appropriately. These evaluations most commonly include classification, object detection, video analysis and image segmentation.
To perform these tasks, CV uses algorithms to break down images into pixels and process the pixels to detect edges, shapes and colours. The system then uses this information to recognise objects, people or scenes – similarly to how our brains process visual information – allowing computers to identify the content of images.
Applications of CV
CV has many applications in fields such as facial recognition, quality control, healthcare and medical imaging, surveillance, robotics, and more.
Natural language processing
NLP is a field within AI that focuses on processing human language. NLP combines computational linguistics and machine learning to analyse large amounts of natural language data and comprehend, interpret, translate, and generate language and language-related data. LLMs, which are in widespread use, are a subset of NLP, with NLP preceding them by several decades.
How does NLP work?
NLP uses algorithms to convert text into numerical representations that are then processed by an ML model. This process involves a series of steps including noise reduction, tokenisation, stop-words removal, stemming or lemmatisation, vectorisation, and embeddings. The model uses the weights learned during training to adjust the importance of different features in the input data, and considers various elements, including the order and context of words, to understand the overall meaning of the text and produce the required outputs.
Applications of NLP
NLP is used for a wide variety of applications and tasks related to language, including machine translation, document classification, sentiment analysis, parts-of-speech tagging, named-entity recognition, text summarisation and conversational AI.
For government departments, NLP can play an important role in managing and processing large volumes of human language data from sources like emails, letters and online forms, enabling efficient and meaningful analysis.
Generative AI
Generative AI is a subset of AI capable of generating text, images, video or other forms of output by using probabilistic models trained across various domains. Generative AI learns from large amounts of specially curated training data to discern and replicate complex patterns and structures. The output of generative AI models mimics the characteristics learned from the training data, enabling a range of novel applications. These include personalised content generation, advanced analysis and evaluation, and aiding creative processes.
Examples of publicly accessible generative AI tools are ChatGPT, Claude, Gemini and Dall-E. Generative AI is also becoming increasingly integrated into mainstream products. Examples include the Adobe Photoshop Generative Fill tool, AWS ChatOps Chatbot, Microsoft 365 Copilot and Google Duet AI.
How does generative AI work?
Generative AI uses large quantities of carefully selected data to train models so they may learn the underlying patterns and structure of data. A well-known example of generative AI is LLMs, which are large neural networks specifically trained on text and natural language data to generate high quality, text-based outputs. However, there are many other generative AI models that are not purely text based.
Once trained, generative AI models are capable of generating new content consistent with the features and relationships learned from the training data. When a user provides a prompt or input to an LLM, the model evaluates the probability of potential responses based on what it has learned from its training data. It then selects and presents the response that is likeliest to be the right fit for the given prompt.
Applications of generative AI
The applications of generative AI go beyond the simple generation of new text or images. For example, generative AI is used in medicine to test molecular structures for drug discovery, and in the financial sector to generate multimodal data surfaces approximating trading and market conditions in order to test safety, security, trading and trade surveillance models. Generative Adversarial Networks (GANs) are also used for creating synthetic data sets to train ML models, as well as in other areas such as deepfake detection, self-driving model ensembles, and text-to-image synthesis. You can explore more applications of generative AI in the Applications of AI in government section.
For more on how to build, fine-tune and use generative AI solutions, refer to our series of AI insights.
Agentic AI
Agentic AI refers to autonomous AI systems that can make decisions and perform actions with minimal human intervention. These agents are capable of understanding their environment, identifying the set of tools and functions at their disposal, and using those to take actions to achieve their objectives.
Agent-based systems use Foundation Models (primarily LLMs) to match their capabilities with their objectives. For example, an order processing system may have multiple agents autonomously capturing pricing and market-related data. When a request for an order is raised, the agentic system may utilise those prices or, seeing that they haven’t been updated recently, may use another agent to retrieve the latest price available.This all happens automatically in the background because the system knows which agents can perform each task.
These systems provide a simpler way to build powerful AI-driven solutions by focusing on the capabilities of each agent and letting the AI model itself figure out the best way to achieve the system’s objectives. The high level of abstraction involved in this technology makes it easier to create more efficient and effective systems that take full advantage of AI.
Ethics and societal impact
As research developments have increased the presence of AI in the public sector and everyday life, ethical and societal considerations remain essential to the adoption and use of AI.
Applications of AI in government offer important benefits, such as improving productivity and enhancing access to services. However, AI systems also have limitations and can have two-sided impacts if not used in responsible ways and with the appropriate safeguards in place.
You can read more on the legal, ethical and security implications of AI in the Using AI safely and responsibly section.
Applications of AI in government
AI has a broad range of capabilities and has been relevant to the work of government for many years. The ability of generative AI to process language and produce new text, images and code has further enhanced the potential applications of AI technology.
You can find an overview of how government bodies are using AI – including the central government’s plans for supporting the adoption of AI – in the report on the Use of artificial intelligence in government (2024) by the National Audit Office (NAO).
The following table presents some examples of potential applications of both AI and generative AI technologies in government.
Application | AI | Generative AI |
Speed up the delivery of services | Machine learning (ML) and optical character recognition (OCR) algorithms can support the processing of thousands of handwritten letters per day, reducing response times. | Can retrieve relevant organisational information faster to answer digital queries or route email correspondence to the right parts of the business. |
Reduce staff workload | AI technologies for facial recognition and data analytics allow for the automatic control of passports in airports, freeing staff from this task. | Can suggest first drafts of routine email responses or computer code, acting as an autocomplete tool for algorithms. |
Perform complicated tasks | ML can analyse very large data sets, identify trends and anomalies in complex historical data, and support data-driven decision making. | Can help review and summarise huge amounts of information, as well as identify and correct errors in long algorithms. |
Perform specialist tasks more cost-effectively | Predictive analytics can identify future needs for resources, optimising budget allocations and planning. ML can detect fraudulent activities in different fields, preventing financial losses and protecting against cyber threats. | Can summarise documentation containing specialist language like financial or legal terms, or translate a document into several different languages. |
Improve the quality of services | Recommender systems can help users navigate government web pages and find the information they need. AI can also analyse thousands of feedback messages and suggest service improvements. | Can improve the readability and accessibility of information on web pages. For example, by simplifying complex language, improving formatting and generating alternative text for images. |
The Identifying use cases for AI section can help you select appropriate applications of AI tools. For real-life examples of some of these applications, refer to the AI use cases in the public sector appendix.
However, AI systems still have limitations. You should make sure that you understand these and build appropriate testing and controls into any AI solutions.
Limitations of AI
The capabilities of AI are improving over time. However, they do not provide the answer to every problem. Some of the limitations of AI systems are:
- bias: AI systems lack consciousness and their outputs tend to replicate the bias present in the data they were trained on. For example, their performance can be affected by model bias – an innate deviation in the model giving rise to an error between predicted and actual values – and algorithmic bias – which refers to systematic inequality in outcome from a model. For more information, refer to the Review into bias in algorithmic decision-making (PDF, 9.9MB) and the Introduction to AI assurance (PDF, 1,149KB) guidance. There is more about the ethical implications of bias in the Fairness, bias and discrimination section
- data quantity and quality: AI systems heavily rely on the quality and quantity of data to drive accuracy. Insufficient data can lead to the model failing to generalise effectively, and, similarly, poor quality, biased or noisy data can lead to inaccurate outcomes if not managed correctly
- accuracy: it’s difficult to produce an AI system which provides 100% accurate outputs under all conditions. You must be clear about what objective measures you’re assessing the AI outputs against and any factors that impact them
- transparency and explainability: some AI models – for example, deep learning architectures and convolutional or recurrent neural networks – can be so sophisticated that it is challenging to trace how a specific input leads to a specific output
- cost and sustainability: depending on the tool you use, AI implementation can be complex, time consuming and have considerable compute costs. Ongoing investment is needed to maintain these systems, update them with new data if required, and ensure their performance remains stable over time. It’s important to consider the sustainability impact of deploying AI systems and check if there are better alternatives
Generative AI also has further specific limitations. You need to be aware of these limitations and have checks and assurance in place when using generative AI in your organisation.
- hallucination (confabulation): large language models (LLMs) and other probabilistic generative models are vulnerable to creating content that appears plausible but may actually be factually incorrect, as they generate content by returning the most likely output for a given input based on the patterns learnt from the data they were trained on, without an actual understanding of the content
- lack of critical thinking, personal experience and judgement: although some LLM-based AI systems can give the appearance of reasoning and their outputs may appear as if they come from a person, they are in no way sentient
- sensitive or inappropriate context: LLMs can generate offensive or inappropriate content if not properly guided, since they can replicate any bias or toxic material present in the data they were trained on
-
domain expertise: LLMs are not domain experts. They are not a substitute for professional advice, especially in legal, medical or other critical areas where precise and contextually relevant information is essential
- dynamic real-time information retrieval: some well-known LLMs such as ChatGPT, Gemini and Copilot are now able to include access to real-time internet data in their results. But there are still many LLMs that do not have real-time access to the internet or data outside their training set
Building AI solutions
This section outlines the practical steps you’ll need to take when building AI solutions, including defining the goal, building the team, managing business change, buying AI and building the solution. It supports:
- Principle 1: You know what AI is and what its limitations are
- Principle 3: You know how to use AI securely
- Principle 4: You have meaningful human control at the right stage
- Principle 5: You understand how to manage the AI life cycle
- Principle 6: You use the right tool for the job
- Principle 8: You work with commercial colleagues from the start
- Principle 9: You have the skills and expertise needed to implement and use AI
- Principle 10: You use these principles alongside your organisation’s policies and have the right assurance in place
However, following the guidance in this section is only part of what is needed to build AI solutions. You also need to make sure that you’re using AI safely and responsibly.
Building the team
Like any other digital service, developing AI projects requires more than just technology. You will need to work collaboratively in a multidisciplinary team with diverse expertise.
The government Service Manual offers step-by-step guidance on how to set up a service team for the development and management of digital services. At the outset of your AI project, you need to form a service team and identify key collaborations with other teams and departments.
Your minimum viable AI team must be able to:
- identify user needs and accessibility requirements
- manage and report to stakeholders and other teams, collaborating with different field experts
- design, build, test and iterate AI products, using agile methodologies
- ensure the responsible development of lawful, ethical, secure and safe-by-design AI services
- be able to collect, process, store and manage data ethically, safely and securely
- test with real users and measure the performance of the service
- support the live running of the service, iterate and retire it
You can use the Government Digital and Data Profession Capability Framework to identify skill gaps in your team, forecast workforce needs and create effective and consistent job adverts. Be aware that your capability needs will change during the project life cycle: use the Service Manual to understand the roles you will need in the different phases of your project.
You will need the right balance between technical and domain expertise, depending on the nature of your project. As well as building a team that contains the right skills, make sure it includes a diversity of groups and viewpoints to help you stay alert to risks of bias and discrimination. Your team should include or be able to collaborate with:
- business leaders and experts who understand the context and impact on users and services
- data scientists who understand the relevant data, how to use it effectively, and how to build and train and test models
- software engineers who can build and integrate solutions
- user researchers and designers who can help understand user needs and design compelling experiences
- legal, commercial and security colleagues, as well as ethics and data privacy experts, who can help you make your AI solution safe and responsible
Considering the current shortage of AI talent, consider adopting strategies that combine new hires, working with contractors or third parties, and internal upskilling.
Acquiring skills and talent
The professional skills required for working in the digital space are outlined in the Government Digital and Data Profession Capability Framework. You can find more information on building a digital and data team in the government Service Manual.
As AI skills are in high demand, their inclusion in the government’s talent strategy is critical. Currently, the Government Digital and Data Profession’s approach involves integrating AI modules and learning materials into existing talent offerings. You can also source AI skills through senior digital secondments and internal collaboration with allied professions such as data engineering.
Raising general awareness of AI throughout the Civil Service and upskilling current digital and data professionals on AI remains crucial. Every civil servant can take up to 5 days of learning per year and some departments allow more days than others. It’s important that the members of your team have the necessary time and resources to update their knowledge and skills. Data, analytics and digital professionals can also benefit from having sandboxes and spaces to experiment with AI in a safe and secure way.
The cross-government user research on AI conducted by the Central Digital and Data Office (CDDO) in 2023 and 2024 identified the 5 groups of learners below.
- Beginners: civil servants who are new to AI and need to be familiar with its concepts, benefits, limitations and risks to be able to work with AI tools safely, responsibly and efficiently. Learning for this audience should focus on having an improved understanding of AI.
- Technical roles outside government digital and data: civil servants in roles such as operational delivery and policy profession who are likely to use AI in their work for tasks such as information retrieval and text or image generation. Learning for this audience should provide the necessary knowledge and skills to make effective and responsible use of appropriate AI tools – with particular attention given to generative AI, its limitations and prompt engineering.
- Data and analytics professionals: civil servants who work on the collection, organisation, analysis and visualisation of data, with varying levels of expertise in AI. Learning for this audience should advance their understanding of AI with a focus on the implementation and use of AI to facilitate automated data analysis, the synthesis of complex information, and the generation of predictive models.
- Digital professionals: civil servants with advanced digital skills who are likely to work on the development of AI solutions in government. Learning for this audience should address the technical aspects and implementation challenges associated with fostering AI innovation, and provide opportunities to collaborate with leading industries and academic institutions.
- Leaders: decision makers and senior civil servants who are responsible for creating an AI-ready culture in government. Learning for this audience should provide resources and workshops that are accessible to non-digital and data professionals and focus on the latest trends in AI, including its potential impact on organisational culture, governance, ethics and strategy.
The AI Policy Directorate in the Department for Science, Information and Technology (DSIT) has published, in collaboration with Innovate UK and the Alan Turing Institute, AI Skills for Business Guidance. While designed for business, this guidance offers a helpful overview of the knowledge and skills needed to harness the opportunities and navigate the practical challenges of AI.
Learning resources
We’ve launched a series of free online learning resources for all civil servants. The resources cover multiple topics:
- fundamentals of AI and generative AI, including their capabilities and limitations
- understanding AI ethics
- the business value of AI
- an overview of the main generative AI tools and applications
- courses on working with large language models, machine learning and deep learning, natural language processing and speech recognition and computer vision
- a technical curriculum with courses leading to certificates in different AI fields
AI courses are freely available within Civil Service Learning, as well as a series of AI courses from the Government Campus which can be accessed through the learning frameworks.
Senior civil servants, grade 7s and grade 6s, can also sign up to the AI course within The Digital Excellence Programme. This was designed by CDDO and the Government Skills and Curriculum Unit (GSCU) to support leaders outside of the digital and data profession to become pioneers of the government’s digital transformation.
Practical recommendations
- Make full use of the training resources available, including those on Civil Service Learning.
- Build a multidisciplinary team with all the expertise and support you need.
- Use multiple talent acquisition strategies and upskill current digital and data professionals in your team.
- Consider providing data, analytics and digital professionals with sandboxes and safe spaces to experiment with AI securely.
Working collaboratively
You need to work collaboratively to develop effective AI solutions. Your team should establish and maintain relationships with internal and external stakeholders with varying levels of familiarity with AI technologies.
You must be ready to collaborate with other teams in your organisation to address occasional knowledge and capability gaps in your team. Building AI solutions requires industry knowledge, whereas scaling and deploying these solutions will require considerations from software and/or technical infrastructure teams. Engaging these teams early on creates clear requirements and parameters for the proposed solutions, and sets a path for success.
To avoid siloed approaches and duplication of work, look for opportunities for cross-government and industry events that will help your team stay updated on the latest developments and best practices.
For example, consider joining the AI community of practice. This offers monthly meetings for people working on or interested in AI in the public sector. You can sign up to this community by using the dedicated form.
Depending on the nature of your project, you should also consider working with industry experts and academic institutions to foster knowledge exchange and access to cutting-edge research. Additionally, engaging with broader civil society will help you understand people’s values, concerns, and priorities, ensuring your AI solution meets the needs of the public we serve.
Defining the goal
Like all technology, using AI is a means to an end – not an objective in itself. Whether planning your first use of AI or a broader transformation programme, you should be clear on:
- the goals you want to achieve
- the needs of your users
- where you can most effectively use AI technologies, and where you should avoid them entirely
For example, you can use AI to automate and streamline processes, optimise the use of resources, foster data-driven decision making, and improve the quality and accessibility of some services. Goals for the use of generative AI may include improved public services or productivity, increased staff satisfaction, cost savings or risk reduction.
The sections below will help you understand when AI might be the right tool for your project.
User research for AI
User research (UR) can be critical to the success of your AI project. It is an important mechanism for engaging with people both inside and outside government to understand their needs, values and priorities. It provides key insights into the way the humans who will use the product or service behave, think and feel. This insight helps us ensure we use AI to deliver tangible benefits to individuals and society as a whole. It’s an exciting opportunity to collaborate on the design of an experimental approach, including the development of metrics, which will support the continuous improvement of your AI solution.
Doing UR for an AI project helps you keep the human in the loop and understand the human intelligence that the AI will replicate or imitate. Through UR, you can observe how people complete tasks and solve problems, understand their attitudes, and uncover the cultural issues that may impact the adoption and use of an AI solution. These insights will help you to identify where and how a human needs to be involved. You can find information about how to do user research in the government Service Manual.
