Generative AI Framework for HMG (HTML)
Published 18 January 2024
Acknowledgements
The publication of this report has been made possible by the support from a wide number of stakeholders.
Central government department contributions have come from Home Office (HO), Department for Environment, Food & Rural Affairs (DEFRA), Department for Business and Trade (DBT), Foreign, Commonwealth & Development Office (FCDO), Department for Science, Innovation and Technology (DSIT), Cabinet Office (CO), Department for Work and Pensions (DWP), HM Treasury (HMT), HM Revenue and Customs (HMRC), Ministry of Defence (MoD), Ministry of Justice (MoJ), Department for Levelling Up, Housing and Communities (DLUHC), Department of Health and Social Care (DHSC), Department for Transport (DfT), Crown Commercial Service (CCS), Government Legal Department (GLD) and Number 10 Data Science team.
Arms length bodies, devolved administrations and public sector bodies contributions have come from National Health Service (NHS), HM Courts and Tribunals Service (HMCTS), Government Internal Audit Agency (GIAA), Information Commissioner’s Office (ICO), Office for National Statistics (ONS), Driver and Vehicle Licensing Agency (DVLA), Met Office, Government Communications Headquarters (GCHQ) and Scottish Government.
Industry leaders and expert contributions have come from Amazon, Microsoft, IBM, Google, BCG, the Alan Turing Institute, the Oxford Internet Institute, the Treasury Board of Canada Secretariat.
User research participants have come from a range of departments and have been very generous with their time.
Foreword
At the time of writing, it has been a year since generative artificial intelligence (AI) burst into public awareness with the release of ChatGPT. In that time, the ability of this technology to produce text, images and video has captured the imagination of citizens, businesses and civil servants. The last year has been a period of experimentation, discovery and education, where we have explored the potential - and the limitations - of generative AI.
In 2021, the National AI Strategy set out a 10 year vision that recognised the power of AI to increase resilience, productivity, growth and innovation across the private and public sectors. The 2023 white paper A pro-innovation approach to AI regulation sets out the government’s proposals for implementing a proportionate, future-proof and pro-innovation framework for regulating AI. We published initial guidance on generative AI in June 2023, encouraging civil servants to gain familiarity with the technology, while remaining aware of risks. We are now publishing this expanded framework, providing practical considerations for anyone planning or developing a generative AI solution.
Generative AI has the potential to unlock significant productivity benefits. This framework aims to help readers understand generative AI, to guide anyone building generative AI solutions, and, most importantly, to lay out what must be taken into account to use generative AI safely and responsibly. It is based on a set of ten principles which should be borne in mind in all generative AI projects.
This framework differs from other technology guidance we have produced: it is necessarily incomplete and dynamic. It is incomplete because the field of generative AI is developing rapidly and best practice in many areas has not yet emerged. It is dynamic because we will update it frequently as we learn more from the experience of using generative AI across government, industry and society.
It does not aim to be a detailed technical manual: there are many other resources for that: indeed, it is intended to be accessible and useful to non-technical readers as well as to technical experts. However, as our body of knowledge and experience grows, we will add deeper dive sections to share patterns, techniques and emerging best practice (for example prompt engineering. Furthermore, although there are several forms of generative AI, this framework focuses primarily on Large Language Models (LLMs), as these have received the most attention, and have the greatest level of immediate application in government.
Finally, I would like to thank all of the people who have contributed to this framework. It has been a collective effort of experts from government departments, arms-length bodies, other public sector organisations, academic institutions and industry partners. I look forward to continued contributions from a growing community as we gain experience in using generative AI safely, responsibly and effectively.
David Knott,
Chief Technology Officer for Government
Principles
We have defined ten common principles to guide the safe, responsible and effective use of generative AI in government organisations. The white paper A pro-innovation approach to AI regulation, sets out five principles to guide and inform AI development in all sectors. This framework builds on those principles to create ten core principles for generative AI use in government and public sector organisations.
You can find posters on each of the ten principles for you to display in your government organisation.
- Principle 1: You know what generative AI is and what its limitations are
- Principle 2: You use generative AI lawfully, ethically and responsibly
- Principle 3: You know how to keep generative AI tools secure
- Principle 4: You have meaningful human control at the right stage
- Principle 5: You understand how to manage the full generative AI lifecycle
- Principle 6: You use the right tool for the job
- Principle 7: You are open and collaborative
- Principle 8: You work with commercial colleagues from the start
- Principle 9: You have the skills and expertise needed to build and use generative AI
- Principle 10: You use these principles alongside your organisation’s policies and have the right assurance in place
Principle 1: You know what generative AI is and what its limitations are
Generative AI is a specialised form of AI that can interpret and generate high-quality outputs including text and images; opening up the potential for opportunities for organisations, including delivering efficiency savings or developing new language capability.
You actively learn about generative AI technology to gain an understanding of what it can and cannot do, how it can help and the potential risks it poses.
Large language models lack personal experiences and emotions and don’t inherently possess contextual awareness, but some now have access to the internet.
Generative AI tools are not guaranteed to be accurate as they are generally designed only to produce highly plausible and coherent results. This means that they can, and do, make errors. You will need to employ techniques to increase the relevance and correctness of their outputs, and have a process in place to test them. You can find more about what generative AI is in our Understanding generative AI section and what it can and cannot do for you in the Building generative AI solutions section.
Principle 2: You use generative AI lawfully, ethically and responsibly
Generative AI brings specific ethical and legal considerations, and your use of generative AI tools must be responsible and lawful.
You should engage with compliance professionals, such as data protection, privacy and legal experts in your organisation early in your journey. You should seek legal advice on intellectual property equalities implications and fairness and data protection implications for your use of generative AI.
You need to establish and communicate how you will address ethical concerns from the start, so that diverse and inclusive participation is built into the project lifecycle.
Generative AI models can process personal data so you need to consider how you protect personal data, are compliant with data protection legislation and minimise the risk of privacy intrusion from the outset.
Generative AI models are trained on large data sets, which may include biased or harmful material, as well as personal data. Biases can be introduced throughout the entire lifecycle and you need to consider testing and minimising bias in the data at all stages.
Generative AI should not be used to replace strategic decision making.
Generative AI has hidden environmental issues that you and your organisation should understand and consider before deciding to use generative AI solutions. You should use generative AI technology only when relevant, appropriate, and proportionate, choosing the most suitable and sustainable option for your organisation’s needs.
You should also use the AI regulation white paper’s fairness principle, which states that AI systems should not undermine the legal rights of individuals and organisations. And that they should not discriminate against individuals or create unfair market outcomes.
You can find out more in our Using generative AI safely and responsibly section.
Principle 3: You know how to keep generative AI tools secure
Generative AI tools can consume and store sensitive government information and personal identifiable information if the proper assurances are not in place. When using generative AI tools, you need to be confident that your organisation’s data is held securely; that the generative AI tool can only access the parts of your organisation’s data that it needs for its task.
You need to ensure that private or sensitive data sources are not being used to train generative AI models without the knowledge or consent of the data owner.
Generative AI tools are often hosted in places outside your organisation’s secure network. You must make sure that you understand where the data you give to a generative AI tool is processed, and that it is not stored or accessible by other organisations.
Government data can contain sensitive and personal information that must be processed lawfully, securely and fairly at all times. Your approach must comply with the data protection legislation.
You need to build in safeguards and put technical controls in place, this includes: content filtering to detect malicious activity and validation checks to ensure responses are accurate and do not leak data.
You can find out more in our Security, Data protection and privacy, and Building the solution sections.
Principle 4: You have meaningful human control at the right stage
When you use generative AI you need to make sure that there are processes for quality assurance controls which include an appropriately trained and qualified person to review your generative AI tool’s outputs and validation of all decision making that generative AI outputs have fed into.
When you use generative AI to embed chatbot functionality into a website, or other uses where the speed of a response to a user means that a human review process is not possible, you need to be confident in the human control at other stages in the product life-cycle. You must have fully tested the product before deployment, and have robust assurance and regular checks of the live tool in place. Since it is not possible to build models that never produce unwanted or fictitious outputs (i.e. hallucinations), incorporating end-user feedback is vital. Put mechanisms into place that allow end-users to report content and trigger a human review process.
