Extracting Data from Archives: Best Practice Guide
Updated 12 November 2021
Glossary of Terms
The following terms are provided for your reference throughout the report.
Archive: In this report an archive is considered in the widest sense, so includes any collection of data and/or information held in either physical form (e.g. as paper) or in a digital format, which may simply be a digital image of the physical object.
Location: Location implies a geographic location with which the archive content can be associated.
Scanning: Scanning describes the process by which a material such as a map is scanned and a digital/electronic copy created.
Digitise (or vectorise): The process of converting information into the digital codes stored and processed by computers. In geographic applications, digitising usually means tracing map features into a computer using a digitising tablet, graphics tablet, mouse, or keyboard cursor (source: OGC).
Extracting: The extraction of values and/or information that informs understanding. An example could be extraction of map features, annotation and associated text or other information that describe a feature on a map. Alternatively, it could be the extraction of tabular data in a report that relates to a location. These processes may be undertaken manually or automatically.
For a full glossary of the terms, names or phrases listed in this report, please see the Geospatial Commission Glossary.
Introduction
Purpose
This guide is intended to provide a best practice framework for working with location data in archive materials throughout all stages of a data extraction pipeline – from identification of location data in archives, through to scanning and digitisation, extraction and subsequent data management.
Aim
An organisation may take years to develop experience of the activities, stages and resources required to digitise archive collections such as map collections. This guide is designed to provide a high-level overview of key considerations when undertaking a project to extract location data from archive materials. This report is intended to provide guidance on the principles of data capture relating to the range of data types and materials that exist in archives. This report is not intended as a guide to working with specific data types.
The aim of this guide is to:
a. provide a best practice overview for data extraction work
b. promote the development of considered data extraction pipelines
Audience
Our intended audience is everyone working with and extracting data from archives and especially those undertaking data extraction work for the first time. The primary source of this work is the geospatial data held by the Geo6 partner bodies: British Geological Survey, Coal Authority, HM Land Registry,Ordnance Survey,UK Hydrographic Office and Valuation Office Agency. However, the guidance is applicable to all those working with archive data in academia and the public and private sectors.
Background
The Geospatial Commission (GC) was established in 2018 to improve the quality of key, publicly held geospatial data and to make it easier to find, access and use. By doing so, it is estimated the GC will unlock up to £11 billion of extra value for the UK economy each year. In January 2020, the GC launched the Archive Data Capture Methodologies project to identify the challenges that agencies meet when extracting location data from archives and to identify more efficient ways of extracting location data from varying types of archive materials across the Geo6 partner bodies. While the project has focused on location data, many of the outputs and recommendations will be applicable to other data types held in archive materials.
The Archive Data Capture Methodologies project included research into archive data capture by the Geo6 partner bodies, Geo6+ organisations and a landscape review within academia, research bodies and the wider industry.
This report is a high-level summary of the findings resulting from activities involving the Geo6 organisations and those interviewed during the landscape review. During these activities, best practice for extracting data from archives was discussed and key issues, challenges, learnings and recommendations from data extraction projects were documented. An archive data extraction flowchart was drafted, shared on the Microsoft Teams project collaboration site and updated over the course of the project by the project partners. The resulting flowchart which can be found at the bottom of this page forms the basis of this best practice guide.
General Considerations
Extracting data from archives can be a unique and complex undertaking. The following are some of the factors, frequently interrelated, to consider at all stages of a project to extract location data from archives.
Costs and Funding
When extracting data from archives, costs may be associated with: resourcing and deployment of appropriately skilled and expert staff at each project stage, such as archive reconnaissance, scanning in house or procuring scanning services; the development of automated or manual data extraction techniques; or on resulting quality assurance and quality control measures.
What this means: Understanding the processes and the work involved at all stages of a data extraction project will facilitate the creation of a representative cost benefit analysis (and project plan including resource requirements) which can be widely communicated with the relevant project stakeholders and potential funders.
Data Domain Expertise
A project to extract data from archives will potentially require the involvement and collaboration of skilled and expert staff at different stages of the project. For example, dedicated specialists in project management, data management, archiving, scientific scanning, data science and location data may all be required to work together at different stages of a data extraction project.
What this means: Knowing which staff are required at each stage of a data extraction project will facilitate an understanding of when and where skills are required on a project.