Developing AI products and services involves some specific activities that user research can be particularly useful for. Some of these are critical to your AI project and some supplement the existing data science process. These include:
- understanding if AI is the right tool: do early UR to observe and speak with people who are involved in processes, whether these are internal users or the general public. This can help you understand users’ problems and aspirations. At this stage, a user researcher can work with a business analyst to find efficiencies in business processes and measure dissatisfaction. This will help establish a baseline of metrics to measure your project outcomes against. UR insights can also help you to work out an appropriate level of model accuracy when working with subjective outputs, and understand biases in the existing human process
- defining performance metrics: user researchers can work with analytical colleagues to use insights from research to define model and system metrics and methodologies for measuring ongoing performance
- preparing data: understand what data your users use, and how they think and talk about an activity. This will help you find, categorise and summarise appropriate training data. User researchers can help with supervised learning if you need human judgement to produce correct labelling of your training data. To consider how bias can affect labelling, refer to the Fairness, bias and discrimination section
- synthesising data: user researchers can help review and assess the appropriateness of generated synthetic data by developing and managing a process for bringing subject matter experts (SMEs) into the loop. If you’re planning to use synthetic data, check the UK Statistic Authority’s Ethical considerations relating to the creation and use of synthetic data
- evaluating your model’s output: as well as methods like statistical evaluation, it’s good practice to test the accuracy and relevance of your model’s outputs with a sample of your users. Methods such as reinforcement learning from human feedback (RLHF) are important to consider when training models – for example, when ranking different outputs. Humans can be particularly helpful when working on tasks that are difficult to specify but easy to judge, such as producing text that lacks bias, toxicity or other harmful content
- measuring usability of the product: observing how users respond to and use the outputs of your AI system will help you understand if they can use it to confidently complete their tasks. Design this testing into the way the AI solution is monitored, so that you can better understand data drift through user behaviour and changes over time
- understanding attitudes to the product or system: UR will help you understand levels of trust, confidence, and how the solution is used for decision making. These insights are useful to understand why model metrics might look good but service metrics are less positive. Model metrics measure how well your technology is performing, whereas service metrics help you understand if users’ needs and business goals are being met, which can be very different. For example, a machine learning (ML) algorithm could have a very high accuracy rate, but the users of the wider system could misinterpret its output and follow an unexpected course of action or completely ignore its recommendation through lack of trust
- accessibility: it’s particularly important to work out whether any users are being excluded from using your product or service, either intentionally or unintentionally. UR with a realistic sample can help you identify groups that may be excluded and why
- identifying how the system is being used: your AI solution may be used in ways you did not originally intend. Understanding why and what is happening can help you better meet the needs of your users and identify both risks and opportunities for innovation
- monitoring the AI solution while in service: building regular UR into how the solution is managed will help you continuously improve your product
The skills needed to collaborate on the steps above are within the usual skillset of most experienced user researchers. A user researcher on an AI project will need to:
- engage with the technology
- be adaptable in their approach
- design studies with technical and analytical colleagues
- use attitudinal methods
- understand how to do research that supports change management
- design studies to assess how user behaviour changes over time. For example, developing strategies for creating continuous feedback loops
- have experience doing generative research
- do concept testing
- understand privacy and data ethics
- be able to communicate in non-technical language to explain the AI system from a user perspective
Practical recommendations
- Consult the government Service Manual’s sections on user research.
- Get your organisation’s user researchers involved in the project from the start so that research and evaluation is designed into the way the AI solution is supported.
- Work with user researchers to design into the product or service ways of continuously assessing how effectively the AI model meets user needs.
- Read the example use case GOV.UK Chat: doing user research for AI products.
Identifying use cases for AI
When thinking about how your organisation could benefit from AI, consider the possible situations or appropriate use cases. This must be led by business and user needs, pain points and inefficiencies, not what the technology can do.
Once you’ve identified the challenges and opportunities through user research, focus on use cases that can only be solved by AI or where AI offers significant advantages over existing techniques.
You can do this by considering whether traditional solutions might be unable to handle the volume, complexity or real-time nature of the task; or whether the problem relates to, for example, advanced pattern detection in large data sets, automating complex, dynamic decision-making processes, or providing personalisation. Evaluate the potential impact of implementing AI solutions on these problems using tools like cost-benefit analysis to consider improvements in efficiency, accuracy or cost reduction.
You should also assess the feasibility of implementing AI in your team. Are the necessary skills and infrastructure in place to implement and maintain AI solutions? Would you need to train current employees, hire new talent or partner with AI technology providers?
When deciding if you’re going to use AI, you should also consider the capabilities and limitations of AI, the use cases to avoid, and discuss your project with technical experts.
To consider potential use cases of AI in your organisation, check the:
- examples of applications of AI in government
- appendix with case studies on AI projectsrecently developed in the public sector
- projects recorded in the Algorithmic Transparency Recording Standard (ATRS) register
Use cases to avoid
Given the current limitations of AI, and their ethical, legal and social implications, there are use cases that are not appropriate and which should be avoided in the public sector. These include but are not limited to:
- fully automated decision making: be cautious about any use case involving significant decisions, such as those involving someone’s health or safety. For more detail, refer to the section on Human oversight
- high-risk or high-impact applications: AI should not be used on its own in high-risk areas which could cause harm to someone’s health, safety, fundamental rights or the environment
Follow the principles of using AI safely and responsibly to check if your use case involves significant risks related to bias, fairness, transparency, privacy or human rights.
Practical recommendations
- Define clear goals for your use of AI, and ensure they’re consistent with your organisation’s AI roadmap.
- Select use cases which meet a clear need and fit the capabilities of AI.
- Find out what use cases other government organisations are considering.
Understand the limitations of AI and avoid high-risk use cases, considering the principles of using AI safely and responsibly.
Creating the AI support structure
To ensure your organisation adopts AI smoothly, consider how AI will change the way your people and processes work. Check that you have the structures in place to support its adoption.
These structures do not need to be fully mature before your first project. Your experience in your first AI project will shape the way you organise these structures.
However, you should make sure that you have sufficient control over how you use AI and ensure all AI systems operate in a safe and responsible environment. If you do not already have them in place, you should establish the following:
- AI strategy and adoption plan: a clear statement of the way that you plan to use AI within your organisation, including the impact on existing organisation structures and change management plans
- AI principles: a simple set of top-level principles which embody your values and goals, and that can be followed by all the people building solutions
- AI governance board: a group of senior leaders and experts to set principles and review and authorise uses of AI which fit these principles
- AI communication strategy: your approach for engaging with internal and external stakeholders to gain support, share best practice and show transparency
- AI sourcing and partnership strategy: a definition of which capabilities you will build within your own organisation, and which you will seek from partners
- AI training: resources that your team can use to upskill, based on a learning needs analysis
You should also consider using:
- a change management team: a small team with access to senior leadership that can shape your approach
- a use cases register: a way of capturing use cases and prioritising which you would like to explore first
- monitoring systems: to gather feedback and quickly identify emerging risks throughout change processes and for the duration of the system’s life cycle
- review and change processes: to provide staff with sufficient time, information and tools to identify and adapt to risks that emerge during a change process
- fallback processes: to ensure that critical functionality and services can be maintained if a change must be reverted and/or an AI system terminated
Practical recommendations
- Identify the support structures you need for your level of AI maturity and adoption, or reuse support structures already in place for other technologies.
- Design an appropriate method of capturing and prioritising opportunities based on feasibility and business value.
- Develop a communication strategy for engaging a wider community of leaders and staff to explain AI, demonstrate activity and reduce resistance to change.
Buying AI
While AI is not new, the AI supply market is evolving rapidly. It’s important that you engage with commercial colleagues to discuss partners, pricing, products and services.
The Crown Commercial Service (CCS) can guide you through:
- existing guidance
- routes to market
- specifying your requirements
- running your procurement
They can also help you navigate:
- running your procurement in an emerging market
- regulation and policy
- aligning procurement and ethics
AI business cases
Creating the business case for your AI project is an important stage in the process of AI adoption as it gives decision makers the opportunity to assess the return of investment in both resources and costs.
Before writing your business case, you should engage with stakeholders and discuss your project to understand if AI needs to be used, and, if so, which solutions offer the best improvements in productivity.
There are several resources you can use to write a strong business case. Consider following the Treasury’s Green Book (2022) guidance to create a fully fledged, five-part business case. This may be a requirement depending on the scale of your project and investment.
Your organisation will likely have investment thresholds. Typically, any investment approaching £10 million will require a five-part business case. You must use the Green Book when that is the case. If the scale of investment is below this threshold, you should strongly consider using the guidance on agile business cases, which was developed by the Central Digital and Data Office (CDDO).
When working on your business case, note that it’s mandatory to assure all non-business-as-usual spend above £100,000 for digital activities and £1 million for technology activities through your assurance boards. The process for doing so will be subject to your organisation’s requirements. Follow the Cabinet Office’s guidance on getting spend approvals.
In the following section, we summarise existing guidance on purchasing AI products and services. However, as the field is evolving rapidly this is not exhaustive, and engaging with commercial experts early in your project remains crucial.
Existing guidance
There is detailed guidance to support the procurement of AI in the public sector. You should familiarise yourself with this guidance and make sure you’re taking steps to align with best practice. The:
- Guidelines for AI procurement provide a summary of best practice when buying AI technologies in government, including preparation and planning, publication, selection, evaluation and award and contract implementation and ongoing management
- Digital, Data and Technology Playbook provides general guidance on sourcing and contracting for digital and data projects and programmes. All central government departments and their arm’s length bodies are expected to follow this on a ‘comply or explain’ basis. It includes specific guidance on AI and machine learning, as well as Intellectual Property Rights (IPR)
- Sourcing Playbook defines the commercial process as a whole and includes key policies and guidance for making sourcing decisions for the delivery of public services
- Knowledge asset management in government (The Rose Book) provides guidance on managing and exploiting the wider value of knowledge assets (including software, data and business processes). Annex D contains specific guidance on managing these in procurement
- Digital and technology spend control version 6 details in which instances your requirements will be subject to functional assurance of central government spending. Spend controls enable the UK government to achieve greater efficiency and better outcomes
- Procurement Policy Note (PPN) 02/24: Improving Transparency of Artificial Intelligence Use in Procurement provides optional questions to help identify suppliers’ use of AI in response to government procurements and in the delivery of services to government
Routes to market
Consider the available routes to market and commercial agreements and determine which one is most suitable based on your requirements.
However you decide to source your requirement, you must ensure you comply with procurement legislation. This is primarily the Public Contract Regulations 2015 and the Procurement Act 2023, which is set to come into effect in 2025. Commercial colleagues will be able to assist with this.
There are various routes to market to purchase AI systems. Depending on the kind of challenges you’re addressing, you may prefer to use a framework or a Dynamic Purchasing System (DPS). CCS offers several frameworks and DPSs for the public sector to procure AI.
A public-sector procurement route also exists, sometimes known as a ‘Find a tender service procurement’ due to the requirement to advertise on that platform. This may be appropriate for bespoke requirements or contractual terms, or where there is no suitable standard offering.
The table below summarises the differences between a framework agreement and a DPS. There’s more information on the CCS website and, on the use of frameworks, in the Digital, Data and Technology Playbook.
Category | Framework | DPS |
---|---|---|
Supplier access | Successful suppliers are awarded to the framework at launch. Closed to new supplier registrations. Prime suppliers can request to add new subcontractors. |
Open for new supplier registrations at any time. |
Structure | Often divided into lots by product or service type. | Suppliers filterable by categories. |
Compliance | Thorough ongoing supplier compliance checks carried out by CCS, meaning buyers have less to do at call-off (excluding G-Cloud). | Basic compliance checks are carried out by CCS, allowing the buyer to complete these at the call-off. |
Buying options | Various options, including direct award, depending on the agreements. | Further competition only. |
Note that a number of CCS agreements include AI within their scope.
Examples of DPSs include:
Examples of frameworks include:
- Big Data and Analytics
- Technology Products & Associated Services 2
- Technology Services 3
- Back Office Software
- Cloud Compute 2
In addition to commercial agreements, CCS has signed a number of Memorandums of Understanding (MoUs) with suppliers. These MoUs set out preferential pricing and discounts on products and services across the technology landscape, including cloud, software, technology products and services, and networks. You can access MoU savings through any route to market. To get support or find out more, email info@crowncommercial.gov.uk.
Specifying your requirements
When buying AI products and services, you’ll need to document your requirements to tell your suppliers what you need. To define what you need, you should engage with subject matter experts (SMEs) as soon as possible, and take time to consider the most appropriate type of AI solution for your project. This might be an off-the-shelf product, an existing technology with bolt-on AI elements (paid or free), outsourcing AI builds (if applicable), or co-creating AI with suppliers. The Digital, Data and Technology Playbook has guidance on commercial off-the-shelf (COTS) software licensing terms and build versus buy decisions.
When drafting requirements for AI, you should:
- start with your problem statement
- highlight your data strategy and requirements
- focus on data quality, bias (mitigation) and limitations
- underline the need for you to understand the supplier’s AI approach
- consider strategies to avoid vendor lock-in
- apply the Data Ethics Framework principles and consider the appropriate Data ethics requirements and questions (PDF, 62.7KB)
- mention any integration with associated technologies or services
- consider your ongoing support and maintenance requirements
- consider data format and provide suppliers with dummy data where possible
- provide guidance on budget to consider hidden costs
- consider intellectual property rights and who will have these if new software is developed
- consider any acceptable liabilities and appetite for risk, to match against draft terms and conditions, once provided
For more information, read the ‘Selection, evaluation and award’ section of the Guidelines for AI Procurement and CCS’s guide on How to write a specification.
Having prepared your procurement strategy, defined your requirements and selected your commercial agreement, you can proceed with your procurement. Your commercial team will lead this.
If you’re using an existing commercial agreement such as a framework or DPS, you’ll conduct a call-off in accordance with the process set out in the relevant commercial agreement. Non-framework or DPS procurements must comply with procurement legislation and relevant policy – for example, the Public Contract Regulations 2015, the Procurement Act 2023 and PPNs.
CCS offers buyer guidance tailored to each of its agreements, which describe each step in detail, including completing your order contract and compiling your contract.
Running your procurement in an emerging market
Commercial agreements
While AI is not new, it is an emerging market from a commercial perspective. As well as rapidly evolving technology, there are ongoing changes in the supply base and the products and services it offers. DPSs offer flexibility for new suppliers to join, which often complement these dynamics for buyers.
Any public sector buyers interested in shaping CCS’s longer term commercial agreement portfolio should express their interest by emailing info@crowncommercial.gov.uk.
Regulation and policy
Regulation and policy will also evolve to keep pace. However, there are already a number of legal and regulatory provisions which are relevant to the use of AI technologies. These include:
- A pro-innovation approach to AI regulation: government response: this details the government’s response to the white paper consultation published in March 2023, which set out early steps towards establishing a regulatory regime for AI, including 5 principles to guide responsible AI innovation in all sectors
- Portfolio of AI assurance techniques: this portfolio has been developed by the Responsible Technology Adoption Unit (RTAU), initially in collaboration with techUK. It’s useful for anybody involved in designing, developing, deploying or procuring AI-enabled systems. It shows examples of AI assurance techniques being used in the real world to support the development of trustworthy AI
- Introduction to AI assurance: this guidance has been developed by the Department for Science, Information and Technology (DSIT) to help private sector organisations better understand how to implement AI assurance to ensure the responsible development and deployment of AI systems. It’s designed to be accessible to a range of users, especially those who may not engage with assurance on a day-to-day basis. It introduces them to core assurance definitions and concepts and then how these can be applied to support the development and use of trustworthy AI
- AI Management Essentials: DSIT is developing guidance to support private sector organisations to engage in the development of ethical, robust and responsible AI organisational practice. This self-assessment tool will distil key principles from existing AI-related standards and frameworks and provide simple baseline requirements for government suppliers of AI products and services. After testing and consultation, DSIT is planning to work with the Cabinet Office to embed the tool in government procurement frameworks
This list is not exhaustive. For further guidance, refer to the Legal considerations section.
Aligning procurement and ethics
It’s important to consider and factor data ethics into your commercial approach from the outset.
There’s a range of guidance relating to AI and data ethics to support public servants working with data and/or AI. This guidance collates existing ethical principles, developed by government and public sector bodies. You can also refer to the:
- Data ethics framework: this outlines appropriate and responsible data use in government and the wider public sector. The framework helps public servants understand ethical considerations, address these within their projects, and encourages responsible innovation
- Data ethics requirements checklist (PDF, 62.7KB) for suppliers: this will mitigate bias. It will also ensure diversity in development teams, transparency and interpretability, and explainability of the results
- Public Sector Contract (PSC): this includes provisions related to intellectual property rights, data protection, and equality, diversity and human rights
Practical recommendations
- Engage your commercial colleagues from the outset. Understand and make use of existing guidance.
- Understand and make use of existing routes to market, including frameworks, DPSs and MoUs.
- Specify clear requirements and plan your procurement carefully.
- Seek support from your commercial colleagues to help navigate the evolving market, regulatory and policy landscape.
- Ensure that your procurement is aligned to ethical principles.
Using AI safely and responsibly
This section outlines the steps you’ll need to take to ensure that you build AI solutions in a safe and responsible way, taking account of legal considerations, ethics, data protection, privacy, security and governance.
Many of these considerations interact with each other, so you should read all of these topics together and seek support from data ethics, privacy, legal and security experts when planning and developing your project.
This section supports:
- Principle 2: You use AI lawfully, ethically and responsibly
- Principle 3: You know how to use AI securely
- Principle 4: You have meaningful human control at the right stage
- Principle 10: You use these principles alongside your organisation’s policies and have the right assurance in place
Ethics
The ethical and responsible use of AI is crucial for:
- maintaining public trust
- protecting individual rights
- fostering equitable societal progress
This is covered in the white paper A pro-innovation approach to AI regulation and its 5 principles to guide AI development in all sectors.