You can find out more in our Ethics, Data protection and privacy, Building The solution and Security sections.
Principle 5: You understand how to manage the full generative AI lifecycle
Generative AI tools, like other technology deployments, have a full project lifecycle that you need to understand.
You and your team must know how to choose a generative AI tool and how to set it up. You need to have the right resource in place to support day-to-day maintenance of the tool; you need to know how to update the system, and how to close the system securely down at the end of your project.
You need to understand how to monitor and can mitigate generative AI drift, bias and hallucinations. You have a robust testing and monitoring process in place to catch these problems.
You should use the Technology code of practice to build a clear understanding of technology deployment lifecycles, and understand and use the NCSC cloud security principles.
You should understand the benefits, other use cases and applications that your solution could support across government. The Rose Book provides guidance on government-wide knowledge assets and The Government Office for Technology Transfer can provide support and funding to help develop government-wide solutions.
If you develop a service you must use the Service Standard for government.
You can find out more about development best practices for generative AI in our Building the solution section.
Principle 6: You use the right tool for the job
You should ensure you select the most appropriate technology to meet your needs. Generative AI is good at many tasks but has a number of limitations and can be expensive to use. You should be open to solutions using generative AI as they can allow organisations to develop new or faster approaches to the delivery of public services, and can provide a springboard for more creative and innovative thinking about policy and public sector problems. You can create more space for you and your people to problem solve by using generative AI to support time-consuming administrative tasks.
When building generative AI solutions you should make sure that you select the most appropriate deployment patterns and and choose the most suitable generative AI model for your use case.
You can find out about how to choose the right generative AI technology for your task or project in our Identifying the right use cases, Patterns, Picking your tools and Things to consider when evaluating LLMs sections.
Principle 7: You are open and collaborative
There are lots of teams across government who are interested in using generative AI tools in their work. Your approach to any generative AI project should make use of existing cross-government communities, where there is a space to solve problems collaboratively.
You should identify which groups, communities, civil societies, Non-governmental Organisations (NGOs), academic organisations and public representative organisations have an interest in your project. You should have a clear plan for engaging and communicating with these stakeholders at the start of your work.
You should seek to join cross-government communities and engage with other government organisations. Find other departments who are trying to address similar issues and learn from them, and also share your insights with others. You should reuse ideas, code and infrastructure where possible.
Any automated response visible to the public such as via a chatbot interface or email should be clearly identified as such (e.g. “This response has been written by an automated AI-chatbot”)
You should be open with the public about where and how algorithms and AI systems are being used in official duties (e.g. GOV.UK digital blogs). The UK Algorithmic Transparency Recording Standard (ATRS) provides a standardised way to document information about the algorithmic tools being used in the public sector with the aim to make this information clearly accessible to the public.
You can find out more in our Ethics section.
Principle 8: You work with commercial colleagues from the start
Generative AI tools are new and you will need specific advice from commercial colleagues on the implications for your project. You should reach out to commercial colleagues early in your journey to understand how to use generative AI in line with commercial requirements.
You should work with commercial colleagues to ensure that the expectations around the responsible and ethical use of generative AI are the same between in-house developed AI systems and those procured from a third party. For example, procurement contracts can require transparency from the supplier on the different information categories as set out in the Algorithmic Transparency Recording Standard (ATRS).
You can find out more in our Buying generative AI section.
Principle 9: You have the skills and expertise needed to build and use generative AI
You should understand the technical requirements for using generative AI tools, and have them in place within your team.
You should know that generative AI requires an understanding of new skills such as prompt engineering and you, or your team, should have the necessary skill set.
You should take part in available civil service learning courses on generative AI, and proactively keep track of developments in the field.
You can find out more in our Acquiring skills section.
Principle 10: You use these principles alongside your organisation’s policies and have the right assurance in place
These principles and this framework set out a consistent approach for the use of generative AI tools for UK government. While you should make sure that you use these principles when working with generative AI, many government organisations have their own governance structures and policies in place, and you also should follow any organisation-specific policies.
You need to understand, monitor and mitigate the risks that using a generative AI tool can bring. You need to connect with the right assurance teams in your organisation early in the project lifecycle for your generative AI tool.
You need to have clearly documented review and escalation processes in place, this might be a generative AI review board, or a programme-level board.
You can find out more in our Governance section.
Understanding generative AI
This section explains what generative AI is, the applications of generative AI in government and the limitations of generative AI and LLMs.
It supports: Principle 1: You know what generative AI is and what its limitations are.
This section is centred on explaining generative AI and its limitations. You can find explanations of the core concepts around managing, choosing and developing generative AI solutions in the Building generative AI Solutions section.
What is generative AI?
Generative AI is a form of Artificial Intelligence (AI) - a broad field which aims to use computers to emulate the products of human intelligence - or to build capabilities which go beyond human intelligence.
Unlike previous forms of AI, generative AI produces new content, such as images, text or music. It is this capability, particularly the ability to generate language, which has captured the public imagination, and creates potential applications within government.
Generative AI fits within the broader field of AI as shown below:
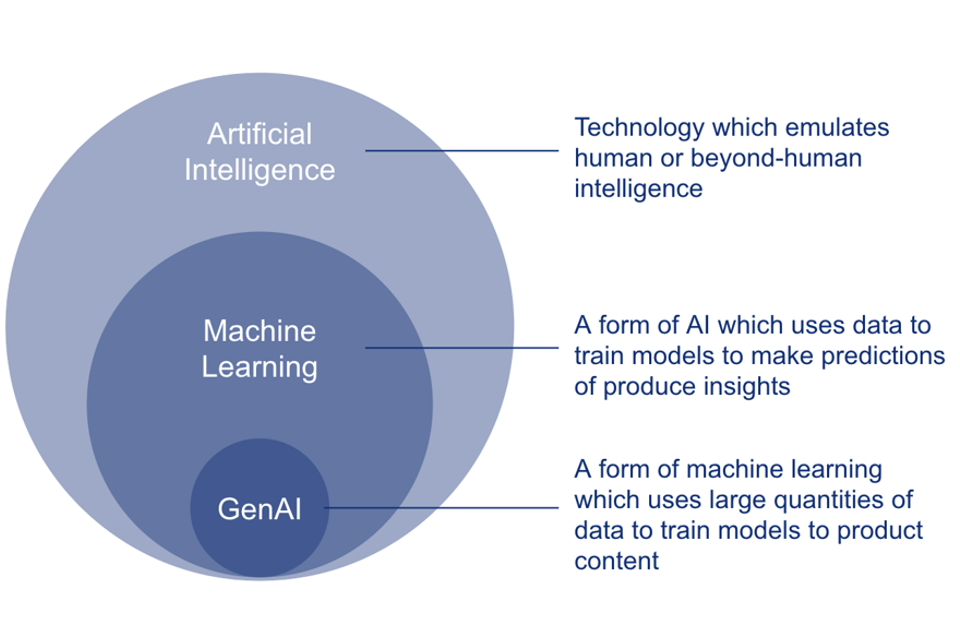
Models which generate content are not new, and have been a subject of research for the last decade. However, the launch of ChatGPT in November 2022 increased public awareness and interest in the technology, as well as triggering an acceleration in the market for usable generative AI products. Other well known generative AI applications include Claude, Bard, Bedrock, and Dall-E. These applications are a type of generative AI known as a Large Language Model (LLM).