Value
Valuing data in an archive is a challenging topic and the value of the data in an archive may be monetary, cross-domain, strategic, historic, resulting from the uniqueness of the archive materials or even unknown. Value across the process of extracting data from an archive may go up and down for different reasons during an extraction project and it is potentially complex and difficult to communicate the value of archive materials and/or extracted data.
The value of the data may frequently be primarily linked to its primary end use(s). Subsequent reuse of data (predicted or unpredicted) will add value.
What this means: Though complex, it is important to consider the potential range of value of archive materials and extracted data. Consider the value of potentially unconsidered uses in addition to identified and priority use case(s).
Quality assurance and quality control (QA and QC)
The QA and QC aspect applies to each stage of the extraction of data from an archive through the QA of the original materials in the archive, the QA and QC of any scanning work and of any extraction and data management. Limited resources may result in limited or minimal QA/QC.
What this means: Insufficient QA/ QC at one point in a data extraction pipeline may impact other stages. QA/QC is a potentially resource-intensive but critical process which will influence users trust with extracted data.
Data Quality
Data quality is important at all stages of an extraction project and the success of each stage relies on the quality of the original data, the metadata, the scanning and the extraction and the delivery. In addition, data quality is wrapped up in, and relies on, rigorous QA and QC measures.
When considering the quality of the source material, it is important to record information about the quality and ensure this is maintained throughout the pipeline to provide context for the use of the data. Communicating the quality of the data at each stage is critical.
What this means: Ensure that data quality is recorded and communicated and that data quality is maintained throughout a pipeline using QA and QC measures.
Usability
The usability of data that results from a data extraction pipeline can be measured against how well the output data meets the original requirements outlined in the cost benefit analysis for the extraction project. The usability of the data is highly dependent on the maintenance, development and communication of the data quality, the quality of delivery mechanisms and of the metadata.
What this means: At all stages, refer to the cost benefit analysis to ensure the data extracted will be fit for requirements.
Data Standards
The use of industry standards for archives, data extraction, metadata and delivery of data will help ensure that the data quality and usability of the final product is maintained. For example, using file formats throughout a pipeline that maintain metadata and any georeferencing that has been captured will help ensure that important information is not lost.
What this means: Use industry standards where they exist throughout a data extraction pipeline. Following data standards will increase the data interoperability and confidence in the data extracted, therefore potentially increasing data value as a product/service.
Best Practice Guide
Archive data extraction flowchart
The image at the end of this page shows the archive data extraction flowchart developed as part of the Archive Data Capture Methodologies project. This flowchart captures key considerations for the best practice of extracting location data from archives. Each section of the flowchart is detailed further in the following sections.
Box 1a - User requirement
Box 1a outlines the need for a clear ‘user requirement’ for the data to be extracted from the archive. Future access and use, including interest beyond the original scope and to promote novel re-use in combination with possible new interest groups, should also be factored into consideration.
Considerations/steps:
The following are recommended steps which will support the development of user requirements.
- Identify the user requirement level i.e. Is the user community at the organisation, project, local, regional or global level?
- Undertake user research to support the development of user requirements.
Create a business case detailing priority use case(s) for the extracted data and a cost benefit analysis which balances the costs of a data extraction project against potential reduced future costs. Reduced costs or increased income may result from:
- fewer future interactions with archives
- lowering the staff cost of dealing manually with enquiries and related physical handling of archive material
- the speed at which the material can be accessed and by multiple users at the same time
- unknown interest, including potential commercial interest, in using digital versions of archive content
Note: When developing user requirements, although challenging, try to think beyond the immediate requirement(s) to possible wider interest. Questions to consider include:
- What other potential uses might there be for the data?
- What quality is required to meet future requirements?
- What is the cost to extract data out with the original requirements?
Feedback loop between 1a and 1b – Inter-related tasks
4.1.2 Box 1b - Archive reconnaissance
Box 1b outlines the requirements to identify, understand and communicate the materials, such as documents and maps, in an archive and any restrictions on how and where the materials can be used in an extraction pipeline. Archive reconnaissance involves generating an overview of the content of an archive and understanding any data management requirements for digital extraction.
Considerations/steps:
The following are key questions to consider during archive reconnaissance.
- Is there an up-to-date and comprehensive overview of content in the archive? A well written and easily comprehensible summary or abstract of content is essential.
- What level of indexing is available for the materials in an archive?
- If possible, ensure any index is standardised and consistent with other archive indexes to allow easy merging and cross-referencing.
- What level of metadata detail is available? In other words, how much detail is available for each piece of material held in an archive? E.g. Is there an overview of the entire archive, each box of archive material, information about each individual piece of archive material in each box?