The ethical risks and opportunities presented by your use of AI will depend on your context and the nature of your solutions. The key themes you should address are:
- safety, security and robustness
- transparency and explainability
- fairness, bias and discrimination
- accountability and responsibility
- contestability and redress
- societal wellbeing and public good
The following sections explore these themes separately. However, there may be overlaps, with the impacts and outputs of one area introducing considerations in others. This means that, in some instances, the promotion of one ethical value may come at the cost of one or more other ethical values. For example, to promote fairness, you may need to collect demographic data to accurately assess the impact of a tool on different groups, which would have a detrimental impact on privacy. You must consider early on whether trade-offs are appropriate, if the benefits outweigh the risks, and that you’re avoiding any unacceptable risks.
Where possible, you should make specific and robust measurements to assess AI systems. These may, for instance, evaluate the accuracy and quality of a model’s outputs against the protected characteristics listed in the Equality Act 2010. You should select the most appropriate assessments for your technology and use case, being aware that the state of the art is rapidly developing.
As well as the guidance in this playbook, you can use the Data Ethics Framework and the UKSA ethics self-assessment (PDF, 449KB) guidance.
Safety, security and robustness
You must build safe, secure and robust AI solutions. This means that your AI systems must be resilient, sustainable and function reliably, even in unforeseen situations or against adversarial attacks.
Safety, security and robustness are important because they promote privacy rights, reduce harm, and uphold all the other ethical principles across the life cycle of your AI system.
Safety refers to a system’s ability to operate without causing harm to people or the environment. This is particularly important in high-risk areas such as healthcare, policing and justice.
Security refers to the protection of data, assets and functionality against unauthorised access, misuse or damage.
Robustness refers to the ability of your algorithm or model to maintain its performance and stability under different conditions. A core component of robustness is reliability: to be considered reliable, an AI system should consistently perform as intended across all expected scenarios within the system’s life cycle. You should therefore establish accuracy measures to ascertain whether the system is producing correct outputs. If a reliable system encounters an unexpected event, it should adapt or respond in a consistent way, minimising harm and providing teams with suitable warnings to respond and rectify issues.
Building safe, robust and secure AI solutions includes elements relating to privacy. You must understand that considerations of privacy extend beyond the requirements set out in the UK General Data Protection Regulation (UK GDPR), the Data Protection Act 2018 and other relevant legislation. For example, risks relating to group privacy, which consider the rights and interests of a group or collective rather than individuals, may include characteristics or behaviours that, if exposed, could lead to discrimination, stigmatisation or other forms of harm.
Wider themes relating to safety, security and robustness are described in the Data protection and privacy and Security sections.
Practical recommendations
- Establish performance metrics for AI systems that measure the accuracy of model outputs.
- Test your system in a variety of scenarios, including those capturing extreme or rare potential events.
- Implement red teaming processes and record how your model behaves when it encounters unexpected and anomalous scenarios.
- Make full use of the training resources available, including the courses on risks and ethics on Civil Service Learning.
Transparency and explainability
The AI regulation white paper establishes that AI systems should be appropriately transparent and explainable.
Transparency is the communication of appropriate information about an AI system to the right people – for example, information on how, when and for which purposes an AI system is being used. A lack of transparency can lead to:
- harmful outcomes
- public distrust
- a lack of accountability and the ability to appeal
You must consider transparency issues before deploying an AI system.
Explainability describes the ability to clarify how an AI system arrives at a given output or decision, such as explaining what factors lead to a loan application being granted or denied. Explainability may be impacted by the technologies used to build a system. You should consider this when designing your system.
Ensuring transparency and explainability can be challenging in the context of AI. Transparency can be limited by proprietary and ‘black box’ commercial tools, while explainability may not be possible for certain forms of machine learning, or may only be achievable at the cost of performance.
When checking the transparency of your AI systems, you should consider the:
- technical transparency: information about the technical operation of the AI system, such as the code used to create the algorithms and the underlying data sets used to train the model
- process transparency: information about the design, development and deployment decisions and practices behind your generative AI solutions, and the mechanisms used to demonstrate that the solution is responsible and trustworthy. Putting in place robust reporting mechanisms, process-centred governance frameworks and AI assurance techniques is essential for facilitating process-based transparency
- outcome-based transparency and explainability: the ability to clarify to any user using or impacted by a service that utilises AI how the solution works and which factors influence its decision making and outputs, including individual-level explanations of decisions where this is requested
- internal transparency: retention of up-to-date internal records on technology and processes, and process-based transparency information
- public transparency: communication about the department’s use of AI systems, made available to the general public in an open and accessible format
All central government departments and certain arm’s length bodies that are in scope of the Algorithmic Transparency Recording Standard (ATRS) must use it to ensure transparency around the algorithmic tools used in decision-making processes by public bodies. We also recommend ATRS for use by other public sector bodies, although they are not required to use it yet. You can refer to additional standards and external resources. These include:
- the UK’s national public sector AI ethics and safety guidance, understanding AI ethics and safety, which outlines a process-based governance framework that can help project teams establish and document proportionate governance actions
- data and model cards or fact sheets, which can be used as a reference point when documenting information about AI models and the data sets used in training and testing. A good example of these are Google’s data cards and model cards
- the Information Commissioner’s Office (ICO), which also offers AI auditing consultation and support to government organisations. Refer to A guide to ICO Audit: Artificial Intelligence (AI) Audits (PDF, 156KB) for more information
- Explaining decisions made with AI guidance, which is the UK’s national AI explainability guidance co-produced by The Alan Turing Institute and the ICO. This details 6 types of explanations as well as documentation processes
Practical recommendations
- Use existing standards and recording mechanisms such as the ATRS to communicate information about AI solutions to the general public. ATRS is particularly relevant if your tool is deployed, directly or indirectly, in decision-making processes.
- Clearly signpost when AI has been used to create content, is interacting with members of the public, or is used in decision-making processes that impact members of the public.
- Put in place evaluation and auditing structures, tracking data provenance, design decisions, training scenarios and processes.
- Implement transparency and auditing requirements for suppliers.
- Use external resources and emerging best practice, such as data cards and model cards for internal transparency.
- Make model outputs as explainable as possible, and avoid using less explainable AI methods in areas where explainability is essential.
- Consider the use of open source models, which provide more transparency about data sets, code and training processes.
Fairness, bias and discrimination
The white paper A pro-innovation approach to AI regulation sets out that AI systems should not undermine the legal rights of individuals or organisations, discriminate unfairly against individuals or create unfair market outcomes.
You must ensure fairness in the development and use of AI solutions to comply with legal and human rights requirements, including consumer and competition law, public and common law, and rules protecting vulnerable people. In the context of AI, fairness has many facets. It means ensuring that a system’s outputs are unprejudiced and do not amplify existing social, demographic or cultural disparities.
This includes ensuring that:
- AI systems fairly allocate resources or services to all people, across all protected characteristics
- certain subgroups are not disproportionately adversely impacted or harmed – for example, in employment-related decisions
- in the context of generative AI systems, different groups are not, for example, represented in harmful, prejudiced or offensive ways
You should understand that AI systems are designed, developed and deployed by human beings who are bound by the limitations of their contexts and biases. AI models are also trained on data which encodes present and past biases and inequalities of society. These can be present across the AI life cycle. Be aware that generative AI models are particularly vulnerable to bias because they’re trained on vast amounts of unfiltered data scraped from the internet, which is likely to contain a wide range of content reflecting historical and social biases. The wording of prompts may also inadvertently introduce bias.
A well-documented form of algorithmic bias is representational bias, which describes instances in which people are underrepresented, overrepresented or misrepresented in data sets used as training data. This form of biased input data can lead to the generation of harmful stereotypes or abusive content targeted at people from specific genders, sexual orientations and identities, ethnicities, countries of origin, or religions.
Another form of harm that can result from representational bias in the input data is performance disparities across different social groups. For example, a given system may perform more poorly on certain underrepresented dialects or skin colours. Other systems, such as AI applications that support healthcare, may provide beneficial results primarily to privileged members of society who are more heavily represented in the data.
Bias issues may be compounded when protected characteristics are considered in combination. The opacity and complexity of some AI systems can make it difficult to identify exactly where and how biases are introduced. Issues of fairness and bias may manifest when an AI system is implemented and used, even if issues were checked during system testing and validation. This may be due to a variety of reasons – from unexpected interactions by the users with software and hardware, to cultural or personal needs and specificities that were not captured earlier in the development process. For example, users may choose to ignore or only selectively pay attention to recommendations provided by an AI system, which may introduce new forms of bias.
It’s also important to consider accessibility, ensuring that equal benefits can be achieved by all and considering interactions with assistive technologies. You can find more on designing and testing for accessibility in the government Service Manual.
Practical recommendations
- Implement bias mitigation and fairness evaluation across the entire AI project life cycle.
- Comply with human rights law, the Equality Act 2010, the Public Sector Equality Duty and the Equality and Human Rights Commission guide to using AI in public services.
- Review model outputs and decisions for bias, measuring performance and decisions for different groups of people including intersectional groups.
- Put feedback mechanisms in place to allow individuals to report unfair decisions, harmful outputs and accessibility challenges.
- Beware of instances in which the use of AI can make legal obligations to the Equality Act 2010 more difficult to uphold – for example, difficulties with making links between abstract algorithmic groupings and protected groups.
- Adopt an approach of continuous evaluation to keep pace with changing fairness considerations and societal expectations.
Accountability and responsibility
Ensuring accountability and responsibility in the context of AI means that individuals and organisations can be held responsible for the effects that the AI systems they develop, deploy or use, have on people and society.
You must think about this at the start of your project to:
- encourage mindful creation and usage of AI systems
- ensure that people who design and deploy AI systems can be held accountable for their outputs and impacts
Accountability mechanisms will help you address and remediate issues when an error occurs. This involves establishing which parties are responsible at each stage of the system’s life cycle. Governance mechanisms may help to establish clear guidelines and structures for the development and deployment of AI systems. Refer to the Governance section for more information.
To establish accountable practices across the AI life cycle, you should consider 3 key elements: answerability, auditability and liability.
Answerability
You should establish a chain of human responsibility across the AI project life cycle, including responsibility throughout the supply chain. In cases of harm or errors caused by AI, you need to establish recourse and feedback mechanisms for affected individuals.
Identifying the specific actors involved in AI systems is vital to answerability. This includes model developers, application developers, policymakers, regulators, system operators and end-users. In each case, you must define their roles and responsibilities, and align these with legal and ethical standards.
Auditability
You should demonstrate the responsibility and trustworthiness of your development and deployment practices by:
- upholding robust reporting and documentation protocols
- retaining traceability throughout the AI project’s life cycle
Auditability is the process of documenting every stage of the AI innovation life cycle – from data collection and base model training to implementation, system deployment, updating and retirement – in a way that’s accessible to relevant stakeholders and easily understood.
Liability
You should make sure that all parties involved in the AI project life cycle, from vendors and technical teams to system users, are acting lawfully and understand their respective legal obligations.
As an end-user, being accountable means that you take responsibility for a system’s outputs and its potential consequences. You should check that outputs are accurate, non-discriminatory, non-harmful, and do not violate existing legal provisions, guidelines, policies or the provider’s terms of use.
You must also put the necessary oversight and human-in-the-loop processes in place to validate output in situations with high impact or risk. Where these risks are too high, you must reconsider if AI should be used at all. Refer to the Identifying use cases for AI section for more on this.
Ultimately, responsibility for any output or decision made or supported by an AI system always rests with the public organisation. Where AI is bought commercially, ensure that vendors understand their responsibilities and liabilities, put the required risk mitigations in place and share all relevant information.
Practical recommendations
- Follow existing legal provisions, guidelines and policies as well as the provider’s terms of use when developing, deploying or using AI.
- Clearly define responsibilities, accountability and liability across all actors involved in the AI life cycle. Where AI is bought commercially, define detailed responsibilities and liability contractually.
- Nominate a senior responsible owner (SRO) who will be accountable for the use of AI in a specific project.
- Where AI is used in situations of high impact or risk, establish a human-in-the-loop process to oversee and validate outputs and decisions. Make sure that these people can effectively identify risks and intervene, where appropriate.
- As an end-user, assume responsibility for the outputs and decisions made by the AI systems you use.
- Adopt a risk-based approach to the use of AI and consider whether it’s appropriate to use AI in high-risk applications.
Use assurance techniques to evaluate the performance of AI systems. The Introduction to AI assurance provides a useful starting point, and the Portfolio of AI assurance techniques offers real-world examples.
Contestability and redress
The principles of contestability and redress refer to the mechanisms through which AI systems and their outputs or decisions can be challenged, and how impacted individuals can seek remedy.
Contestability and redress are important because they help identify and correct ethical issues in AI systems after deployment. You must design appropriate mechanisms before deployment, and continue to maintain them throughout the full life cycle of your AI system.
These principles are interlinked with transparency and explainability requirements, as public awareness of the use of algorithms and effective explainability of AI systems are essential for questioning or challenging their use or outputs.
You should make people aware when an AI system is used, and clearly signpost mechanisms for contestability and redress to impacted individuals. These include:
- public awareness: to enable users to contest and seek redress about your AI system, you must ensure that they are aware of the presence of the AI system and the function that it plays in the services that they’re interacting with. This includes making users aware of mechanisms for contestability and redress clearly and in a timely fashion
- mechanisms for appeal: you must establish and promote clear and accessible mechanisms for people to challenge the decisions made by AI systems, and ask wider questions concerning the training, deployment and impacts of AI systems employed by the UK government
- change processes: you must ensure that mechanisms are in place to investigate any areas highlighted by users, and make changes to or decommission AI systems if unacceptable risks or harms are identified
Though contestability and redress mechanisms offer a route to mitigate harms, by themselves they do not sufficiently ensure the responsible use of these technologies, as harms may not be apparent to all who are impacted. Adhering to the principles discussed in the Using AI safely and responsibly section will help you identify risks before harms occur.
Practical recommendations
- Put mechanisms in place for users or impacted individuals to report instances of potential risk or harm relating to AI systems.
- Nominate one or more SROs to be responsible for monitoring reports and implementing adequate changes throughout the AI system’s life cycle.
- Ensure that routes for reporting risks or harms are clearly disclosed, and signposted to users at the point where they are, directly or indirectly, interacting with or impacted by an AI system.
- Where possible, provide impacted individuals with the option to contact the responsible team directly.
- For each project using AI, ensure you allocate adequate resources to respond to messages received through public communication channels and make changes where necessary across the life cycle of the system.
- Create contingency plans to maintain essential services in the event that an unacceptable risk is identified and the use of an AI system needs to be temporarily or permanently stopped.
Societal wellbeing and public good
Societal wellbeing in the context of AI means not only ensuring that AI is developed in a way that minimises and mitigates harms, but also actively promoting ethical applications of AI that solve societal challenges and deliver good for society.
AI may be perceived as an impersonal, distant, or even alienating technology. Government engagement with academia, industry and especially the broader civil society is crucial to dispel fears and foster understanding of the potential benefits of AI technologies.
The AI regulation white paper and the AI opportunities action plan highlight the potential for AI to deliver tangible benefits to members of the public and the economy. The applications of AI in government and the potential public benefits that we can achieve using AI are wide-ranging. AI technologies can be used to improve productivity and access to services; advance the effectiveness of health interventions and diagnoses; create and deliver more personalised training; promote sustainability (by supporting the work of conservationists, for example); and even reduce existing inequalities, for example by increasing access to health services for at-risk groups. By engaging in dialogue with civil society and acting as a leading, responsible user of AI, government can help the people of the UK better embrace AI’s potential for positive change.
However, AI systems and applications may have two-sided impacts. If not used in responsible ways and with appropriate safeguards in place, AI tools may risk generating harm, entrenching inequalities or undermining democratic processes. When considering the use of an AI system, you should identify and weigh both the potential positive impacts of new technologies as well as any negative impacts or unintended consequences.
You must ensure that AI systems generate a net positive impact on stakeholders and society at large, while minimising potential harms as much as possible. If the potential negative consequences are too high, you must consider terminating the project.
Some of the elements you should consider when assessing the potential impact of AI tools are:
- justified trust: this is essential to promote the uptake and long-term adoption of technology. AI can promote justified public trust in the government as a whole if the public understands and recognises that the AI solution has been developed competently, responsibly and ethically
- public benefit: you must ensure that the AI solutions you develop and/or use represent good value for money and benefit the public. This aligns with the UK government’s ambitions to use AI to help solve societal and global challenges – as long as the AI solution is safe, lawful and compatible with other ethical principles
- harm minimisation: illustrating public benefit is an important principle when evaluating the ethics of an AI project, but it’s equally important to minimise harms
- misinformation and disinformation: both unintentionally and intentionally, AI systems can be used to generate factually incorrect information. Some AI systems may facilitate the spread of misinformation or disinformation through their ability to generate plausible but false content, or by promoting this content online with recommender systems. This risk is particularly prevalent in generative AI systems, which are typically designed to create statistically likely language patterns rather than reliable accounts of reality. The ability of large language models (LLMs) to create text that appears credible and convincing enhances the potential for false or misleading information to be believed
- sustainability: AI can potentially provide a public benefit by helping meet sustainability goals. However, it can also present risks relating to energy and resource consumption derived from both the training and deployment of some AI technologies. If you plan to use generative AI tools, consider that it’s usually less environmentally sound to train your own model if appropriate pre-trained models are available. Generative AI can be expensive to operate, so it should not be used for tasks that could be undertaken by other available technologies
In addition to the points above, you can consider the ethics guidance from the Office for National Statistics to understand and articulate the potential public goods of your project.