Public LLM interfaces fit within the field of generative AI as shown below:
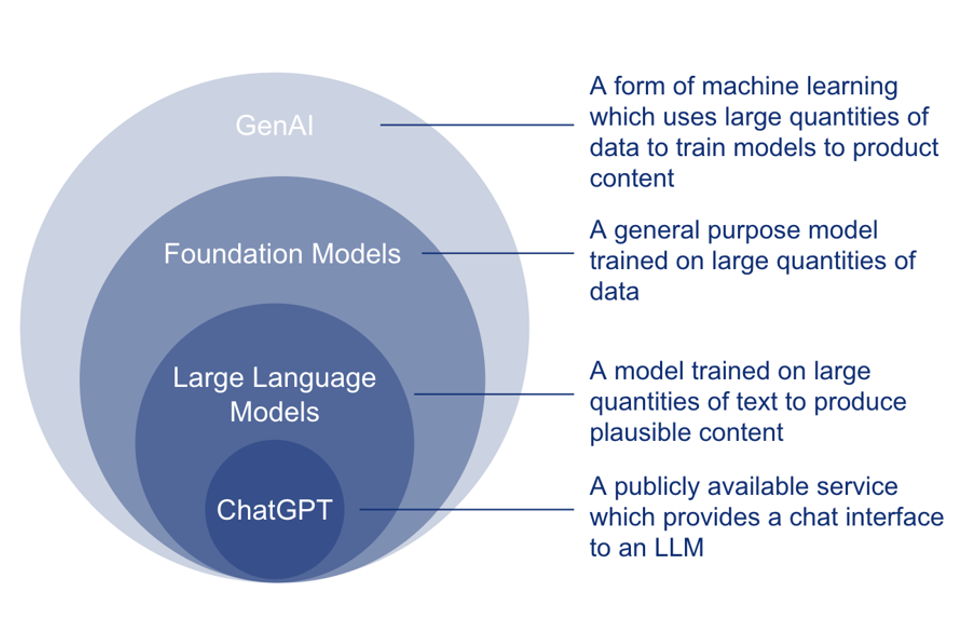
Foundation models are large neural networks trained on extremely large datasets to produce responses which resemble those datasets. Foundation models may not necessarily be language-based, and they could have been trained on non-text data, e.g. biochemical information.
Large Language Models (LLMs) are foundation models specifically trained on text and natural language data to generate high-quality text based outputs.
User interfaces for foundation models & LLMs, are user-friendly ways that people without technical experience can use foundation models or LLMs. ChatGPT and Bard are examples of these, at present they are mostly accessed by tool-specific URLs, but they are likely to be embedded into other consumer software and tools in the near future.
Generative AI works by using large quantities of data, often harvested from the internet, to train a model in the underlying patterns and structure of that data. After many rounds of training, sometimes involving machines only, sometimes involving humans, the model is capable of generating new content, similar to the training examples.
When a user provides a prompt or input, the AI evaluates the likelihood of various possible responses based on what it has learned from its training data. It then selects and presents the response that has the highest probability of being the right fit for the given prompt. In essence, it uses its training to choose the most appropriate response for the user’s input.
Applications of generative AI in government
Despite their limitations, the ability of LLMs to process and produce language is highly relevant to the work of government, and could be used to:
- Speed up delivery of services: retrieving relevant organisational information faster to answer citizen digital queries or routing email correspondence to the right parts of the business.
- Reduce staff workload: suggesting first drafts of routine email responses or computer code to allow people more time to focus on other priorities.
- Perform complicated tasks: helping to review and summarise huge amounts of information.
- Improve accessibility of government information: improving the readability and accessibility of information on webpages or reports.
- Perform specialist tasks more cost-effectively: summarising documentation that contains specialist language like financial or legal terms, or translating a document into several different languages.
However, LLMs and other forms of generative AI still have limitations: you should make sure that you understand these, and that you build appropriate testing and controls into any generative AI solutions.
Limitations of generative AI and LLMs
LLMs predict the next word in a sequence: they don’t understand the content or meaning of the words beyond how likely they are to be used in response to a particular question. This means that even though LLMs can produce plausible responses to requests, there are limitations on what they can reliably do.
You need to be aware of these limitations, and have checks and assurance in place when using generative AI in your organisation.
- Hallucination (also called confabulation): LLMs are primarily designed to prioritise the appearance of being plausible rather than focusing on ensuring absolute accuracy, frequently resulting in the creation of content that appears plausible but may actually be factually incorrect.
- Critical thinking and judgement: Although LLMs can give the appearance of reasoning, they are simply predicting the next most plausible word in their output, and may produce inaccurate or poorly-reasoned conclusions.
- Sensitive or ethical context: LLMs can generate offensive, biased, or inappropriate content if not properly guided, as they will replicate any bias present in the data they were trained on.
- Domain expertise: Unless specifically trained on specialist data, LLMs are not true domain experts. On their own, they are not a substitute for professional advice, especially in legal, medical, or other critical areas where precise and contextually relevant information is essential.
- Personal experience and context: LLMs lack personal experiences and emotions. Although their outputs may appear as if they come from a person, they do not have true understanding or a consciousness.
- Dynamic real-time information retrieval: LLMs do not always have real-time access to the Internet or data outside their training set. However, this feature of LLM products is changing: as of October 2023, ChatGPT, Bard and Bing have been modified to include access to real-time Internet data in their results.
- Short-term memory: LLMs have a limited context window. They might lose track of the context of a conversation if it’s too long, leading to incoherent responses.
- Explainability: Generative AI is based on neural networks, which are so-called ‘black boxes’. This makes it difficult or impossible to explain the inner workings of the model which has potential implications if in the future you are challenged to justify decisioning or guidance based on the model.
These limitations mean that there are types of use cases where you should currently avoid using generative AI, such as safety-of-life systems or those involving fully automated decision-making which affects individuals.
However, the capabilities and limitations of generative AI solutions are rapidly changing, and solution providers are continuously striving to overcome these limitations. This means that you should make sure that you understand the features of the products and services you are using and how they are expected to change.
Building generative AI solutions
This section outlines the practical steps you’ll need to take in building generative AI solutions, including defining the goal, building the team, creating the generative AI support structure, buying generative AI and building the solution.
It supports:
- Principle 1: You know what generative AI is and what its limitations are
- Principle 3: You know how to keep generative AI tools secure
- Principle 4: You have meaningful human control at the right stage
- Principle 5: You understand how to manage the full generative AI lifecycle
- Principle 6: You use the right tool for the job
- Principle 8: You work with commercial colleagues from the start
- Principle 9: You have the skills and expertise needed to build and use generative AI
However, following the guidance in this section is only part of what is needed to build generative AI solutions: you also need to make sure that you are using generative AI safely and responsibly.
Defining the goal
Like all technology, using generative AI is a means to an end, not an objective in itself. Whether planning your first use of generative AI or a broader transformation programme, you should be clear on the goals you want to achieve and particularly, where you could use generative AI.
Goals for the use of generative AI may include improved public services, improved productivity, increased staff satisfaction, increased quality, cost savings and risk reduction. You should make sure you know which goal you are seeking, and how you will measure outcomes.
Identifying use cases
When thinking about how you could leverage generative AI in your organisation you need to consider the possible situations or use cases. The identification of potential use cases should be led by business needs and user needs, rather than directed by what the technology can do. Encourage business units and users to articulate their current challenges and opportunities. Take the time to thoroughly understand users and their needs as per the Service Manual to make sure you are solving the right problems. Try to focus on use cases that can only be solved by generative AI or where generative AI offers significant advantages above existing techniques.
The use of generative AI is still evolving, but the most promising use cases are likely to be those which aim to:
- Support digital enquiries: enable citizens to express their needs in natural language online, and help them find the content and services which are most helpful to them.
- Interpret requests: analyse correspondence or voice calls to understand citizens’ needs, and route their requests to the place where they can best get help.
- Enhanced search: quickly retrieving relevant organisational information or case notes to help answer citizen’s queries.
- Synthesise complex data: help users to understand large amounts of data and text, by producing simple summaries.
- Generate output: produce first drafts of documents and correspondence.
- Assist software development: support software engineers in producing code, and understanding complex legacy code.
- Summarise text and audio: converting emails and records of meetings into structured content, saving time in producing minutes and keeping records.
- Improve accessibility: support conversion of content from text to audio, and translation between different languages.
Use cases to avoid
Given the current limitations of generative AI, there are many use cases where its use is not yet appropriate, and which should be avoided:
- Fully automated decision-making: any use cases involving significant decisions, such as those involving someone’s health or safety, should not be made by generative AI alone.
- High-risk /high-impact applications: generative AI should not be used on its own in high-risk areas which could cause harm to someone’s health, safety, fundamental rights, or to the environment.
- Low-latency applications: generative AI operates relatively slowly compared to other computer systems, and should not be used in use cases where an extremely rapid, low-latency response is required.