- Ensure any metadata is standardised to match other archive indexes and that the metadata meets appropriate standards.
- The copyright of the material and any other associated terms and conditions (T&Cs) or intellectual property rights (IPR) will determine how the archive material can be used and reused in the future.
- Is the archive well organised and accessible? What condition is the archive in? Do the materials in the archive have special handling needs or security concerns? For example, are the materials fragile and do they include, staples, paper clips or bound paper?
- Can the material in the archive go offsite for scanning, digitising or data extraction purposes or should any processing be undertaken on site?
- Does the archive contain analogue, digital or mixed data and in what format does the material come in i.e. paper map, photograph, field slip, report, PDF?
- How is the data presented?
- If and when the material is processed for data extraction, what additional information, aside from the location data, requires capture e.g. annotations?
- If the material is moved off site, does it need to be returned in the same state after scanning or data transcription?
- Can the material be scanned and destroyed?
Note: Avoid part extraction in case there is a future requirement for the wider content
Feedback loop between 1a / 1b and 2 – Expert input
4.1.3 Box 2 - Scanning or data transcription The process of scanning or transcribing data from the archive material is the process of creating a digital image or digital data from the original archive material. At this stage of the process it may be crucial to liaise with content experts to agree best methods for the extraction of specialist content.
Considerations/steps:
- Scan paper archives.
- Transcribe obsolete analogue media to digital format.
- Transcribe obsolete digital media to a modern digital format.
- For all of above, consider disposing of or retaining the original.
- Define QC measures, whether checks are manual or automated or a combination of both.
- Define the QA process and include auditable process steps.
- Undertake QC and QA of the scanned or transcribed materials during and after the conversion process.
- During the scanning or transcribing process, record the source information.
- Record information on the quality of the original source material.
- During the scanning or transcribing process, record information about the scanning or transcription undertaken.
- Use a standardised file naming convention for new digital materials and use a unique identifier for files.
- Ensure post processing to link digital image to existing metadata.
- Where possible, use batch control for large datasets to create manageable subsets.
- Consider digital preservation and archive snapshots to create static, retrievable copies.
Note: Any scanning should be done at maximum realistic resolution to facilitate future reuse. Where original materials contain colour content, scan in colour. When original materials are black and white, scan in greyscale as this facilitates further processing e.g. when using optical character recognition (OCR) technologies.
Feedback loop between 2 and 3– Expert input
4.1.4 Box 3 - Data extraction Data extraction involves extracting data, such as the outline of features or text on a map, from either an analogue, scanned or previously digital copy of a piece of archive material. Again, at this stage it may be important to liaise with content experts to agree the best methods for the extraction of content.
Considerations/steps:
- Identify the best method and type of data extraction.
- Gain input from archive and data domain experts and from data processing specialists.
- Assess whether clear examples of content for extraction exist.
- Consider the key information required to go with the data e.g. parameter units, scale information, georeferencing and spatial data standards.
- Consider file naming, format, storage and create a data management plan (DMP) for extracted data.
- Undertake QC during the extraction process and on the extracted data.
Note: Consider how the extraction can best be achieved and whether this is a manual or automated process or if crowd sourcing or citizen science methods can be used? The best extraction process may result from a combination of these methods.
Feedback loop between 3 and 4 – Expert input
Box 4 - Data storage Data storage involves loading and storing extracted data ensuring it is linked back to the source archive index and metadata.
Considerations/steps:
- Implement the DMP.
- Update the original archive summary (abstract, index, metadata, T&C, IPR) to record that extraction has taken place and update/add any associated information.
- Load the data to the storage locations.
- If parametric data, consider what QC checks can be built into loading process.
- If non-parametric data, consider what QC checks can be applied.
Note: Consider how the results will be stored and how quickly storage space may run out. In addition, consider how related, detailed metadata will be stored and how this is linked with the data. Consider how discovery metadata will be created or linked to if it already exists. Ensure the IPR and T&Cs of use are clearly recorded.
Feedback loop between 4 and 5– Expert input
Box 5 - Deliver data
Deliver extracted archive data in a way to meet the original user requirements.
Considerations/steps:
- Check output meets the technical delivery needs and the original user requirements.
- Document the QA and QC audits and checks that have been completed on the data.
- Present the data in a clear way for end users.
- Ensure the initial user requirement considers how the end results will be provided to the key user group(s).
- Ensure appropriate standards are met for data, metadata, accessibility and usability.