Practical recommendations
- Engage with broader society – including civil society groups, underrepresented individuals, those most likely to experience harm, NGOs, academia, and more – when defining and deploying AI systems. Understanding their values, needs and priorities will help ensure your AI products deliver tangible benefits to society.
- Weigh any positive impacts of using an AI system against potential negative ones.
- Verify the information generated by AI systems to ensure it’s accurate.
- Assess the potential risks of your AI system being used to generate or spread misinformation or disinformation.
- Assess the environmental impact of training and/or deploying your AI system before commencing development. Consider whether the impact represents a reasonable trade-off between benefits and energy consumption, and whether a less energy-intensive system might be able to achieve the same or similar results. Also consider any actions you can take to make technology sustainable.
- Evaluate the environmental credentials of potential model providers and wider partner organisations – including their use of renewable energy, energy-efficient infrastructure and sustainable practices – and select low carbon emission energy grids.
Legal considerations
Different types of AI and use cases will likely create different types of legal issues. You’re not alone and should seek advice from government legal advisers who can help you navigate the design and use of AI in government.
Many of the legal issues that surround AI are not new. For example, the ethical principles discussed in this playbook, such as fairness, discrimination, transparency and bias, have sound foundations in public and other law. The ethical issues that your team identifies are also likely to be legal issues that your lawyers will be able to help guide you through.
The Lawfulness and purpose limitation section explains how to ensure that personal data is processed lawfully, securely and fairly at all times. Your lawyers can advise you on that.
You may face procurement and commercial issues when buying AI products. Alongside commercial colleagues, your lawyers can help you navigate those challenges.
When you contact your legal team, you should explain your aims for the AI solution, what it will be capable of doing, and any potential risks you’re aware of. This will help you to understand, for example, if you need legislation to achieve what you want to do.
It will also help to minimise the risk of your work:
- being challenged in court
- having unintended and/or unethical consequences
- having a negative impact on the people you want it to benefit
Example legal issues
These are designed to help you understand when you might want to consider getting legal advice. They should not be read as real legal advice and their application to any given scenario will depend on the specific facts. You should always consult your organisation’s lawyer.
Data protection
Data protection is a legal issue, with potentially serious consequences if the government gets it wrong.
Although your organisation will likely have a data protection officer, and there may also be data protection experts in your team, your legal team can help you unpick some of the difficult data protection issues that are created by AI.
Refer to the Data protection and privacy section for more information.
Contractual issues
Your lawyers will help you draw up the contracts and other agreements for the procurement or licensing of AI tools. There may be special considerations for these contracts. For example, how to:
- deal with intellectual property
- ensure the level of transparency needed to help buyers understand their systems
- transfer a project to new or successor suppliers
- assist with the defence against any legal challenge
Contracts for technology services may need to incorporate procedures for system errors and outages that recognise the potential consequences of performance failures.
It’s important that you consider appropriate contractual terms early on because this may, in part, drive decisions on the appropriate route to market. Refer to the Buying AI section for more information.
Intellectual property, including copyright
The potential intellectual property issues with AI have been much discussed. Your lawyers can help you navigate these.
For example, you should consider at the outset:
- which parties will own which parts of any intellectual property generated during the project
- which parties will have ongoing rights to use any intellectual property that is generated (and on what basis)
- how the balance of risk and liability should be determined between the parties, as this will be relevant to any claims for infringement of third party intellectual property
Equality issues
Lawyers can help you navigate the equality issues raised by the use of AI in government – for example, obligations arising under the Equality Act 2010 and the Public Sector Equality Duty. Conducting an assessment of the equality impacts of your use of AI can also be one way to guard against bias, which is particularly important in the context of AI.
If approached early, before contracts are signed, your legal advisers can help you ensure the government is fulfilling its responsibilities to the public to assess the impacts of the technology it’s using.
Public law principles
Public law principles explain how public bodies should act rationally, fairly, lawfully and in compatibility with human rights. These are guidelines for public bodies on how to act within the law.
Many of these public law principles overlap with the ethical principles set out in this guidance. As a result, your lawyers will likely be able to guide you on how to apply the ethical principles based on their knowledge of public law, the court cases that have occurred and the detail of the judgments.
For example, public law involves a principle of procedural fairness. This is not so much about the decision that is eventually reached but about how a decision is arrived at. The transparency and explainability of the AI tool may well be key in being able to demonstrate that the procedure was fair. Similarly, an inability to determine how AI tools have arrived at their decisions or outputs may introduce risk into the decision-making process.
Public law also considers rationality. Rationality may be relevant in testing the choice of an AI system, considering the features used in a system, and considering the outcomes of the system and the metrics used to test those outcomes.
If you’re considering using AI in decision making, public law can also guide you. For example, it can help you determine whether a particular decision should be delegated to a decision maker, rather than letting an AI tool make an automated decision. When operating in a regulated environment, such as a procurement process, automated decision making or assessments could be subject to legal challenge if procedural fairness, lack of bias and rationality cannot be evidenced.
Human rights
Public authorities must act in a way that is compatible with human rights. It’s possible that AI systems (especially those involving the use of personal data) may in some way affect at least one of an individual’s rights, as set out in the European Convention on Human Rights (ECHR). Examples of the rights most likely to be impacted are Article 8 (right to a private and family life) and Article 10 (freedom of expression).
Legislation
Sometimes, in order to do something, a public authority needs a legislative framework. Your lawyers will be able to advise you whether your use of AI is within the current legal framework or needs new legislation.
For example, the legislative framework might not allow the process you’re automating to be delegated to a machine, or it might provide for a decision to be made by a particular person.
Practical recommendations
- Ensure you engage legal professionals at the outset of your AI project. They can help you navigate legal complexities and identify potential legal risks associated with data protection, contractual agreements, intellectual property, equality issues, and compliance with public law principles.
- Given the potential consequences of mishandling personal data, collaborate with legal experts to ensure you comply with data protection regulations and understand how to mitigate risks associated with data privacy and security.
- Work with legal experts to develop robust contracts and agreements for procuring or licensing AI tools, considering issues such as intellectual property rights, transparency levels, liability distribution, and procedures for addressing system errors or failures.
- Seek legal advice to determine whether your AI project aligns with existing legislative frameworks or requires new legislation. Understanding legislative constraints helps mitigate the risk of legal challenges and ensures you comply with legislative requirements.
Data protection and privacy
AI-driven technologies offer significant benefits but they also pose potential risk of harm to individuals and groups if they’re not implemented with specific focus on protecting individuals’ personal data and right to privacy.
Be aware that organisations developing and deploying AI systems must consider the principles of data protection outlined in the UK General Data Protection Regulation (UK GDPR) and the Data Protection Act 2018, and minimise the risk of privacy intrusion from the outset.
The UK data protection law applies irrespective of the type of technology used, so its basic principles of compliance will also apply to any AI system. The data protection principles most relevant to the use of AI are:
- accountability: your organisation has clear ownership of risk and responsibility for mitigations and compliance
- lawfulness: you have an applicable lawful basis for processing personal data and ensure the processing is lawful under data protection or any other regulation
- purpose limitation: you define why you’re processing personal data and only process data for that purpose
- transparency and individual rights: you’re open about what it uses personal data for, and your users can exercise their information rights
- fairness: you avoid processing personal data in ways that are detrimental, unexpected or misleading
- data minimisation: you develop systems that process only the data that is needed for the task at hand
- storage limitation: you don’t accumulate large amounts of personal data for unjustifiably long periods
- human oversight: you build in human oversight to automated decision making
- accuracy: you have steps in place to ensure the accuracy of AI-generated responses and data related to individuals
- security: you implement appropriate technical and organisational mitigations to protect sensitive and personal data
Data protection and privacy considerations require specialist expertise, so it’s crucial to involve relevant data protection, legal and other information governance professionals in AI projects from the outset to follow data protection by design principles.
Accountability
Accountability is a key principle that establishes ownership of risk, responsibility for mitigations, compliance with legislation, the ability to demonstrate compliance, and high standards for privacy.
Organisations should take the following steps when planning AI solutions:
- make a strategic decision on how any use of AI technology fits with your existing risk tolerance
- review your risk governance model to establish clear ownership of AI risks at a senior level
- implement measures to mitigate these risks and test their effectiveness
- make sure you identify residual risks and align them with your organisation’s risk threshold
- be collaborative, work transparently and demonstrate how you mitigate risks
- due to the evolving nature of AI technologies and new regulations, ensure you conduct regular reviews, with a view to making further iterations
- engage with internal data protection, privacy and legal experts from the outset
Data protection by design is an important component of the UK GDPR risk-based approach. It requires you to integrate data protection safeguards into personal data processing activities throughout the AI product life cycle.
This will ensure that you implement appropriate technical and organisational measures to protect data subject rights, and comply with the data protection principles defined in the UK GDPR and Data Protection Act 2018.
Practical recommendations
- Establish ownership of AI risks at a senior level.
- Integrate oversight of AI into your governance processes.
Take a risk-based approach, defining risk appetite and following principles of data protection by design and by default.
Lawfulness and purpose limitation
Before implementing AI solutions, you need to undertake a data protection impact assessment (DPIA). This involves an assessment of data protection and privacy risks, and the implementation of appropriate technical and organisational measures to sufficiently mitigate them.
Article 35(3)(a) of the UK GDPR requires you to undertake a DPIA if your use of AI involves any of the following:
- systematic and extensive evaluation of personal data aspects based on automated processing, including profiling, on which decisions are made that produce legal or similarly significant effects
- large-scale processing of special categories of personal data
- systematic monitoring of publicly accessible areas on a large scale
The [Information Commissioner’s Office (ICO)](https://www.gov.uk/government/organisations/information-commissioner-s-office#:~:text=The%20Information%20Commissioner’s%20Office%20(ICO,for%20Science%2C%20Innovation%20and%20Technology.) also requires a DPIA if your processing of personal data involves the use of innovative technologies. In your DPIA, you should take all of the actions below.
- Describe the purpose of personal data processing activities.
- Assess the necessity and proportionality of personal data processing.
- Identify all personal data, including special category data, that is being processed, including sources and flows of data.
- Identify the valid lawful basis under Article 6 (and any additional special conditions under Article 9 for special category data) of the UK GDPR.
- Identify your organisation’s role and obligations as a data controller and whether any data processors are involved.
- Identify the stages when AI processes and automated decisions may have an impact on individuals.
- Seek and document the views of individuals whose personal data is being processed. This includes finding out whether data subjects are aware that this processing is taking place.
- Identify the stages when any human is involved in the decision-making process.
- Consider any potential detriment to individuals due to bias or inaccuracy.
- Document measures and safeguards put in place, and any residual levels of risk posed by the processing.
The purpose for which data is collected and used has a significant effect on whether individuals perceive it as being invasive to privacy. A clear and well-defined articulation of the purpose from the outset will guide your deliberations about an applicable, lawful basis and the minimum personal data that is absolutely necessary to deploy the AI service.
AI systems often reuse personal data for new purposes that are different from those for which it was originally collected. This may cause tension with the purpose limitation of the UK GDPR. Repurposing of personal data is only legitimate if a new purpose is ‘compatible’ with the purpose for which the data was originally collected.
You should consider the following criteria when repurposing personal data:
- whether the new purpose aligns with the data subjects’ expectations
- what type of personal data is involved
- what potential impact it will have on data subjects’ interests
- whether the data controller will need to adopt additional safeguards to ensure fairness and transparency
The DPIA process should identify personal data processing at each stage of the AI life cycle – from design to data acquisition and preparation, training, testing, deployment and monitoring. Although it’s common to characterise AI with large volumes of data, AI systems are able to directly perceive and evaluate their environment, and adapt to the data received. You should not underestimate AI’s interactive qualities, such as its ability to collect new data in real time from touchscreens and audiovisual inputs, and adapt its responses and subsequent functions based on these inputs.
When mapping personal data flows, you should identify the geographic location of each distinct processing activity because the processing of data outside the United Kingdom will increase the risk of losing the protection of UK data protection laws. Data controllers may need to bring in additional safeguards, such as international data transfer agreements if personal data is being processed in jurisdictions where the data protection regime is not deemed to be adequate and transfers of personal data are restricted under Article 46 of the UK GDPR.
If your assessment indicates that there’s a high risk to the data protection rights of individuals, and that you’re unable to sufficiently reduce these risks despite mitigating actions, you must consult the ICO before you can start processing personal data.
Practical recommendations
- When building your team, seek support from data compliance professionals – including data protection, legal and privacy experts.
- Identify data processing operations and their purpose, and map personal data sources and flows.
- Determine whether personal data is necessary for each activity, and whether you’re processing special category data or children’s data.
- Identify the applicable lawful basis of your data processing and assess data protection and privacy risk through DPIAs and legitimate interest assessments.
- If data protection and privacy risks remain high even after mitigations, consult with the ICO.
- Identify any processing outside the UK to take additional safeguards to protect personal data in jurisdictions where the data protection regime may not be adequate.
- Assess any changes in the purpose of your AI system and make sure your AI system remains compliant and lawful.
Transparency and individual rights
In addition to the ethical reasons for seeking transparency, organisations need to be transparent about how they process personal data in an AI system so that individuals can effectively exercise the rights granted to them by the UK GDPR.
The UK GDPR requires data controllers to:
- provide information to users in a concise, transparent, intelligible and easily accessible form using clear and plain language
- be transparent about the purpose for processing personal data, retention periods and third parties involved in the processing activity
- be transparent about the existence of automated decision making, providing meaningful information about the logic involved, and about the significance and envisaged consequences for the data subject of processing in this way
- provide a clear explanation of the results these systems produce
- uphold individuals’ rights, including the right of access to the personal data that you hold on them, and have a simple and clear process to exercise their right to correction and to object to the processing of their personal data at any time
The transparency principle applies to personal data collected from all sources, including the interactive qualities of AI systems that have the ability to collect new data, which may include text and audiovisual inputs. For example, if you’re using facial recognition technology for public area monitoring, you need to be transparent by clearly informing data subjects. You can do this with clear signage and information on relevant data controllers, what information is collected, the purpose and legal basis of processing, and for how long the data is kept.
Practical recommendations
- Explain your system in plain English.
- Be transparent about the purpose for processing personal data, retention periods and third parties involved in the processing activity.
- Be transparent about the existence and nature of automated decision making, using the Algorithmic Transparency Recording Standard (ATRS) where required or on a voluntary basis as best practice.
Provide a clear explanation of the results these systems produce, following guidance such as the ICO’s Explaining decisions made with AI.
Fairness
Fairness in processing is another principle under the UK GDPR which applies to AI systems that process personal data. In the context of data protection legislation, fairness means that ‘you should only process personal data in ways that people would reasonably expect and not use it in any way that could have unjustified adverse effects on them’.
DPIAs are the main tool to help you consider the risks to the rights and freedoms of individuals, including the potential for any significant social or economic disadvantage. DPIAs also help demonstrate whether your processing is necessary to achieve your purpose, proportionate and fair.
The Responsible Technology Adoption Unit (RTA) in the Department for Science, Information and Technology (DSIT) published the results of its Public Attitudes to Data and AI survey in December 2023. This report found that people’s comfort with the use of AI greatly depends on the specific context. Perceptions of the need for AI governance also vary considerably by sector, with a substantial proportion of the public prioritising careful management of AI used in healthcare, by the military, or in banking and finance.
You must make sure that AI systems do not process personal data in ways that are unduly detrimental, unexpected or misleading to the individuals concerned. If AI systems infer data about people, you need to ensure that the system is accurate and is not discriminatory. You need to uphold the ‘right to be informed’ for individuals whose personal data is used at any stage of the development and deployment of AI systems. This is part of fulfilling the transparency and fairness principles.
Data protection aims to protect individuals’ rights and freedoms with regard to the processing of their personal data, not just their information rights. This includes the right to privacy but also the right to non-discrimination. For example, computer vision technologies such as facial recognition have raised concerns due to the risk of errors in matching faces. This technology has proven to be less accurate when used on women and people of colour, producing biased results. Ultimately, this can create discrimination, raising fundamental rights concerns because of the disadvantage to some individuals whose facial images are captured and processed.
People’s facial images constitute biometric data. This is personal data because it’s the result of specific technical processing related to physical, physiological or behavioural characteristics of a natural person, which can confirm the unique identification of the person. Facial images may fall into the special categories of personal data because they’re likely to reveal sensitive characteristics such as racial or ethnic origin, and so require enhanced protection and additional safeguards.
Biometric data is also considered special category data when processed for the purposes of identification. You must ensure that the technologies used to capture and process this data are overt, accurate, proportionate, fair and deploy a narrow ‘zone of recognition’. For example, if someone walks past a camera and their image does not meet the threshold for a potential match, their data needs to be promptly deleted.
Practical recommendations
- Identify the risks to the rights and freedoms of individuals through DPIAs and assess whether your processing is necessary, proportionate and fair to achieve your purpose.
- Use the ICO’s AI data protection and risk toolkit to reduce the risks to individuals’ rights and freedoms.
- Mitigate risks using the ICO’s guidance on fairness in AI systems.
- Provide users with clear reassurance that you’re upholding their right to privacy, including simple processes to exercise their rights in clear privacy notices.