- High-accuracy results: generative AI is optimised for plausibility rather than accuracy, and should not be relied on as a sole source of truth, without additional measures to ensure accuracy.
- High-explainability contexts: like other solutions based on neural networks, the inner workings of a generative AI solution may be difficult or impossible to explain, meaning that it should not be used where it is essential to explain every step in a decision.
- Limited data contexts: The performance of generative AI depends on large quantities of training data. Systems that have been trained on limited quantities of data, for example in specialist areas using legal or medical terminology, may produce skewed or inaccurate results.
This list is not exhaustive: you should make sure that you understand the limitations of generative AI, as well as the features and roadmap of the products and services you are using.
Practical recommendations
- Define clear goals for your use of generative AI, and ensure they are consistent with your organisation’s AI roadmap.
- Select use cases which meet a clear need and fit the capabilities of generative AI.
- Understand the limitations of generative AI, and avoid high-risk use cases.
- Find out what use cases other government organisations are considering and see if you can share information or reuse their work.
Building the team
While public-facing generative AI services such as ChatGPT are easy to use and access, building production-grade solutions which underpin services to citizens requires a range of skills and expertise.
You should aim to build a multi-disciplinary team which includes:
- Business leaders and experts who understand the context and impact on citizens and services.
- Data scientists who understand the relevant data, how to use it effectively, and how to build/train and test models.
- Software engineers who can build and integrate solutions.
- User researchers and designers who can help understand user needs and design compelling experiences.
- Support from legal, commercial and security colleagues, as well as ethics and data privacy experts who can help you make your generative AI solution safe and responsible.
You should ensure that you not only have the team in place to build your generative AI solution, but that you have the capability to operate your solution in production.
As well as building a team which contains the right skills, you should strive to ensure that your team includes a diversity of groups and viewpoints, to help you stay alert to risks of bias and discrimination.
Generative AI is a new technology, and even if you have highly experienced experts in your team, they will likely need to acquire new skills.
Acquiring skills
The broad foundational skills required for working in the digital space are outlined in the digital, data and technology capability framework including data roles, software development and user-centred design. To help you acquire the more specific skills needed to build and run generative AI solutions, we have defined a set of open learning resources available to all civil servants from within Civil Service Learning:
- Generative AI - Introduction: in this course you will learn what generative AI is, what the main generative AI applications are, and their capabilities and potential applications across various domains. It will also cover the limits and risks of generative AI technologies, including ethical considerations.
- Generative AI - Risks and ethics: in this course you will learn about the generic risks and technical limitations of AI technologies. You will consider the ethical implications of using AI, including the issues of bias, fairness, transparency and potential misuse. The course also includes the dos and don’ts of using generative AI in government.
- Generative AI - Tools and applications: in this course you will learn about the most important generative AI tools and their functionalities.
- Generative AI - Prompt engineering: in this course you will learn what prompt engineering is and how it can be used to improve the accuracy of generative AI tools.
- Generative AI - Strategy and governance: in this course you will learn how to evaluate the business value of AI and assess its potential impact on organisational culture and governance to develop a holistic AI strategy.
- Generative AI - Technical curriculum: in this course you will learn about the functionalities of various AI technologies and cloud systems, including copilots. You will also consider how to address technical and innovation challenges concerning the implementation and training of generative AI to generate customised outcomes.
A series of off-the-shelf courses on more specific aspects of generative AI has been made available on Prospectus online through the Learning Framework.
You should tailor your learning plan to meet the needs of five groups of learners:
- Beginners: All civil servants who are new to generative AI and need to gain an understanding of its concepts, benefits, limitations and risks. The suggested courses provide an introduction to generative AI, and do not require any previous knowledge.
- Operational delivery and policy professionals: Civil servants who primarily use generative AI for information retrieval and text generation purposes. The recommended resources provide the necessary knowledge and skills to make effective and responsible use of appropriate generative AI tools.
- Digital and technology professionals: Civil servants with advanced digital skills who work on the development of generative AI solutions in government. The suggested learning opportunities address the technical aspects and implementation challenges associated with fostering generative AI innovation.
- Data and analytics professionals: Civil servants who work on the collection, organisation, analysis and visualisation of data. The recommended resources focus on the use of generative AI to facilitate automated data analysis, the synthesis of complex information, and the generation of predictive models.
- Senior Civil Servants: Decision-makers who are responsible for creating a generative AI-ready culture in government. These resources and workshops help understand the latest trends in generative AI, and its potential impact on organisational culture, governance, ethics and strategy.
Practical recommendations
- Make full use of the training resources available, including those available on Civil Service Learning.
- Build a multi-disciplinary team with all the expertise and support you need.
Creating the generative AI support structure
As generative AI is a new technology, you should make sure that you have the structures in place to support its adoption. These structures do not need to be fully mature before your first project: indeed, your experience in your first project will shape the way you organise these structures. However, you should ensure that you have sufficient control to make your use of generative AI safe and responsible.
The supporting structures required for effective generative AI adoption are the same as those required to support the broader adoption of other forms of AI. If your organisation is already using other forms of AI, these structures may already be in place.
If you do not already have them in place, you should consider establishing:
- AI strategy and adoption plan: a clear statement of the way that you plan to use AI within your organisation, including impact on existing organisation structures and change management plans.
- AI principles: a simple set of top level principles which embody your values and goals, and which can be followed by all people building solutions.
- AI governance board: a group of senior leaders and experts to set principles, and to review and authorise uses of AI which fit these principles.
- Communication strategy: your approach for engaging with internal and external stakeholders, to gain support, share best practice and show transparency.
- AI sourcing and partnership strategy: definition of which capabilities you will build within your own organisation and which you will seek from partners.
Practical recommendations
- Identify the support structures you need for your level of maturity and adoption.
- Reuse support structures which are already in place for AI and other technologies.
- Adapt your support structures based on practical experience.
Buying generative AI
The generative AI market is still new and developing engagement with commercial colleagues is particularly important to discuss partners, pricing, products and services.
Crown Commercial Service can guide you through existing guidance, routes to market, specifying your requirements, and[running the procurement process. They can also help you navigate procurement in an emerging market and regulatory and policy landscape, as well as ensure that your procurement is aligned with ethical principles.
Existing guidance
There is detailed guidance to support the procurement of AI in the public sector. You should familiarise yourself with this guidance and make sure you’re taking steps to align with best practice.
-
Guidelines for AI procurement - provides a summary of best practice when buying AI technologies in government:
- Preparation and planning: Getting the right expertise, data assessments and governance, AI impact assessment and market engagement
- Publication: Problem statements, specification, avoiding vendor lock-in
- Selection, evaluation and award: Setting robust criteria, ensuring you have the correct expertise
- Contract implementation and ongoing management: Managing your service, testing for security and how to handle end of life considerations
- Digital, Data & Technology (DDaT) playbook - provides general guidance on sourcing and contracting for digital and data projects and programmes, which all central government departments and their arms length bodies are expected to follow on a ‘comply or explain’ basis. It includes specific guidance on AI and machine learning, as well as Intellectual Property Rights (IPR).
- Sourcing playbook - defines the commercial process as a whole and includes key policies and guidance for making sourcing decisions for the delivery of public services.
- Rose Book - provides guidance on managing and exploiting the wider value of knowledge assets (including software, data and business processes). Annex D contains specific guidance on managing these in procurement.
Routes to market
Consider the available routes to market and commercial agreements, and determine which one is best to run your procurement through based on your requirements.
There are a range of routes to market to purchase AI systems. Depending upon the kind of challenges you’re addressing, you may prefer to use a Framework or a Dynamic Purchasing System (DPS). A ‘Find a Tender Service’ (FTS) procurement route also exists which may be an option for bespoke requirements or contractual terms, or where there is no suitable standard offering.
Crown Commercial Service (CCS) offers a number of compliant Frameworks and DPSs for the public sector to procure AI.
A summary of the differences between a Framework agreement and DPS is provided below, with further information available at www.crowncommercial.gov.uk, and more information on use of frameworks in the Digital, Data & Technology (DDaT) Playbook.