- Provide clear statements on data use (IPR/T&Cs).
- Is the data delivered using consumable services to allow online integration?
- Having one master dataset accessed from a specialist central repository for specific data types reduces the need for copies to be distributed.
- Providing a range of desirable outputs from the master dataset ensures that data integrity and contextual information are maintained.
- Is the data discoverable and usable beyond original planned domain?
- Are there file/dataset size considerations? Reduced resolution or compressed files may be required.
- Consider any technical or storage costs, issues or requirements
Feedback loop between 5 and 1a/ 1b – Expert input
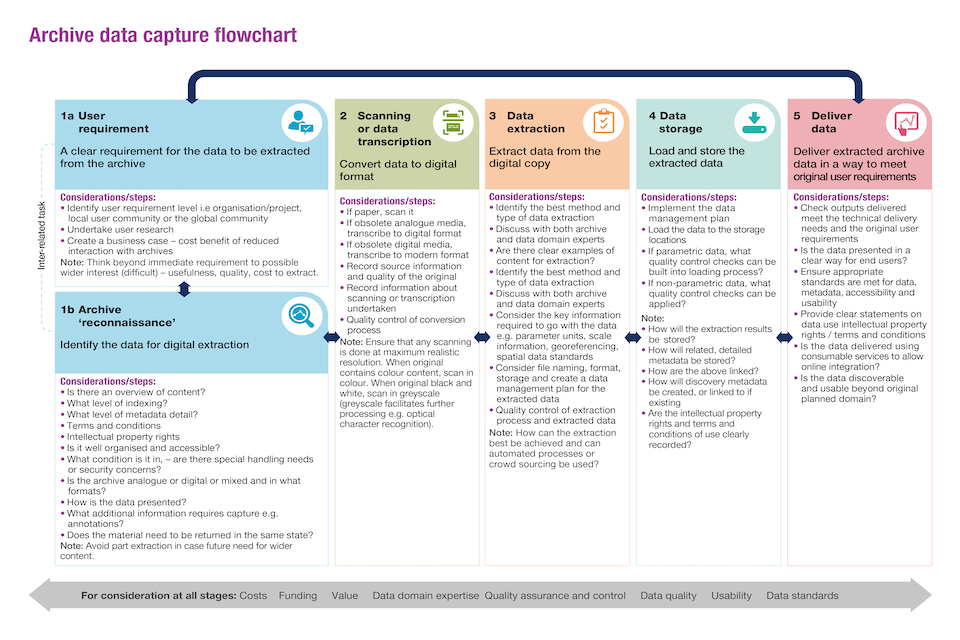
This is a flowchart image which captures key considerations for the best practice of extracting location data from archives. Each section of the flowchart has been detailed in the text above.
Conclusion
Extracting location data from archives can be a unique, complex and resource-intensive undertaking and agencies may take years to develop the experience, skills and knowledge of the stages and requirements for this work.
This report provides a high-level summary of best practice for data extraction from archive materials as captured from activities undertaken as part of the Geospatial Commission Archive Data Capture Methodologies project. This guide is intended to provide an introduction, a starting point and an overview of the individual stages of a data extraction project.
The archive data capture flowchart can be used as a starting point on a data extraction project.
Key considerations throughout a project include costs, funding, data domain expertise, value, QA/QC, data quality, usability and standards.
The stages of a data extraction project include identifying user requirements, undertaking archive reconnaissance, scanning or data transcription, data extraction, data storage and data delivery.
This best practice guide is intended to serve as a quick reference guide to all those doing data capture work and especially to those new to data capture. In addition, this guide is intended as a communication tool to promote this field of work to stakeholders and location data communities.
It is assumed that this best practice guide will be used in conjunction with complementary technical guidelines and standards for relevant components of the workflow.
References
ISAD(G): The International Standard for Archival Description (General) provides guidance for the capture of data from archival records in a consistent format to aid the retrieval of information. There are 6 types of information which are required for an archival description:
Reference code
- Title
- Name of creator
- Dates of creation
- Extent of the document or collection
- Level of description (e.g.collection/file/item)
ISAAR(CPF): The International Standard Archival Authority Record for Corporate Bodies, Persons and Families is a standard used by repositories to more easily share or link information about archival documents created by the same source.
UK Archival Thesaurus: A subject thesaurus created for the UK archive sector to improve access for users by ensuring consistent subject searches. Please note this is no longer being developed and is only updated on a voluntary basis.