- Address any objections from users – for example, related to solely automated decisions, or where there’s a significant legal impact – by implementing safeguards such as meaningful human intervention, or an effective process to obtain and consider individuals’ views and corrections of factual errors.
Data minimisation
The data minimisation principle requires you to identify the minimum amount of personal data you need to fulfil your purpose, and to only process that information and no more. This does not mean that AI tools should not process personal data, but if you can achieve the same outcome by processing less personal data then, by definition, the data minimisation principle requires you to do so.
Retaining data that is not strictly necessary is a risk to the individuals from whom the data is derived. Excluding irrelevant data prevents algorithms from identifying correlations that lack significance or are coincidental. There are a number of techniques that you can adopt to develop AI systems that process only the data you need, while still remaining functional.
For example, you can consider using privacy-enhancing technologies (PETs) to offer stronger protections and preserve data privacy while enabling effective use of data. Some PETs provide new tools for anonymisation, and some enable collaborative analysis on privately held data sets, allowing data to be used without disclosing copies of the data. PETs are multi-purpose: you can use them to reinforce data governance choices, or as tools for data collaboration and greater accountability through audits. A data-focused example solution is to create ‘synthetic data’. This is an artificial data set that does not include any actual data on ‘real’ individuals but mirrors in characteristics and proportional relationships all statistical aspects of the original data set.
Practical recommendations
- Justify your use of personal data, using your DPIA to think about the problem you’re solving so that you settle with the minimum personal data that’s required. Less personal data means less risk.
- Reduce the risk of individuals being identified through the processing of their personal data by using appropriate de-identification techniques (such as redaction, pseudonymisation and encryption).
Refer to the ICO guidance on privacy-enhancing technologies (PETs).
Storage limitation
The UK GDPR states that you should only hold personal data as long as you can reasonably justify it for the purpose of your processing, and that you should not retain personal data longer than you need it. Think through:
- what personal data the technology will hold
- why you have it and what it’s used for
- whether you can justify keeping it for that period of time
You should map all personal data flows through every stage of development, testing and deployment, and utilise data minimisation, anonymisation techniques and eventual deletion to irreversibly transform or remove personal data.
Practical recommendations
- Use data minimisation and anonymisation techniques (PDF, 325KB) as needed to remove or irreversibly transform personal data where possible.
- Be transparent about the length of personal data retention in privacy notices.
Human oversight
Although it is possible to use AI systems for automated decision making where the system makes a decision automatically without any human involvement, this may infringe the UK GDPR. Article 22 currently prohibits decision(s) based solely on automated processing that have legal or similarly significant consequences for individuals. Services using AI that affect a person’s legal status or their legal rights must only use AI to support decisions that must be made by a human decision maker.
AI systems need to introduce deliberation processes into all stages of the life cycle so that the abilities of humans and machines are combined to reach the best results when performing tasks. However, the human input needs to be ‘meaningful’. Several factors determine how much human involvement there should be in AI systems, such as the complexity of the output, its potential impact, and the amount of specialist human knowledge (for example, legal and medical) required.
Practical recommendations
- Design, document and assess the stages when meaningful human review processes are incorporated and what additional information will be taken into consideration when making the final decision.
Use the ICO guidance on automated decision making under UK GDPR for more clarity on types of decisions that have a legal or similarly significant effect.
Accuracy
Accuracy in the context of data protection requires that personal data is not factually incorrect or misleading, and, where necessary, is corrected, deleted and kept up to date without delay.
You should not treat AI outputs as factual information about the individual, but instead consider these as a ‘statistically informed guess’. You also need to factor in the possibility of outputs being incorrect and the impact this may have on any decisions.
You need to make it explicit that the outputs of your AI systems are statistically informed guesses rather than facts, including information about the source of the data and how the inference has been generated.
Practical recommendations
- Test AI outputs against existing knowledge and expertise during training and testing.
- Be transparent that outputs are statistically informed guesses rather than facts.
- Document the source of the data and the AI system used to generate the conclusion.
- Implement processes to consider individuals’ feedback, views and corrections of factual errors.
Security
Cyber security is a primary concern for all government services, as laid out in the Government Cyber Security Strategy. When building and deploying new services, including AI systems, the government has a responsibility to make sure these are secure to use and also resilient to cyber attacks. To meet this requirement, your service must comply with the government’s Secure by Design principles before it can be deployed.
There are some security risks that apply uniquely to AI and/or generative AI technologies. This section takes you through some of these risks to help you keep AI solutions in government secure.
To learn more about AI security, you’re encouraged to join the cross-government AI security group that brings together security practitioners, data scientists and AI experts. Please note that only those with GOV.UK email addresses can currently join this group.
How to deploy AI securely
Depending on how AI systems are used, they can present different security challenges and varying levels of risk that must be managed. This section covers some of the approaches that you need to take for:
- public AI applications and web services
- embedded AI applications
- public AI application programming interfaces (APIs)
- privately hosted open source AI models
- working with your organisational data
- open-source vs closed-source models
Public AI applications and web services
A simple way to implement an AI solution is to use publicly available commercial applications – such as Google Gemini or ChatGPT in the case of generative AI. While you might think that these public tools are more secure, you should consider that you cannot easily control the data input to the models: you must rely on educating users on what data they can and cannot enter into these services.
You also have no control over the outputs from these models, and you’re subject to their commercial licence agreements and privacy statements. For example, OpenAI will use the prompt data you enter directly into the ChatGPT website to improve their models, although individual users can opt out. When using public AI applications, you must not enter official information unless it has been published or is cleared for publication.
Embedded AI applications
Many vendors include AI features and capabilities directly within their products, for example Slack GPT and Microsoft Copilot. While this guidance applies at a high level to each of these applications, they come with their own unique security concerns. Before adopting any of these products it’s important to understand the underlying architecture of the solution, and what mitigations the vendor has put in place for the inherent risks associated with AI.
In addition to embedded applications, there are also many AI-powered plugins or extensions to other software. For example, Visual Studio Code has a large ecosystem of community-built extensions, many of which offer AI functionality. You must take extreme caution before installing any unverified extensions as these can pose a security risk.
There has also been a proliferation of AI transcription tools that are capable of joining virtual meetings and transcribing meeting notes. These present a serious risk of data leakage as they silently upload meeting recordings to an AI service for transcription and analysis. When hosting virtual meetings, organisers should verify the identity of all attendees and state up front that the use of third-party meeting transcription tools is not allowed.
You should always speak with your security team to discuss your requirements before deploying any embedded AI applications, extensions or plugins.
Public AI APIs
Many public AI applications offer the ability to access their services through APIs. By using the API you can integrate AI capabilities into your own applications, intercept the data being sent to the AI model, and also process the responses before returning them to the user.
For example, when integrating a large language model (LLM) through an API you can include privacy-enhancing technology (PET) to prevent data leakage, add content filters to sanitise the prompts and responses, and log and audit all interactions with the model. Be aware that PETs come with their own limitations, therefore selection of the PET should be proportionate to the sensitivity of the data.
Refer to the Information Commissioner’s Office (ICO)’s guidance on privacy-enhancing technologies (PETs) and the Responsible Technology Adoption Unit (RTA)’s PET adoption guide for more information. Consider also that your data is still passed over to the provider when you use an API, although retention policies tend to be more flexible for API use. For example, OpenAI only retains prompt data sent to the API for 30 days.
Privately hosted AI models
Instead of using a public AI offering, the alternative is to host your own AI model. This could be a model taken from one of the many publicly available pre-trained open source models or it could be a model you have built and trained yourself. By running a model in your own private cloud infrastructure, you ensure that data never leaves an environment that you own.
In the case of generative AI, the models you can run in this way are not on the scale of the publicly available ones, but can still provide acceptable results for certain applications. The advantage is that you have complete control over the model and the data it consumes. The disadvantage is that you’re responsible for ensuring the model is secure and up to date. Consider that you must also maintain the infrastructure to host your model, which brings additional costs along with the specialist skills you’ll need in machine learning (ML) operations.
Managed machine learning model hosting platform
An alternative approach to setting up the infrastructure to host your own model from scratch, is to use a fully managed ML model hosting platform. For example, Amazon Bedrock and IBM watsonx.ai allow you to host different open source or commercially available AI models and compare their performance, while Microsoft Azure OpenAI service offers access to the OpenAI GPT models but running in a private instance with zero-day retention policies.
Running AI models locally
Many open source AI models are capable of being run locally on a single machine. This is often attractive because it allows the models to be run in isolation, with limited or no network access. This type of deployment is not recommended for most production services, but might be appropriate for ad-hoc or one-off applications where performance of the model is not paramount.
Training AI models
In addition to where your AI model runs, you should also consider how it was trained because this is important from a security perspective. For example, many of the publicly available generative AI models were trained using data from the public internet. This means that they could include data that is personally identifiable, inaccurate, illegal or harmful, any of which could present a security risk.
It’s possible to train an AI model using your own data, and for many specific and limited tasks this is often the most appropriate approach because it gives you complete control of the training data. For generative AI models, the cost of doing this for larger and more capable systems is prohibitive and the amount of private data required to produce acceptable performance of a large model is beyond the capacity of most organisations. You should assume that any data you use to train your model could be extracted by an attacker. There’s more about this in the Data leakage section.
Working with your organisational data
A key application of AI is working with your organisation’s private data. By enabling the model to access, understand and use this data, insights and knowledge can be provided to users that are specific to their subject domain and will provide more reliable results. For a standard ML model, you can train them directly with your private data set. For generative AI models, you can fine-tune them or use approaches like retrieval augmented generation (RAG) to augment the model with your private data.
If you use your own data with an AI model, you immediately increase the data security risk and you need to apply additional security controls to stop data leakage and privacy violations.
Questions you should consider when using your own private data with an AI model include:
- where is your data being sent and how is it being processed?
- is your data being used to train future models?
- how long is your data being retained?
- is your data being logged and who has access to those logs and for what purpose?
Open-source vs closed-source models
Neither open-source or closed-source AI models are inherently less secure than the other. A fully open-source model may expose not only the model code, but also the weights of its parameters and the data used to train the model. While this increases transparency, it also potentially presents a greater risk, as knowing the weights and the training data could allow an attacker to create attacks carefully tailored to the specific model.
One benefit of fully open-source models is that they allow you to inspect the source code and model architecture, enabling security experts to audit the code for vulnerabilities. Despite this, because of its complexity, even an open source generative AI model remains mostly opaque and hard to analyse.
Security risks
AI security risks are divided into 2 main categories: the risk of using AI and the risk of adversaries using AI against you.
Using AI
There are many resources you can use to explore the risks of using AI:
- the National Cyber Security Centre (NCSC) has published a set of principles around securing machine learning (ML) solutions
- Microsoft has compiled a list of ML failure modes
- MITRE Adversarial Threat Landscape for AI Systems (ATLAS) matrix is an open source knowledge base of techniques used to attack AI systems
- International Scientific Report on the Safety of Advanced AI has an analysis of risks posed by general purpose advanced AI systems
- the Open Worldwide Application Security Project (OWASP) has done significant work to identify the unique risks posed by LLMs – these risks focus on the use of LLMs but many of them will also apply to other types of generative AI models and more widely to AI in general
From a combination of these sources, we can draw out some of the most common vulnerabilities and discuss them in context of AI applications in government.
Data and model poisoning
This is when data used to train an AI model has been tampered with, leading the model to produce incorrect or harmful output.
Attackers can target the data used to train an AI model to introduce vulnerabilities, backdoors or biases that compromise the model’s security and behaviour.
For a traditional ML model that uses a limited amount of training data for a specific task, this type of attack can be prevented by training the model yourself on a known data set that you control.
For frontier generative AI models, the barrier to entry for training a model from scratch is high and fine-tuning an existing model is much easier and cheaper. There are many open source models that are easy to fine-tune (for image generation, for example), and these can and are used to produce specific types of outputs, some of which are harmful or illegal.
When using an AI model – particularly a specialised, fine-tuned model – from a third-party source, it’s difficult to ascertain if it has been tampered with. Poisoned models may appear to be functioning as expected until a specific prompt triggers the malicious behaviour.
Supply chain vulnerabilities of this kind are not unique to AI. For example, when software libraries are hacked, all downstream systems that depend on those libraries are affected – a notable example of this was the Faker NPM hack. Many automated tools exist for detecting, tracking and fixing security issues with open source software, but the current tooling for doing this with open source AI models is much more limited. Popular open source AI model site Hugging Face does have some malware scanning tooling, but this is not capable of determining if a given AI model has been trained on poisoned data.
AI model hosting platforms like Microsoft’s Azure AI, Amazon’s Bedrock and IBM’s watsonx.ai allow developers to use commercial models and other third-party AI models. These services do not make any guarantees about the security and integrity of the third-party models that they’re capable of hosting.
Training data can also be poisoned indirectly through the introduction of malicious data into known collections of open data that are used to train or fine-tune frontier generative AI models. This is likely to become an increasing threat as hackers learn which publicly available data sets (for example, Wikipedia or Reddit) have been used to train generative AI models, and target these to poison future versions of the models.
The impacts of an AI model trained or fine-tuned with poisoned data are wide ranging, including direct security threats to the organisation running the model and biased or harmful outputs to the users of the model. Poisoned models could push people to particular products or subvert confidence in government services.
To help detect and prevent data poisoning, you’ll need to make sure your users and developers are trained on the risks and aware that the results from AI models can be false or biased. Outputs of models should be tested against known good responses and should be systematically tested for biases. ML hosting platforms often include evaluation tools that can measure and test the performance of an ML model, such as Google’s Vertex AI or Microsoft’s Prompt Flow. Improved explainability of the models themselves would also help, enabling the output to be traced back to the source training data. For more information, refer to the Transparency and explainability section.
Data leakage
This is when responses from an AI model reveal confidential information, such as personal data.
AI models are trained using data. In the case of generative AI, data is commonly taken from the public internet and will contain personal data and other confidential information. AI models can suffer from data leakage, depending on their intended mode of operation. For example, a model that is being used as a classifier to rank or sort data into groups based on criteria would necessarily be less likely to leak training data than a generative AI model, as its outputs are confined to the specific classification problem. However, if an AI model has been trained or fine-tuned with private data that has different levels of security controls based on the user who should be seeing it, for example documents that are restricted to a specific group of people, there is currently no way to preserve these controls when training the model.
Generative AI models can also be made to reveal their original training data through their responses, meaning that any outputs from a generative AI model could potentially contain confidential information. For generative AI, a way to preserve user access controls is to use ‘in-context learning’. A search is carried out first on the private data a user is permitted to see, and the retrieved results are passed in context to the generative AI model. This type of approach is known as retrieval augmented generation (RAG), and is used in many commercial generative AI tools (such as Microsoft Copilot).
In-context learning has limitations and can degrade the performance of a generative AI model in certain applications. RAG tools are susceptible to indirect prompt injection, either in the information retrieved by the initial search or through the user prompt, meaning that security controls could be circumvented and private data could still be leaked.
You should make sure your AI model only has access to the data the user of the model should be able to access.
Insecure AI tool chain
This refers to when tools used to train, fine-tune, host, serialise and operate AI models are not secure.
Specialised tools built to support AI models have been found to lack basic security features. For example, the Pickle format used to serialise ML models has serious security flaws. This may be because the tools were developed at pace by AI researchers and data scientists not following secure coding practices. AI tools often have elevated access rights to the systems they’re running on, making the impact of a security breach even worse. This is not a new risk, as any developer tooling can be insecure, but AI tools appear to be particularly prone to security issues. It’s easier for a hacker to target the tool chain for an AI model than the model itself.
You should make sure your cybersecurity team has approved the tools you use to support your AI models. This includes checking that the tools implement user authentication and follow the principle of least privilege, meaning they are not running with administrator permissions to your system.
Exacerbates previously existing risks
This refers to when the use of AI exacerbates previously existing risks, such as poor data management, insufficient security classification, insecure storage of credentials, and more.
An example of this sort of risk is over-privileged access. This happens when an AI tool is used to enhance enterprise-wide search capabilities. A user may not be aware of sensitive data that they currently have access to on a government system, but when they use an AI-enhanced search tool, the power of the tool exposes the lack of access controls and brings back sensitive data that the user was unaware of being able to see. The AI tool is not creating this issue: the problem already exists, but the AI tool is making it worse.
In line with the advice of vendors, you should review all enterprise access controls before deploying an AI tool to your system. This should be an ongoing exercise because no system is static. It’s essential that you’re able to continually monitor and review access controls when deploying AI applications across your organisation.
Perturbation attack
This is when an attacker stealthily modifies the inputs to an AI model to get a desired response.
An example of this type of threat is a computer vision (CV) system for medical diagnostics trained to distinguish between abnormal and normal scans. The system can be fooled when presented with an image containing specific amounts of noise, causing it to classify a scan incorrectly. Mitigations include adversarial training of the model with noisy images to improve robustness against this type of attack.
Prompt injection
This is when hackers use prompts that can make the generative AI model behave in unexpected ways.
Prompt injection is a type of perturbation attack specifically targeted at generative AI systems that use text prompts to generate new content (text, image, audio, video). Developers lay down rules about how a model should behave and respond as system instructions, which are provided to the model along with the user prompt. Fundamentally, a generative AI model cannot distinguish between the user prompt and these system instructions because both are just seen as input to the model. A hacker can exploit this flaw by crafting special prompts that circumvent the system instructions, causing the model to respond in an unintended way.
The potential impact of prompt injections ranges from very mild, like a user making a banking chatbot tell jokes in the style of a pirate, to much more serious. For example, a hacker might trick a generative AI model designed to send alerts to patients about medical appointments into sending fake messages about non-existing appointments.