Category | Framework | DPS |
---|---|---|
Supplier access | Successful suppliers are awarded to the framework at launch. Closed to new supplier registrations. Prime suppliers can request to add new subcontractors. | Open for new supplier registrations at any time. |
Structure | Often divided into lots by product or service type. | Suppliers filterable by categories. |
Compliance | Thorough ongoing supplier compliance checks carried out by CCS, meaning buyers have less to do at call-off. (excluding GCloud) | Basic compliance checks are carried out by CCS, allowing the buyer to complete these at the call-off. |
Buying options | Various options, including direct award, depending on the agreements. | Further competition only. |
A number of CCS agreements include AI within their scope, for example:
Dynamic Purchasing Systems:
Frameworks:
- Big Data & Analytics
- GCloud 13
- Technology Products & Associated Services 2
- Technology Services 3
- Back Office Software
- Cloud Compute 2
In addition to commercial agreements, CCS has signed a number of Memorandum of Understanding (MoU) with suppliers. These MoUs set out preferential pricing and discounts on products and services across the technology landscape, including cloud, software, technology products and services and networks. MoU savings can be accessed through any route to market.
To find out more or for support, please contact info@crowncommercial.gov.uk.
Specifying Your requirements
When buying AI products and services, you will need to document your requirements to tell your suppliers what you need. Read the CCS guide on ‘How to write a specification’ for more details.
When drafting requirements for generative AI, you should:
- Start with your problem statement
- Highlight your data strategy and requirements
- Focus on data quality, bias (mitigation) and limitations
- Underline the need for you to understand the supplier’s AI approach
- Consider strategies to avoid vendor lock-in
- Apply the data ethics framework principles and checklist
- Mention any integration with associated technologies or services
- Consider your ongoing support and maintenance requirements
- Consider data format of your organisation and provide suppliers with dummy data where possible
- Provide guidance on budget to consider hidden costs
- Consider who will have intellectual property rights if new software is developed.
- Consider any acceptable liabilities and appetite for risk, to match against draft terms & conditions, once provided
For further information and detail, read the Selection, Evaluation and Award section of the Guidelines for AI Procurement.
Running your procurement
Having prepared your procurement strategy, defined your requirements, and selected your commercial agreement, you can now proceed to conduct a ‘call-off’ in accordance with the process set out in the relevant commercial agreement. The commercial agreement will specify whether you can ‘call-off’ by further competition, a direct award or either.
CCS offers buyer guidance tailored to each of its agreements, which describe each step in detail, including completing your order contract and compiling your contract.
Detailed guidance on planning and running procurements is available in the Digital, Data & Technology (DDaT) Playbook.
Procurement in an emerging market
Commercial agreements
AI is an emerging market. As well as rapidly evolving technology, there are ongoing changes in the supply base and the products and services it offers. Dynamic Purchasing Systems (DPSs) offer flexibility for new suppliers to join, which often complement these dynamics well for buyers.
Any public sector buyers interested in shaping Crown Commercial Service’s (CCS) longer term commercial agreement portfolio should express their interest via info@crowncommercial.gov.uk.
Regulation and policy
Regulation and policy will also evolve to keep pace. However, there are already a number of legal and regulatory provisions which are relevant to the use of AI technologies, including:
- UK data protection law: regulation around automated decision making, processing personal data, processing for the purpose of developing and training AI technologies. In November 2022, a new Procurement Policy Note was published to provide an update to this: PPN 03/22 Updated guidance on data protection legislation.
- Online Safety Act: provisions concerning design and use of algorithms are to be included in a new set of laws to protect children and adults online. It will make social media companies more responsible for their users’ safety on their platforms.
- A pro-innovation approach to AI regulation: this white paper published in March 2023, sets out early steps towards establishing a regulatory regime for AI. The white paper outlines a proportionate pro-innovation framework, including five principles to guide responsible AI innovation in all sectors.
- Centre for Data Ethics and Innovation (CDEI) AI assurance techniques: The portfolio of AI assurance techniques has been developed by the Centre for Data Ethics and Innovation (CDEI), initially in collaboration with techUK. The portfolio is useful for anybody involved in designing, developing, deploying or procuring AI-enabled systems. It shows examples of AI assurance techniques being used in the real-world to support the development of trustworthy AI.
Further guidance is also from the Information Commissioner’s Office (ICO), Equality and Human Rights Commission (EHRC), Medicines and Healthcare products Regulation Authority (MHRA) and the Health and Safety Executive (HSE).
Aligning procurement and ethics
It’s important to consider and factor in data ethics into your commercial approach from the outset. A range of guidance relating specifically to AI and data ethics is available to provide guidance for public servants working with data and/or AI. This collates existing ethical principles, developed by government and public sector sector bodies.
- The Data ethics framework outlines appropriate and responsible data use in government and the wider public sector. The framework helps public servants understand ethical considerations, address these within their projects, and encourages responsible innovation.
- Data ethics checklist: CCS has created a checklist for suppliers to follow that will mitigate bias and ensure diversity in development teams, as well as transparency/ interpretability and explainability of the results.
- The Public Sector Contract (PSC) includes a number of provisions relating to AI and data ethics.
For further information, please see Data protection and privacy, Ethics and Regulation and policy sections.
Practical recommendations
- Engage your commercial colleagues from the outset.
- Understand and make use of existing guidance.
- Understand and make use of existing routes to market, including Frameworks, Dynamic Purchasing Systems and Memoranda of Understanding.
- Specify clear requirements and plan your procurement carefully.
- Seek support from your commercial colleagues to help navigate the evolving market, regulatory and policy landscape.
- Ensure that your procurement is aligned to ethical principles.
Building the solution
Core concepts
Generative AI provides a wide breadth of capability, and a key part of designing and building a generative AI solution will be to get it to behave accurately and reliably. This section sets out key concepts that you need to understand to design and build generative AI solutions that meet your needs.
- Prompts are the primary input provided to an LLM. In the most simple case, a prompt may only be the user-prompt. In production systems, a prompt will have additional parts, such as meta-prompts, the chat history, and reference data to support explainability.
- Prompt engineering describes the process of adjusting LLM input to improve performance and accuracy. In its simplest form it may be testing different user-prompt formulations. In production systems, it will include adjustments, such as adding meta-prompts, provision of examples and data sources, and sometimes parameter tuning.
- User-prompts are whatever you type into e.g. a chat box. They are generally in the everyday natural language you use, e.g. ‘Write a summary of the generative AI framework’.
- Meta-prompts (also known as system prompts) are higher-level instructions that help direct an LLM to respond in a specific way. They can be used to instruct the model on how to generate responses to user-prompts, provide feedback, or handle certain types of content.
- Embedding is the process of transforming information such as words, or images into numerical values and relationships that the computer algorithms can understand and manipulate. Embeddings are typically stored in vector databases (see below).
- Retrieval Augmentation Generation (RAG) is a technique which uses reference data stored in vector databases (i.e. the embeddings) to ground a model’s answers to a user’s prompt. You could specify that the model cites its sources when returning information.
- Vector databases index and store data such as text in an indexed format easily searchable by models. The ability to store and efficiently retrieve information has been a key enabler in the progress of generative AI technology.
- Grounding is the process of linking the representations learned by the AI models to real-world entities or concepts. It is essential for making AI models understand and relate its learned information to real-world concepts. In the context of large language models, grounding is often achieved by a combination of prompt engineering, parameter tuning, and RAG.
- Chat history is a collection of prompts and responses. It is limited to a session. Different models may allow different session sizes. For example, Bing search sessions allow up to 30 user-prompts. The chat history is the memory of LLMs. Outside of the chat history LLMs are “stateless”. That means the model itself does not store chat history. If you wanted to permanently add information to a model you would need to fine-tune an existing model (or train one from scratch).
- Parameter tuning is the process of optimising the performance of the AI model for a specific task or data set by adjusting configuration settings.
- Model fine-tuning is the process of limited re-training of a model on new data. It can be done to enforce a desired behaviour. It also allows us to add data sets to a model permanently. Typically, fine-tuning will adjust only some layers of the model’s neural network. Depending on the information or behaviour to be trained, fine-tuning may be more expensive and complicated than prompt engineering. Experience with model tuning in HMG is currently limited and we are looking to expand on this topic in a future iteration of this framework.