Prompt injections come in 2 forms:
- direct, which means the user who is interacting with the generative AI model crafts the prompt injection themselves
- indirect, when other information that is being sent to a generative AI model is tampered with to include a prompt injection. For example, an email attachment can include a prompt and when a generative AI model that is tasked with summarising emails reads the attachment, the prompt injection is triggered
Generative AI has the ability to take natural language inputs and have a machine act on them. A common pattern for using LLMs in this way is called ReAct (Reason-Act): the LLM is prompted to reason about how to perform a task, and the response from the model is processed and used to automate calls to different services that perform actions. Hackers can subvert this approach to make the model perform different actions, which significantly limits the utility of generative AI in fully automated solutions. To make sure the model is doing the right thing, there must be a human present to review the action before carrying it out. This is why the majority of commercial applications of generative AI are in the space of human assistants (‘copilots’).
Work is underway to address the prompt injection issue and a number of mitigations are already available. These include:
- filtering prompts before they’re sent to the model (by sending the prompts through another ML model trained to detect likely prompt injections)
- filtering the outputs of the model before they’re returned to the user
- more speculative work around fine-tuning models to better distinguish between user input and system prompts
To defend against prompt injection, you should log all prompts sent to a model and carry out ongoing audits to determine if prompt injection is happening, blocking users you find who are responsible.
Hallucinations
Hallucinations are when the generative AI model responds with information that appears to be truthful but is actually false.
Counterintuitively for a machine, generative AI is better at creative tasks than fact retrieval. This is because all generative AI models predict and generate content by determining the most likely subsequent pattern based on previous training (for example, an LLM will predict the next most likely word). The models are therefore very good at generating plausible predictions that look correct but may not actually be correct. The risk is that overreliance by human operators on the outputs of generative AI models results in misinformation, miscommunication, legal issues and security vulnerabilities.
Fundamentally, generative AI models cannot be trusted to produce factual content. Any generative AI services that output generated content directly to the public – for example, an LLM-powered chatbot giving advice on a government website – would be prone to hallucination and could lead to someone being misled about a government service, policy or point of law.
A legal case in Canada found an organisation that owned a site with a hallucinating chatbot financially responsible for the bad advice it dispensed. In the worst case, hallucination could even lead to direct harm if a user acted on faulty advice. For example, a user being advised not to seek medical attention when they needed to.
In addition to this direct risk, there’s also a significant indirect risk if officials are relying on generative AI as a primary information source when providing the public with guidance, advising ministers or informing policy decisions.
You should make sure your users are trained not to uncritically trust the outputs of generative AI or to rely exclusively on their responses. Specifically around cybersecurity, if security practitioners in government become overly reliant on the advice of generative AI assistants, they may become less effective at spotting novel attacks and may even be misled into following bad advice and exposing systems to increased cybersecurity risks.
Adversaries using AI
Misinformation
This is when AI is used to create realistic synthetic media, leading to the spread of misinformation, undermining trust in digital media and manipulating public opinion.
Adversaries could use AI to interfere in electoral processes and spread misinformation. What makes this threat more potent is the ease with which bad actors can produce content for multiple audiences in many languages, with translations reflecting nuance and common parlance to make them more credible.
The technical ease with which generative AI models can be integrated with social media or other platforms also means that bad actors could spread misinformation automatically and at scale. At present, misinformation is the most pressing risk that AI (particularly generative AI) presents to governments, specifically relating to the integrity of democratic elections. There have already been a number of instances of suspected AI-generated fake media being deployed in the US. With the release of new, more powerful generative AI models capable of generating realistic video content, such as OpenAI’s video generation model Sora, this risk is only going to increase.
Big tech companies have committed to address the issue by including watermarks in the generated AI content their models create, but so far this has not become widespread. Google has announced a tool that can detect and watermark AI-generated content. The C2PA open standard for embedding metadata into media content, which allows the source and provenance of the content to be verified, is also gaining some traction.
When building services that receive and process digital content (text, images or even video), you’ll need to consider the impact of that content being generated by AI and therefore being unreliable, misleading or malicious. For more information about the ethical implications of misinformation, refer to the Societal wellbeing and public good section.
Phishing
This is when generative AI is used to craft more convincing phishing emails and messages that can be tailored to specific user groups, leading to an increase in internet fraud.
LLMs make it easy for fraudsters to create convincing phishing emails and messages in different languages, even those they do not speak. LLMs can be easily automated to produce unique, targeted and personalised phishing emails at scale, making detection much harder. There is already some evidence that the amount of phishing emails and messages is rising, with the likely cause being the advent of generative AI.
Government is likely to see an increase in phishing emails and social engineering attacks as a result of generative AI. The risk of cyber security breaches through targeted, socially engineered attacks driven by generative AI could become more acute, as it may become easier to identify likely targets by using generative AI to trawl across social networks and other public resources, looking for contact details for government employees in sensitive roles.
To detect scams of this sort, you’ll need more sophisticated counter measures – for example, using another specially trained ML model to detect and block phishing emails produced by generative AI.
You’ll also need to educate users about how to detect AI-produced fake messages, because previous red flags such as badly formed sentences and incorrect spelling will no longer be enough. The likelihood is that phishing attacks will become more targeted, and use more sophisticated social engineering techniques to gain the recipient’s trust.
Cyber attacks
This refers to when generative AI lowers the bar for entry for hackers to create malware and craft cyber attacks that are more sophisticated and harder to detect.
Generative AI has proved to be highly capable at aiding developers to write effective code: the AI model provides the developer with the majority of the solution, including prerequisites and boilerplate code, leaving the developer only needing to finesse the final details. A hacker using a generative AI model specifically to create malware or craft a cyber attack is likely to more quickly and easily achieve a working attack.
All large commercial generative AI models have filters in place to try to detect if a user is asking the model to create malware. However, these filters can be subverted (refer to prompt injection threats). The expectation from some cyber security experts is that the number and sophistication of cyber attacks is likely to rise due to the use of generative AI, as many more bad actors who previously were excluded from being able to create credible threats are now able to do so.
The unique position of government, and the capabilities and desire of hostile state-sponsored groups, mean that this threat is likely to be a key concern for government cybersecurity teams. The potential for escalating levels of sophisticated cyber attacks fuelled by generative AI is real, although research by Microsoft and OpenAI has yet to observe any particularly novel or unique attacks resulting from the use of AI. The area is under constant review.
You should expect increased numbers of cyber attacks and take steps to increase your existing cyber security defences. For more information, refer to the NCSC report on the near-term impact of AI on the cyber threat.
Fake official correspondence
This is when generative AI is used to craft convincingly human correspondence which can either be automated and sent at scale to organisations, flooding their usual communications channels, or lead to unfair outcomes when judged against human correspondence.
An example of this kind of threat might be a hacker using generative AI to create thousands of requests for information from a government department, seemingly sent from multiple unique people. Similar to the phishing threat, the ability of LLMs to create convincing and plausible text in an automated way, at scale, makes this type of attack particularly concerning. A hacker could overwhelm an organisation’s normal communications channels, causing an organisation to spend time and money responding to seemingly genuine requests while degrading their ability to cope with real people.
Another example of this sort of threat, at a lower scale, is a fraudster submitting official information that will be used to judge a decision, and where the use of generative AI to create fake answers may prejudice the decision.
Areas of particular concern for the UK government are commercial procurement, recruitment, freedom of information requests, and the processing of claims that require an evidence-based decision.
Mitigations are similar to those for phishing or misinformation attacks – for example, processing all correspondence through another ML model trained to detect AI-generated content. When running services that result in decisions based on evidence provided through official correspondence, you should consider the potential impact on the service of the correspondence being AI generated.
Security opportunities
In addition to the threats posed by AI there are also opportunities to improve cyber security through the use of AI. Some of these opportunities are:
- threat detection: AI can be used to improve threat detection systems by generating synthetic cyber attack data for training more robust models, or directly detecting anomalies in real-time cyber security data. AI models can also be used to analyse historical cyber security data and identify patterns associated with known threats. These patterns can then be used to detect anomalies in real-time network traffic or system behaviour. When unusual activity occurs, the AI model can trigger an alert to be raised to human operators.
- incident response: AI models trained or fine-tuned on large amounts of historical cyber security data can predict future threats. By recognising subtle changes in patterns, they can be used to anticipate emerging attack vectors. Generative AI can assist in incident response by automating the generation of reports, recommending remediation actions based on past data, or filtering out noise in verbose cyber security logs, allowing human analysts to focus on the most important information.
- security testing: generative AI can create security test cases, improving the efficiency and coverage of security testing. Instead of manually crafting test scenarios, security professionals can use generative AI models to mimic adversary behaviour, simulate various attacks, and analyse existing vulnerabilities, attack patterns and system behaviours. LLMs are good at analysing code, meaning they can also be used to review source code, point out security flaws, and generate secure code snippets based on security best practices.
- enhancing vulnerability management: generative AI can assist in documenting security products. LLMs can be used to process the large amounts of documentation, guidance and online help around different security tools and their features and limitations, providing summarised information and enhanced search capabilities. Internet-enabled LLMs can also provide up-to-date insights, helping prioritise vulnerability patches and updates.
Scenarios
The scenarios discussed below build on the security risks identified in this section, and will help you understand how they apply to some of the applications of AI in government.
Each scenario includes descriptions of potential impacts and mitigations. The likelihood and impact of each risk is scored following the approach outlined in the OWASP risk rating methodology. In addition to the impact factors included in the OWASP approach, user harm and misinformation are discussed as significant impact factors.
This list of security threat scenarios is not exhaustive, but you can use the scenarios as a template for assessing the risks associated with different applications of AI.
- Perturbation attack: an attacker stealthily modifies the inputs to an AI model to get a desired response: Identity verification using image and video capture technology.
- Insecure AI tool chain: tools used to train, fine-tune, host, serialise and operate AI models are not secure: Machine learning operations (MLOps) tools used with default configuration.
- Prompt injection threats: using prompts that can make the generative AI model behave in unexpected ways: LLM chatbot on a government website.
- Data leakage: responses from the LLM reveal sensitive information, for example personal data: Enterprise AI search tool summarising emails.
- Hallucinations: the LLM responds with information that appears to be truthful but is actually false: Developer uses LLM-generated code without review.
Identity verification using image and video capture technology
Scenario
A government service requires users to prove their identity by capturing an image of an identity document containing their picture, such as a passport or driving licence. A CV AI system then compares this image to a live video clip of the person to verify that the person is who they claim to be. A malicious user uses AI deepfake technology to insert their own image over the genuine photo of another person on a stolen identity document. The CV system is tricked into wrongly verifying that the malicious user’s identity matches the credentials on the other user’s identity document.
Impact
Misidentification of the true user, leading to identity fraud.
Fraudulent access to a government service.
Data loss of personal and confidential data about the genuine user.
Serious security breach if the service provides access to sensitive government information.
Mitigation
Ensure the service only uses biometric identity documents. For example, biometric passports contain electronic passport photos which can be securely transferred to the service for verification.
Use a trusted third-party service to look up and provide reference images for identity documents, rather than relying on images captured by users themselves. For example, the service could use licence images stored in the DVLA system and check this against the live video image.
Use deepfake detection methods to scan input digital images and video clips which are received by the service.
Risk rating
Likelihood: MEDIUM
Impact: HIGH
Recommendation
In this specific example, use of a biometric passport can prevent the attack. However, if the deepfake were applied to the live video clip of the user instead of the image of the identity document, the system could still be fooled. Access to this type of deepfake technology is becoming increasingly available, meaning that when you build services to receive and process images or video, you must put in place mechanisms to detect if the content has been manipulated by AI.
MLOps tools used with default configuration
Scenario
A data scientist working in a government organisation wants to experiment with training their own ML model using organisational data. The experiment aims to test whether the model can be used to triage official correspondence, improving efficiency. The data scientist starts by using an open source MLOps tool to host and deploy their ML model in their local environment. The default configuration of the tool exposes a public endpoint with no authentication on the public internet. By default, the tool runs with administrator permissions on the host machine. A hacker discovers the exposed endpoint and sends commands to the MLOps tool, using it to gain a foothold in the organisation’s network.
Impact
Serious security breach, which could lead to catastrophic damage to the organisation’s computer systems.
Data loss, including the organisational data used to train the ML model, and other sensitive data that can be accessed through the tool’s elevated permissions.
Mitigation
Check the default configuration of all ML tools before deploying them and ensure basic security controls are in place:
- authentication is enabled
- no public-facing endpoints are exposed unless explicitly required
- the principle of least privilege is applied so that tools only run with the minimum permissions they require
Risk rating
Likelihood: MEDIUM
Impact: HIGH
Recommendation
AI tools should be treated in the same way as all other third-party software. Even when they’re being used for experimentation, secure by design principles should always be applied.
LLM chatbot on a government website – full chat interface
Scenario
A chatbot deployed to a government website to assist with queries relating to a particular public service. The chatbot uses a private instance of one of the publicly trained LLMs. The user’s question is combined with system instructions that tell the LLM to only respond to questions relevant to the specific service. The system instructions are combined with the user’s original question and sent to the LLM. A malicious user could craft a specific prompt that circumvents the system instructions and makes the chatbot respond with irrelevant and potentially harmful information.
This is an example of a direct prompt injection attack.
Impact
Actual risk of user harm if a user is tricked into using an unsafe prompt that then results in harmful content being returned and acted on. For example, a user looking for information on how to pay a bill is directed to a fraudulent payment site.
Reputational damage to the government if a user made public potentially harmful responses received from the chatbot – for example, a user asking for generic information and receiving an inflammatory response.
Mitigation
Use prompt engineering to attach a meta prompt to any user input to prevent the LLM from responding to malicious input.
Apply content filters trained to detect likely prompt injections to all prompts sent to the LLM.
Choose a more robust model: some models have been shown to be more resistant to this kind of attack than others.
None of these mitigations are sufficient to guarantee that a prompt injection attack would not succeed. Fundamentally, an LLM cannot distinguish between user input and system instructions. Both are processed by the LLM as natural language inputs so there is no way to prevent a user prompt affecting the behaviour of the LLM.
Risk rating
Likelihood: HIGH
Impact:
LOW – response is returned to a single user with limited repercussions.
HIGH – response causes actual harm to a user.
Recommendation
Deploying an LLM chatbot to a public-facing government website comes with a significant risk of a direct prompt injection attack. You should consider the impact of an attack like this in the context of the specific use case. A chatbot deployed in a limited function or in controlled conditions – by restricting the number of users, for example – is far lower risk than one that is more widely available.
Enterprise AI search tool summarising emails
Scenario
A hacker sends a malicious email attachment to a government recipient who is using an enterprise generative AI tool to assist them. The tool uses a retrieval augmented generation (RAG) pattern, searching the private data the recipient can access and sending relevant data in-context to an LLM. The tool searches the recipient’s inbox, including their unread emails and attachments, for relevant information. The tool passes the prompt injection contained in the attachment to the LLM along with other private data. The prompt injection causes the LLM to respond by summarising the private data in the form of an obfuscated link to a third-party website. When returned to the recipient, the link may, depending on the tools being used, automatically unfurl a preview and instantly exfiltrate the private data to the third-party website.
Impact
Data loss: confidential information contained in the user’s emails is transferred to a third party.
Reputational damage to the department due to loss of data.
Regulatory breaches with financial consequences.
Mitigation
Configure the enterprise AI tool so that unread emails and attachments are not included in the initial search.
Apply filters before the in-context data is added to the prompt to remove likely prompt injections.
Apply filters to the response generated by the LLM to ensure any links contained in it are only to known resources.
Ensure network controls are enforced that prevent applications making calls to dangerous URLs.
Risk rating
Likelihood: LOW
Impact: HIGH
Recommendation
In this scenario, indirect prompt injection in an email attachment can be used to perform data exfiltration without any action required by the user. Similar data exfiltration techniques have already been shown to work against commercial LLMs. With the increased adoption by government departments of enterprise AI tools, we will likely see more of these novel generative AI-specific cybersecurity threats.
Developer uses LLM-generated code
Scenario
A developer uses a public LLM to answer coding questions and receives advice to install a specific software package, ArangoDB, from the JavaScript package management system npm. When the LLM was trained, the package did not exist. A hacker has previously interrogated the LLM with common coding questions and identified this hallucination. They then created a malicious package with the fictitious name and registered it with the package management system. When the developer installs the package, they receive the malicious code.
Impact
Unauthorised code execution when the software containing the fake package is deployed and run. This could result in significant data loss and other serious consequences.
Mitigation
Do not rely on the responses of the LLM: double-check all outputs before including them in your code. Check all package dependencies of your code before deployment. Use an automated tool such as a ‘dependabot’ or ‘snyk’ to scan for supply chain vulnerabilities.
Risk rating
Likelihood: LOW
Impact: HIGH
Recommendation
If developers follow secure coding best practices, the risk should never arise because all dependencies should be checked before deployment. Over-reliance on LLM-generated code without sufficient human oversight is likely to become an increasing risk. Treat all LLM-generated code as inherently insecure and never use it directly in production code without first doing a code review.
References
Practical recommendations
Applies to AI
- Design risk-driven security, taking account of the failure modes for the type of AI you’re using – for example, OWASP Top 10 security risks for LLMs or the ATLAS Matrix.
- Use a consistent risk rating methodology to assess the impact and likelihood of each risk – for example, the OWASP risk rating methodology.
- Minimise the attack surface by only using the AI capabilities you require – for example, by not sending user input directly to an LLM.
- Defend in depth by adding layers of security – for example, by using PET to prevent data leakage and adding content filters to sanitise output of an AI model.
- Never use private data that needs different levels of user access permissions to train or fine-tune an AI model.
- When building services that receive and process text, images or video, take steps to validate inputs to detect if the content has been generated by AI and could be unreliable, misleading or malicious.
- Review all enterprise access controls before deploying an AI tool to your environment to make sure users can only access the data they have permission to view.
- Never enter any official information directly into public AI applications or APIs unless it’s already publicly available or cleared for publication. Exceptions may apply for specific applications with different data handling terms provided under commercial licences, for example Microsoft Copilot.
- When experimenting with AI tools, pay attention to security and never assume default configurations are secure.
Applies to generative AI
- Avoid using generative AI where it’s not appropriate or required. Ask yourself if a non-AI solution or a traditional ML model trained for a specific purpose could work just as well.
- Prevent generative AI responses automatically leading to destructive or irreversible actions, such as sending emails or modifying records. In these situations a human must be present to review the action.
- Avoid using links to external resources in LLM responses that will be read by humans. If external links are provided, the response must be filtered to remove malicious URLs.
- Train your users not to trust the outputs of generative AI or rely exclusively on generated responses.
- Treat all LLM-generated code as inherently insecure and never use it directly in production without code review.
- Avoid putting LLM chatbots on public-facing government websites unless the risk of direct prompt injection is acceptable under the specific use case.
- When hosting virtual meetings, organisers should verify the identity of all attendees and state up front that the use of third-party AI meeting transcription tools is not allowed.
Governance
To successfully develop an AI programme, you’ll need strong governance processes because of the risks related to lawfulness, security, bias and data. Whether these processes are already built into your existing governance frameworks or implemented as a new governance framework, they should focus on:
- continuous improvement through the inclusion of new knowledge, methods and technologies
- identifying and working with important stakeholders representing different organisations and interests, including Civil Society Organisations (CSOs) and sector experts. This will help create a balanced view throughout the life cycle of any AI project or initiatives
- planning for the long-term sustainability of AI initiatives, considering scalability, long-term support, maintenance, ongoing stakeholder involvement and future developments
You should manage governance of AI through an AI governance board or AI expert representation on an existing governance board. You can include an ethics committee as part of your governance framework, depending on your operating context. Each has different roles and responsibilities.
AI governance board or AI representation on an existing board
The role of an AI governance board or representation on a board is to provide oversight, accountability and strategic guidance to help the organisation or team make informed decisions about AI adoption and use. It covers aspects such as risk management, compliance, assurance, resource allocation, stakeholder engagement, and aligning with business objectives and ethical principles.
An AI governance board helps you make sure your project is on track and that strategic, legal, ethical and operational risks are managed.
Ethics committee
The primary focus of an ethics committee is to assess the ethical implications of various actions, projects and decisions about AI within an organisation or programme. It evaluates AI from an ethical standpoint, focusing on values such as fairness, transparency and privacy, and is more specialised than that of an AI governance board.
An ethics committee usually includes legal experts, representatives from other relevant organisations related to the service you’re delivering, community members and stakeholders – all of whom can provide a specialised perspective on ethical matters such as health or security issues.
Before creating an ethics committee, you should consider the ethical, strategic and operational context of your organisation or programme. For example, the department may be too small or the programme too low risk to have a committee like this. It might be sufficient to have an AI governance board or an AI expert representative on a programme board to help you manage ethical considerations. An AI governance board should be able to guide you on whether you need an ethics committee. Refer to the Ethics section for more information.
Creating an AI systems inventory
To provide a comprehensive view of all deployed AI systems within an organisation or programme, organisations should set up an AI and machine learning (ML) systems inventory. This is in addition to the Algorithmic Transparency Recording Standard (ATRS) that all government departments and certain arm’s length bodies must use to ensure public transparency around the algorithmic tools used in their decision-making processes.
A live inventory will help your team, your organisation and your stakeholders understand the scope and scale of AI usage. It does this by providing better oversight and awareness of any potential risks such as data quality, model accuracy, bias, security vulnerabilities and regulatory compliance. An inventory will also be helpful for audit purposes.
The inventory should be regularly kept up to date with:
- a description of each system’s purpose, usage and associated risks
- details like data elements, ownership, development and key dates
- use protocols, structures and tools for maintaining an accurate, detailed inventory
You should consider sharing your inventory with the AI community of practice. This will enable the community to support your work and connect you with the teams that have developed similar projects across government so that you can share expertise and best practices and possibly reuse existing solutions.
Governance structures for teams
For all programmes or services that use AI systems, teams should:
- set out how the AI model will be maintained and managed over time
- develop a comprehensive plan for knowledge transfer, and for training new and existing staff to ensure the model’s sustainable management
- establish clear roles and responsibilities to ensure accountability within teams, including who has the authority to change and modify the code of the AI model
- ensure diversity within the project team by incorporating a range of subject matter expertise, skills and lived experiences
- establish pathways for escalation and identify key points of contact for specific AI-related issues
- adopt a risk prioritisation plan with specific project controls throughout the delivery and post-delivery cycle, such as how you will evaluate data sets for bias
- establish a data reporting mechanism that captures how data flows are managed and maintained throughout the delivery and post-delivery cycle
- set out how the programme or project team will work with and report to their programme board(s) and the ethics committee, if one has been set up
Managing risk
Risk management is part of governance. It helps you to strategically plan and manage your AI project to achieve objectives and respond to challenges in an agile way.
A risk assessment is critical in ensuring that AI projects are only undertaken if the potential benefits outweigh the risks. You should base this threshold on an objective assessment of the project’s potential risks and benefits, defining acceptable levels of risk and ensuring that any possible risks are identified and addressed early in the project life cycle. Relevant laws, regulations and ethical considerations should inform the assessment. If you’re managing AI programmes as part of a portfolio of work, The Orange Book (Portfolio Risk Management Guidance (PDF, 1,958KB)) provides a complete overview of risks.
As part of the design of your project, you should conduct a risk assessment to understand the risks and their potential to cause harm to individuals or groups, as well as the likelihood of the AI service being misused or exploited. The impact should be calculated based on the complexity of the AI system, the quality of the data used to train the system, and the potential for human error or malicious intent.
When conducting your assessment, you should consider a number of risks around AI including security, managing bias, legal and operational risks. Consider also that the scale of autonomy of an AI service can increase operational risks. For example, in the case of autonomous vehicles, the Society of Automotive Engineers’ Levels of Driving Automation ranks autonomy on a scale from 0 (no autonomy) to 5 (full automation for all features under all conditions). This scale correlates to the level of risk.
Alongside the risk assessment, you need to create a robust risk management framework that sets out defined roles and responsibilities and includes clear escalation routes to help mitigate risks.
Mitigating risks
You can mitigate some risks related to how the AI service performs by building or establishing programme and technical guardrails (best practices). These will guide the design, implementation and operation of an AI service or application, and are an essential element of delivering great services.
In the case of autonomous AI services that make decisions in areas such as social care or healthcare, the impact of autonomy of an AI service can be mitigated by including human intervention. These decisions need to be made in a controlled environment so as to not reintroduce bias into the AI service.
Whether your AI service is autonomous or includes elements of human intervention, it should be evaluated throughout the all stages of the project life cycle – including design, development and operation. Your risks and mitigation strategy should also cover how your team will manage continuous performance monitoring to prevent biased or inaccurate outputs.
There are also security and data protection risks which are covered in detail in the Security and Data protection and privacy sections.
AI quality assurance
AI quality assurance ensures that the AI service meets the service level requirements and provides evidence that the service is fit for purpose. It helps you check that robust techniques have been used to build, test, measure and evaluate AI systems. It also helps organisations communicate that their systems are trustworthy and aligned with relevant regulatory principles. It should be used throughout the AI service life cycle, including during testing and validation in the development phase and monitoring once the AI service is being used.
To meet quality assurance requirements, AI systems must be trustworthy, accountable, transparent and robust. They must ensure safety, respect privacy, mitigate bias, ensure fairness, and be secure and resilient. Given the complexity of AI systems, you may require a toolbox of different products, services and standards to ensure their effectiveness. For example, the Department for Science, Innovation, and Technology (DSIT)’s Introduction to AI assurance (PDF, 1,419KB) identifies the key elements of an assurance process – including risk assessment, impact assessment, bias audit, compliance audit, conformity assessment and formal verification.
Validation of AI
Being able to assert the quality of an AI service is critical to ensuring the safety of the system and the reliable accuracy of the service.
You must ensure that any updates to the AI system have a quantitative testing and validation process as part of the change control process. Validation is part of the testing of an AI system and is the ‘confirmation, through the provision of objective evidence, that the requirements for a specific intended use or application have been fulfilled’ (source: ISO9000:2015).
Deployment of AI systems that are inaccurate, unreliable, or poorly generalised to data beyond their training creates and increases negative AI risks and reduces trustworthiness. You should consider the complexity of your AI systems and identify the different products, services and standards necessary to ensure their effectiveness. To do so, you must make sure that these products and services comply with the required standards as defined by standards development organisations (SDOs), such as the International Standards Organisation (ISO).
Operational monitoring
Once you’ve released your AI system for use and it’s operational, you should have ongoing performance monitoring in place. This will ensure your system is operating as expected, and you should be able to provide evidence of this. It will also help you to identify and manage any changes to the model.
Any updates to the model need to go through a managed release process. This will help you mitigate the impact of any process change and clearly document changes made for future reference. You should ensure that the release can be withdrawn and the system reverted to an earlier version if required.
As systems and environments evolve, the current process may diverge sufficiently from the training period of the AI system. This is known as model drift and may require retraining or implementation of a new model within the AI system. Close monitoring is essential so that you can catch this as early as possible and reduce possible disruption to the AI system.
Practical recommendations
- Connect with your organisation’s assurance team and review the Portfolio of AI assurance techniques.
- Set up an AI governance board or include AI experts on existing governance boards.
- Consider setting up an ethics committee made up of internal stakeholders, cross-government stakeholders, sector experts and external stakeholders like Civil Society Organisations.
- Set up an AI/ML systems inventory to provide a comprehensive view of all deployed AI systems within your department.
- Make sure your programme or project teams have clear governance structures in place.
- Evaluate your AI product throughout the development and project life cycle, identify risks and implement a robust mitigation strategy.
- Use quality assurance techniques to make sure your AI product is trustworthy, accountable, transparent, robust, secure and resilient, and respects privacy, mitigates bias and ensures fairness.
- Make full use of the training resources available, including the courses on the business value of AI and understanding AI ethics on Civil Service Learning.
Appendix: example AI use cases in the public sector
This section includes sample case studies provided by teams that have implemented AI solutions in government departments and public sector organisations. These are real-life examples of AI adoption, presenting the technologies that were deployed, reflections on their capabilities and shortcomings, and discussions of the challenges and risks encountered in each project.
Be aware that these case studies were submitted in spring 2024 and only discuss the aspects of AI projects that each team deemed most relevant and interesting. They are not exhaustive and should not be treated as formal advice. You can find guidance in the Building AI solutions section of this playbook.
You’re encouraged to share your AI project with the AI community of practice to be considered for future updates of this appendix. This will also enable the community to connect you with the teams that have developed similar projects in other departments so that you can share expertise and best practice.
GOV.UK Chat: experimenting with generative AI
GOV.UK Chat is a pilot tool that uses relevant website content to generate responses, aiming to simplify navigation across more than 700,000 pages on GOV.UK and help users find the information they need. The project highlighted the importance of a careful and phased development approach. The experience gained from this prototype led the Government Digital Service (GDS) to focus on enhancing the system’s accuracy with the objective of launching a limited public pilot, provided that accuracy thresholds could be met.
Our tool and the problem it solves
GOV.UK Chat uses a retrieval augmented generation (RAG) approach. This allows users to engage with GOV.UK content through natural language queries. We preferred an RAG approach to fine-tuning a large language model (LLM) because GOV.UK content is updated regularly with edits and new pages published. The initial version of GOV.UK Chat was set up to test how we could improve user interaction with our website. We chose ‘business’ as our focus area because it’s complex for a user, involving many different departments’ policies and content.
Project development
The project was managed through a series of phased experiments, each lasting a couple of weeks and focusing on iterative development and evaluation. This approach facilitated controlled experimentation and rapid data gathering for system refinement.
Measuring success
The efficacy of GOV.UK Chat was evaluated using a multifaceted approach to ensure the reliability and accuracy of its responses. Content designers from GOV.UK and across government assessed the answers generated by the system. This involved exploring how GOV.UK Chat processed user queries, understood the intent behind questions, and retrieved relevant GOV.UK pages to inform its responses.
Experts then evaluated the appropriateness of the questions posed, the system’s interpretation of them, and the factual accuracy and completeness of answers provided.
Value delivered
Available through specific ‘magic links’, GOV.UK Chat was tested by hundreds of users in a controlled environment. Feedback suggested a preference for GOV.UK Chat over traditional search and navigation methods. This highlighted the convenience of direct question-answering systems, particularly for users with more complex questions.
However, the experiment also raised concerns about the reliability of the information provided, as the system occasionally produced ‘hallucinated’ responses.
Satisfaction surveys were sent out to users after use. Notably, nearly 70% of users found the chatbot’s responses useful and about 65% were satisfied with their experience. The primary value of this experiment is in what we learned from observing user interactions, understanding the types of queries posed, common failure modes, and assessing the feasibility of integrating such a system into GOV.UK more broadly.
Technologies
The development of GOV.UK Chat was underpinned by a selection of modern cloud and AI technologies, primarily hosted on Google Cloud. This choice was not exclusive, in that similar setups could be effectively implemented on alternative, functionally equivalent cloud services.
The answer-generation part of the system was powered by direct application programming interface (API) calls to OpenAI, leveraging its advanced generative LLMs for dynamic answer generation. Additionally, we used a Qdrant vector store to facilitate efficient data retrieval and management to support the RAG approach.
Challenges and solutions
It’s important to acknowledge the rapid evolution of the technology in the generative AI domain. When the GOV.UK Chat system was initially conceived and developed (July 2023), the options for technologies like this were relatively limited.
In our first experiment we used a simplistic approach to retrieval, whereby we returned whole pages. This approach occasionally resulted in errors because a niche search might return lots of very long pages that would exceed the LLM’s token limit, resulting in an error.
In response, we started exploring improvement strategies, specifically through nuanced chunking, alternative embedding models, vectorisation strategies, re-ranking and improved few-shot examples in the prompt. With an answer accuracy of 80%, the experiment suggested that accuracy gains would be required prior to the product being deployed for users on the site.
If we’re to scale GOV.UK Chat into a full live pilot, a major challenge will be quality assurance. The techniques we used in the first stage of the work are highly manual and not practical to scale. We believe a solution will be to build a knowledge base of quality-assessed questions so that a semi-automated quality assurance mechanism can be developed.
Example ethical, legal and security considerations
Safeguards were implemented to protect user privacy and prevent personal data submission. We complied with UK data protection legislation and conducted a thorough data protection impact assessment, removing any personal data from GOV.UK pages accessible to the LLM. Additionally, collaboration with cyber security experts from CDDO, Number 10DS and i.AI through ‘red teaming’ helped identify and mitigate system vulnerabilities, reinforcing our commitment to responsible and secure technology deployment. For more details, refer to the GOV.UK Chat blog.
GOV.UK Chat: doing user research for AI products
This case study focuses on the user research we conducted in the Government Digital Service (GDS) to help the team developing the GOV.UK Chat tool explore user reactions and evaluate the system with experts. As an experimental team, we also wanted to learn about appropriate tools and approaches to develop GOV.UK Chat.
Initially, we focused on testing the accuracy of the tool using surveys, presenting typical user questions and answers for subject matter experts (SMEs) to evaluate. We supported this assessment with a round of online testing with internal users from the content design community to gain feedback on the initial design of the chatbot interface. This approach allowed us to develop the system safely before showing it to business users. Data was collected using a survey, where SMEs evaluated a series of questions and answers without being told which answers were from the large language model (LLM) underpinning GOV.UK Chat and which were human. Accuracy ratings were collected via Likert scales supported by qualitative comments.
We needed to determine if the SMEs agreed about their assigned ratings, as this would tell us about the reliability of the data. We calculated the level of agreement and found it to be high, indicating that SME ratings were consistent. We were then able to compare the ratings for the LLM with the human model answers to see if they were significantly different. We found no significant differences – the accuracy of the LLM was rated on par with the human model answers.
A subsequent round of online testing provided further insights on accuracy from live queries, and helped us examine the tool’s conversational memory. During remote testing, internal users from our content design community interacted with GOV.UK Chat to find information to support a fictitious user who was planning to start a business. After the interaction, participants completed a short survey about their experience. We analysed the test session videos and triangulated this data with their survey responses.
Project development
We worked with users already in a business, and those about to start one. Our testing was conducted in 2 phases. First, we conducted a qualitative study that combined an interview with a usability test of the GOV.UK Chat prototype. This allowed us to explore users’ attitudes and experiences with AI tools, gain feedback on our tool and further assess accuracy.
In the second phase of testing, we invited 1,000 users to test GOV.UK Chat via a research banner on GOV.UK. Users interacted with the tool, providing message-level feedback on answer usefulness, and completed a short survey about their experience. During both rounds of testing with business users, we repeatedly assessed accuracy by gathering SME ratings of the LLM’s answers.