- Open source models are publicly accessible, and their source code, architecture, and parameters are available for examination and modification by the broader community.
- Closed source models on the other hand, are proprietary and not openly accessible to the public. The inner workings and details of these models are kept confidential and are not shared openly.
Practical recommendations
Learn about generative AI technology; read articles, watch videos and undertake short courses. See section on acquiring skills.
Patterns
Generative AI can be accessed and deployed in many different ways or patterns. Each pattern provides different benefits and presents a different set of security challenges, affecting the level of risk that you must manage.
This section explains patterns and approaches as the main ways that you are likely to use and encounter generative AI, including:
- Public generative AI applications and web services
- Embedded generative AI applications
- Public generative AI APIs
- Local development
- Cloud solutions
Public generative AI applications and web services
Applications like OpenAI’s ChatGPT, Google’s Bard, Microsoft’s Bing search, are the consumer side of generative AI. They have a simple interface, where the user types in a text prompt and is presented with a response. This is the simplest approach, with the benefit that users are already familiar with these tools.
Many LLM providers offer web services free of charge, allowing users to experiment and interact with their models. Generally, you’ll just need an email address to sign up.
There are a few things you’ll need to consider before signing up to a generative AI web service:
- You must make sure you’re acting in line with the policies of your organisation.
- While the use of these web services is free of charge, you should be aware that any information provided to these services may be made publicly available and/or used by the provider. Make sure you have read and understood the terms of service.
- Generative AI web services and applications are often trained using unfiltered material on the internet. This means that they can reproduce any harmful or biassed material that they have found online. You can learn more about bias and how to use generative AI safely in the Using generative AI safely and responsibly section.
- Generative AI web services and applications may produce unreliable results, you should not trust any factual information provided without a validated reference.
Embedded generative AI applications
LLMs are now being embedded, or integrated, into existing and popular products; embedded generative AI allows people to use language-based prompts to ask questions about their organisation’s data, or for specific support on a task.
Embedded generative AI tools provide straightforward user interfaces in products that people are already familiar with. They can be a very simple way to bring Generate AI into your organisation. Examples of embedded generative AI tools include:
- Adobe Photoshop Generative Fill tool: helps with image editing by adding or removing components.
- Github Copilot and AWS CodeWhisperer: helps to develop code by providing auto-complete style suggestions.
- AWS ChatOps: an AI assistant that can help to manage an AWS cloud environment.
- Microsoft 365 Copilot: an AI assistant that can support use of Microsoft products.
- Google Duet AI: an AI assistant that can support the use of Google products including writing code.
You must be certain you understand the scope of access and data processing of these services. Most enterprise licenced services will assure your control over your data. However, supporting services like abuse monitoring may still retain information for processing by the vendors.
If data sovereignty is a concern, you must also clarify the data processing geolocation with a vendor.
LLMs that are integrated into organisations existing enterprise licences may have access to the data that’s held by your organisation by default. Before enabling a service, you must understand what data an embedded generative AI tool has access to in your organisation.
The use of code assistance tools requires the addition of integrated development environment (IDE) or editor plugins. You must be certain to only use official plugins. If you use a coding assistant to generate a complex algorithm, it may be necessary to verify the licensing status manually by searching for the code on the internet to double-check you’re not inadvertently violating any copyrights or licences.
Public generative AI APIs
Most big generative AI applications will offer an Application Programming Interface (API). This allows developers to integrate generative AI capabilities directly into solutions they build. It takes only a few lines of code to build a plugin to extend the features of another application.
As with web services, signing up is typically required to obtain an access token. You need to be aware of the terms and conditions of using the API.
By using an API your organisation’s data is still sent over to the provider, and you must be sure that you are comfortable with what happens to it before using an API.
The benefit of using APIs is that you will have greater control over the data. You can intercept the data being sent to the model and also process the responses before returning them to the user. This allows you to e.g.:
- Include privacy enhancing technology (PET) to prevent data leakage
- Add content filters to sanitise the prompts and responses
- Log and audit all interactions with the model
However, you will also need to perform additional tasks commonly performed by the user interface of web and embedded services, such as:
- Maintaining a session history
- Maintaining a chat history
- Developing and maintaining meta-prompts and general prompt engineering
Local development
For rapid prototyping and minimum viable product (MVP) studies, the development on personal or local hardware (i.e. sufficiently powerful laptops) may be a feasible option.
Development best practices like distributed version control systems, automated deployment, and regular backups of development environments are particularly important when working with personal machines.
When working on local development you should consider containerisation and cloud-native technology paradigms like twelve-factor applications. These will help when moving solutions from local hardware into the cloud.
Please note that the recommendation for production systems remains firmly with fully supported Cloud environments.
Cloud solutions
Cloud services provide similar functionality to public and paid-for APIs, often with a familiar web interface with useful tools for experimentation. In addition to compliance with HMG’s Cloud First Policy, their key advantages is that they allow increased control over your data. You can access cloud service providers’ LLMs by signing up through your organisation’s AWS, Microsoft or Google enterprise account.
When establishing your generative AI cloud service, make sure the development environment is compliant with your organisations’ data security policies, governmental guidelines and the Service Standard.
If your organisation and/or use case requires all data to remain on UK soil, you might need to plan in additional time for applying for access to resources within the UK as these may be subject to additional regulation by some providers. Technical account managers and solution architects supporting your enterprise account will be able to help with this step.
Practical recommendations
- Learn about the different patterns and approaches, evaluate them against the needs of your project and users.
- Refer to your organisation’s policies before exploring any use of public generative AI applications or APIs.
- Be aware that any information provided to generative AI web services may be made publicly available and/or used by the provider. Make sure you have read and understood their terms of service.
- Check licensing and speak to suppliers to understand the capabilities and data collection and storage policies for their services; including geographic region if data sovereignty is a concern.
- Before enabling embedded generative AI tools understand what organisational data they would have access to.
- Use only official code assistance plugins.
- Learn from other government organisations who have implemented generative AI solutions.
Picking your tools
In order to develop and deploy generative AI systems you will need to pick the right tools and technology for your organisation. Deciding on the best tools will depend on your current IT infrastructure, level of expertise, risk-appetite and the specific use cases you are supporting.
Decisions on your development stack.
There are a number of technology choices you will need to consider when building your generative AI solutions, including the most appropriate IT infrastructure, which programming languages to use and the best large language model.
- Infrastructure: You should select a suitable infrastructure environment. Microsoft, Google or AWS may be appropriate, depending on your current IT infrastructure or existing partnerships and expertise in your teams. Alternatively, it may be that a specific large language model is considered most appropriate for your particular use case, leading to a particular set of infrastructure requirements.
- As models change and improve, the most appropriate one for your use case may also change, so try to build-in the technical agility to support different models or providers.
Items for consideration include:
- Use of cloud services vs local development. You should be aware of the HMG’s Cloud First Policy, but understand that local development may be feasible for experimentation. Using container technology from the start can help you to move your solution between platforms with minimal overhead.
- Web Services, access modes such as APIs and associated. frameworks, see section on patterns.
- Front-end / user interface and back-end solutions.
- Programming languages.
- Data storage (e.g. Binary Large Object (BLOB) stores and vector stores)
-
Access logging, prompt auditing, and protective monitoring.
-
Programming language: In the context of AI research, Python is the most widely used programming language. While some tools and frameworks are available in other languages, for example LangChain is also available in JavaScript, it is likely that most documentation and community discussion is based on Python examples. If you’re working on a use case that has focused interaction with a generative AI model API endpoint only, the choice of programming language is less important.
- Frameworks: generative AI frameworks are software libraries or platforms that provide tools, APIs, and pre-built models to develop, train, and deploy generative AI models. These frameworks implement various algorithms and architectures, making it more convenient for you to experiment with and create generative models. Example frameworks include LangChain, Haystack, Azure Semantic Kernel and Google Vertex AI pipelines. AWS Bedrock similarly provides an abstraction layer to interact with varied models using a common interface. These frameworks have their own strengths and unique features. However, you should also be aware that their use may increase the complexity of your solution.
The choice of a generative AI framework might depend on:
- Your specific project requirements.