The scaled-up testing was an important step. Having a large data set for analysis added weight and confidence to how we were assessing accuracy. It also provided an opportunity to examine the types of questions that users might typically ask, and, importantly, how those questions were phrased. This would help us understand to what extent users required support in articulating their questions. Finally, scaled-up testing allowed us to combine our understanding of accuracy with users’ feedback on the experience of using the tool.
Value delivered
Overall, 69% of users thought the tool was useful or very useful. We also achieved an accuracy threshold of 80%.
Challenges and solutions
Our research approach was inspired by a desire to safely and responsibly test the LLM before showing it to users. However, this was a labour-intensive approach. Each investigation required us to quantify accuracy alongside exploring the user experience, sometimes uncovering broader insights about the propositional value of the tool.
The scaled testing was particularly useful in combining different data sources, such as survey ratings, question types, participant feedback about whether the generated answer was useful, accuracy of answers (evaluated by SMEs), and response times. To not dissuade users from completing the survey, we kept it short and asked a small number of questions.
In future live-scaled testing, we plan to bolster this data with live intercept interviews, speaking to business users who are using GOV.UK and GOV.UK Chat to solve their emerging information needs. This will help us to explore their experience and attitudes to the tool in more detail.
CCS commercial agreement recommendation system
The Crown Commercial Service (CCS) built a commercial agreement recommendation system using spend and customer market segmentation data. Our goal was to generate relevant agreement recommendations for customers to help them discover new agreements by considering their past procurement activity and their market sector peers’ activity. The system was inspired by the technologies used by companies such as Netflix to deliver intelligent and personalised recommendations for users based on their historical data.
Our tool and the problem it solves
When a customer uses one of our hundreds of agreement lots to procure products and services, the supplier provides data about that transaction. Over time, we collate these submissions into a historical customer-product interaction data set.
A machine learning (ML) recommendation system trained on data like this can provide relevant and detailed recommendations, operate without customer or staff input, keep pace with evolving behaviour, and take into account other useful information.
This recommendation system focuses on discovery, generating recommendations in the form of agreements that are new to each customer, which makes them aware of relevant agreements that they might not have known about or found otherwise.
Project development
In the absence of an online digital platform ready for recommendation serving, we used this configuration to enable a small team to rapidly develop a pilot solution using historical data that could be served to customers through email marketing or during conversations. Once this platform was ready, new challenges appeared, including how to ensure continuity of recommendation serving and allow for a richer feedback loop by measuring interactions with recommendations in the digital service.
Measuring success
A number of customers were given relevant recommendations and an equivalent number in a control group were not. Acting on these recommendations translated to an increase in the average number of unique agreements used by those customers compared to the control group. This is because we made customers aware of new agreements beyond those which they currently use.
Value delivered
We now have the potential to provide commercial agreement recommendations to many more customers. This was previously limited to customers with dedicated account managers who have specialist customer knowledge. The ultimate goal is to integrate this system into an online digital platform where customers can access their recommendations at their convenience.
Technologies
The algorithm we used to build our tool is based on TensorFlow Recommenders and consists of a ‘two-tower’ neural network (NN). This is a popular architecture for modern recommendation systems. When training, each NN learns the embeddings for the transaction context and candidate products so that the combination of these embeddings correlates with the observed affinity of that context and candidate in the training data. This then allows the embedding of any number of potential candidates, which are ranked by mathematical distance from a given context to get recommendations.
Challenges and solutions
Offline historical data of customer-product interactions allows the reconstruction of the buying behaviour of a customer through time and the comparison of this to generated recommendations. These simple offline ‘hit’ metrics can be flawed in a discovery context.
In our interaction data set, customers generally use the same products with some variability. A lack of discovery ‘hits’ with what a customer interacted with next is therefore not necessarily a bad thing, as we are specifically trying to predict what a customer has not interacted with yet. This causes challenges when assessing the performance of a discovery-based recommendation system using offline data only.
Example ethical, legal and security considerations
As our customer organisations are legal entities and not people, no personal data is used when making recommendations. Additionally, we use objective past behaviour as a guide to produce recommendations for consideration only by customer organisations.
DWP whitemail vulnerability scanner
To reduce delays in answering post that cannot be automatically processed (‘whitemail’), the Department for Work and Pensions (DWP) is training a large language model (LLM) to support the processing of this correspondence.
Our tool and the problem it solves
We developed a service using AI that may already be saving lives. Every day, DWP receives about 25,000 pieces of post that are not standard DWP forms and cannot be automatically processed and routed. This includes medical certificates, forms and paperwork from other government or non-government bodies, copies of bills and receipts, handwritten letters and correspondence, and more.
This whitemail is currently scanned-in as digital images and sent to our agents to help support their claimants. However, this can incur delays in processing. Where this whitemail concerns someone who is experiencing a vulnerable episode, a timely response is crucial. Evidence has shown that, in the past, delays have led to serious consequences and contributed to cases requiring Serious Case Panel reviews.
The AI-powered whitemail vulnerability scanner that we built helps us to identify and prioritise whitemail requiring urgent attention. The tool, developed by Shared Channels Experience (SCE) within DWP, leverages a pre-trained LLM that was fine-tuned to:
- understand text that has been extracted from the images received (including handwritten correspondence)
- attempt to remove any personally identifiable information (PII) to avoid any bias
- read and, crucially, understand the meaning and sentiment of the whitemail as it comes in
- create an actionable shortlist of prioritised cases that a human can review to remove false positives and decide if urgent intervention is required
Project development
The SCE Whitemail Vulnerability Scanning AI service is currently in a test-and-learn phase. The service has been up and running since December 2023.
During this time, colleagues in the Advanced Customer Support (ACS) team have been receiving the daily feed that flags potentially vulnerable customers, leading to approximately one urgent intervention per day.
The feed is for all benefit lines and vulnerability categories. However, during the test-and-learn period, the focus is on suicide and risk-of-harm categories and only 2 benefit lines. This is to understand the volumes and business impact. ACS colleagues will continue to receive the feed, take out any duplicate entries and pass all documents to the product lines which need a human to look at them.
Value delivered
Without the AI-powered vulnerability scanner, manual analysis of the volume of whitemail that DWP receives daily would require at least 300 dedicated people. This new AI solution has increased the speed at which we are able to identify vulnerable people from the thousands of letters we receive each day, meaning that those most in need can be more quickly directed to the relevant person who can help them.
Challenges and solutions
The AI technologies deployed in the project will generate some false positives and may fail to capture some vulnerable users. In those instances, existing agent-led processes would still apply. In other words, this platform functions in addition to existing ways of working, not as a replacement.
Example ethical, legal and security considerations
A number of strict ethical considerations were taken into account during the development cycle. The platform does not:
- affect whether a claimant is entitled to a benefit or not
- affect the amount or duration of any benefit claimed
- profile by any PII or protected characteristics
- replace the need for human intervention
- save any agent time directly
- correctly flag all potentially vulnerable claimants or correctly identify all of their vulnerability categories
This work will continue and decisions might be made about formally moving into production and expanding the use of this tool to other benefit lines or vulnerability categories.
Digital Sensitivity Review at FCDO Services
FCDO Services has developed an AI-powered Digital Sensitivity Review toolset. This enables departments to select, review and transfer digital records consistently and securely for permanent preservation at The National Archives (TNA), meeting the requirements of the Public Records Act.
Our toolset and the problem it solves
The Digital Sensitivity Review toolset uses AI to significantly increase the efficiency, effectiveness and risk reduction for the review and transfer of digital records. Digital records present several risks, as hidden data within records often contain sensitivities. For example, it’s common to insert cropped images into documents, but users may not realise that the original uncropped image is still present and easily recoverable.
As there’s a limited supply of trained sensitivity reviewers, our toolset helps departments to detect issues like this and better cope with the increasing volume and complexity of digital records. Techniques that reduce digital volume, such as ephemeral identification, duplicate identification and clustering, are essential to this process. So we designed specific solutions for each of the stages involved in the transfer of digital records.
Project development
To develop this project, we established Team Cicero as a collaborative working arrangement in accordance with ISO 44001. This enabled working with specialist partners in industry and Sheffield, Glasgow and Loughborough Universities, who are leading cutting-edge research into topics such as lifelong machine learning (ML) techniques.
We defined the target outcome based on the volume and complexity of the unstructured data, commonly called the ‘digital heap’, and established a set of challenges. Integration of the technologies and the users into one system was a consideration from the outset, along with commercial licensing mechanisms. We decided to use off-the-shelf software wherever possible and ensure that the system was modular in design, making it easy to upgrade.
Measuring success
Our 2 key metrics were the number of sensitivity reviewers needed to achieve the target risk appetite for a given volume and complexity without changing records selection policy, and the risk of release of sensitive content.
Without this technology, we would require over 100 additional operations team members, including sensitivity reviewers, to meet the demands of digital review and transfer for one government department. Moreover, to meet the volume of digital records requiring review, we would require a system that worked at least one order of magnitude faster than the equivalent paper review system.
Value delivered
We have been able to process records with 10% of the sensitivity review effort and reduce the risk of release of sensitive content to well below what was achievable without the use of this technology.
Challenges and solutions
ML techniques require considerable volumes of examples to train the model, but the limited amount of material actually redacted so far is small. Of the records selected for review, typically less than 1% of the documents reviewed have any redaction applied. This becomes a statistical constraint on the use of ML, because sourcing the maximum possible volume of sensitive material is fundamental to maximising the potential of the system.
This problem is exacerbated by the fact that different technologies are more suited to different Freedom of Information Act exemption categories. For example, international relations sensitivities are fundamentally different to those involving personal information. Different departments applying differing policies reduces the availability of statistically significant volumes. Policy examples include the definitions of duplicate, ephemeral and sensitive content.
Example ethical, legal and security considerations
Our system is aligned with security considerations required by different departments and we considered several legal and ethical issues. For example, the technologies are currently used only to assist human decisions and our reviewers are fully trained to understand the ethical and legal aspects of their work. The technology augments the reviewer’s ability to process a greater volume of material than would otherwise be possible, but it does not remove or replace the responsibilities of the reviewer.
We have also identified a potential challenge around the ability of departments to keep sensitive content post-transfer, and to provide statistically meaningful volumes for ML, with differing opinions on the legality of doing so. We believe this needs to be discussed further if ML is to reach its full potential in sensitivity reviews.
NHS user research finder
This project focuses on creating a tool to help colleagues find user research completed by other teams across the organisation.
Our tool and the problem it solves
Our tool allows users to both upload and search for user research. Users can upload user research into our database, which is then summarised using a commercial large language model (LLM) through an application programming interface (API). The user can review and edit the summary generated by AI before confirming the submission. After a review from the user-centred design ops team, the report summary is then published on the tool. Users can query this database using natural language which is interpreted by the same API.
The tool allows users to enter text search queries such as:
- “What do we know about 111 online?”
- “What do we know about records access?”
- “What do GP practices need from patient facing apps?”
Users are then presented with research summaries and relevant contacts from the publishing team.
Project development
We developed this tool over a 13-week period with a commercial partner specialising in AI. It’s currently in private beta testing. We worked using typical agile methodologies and conducted user research and testing throughout.
Measuring success
We’re measuring the success of the tool through a range of key performance indicators (KPIs) including usage, uploads, searches, search queries, satisfaction and completion rate. We’re also conducting user research to measure ease of use and effectiveness at responding to the original user need.
Value delivered
Our tool makes user research more easily findable and visible. If we can refine the summarising capabilities of the tool within the parameters of our information governance, this will save considerable time for user researchers and minimise the risk that relevant user research is neglected or work is duplicated.
Challenges and solutions
The tool makes use of an API to summarise information using an LLM. Limitations and shortcomings include the fact that the AI currently does not always recognise all the sections within a given research output, which then requires the user to fill in the gaps.
Another unexpected challenge is the way the AI summarises reports. When asked to process the same report several times in succession, the tool will often return varied results. The same is true for the search. Furthermore, when asked for very long, specific or complex search queries, the LLM might return only vaguely related results (instead of nothing).
For example, the query: “Has anyone done any research on how young people (so around the ages of 11 to 16 or 18), feel about accessing healthcare, understand how it works (like how to see a GP or book an appointment, for example), their parents’ involvement in managing their healthcare tasks and when there might be a ‘handing over of the baton’ to the young person to managing it?” returns results about a 111 online discovery with parents of children under 5 years old, as well as general research around adult proxy patients’ user needs.
We are inherently reliant on a third-party API for the tool to function. In early prototyping we attempted to run an in-house LLM. However, the quality of the results was not high enough given the time we had available to develop the prototype. This could be explored further.
Example ethical, legal and security considerations
The development of a tool to aggregate and query user research using a commercial LLM for summarisation and search functionalities requires careful consideration of ethics and security. From an ethical perspective, we considered potential accuracy and bias issues of AI-generated summaries. These can impact the integrity of user research.
Privacy and legal concerns are also significant because user research often contains sensitive information. Ensuring the LLM does not compromise participant confidentiality or contravene data protection laws is paramount. The use of a commercial LLM involves checking the security of the systems deployed as well as navigating copyright and intellectual property rights. In particular, the project’s reliance on a third-party API for critical functions introduces dependencies and risks related to data security, service continuity, and control over data processing. This necessitates rigorous vendor assessment and contract management to safeguard organisational and user interests.
Furthermore, the variation in AI-generated outputs and the challenge in handling complex queries underline the need for continuous monitoring, user feedback integration, and iterative improvement to effectively address ethical, privacy and legal concerns.
NHS.UK reviews: an automated reviews moderator
As operators of the NHS.UK website, we need to moderate hundreds of thousands of reviews of NHS services to make sure they meet our guidelines about personal information, abuse, discrimination and other policies. Data scientists from NHS England have automated the review moderation process using natural language processing (NLP) techniques to build bespoke models to implement our policies.
Our tool and the problem it solves
We developed an AI tool to automatically identify reviews that contain suicidal and self-harm ideation content; serious issues which should be formal complaints rather than a review; profanity; email addresses; descriptions of a person; names; fully capitalised text; URLs; and text that doesn’t describe an experience. Our AI solution is scalable, trackable and quick. It has a low running cost and improves user experience, as reviews are moderated more quickly and consistently.
Project development
To deliver this project we deployed a multidisciplinary team. Initially, we developed the tool on a flask app using named entity recognition, part of speech tagging, lookups and simple regex rules. This phase helped to establish a robust architecture and to integrate with the NHS.UK platform where the tool was to be used. Following this, we developed more complicated machine learning (ML) models that required good quality training data, and time to train, test and evaluate.
Measuring success
We measured the success of our AI solution through reductions in solution cost, moderation time, and a reduction in the proportion of unpublishable reviews. Our ML models were also tested through:
- confusion matrices: to describe the outcomes of the models against the test data set. We used balanced test data sets and data sets which represented real-world data volumes to give an idea of real-world performance
- clerical review: false positives and false negatives were subjected to a more detailed review. This allowed us to understand edge cases where the models were underperforming, and identify opportunities to fine-tune
- non-functional testing: metrics included latency, throughput and spin-up time for compute. These tests allowed us to understand whether we would see degradation of the service over a range of likely and extreme scenarios, and allowed us to assure the product owner that the solution was fit for use
Value delivered
The use of AI technologies removed the need for a third-party moderation service, reducing cost, increasing accuracy, and enabling scalability of moderation through automation. It also decreased the time from review submission to publication, improving patient feedback. The immediate feedback provided by the AI system has resulted in an increased percentage of published reviews, a decrease of rejected reviews, and the ability for the NHS team to easily add moderation rules in future based on requirements and reviewing all comments accordingly.
Technologies
The auto moderation software was written in Python and hosted on our virtual private cloud service. It consists of a flask app which handles API calls and payloads to hosted ML inference models. The API call passes the content to the model and the model returns a JSON payload which informs the NHS.UK system how to handle the content. The tool uses a combination of regex and NLP methods, and pretrained embedding models like BERT, bag of word and MNET.
Challenges and solutions
The use of these technologies in the NHS is not widespread and we had to discover how to sign off a solution which contained AI. The compartmentalised nature of the solution helped, as we were able to assign risk to components and get them signed off in isolation before signing off the solution as a whole.
The probabilistic nature of the AI models needed clear explanation and rigorous testing to ensure we were not introducing additional risks to the service. Additionally, one model faced the challenge of insufficient training data as it targeted a less common reason for review rejection – safeguarding concerns. We addressed this by augmenting our data set through the generation of synthetic data using NLP tools, which was then validated by expert moderators to ensure its quality and relevance.
Example ethical, legal and security considerations
Our product involves dealing with user feedback that potentially contains personal or sensitive information. This required us to pay particular attention to ethical legal and security issues throughout the project life cycle. Our data processing complies with the Data Protection Act 2018.
We have made provisions for people to request a human review if they are not content with the automated response. This ensures that human operators are involved in the process and can monitor the outcomes of the automated review of comments.
We paid particular attention to ethical concerns. For example, we developed an effective suicidal and self-harm content detector. We undertook a clinical safety exercise to ensure clinical risks were elaborated and appropriately mitigated. In addition, a bias analysis was conducted on the 3 models trained by the team for the auto moderation tool: safeguarding, complaints and ‘not an experience’. The analysis aimed to identify any potential bias in these models by examining the sentiment of misclassified comments, the length of the texts, and the number of spelling mistakes standardised by text length.
Our solutions assurance team assessed the solution with a particular focus on training data coverage and model behaviour, examining the extent to which literacy levels in reviews affected the model outcomes. Our technical review group – a panel of experts with a wide range of expertise – reviewed the solution and made recommendations regarding improved security and solution architecture.