- The familiarity of the developer with the framework and programming language.
- The size and engagement of the community support around it.
Things to consider when evaluating Large Language Models (LLMs)
There are many models currently available so you need to select the most appropriate for your particular use case. The Stanford Center for Research on Foundation Models (CRFM) provides the Holistic Evaluation of Language Models (HELM) to benchmark different models against criteria such as accuracy, robustness, fairness, bias, and toxicity; and can help you to compare the capabilities of a large number of language models. Here are some of the things you should consider:
- Capability: Depending on your use case, conversational foundation models may not be the best fit for you. If you have a domain-specific requirement in sectors like medical or security applications, pre-tuned, specialised models like Google PaLM-2-med and Google PaLM-2-sec may reduce the amount of work required to reach a certain performance level and time to production. Equally, if you’re mainly focused on indexing tasks, BERT-type models may provide better performance compared to GPT-style LLMs.
- Availability: At the time of writing, many LLMs are not available for general public use, or are locked to certain regions. One of the first things to consider when deciding on which model to use is whether implementation in a production environment is possible inline with your organisation’s policy requirements.
- Mode of deployment: Many LLMs are available via a number of different routes. For production applications the use of fully-featured cloud services or operation of open-source models in a fully controlled cloud environment will be a hard requirement for most if not all use cases.
- Cost: Most access to LLMs is charged by the number of tokens (roughly equal to the word count). If your generative AI tool is hosted in a cloud environment, you’ll have to pay additional infrastructure costs. While the operation of open-source models will not necessarily incur a cost per transaction, the operation of GPU-enabled instances is costly as well. Cloud infrastructure best practices like dynamic scaling and shutting down instances outside of working hours will help to reduce these costs.
- Context limits: LLMs often limit the maximum amount of tokens the model can process as a single input prompt. Factors determining the size of prompts are the context window of conversation (if included), the amount of contextual data included via meta-prompting and retrieval augmented generation as well as the expected size of user inputs.
- API Rate limits: Model providers impose limits on how frequently users can make requests through an API. This may be important if your use case leads to a high volume of requests. Software development best practices for asynchronous execution (such as use of contexts and queues) may help to resolve bottlenecks but will increase the complexity of your solution.
- Language capability: If your use case includes multilingual interaction with the model, or if you expect to operate with very domain-specific language using specific legal or medical terminology, you should consider the amount of relevant language-specific training the LLM has received.
- Open vs closed-source (training data, code and weights): If openness is important to your solution, you should consider whether all aspects of the model, including training data, neural network coding and model weights, are available as Open Source. Sites such as Hugging Face host a large collection of models and documentation. Examples of sites that provide open source low-code solutions include Databricks and MosaicML.
- Non-technical considerations: There may be data-protection, legal or ethical considerations which constrain or direct your choice of technology, for example an LLM may have been trained on copyrighted data, or to produce a procedurally fair decision-making system, one solution should be chosen over another.
Practical recommendations
- Select the simplest solutions that meet your requirements, aligned to your IT infrastructure.
- Understand the key characteristics of generative AI products and how they fit your needs, realising that these characteristics may change rapidly in a fast moving market.
- Speak to other government organisations to find out what they have done, to help inform your decisions.
- Conduct ‘well architected’ reviews at appropriate stages of the solutions’ lifecycle.
Getting reliable results
Generative AI technology needs to be carefully controlled and managed in order to ensure the models behave and perform in the way you want them to, reliably and consistently. There are a number of things you can do to help deliver high quality and reliable performance.
- Select your model carefully: In order to achieve a reliable, consistent and cost-effective implementation, the most appropriate model for a particular use case should be chosen.
- Design a clear interface and train users: Ensure your generative AI system is used as intended. Design and develop a useful and intuitive interface your users will interact with. Define and include any required user settings (for example the size of required response). Be clear about the design envelope for generative AI systems i.e. what it has been designed and built to do, and, more importantly, what its limitations are. Ensure your user community is trained in its proper use and fully understand its limitations.
- Evaluate input prompts: User inputs to the generative AI tool can be evaluated with a content filtering system to detect and filter inappropriate inputs. The evaluation of input using deterministic tools may be feasible and could reduce the amount of comparatively expensive calls to a LLM. Alternatively, calls to a smaller and/or classification-specialised LLM may be required. Make sure that the system returns a meaningful error to allow a user to adjust their prompt if rejected. There are some commercially available tools that can provide some of this functionality. Example checks include:
- Identify whether the prompt is abusive or malicious.
- Confirm the prompt is not attempting to jailbreak the LLM, for example by asking the LLM to ignore its safety instructions.
- Confirm no unnecessary Personally Identifiable Information (PII) has been entered.
- Ground your solution: If your use case is looking for the model to provide factual information - as opposed to just taking advantage of a models’ creative language capabilities - you should follow steps to ensure that its responses are accurate, for example by employing Retrieval Augmented Generation (RAG). With RAG you identify useful documentation then extract the important text, break it into ‘chunks’, convert them to ‘embeddings’ and send them to a ‘vector-database’. This relevant information can now be easily retrieved and integrated as part of the model responses.
- A key application of generative AI is working with your organisation’s private data, by enabling the model to access, understand and use the private data, insights and knowledge can be provided to users that is specific to their subject domain. There are different ways to hook a generative AI model into a private data source.
- You could train the model from scratch on your own private data, but this is costly and impractical. Alternatively, you can take a pre-trained model and further train it on your own private data. This is a process called fine-tuning, and is less expensive and time consuming than training a model from scratch.
- The easiest and most cost-efficient approach to augmenting your generative AI model with private data, is to use in-context learning, which means adding domain-specific context to the prompt sent to the model. The limitation here is usually the size of the prompt, and a way around this is to chunk your private data to reduce its size. Then a similarity search can be used to retrieve relevant chunks of text that can be sent as context to the model.
- Use prompt engineering: An important mechanism to shape the model’s performance and produce accurate and reliable results is prompt engineering. Developing good prompts and meta-prompts is an effective way to set the standards and rules for how the user requests should be processed and interpreted, the logical steps the model should follow and what type of response is required. For example, you could include the following:
- Set the tone for the interactions, for example request a chatbot to provide polite, professional and neutral language responses. This will also help to reduce bias.
- Set clear boundaries on what the generative AI tool can, and cannot, respond to. You could specify the requirement for a model to not engage with abusive or malicious inputs, but reject them and instead return an alternative, appropriate response.
- Define the format and structure of the desired output. For example asking for a boolean yes/no response to be provided in JSON format.
- Define guardrails to prevent the assistant from generating inappropriate or harmful content.
For further information see the deep dive section.
- Evaluate outputs: Once a model returns an output, it is important to ensure that its messaging is appropriate. Off-the-shelf content filters may be useful here, as well as classical or generative AI text-classification tools. Depending on the use case, a human might be required to check the output some or all of the time, although the expenditure of time and money to do this needs careful consideration. Accuracy and bias checks on the LLM responses prior to presentation to the user can be used to check and confirm:
- The response is grounded in truth with no hallucinations
- The response does not contain toxic or harmful information
- The response does not contain biased information
- The response is fair and does not unduly discriminate
- The user has permission to access the returned information
- Include humans: There are many good ways that humans can be involved in the development and use of generative AI solutions to help implement reliable and desired outcomes. Humans can be part of the development process to review input data to make sure it is high-quality, to assess and improve model performance and also to review model outputs. If there is a person within the processing chain preventing the system from producing uncontrolled, automated outputs this is called having a ‘human-in-the-loop’.
- Evaluate performance: In order to maintain the performance of the generative AI system, its performance should be continually monitored and evaluated by logging and auditing all interactions with the model.
- Conduct thorough testing to assess the functionality and effectiveness of the system. See section on testing generative AI solutions for further information.
- Record the input prompts and the returned responses.
- Collect and analyse metrics across all aspects of performance: including hallucinations, toxicity, fairness, robustness, and higher-level business key performance indicators.
- Evaluate the collected metrics and validate the model’s outputs against ground truth or expert judgement. Obtain user feedback to understand the usefulness of the returned response. This could be a simple thumbs-up indicator or something more sophisticated.
Practical recommendations
- Assume the model may provide you with incorrect information unless you build in safeguards to prevent it.
- Understand techniques for improving the reliability of models, and that these techniques are developing rapidly.
- Ground the generative AI system in real organisational data, if possible, to improve accuracy.
- Implement extensive testing to ensure the outputs are within expected bounds. It is very easy to develop a prototype, but can be very hard to produce a working and reliable production solution.
Testing generative AI solutions
Generative AI tools are not guaranteed to be accurate as they are designed to produce plausible and coherent results. They generate responses that have a high likelihood of being plausible based on the data that they have processed. This means that they can, and do, make errors. In addition to employing techniques to get reliable results, you should have a process in place to test them.
During the initial experimental discovery phases you should look to assess and improve the existing system until it meets the required performance, reliability and robustness criteria.
- Conduct thorough testing to assess the functionality and effectiveness of the system.
- Record the input prompts and the returned responses, and collect and analyse metrics across all aspects of performance including hallucinations, toxicity, fairness, robustness, and higher-level business key performance indicators.
- Evaluate the collected metrics and validate the model’s outputs against ground truth or expert judgement, obtaining user feedback if possible.
- Closely review the outcomes of the technical decisions made, the infrastructure and running costs and environmental impact. Use this information to continually iterate your solution.
Technical methods and metrics for assessing bias in generative AI are still being developed and evaluated. However, there are existing tools that can support AI fairness testing, such as IBM fairness 360, Microsoft FairLearn, Google What-If-Tool, University of Chicago Aequitas tool, and PyMetrics audit-ai. You should carefully select methods based on the use case, and consider using a combination of techniques to mitigate bias across the AI lifecycle.
Practical recommendations
Establish a comprehensive testing process and continue to test the generative AI solution throughout its use.
Data management
Good data management is crucial in supporting the successful implementation of generative AI solutions. The types of data you will need to manage include:
- Organisational grounding data: LLMs are not databases of knowledge, but advanced text engines.Their contents may also be out of date. To improve their performance and make them more reliable, relevant information can be used to ‘ground’ the responses, for example by employing Retrieval Augmented Generation.
- Reporting data: It is important to maintain documentation, including methodology, description of the design choices and assumptions. Keep records from any architecture design reviews. This can help to support the ability to audit the project and support the transparency of your use of AI. If possible collect metrics to help to estimate any efficiency savings, value to your business and to taxpayers and the return on investment.
- Testing and operational data: All model inputs and outputs should be logged. When collected during testing and development, this information will be used to improve the performance of the system. When collected during use, it will be used to monitor and maintain performance. The recording of the outcomes and any resulting decisions will also help when examining and looking to explain the model results. See testing generative AI solutions section for further details. Additionally, all user engagement of the generative AI systems should be logged to ensure safe and compliant use.
- User feedback: Both during the initial development stage and whilst in use, you should be collecting feedback from users on their interactions with the system. Collecting and storing metrics such as performance, ease of use and occurrences of problematic behaviour (including hallucinations and potential biases etc) to help control and improve the AI system.
- Financial Operations (FinOps) data: The cost of running your generative AI solutions should be monitored closely to ensure you continue to operate cost-effectively, for the given model and prompts.
Data management needs to also address data loss prevention. Consider using privacy enhancing technology to prevent data leakage, and if you process personal identifiable information take action to protect peoples’ data e.g. pseudonymising data to reduce the risk of leaking sensitive information.
Practical recommendations
- Record, store and analyse data on your use of generative AI solutions.
- Carefully consider any use cases which automatically lead to destructive or irreversible actions such as sending emails or modifying records, and whether a person should be part of the process to authorise any proposed changes (called having a ‘human-in-the-loop’).
Using generative AI safely and responsibly
This section outlines the steps you’ll need to ensure that you Build generative AI solutions in a safe and responsible way, taking account of Legal considerations, Ethics, Data protection and privacy, Security and Governance. Many of these considerations interact with each other, so you should read all of these topics together, and seek support from data ethics, privacy, legal and security experts.
It supports:
- Principle 2: You use generative AI lawfully, ethically and responsibly
- Principle 3: You know how to keep generative AI tools secure
- Principle 4: You have meaningful human control at the right stage
Legal considerations
You should seek advice from government legal profession legal advisers who help you to navigate through the use of generative AI in government.
Although generative AI is new, many of the legal issues that surround it are not. For example, many of the ethical principles discussed in this document, such as fairness, discrimination, transparency and bias, have sound foundations in public law. In that way, many of the ethical issues that your team identifies will also be legal issues, and your lawyers will be able to help to guide you through them.
The lawfulness and purpose limitation section provides a framework to ensure that personal data is processed lawfully, securely and fairly at all times. Your lawyers can advise you on that.
You may face procurement and commercial issues when buying generative AI products. Alongside commercial colleagues, your lawyers can help you to navigate those challenges.
When you contact your legal team, you should explain your aims for the generative AI solution, what it will be capable of doing, and any potential risks you are aware of. This will help you to understand, for example, if you need legislation to achieve what you want to do. It will also help to minimise the risk of your work being challenged in court, having unintended - and unethical - consequences or a negative impact on the people you want it to benefit.
Example legal issues
These are example legal issues designed to help you understand when you might want to consider getting legal advice. They should not be read as real legal advice and their application to any given scenario will be fact specific. You should always consult your departmental lawyer if in doubt.
Data protection
Data protection is a legal issue, with potentially serious legal consequences should the government get it wrong. Although your organisation will have a data protection officer and there may also be experts in your team, your legal team will be able to help you to to unpick some of the more difficult data protection issues that are thrown up by the use of generative AI.
See data protection and privacy section for more information.
Contractual issues
Your lawyers will help you to draw up the contracts and other agreements for the procurement or licensing of generative AI tools. There may be special considerations for those contracts, such as how to apportion intellectual property and how to ensure the level of transparency that would be required in a legal challenge. Contracts for technology services may need to incorporate procedures for system errors and outages, that recognise the potential consequences of performance failures.
See buying generative AI section for more information.
Intellectual property and copyright
The potential intellectual property issues with generative AI have been much discussed. Your lawyers can help you to navigate these, for example by considering at the outset how ownership of intellectual property rights and liabilities will be apportioned throughout the lifetime of the project. They can also give you advice on any copyright issues with the use of these systems in government.
Equalities issues
Lawyers can help you to navigate the equalities issues raised by the use of generative AI in government, for example obligations arising under the Equality Act 2010. Conducting an assessment of the equalities impacts of your use of generative AI can also be one way to guard against bias, which is particularly important in the context of generative AI.
If approached early, before contracts are signed, your legal advisers can help you ensure the government is fulfilling its responsibilities to the public to assess the impacts of the technology it is using.
Public law principles
Public law principles explain how public bodies should act rationally, fairly, lawfully and compatibly with human rights. These are guidelines for public bodies on how to act within the law. Many of these public law principles overlap with the ethical principles set out in this guidance.
As a result, your lawyers will likely be able to guide you on the application of the ethical principles, based on their knowledge of public law and the court cases that have occurred and the detail of the judgments.
For example, public law involves a principle of procedural fairness. This is not so much about the decision that is eventually reached but about how a decision is arrived at. A correct procedure would ensure that relevant considerations are considered. The transparency and explainability of the AI tool may well be key in being able to demonstrate that the procedure was fair.
Public law also considers rationality. Rationality may be relevant in testing the choice of generative AI system; considering the features used in a system; and considering the outcomes of the system and the metrics used to test those outcomes.
Where you are considering using generative AI in decision-making in particular, public law also can guide you for example on whether particular decisions require the exercise of a discretion by a decision maker, which could be unfairly fettered by the use of a tool, or whether in fact the decision can be delegated at all.
Human rights
Public authorities must act in a way that is compatible with human rights. It’s possible that AI systems (especially those involving the use of personal data) may in some way affect at least one of the European Convention on Human Rights (ECHR) of individuals. Examples of those most likely to be commonly impacted are Article 8 (right to a private and family life) and Article 10 (freedom of expression).
Legislation
Sometimes, in order to do something, a public authority needs a legislative framework. Your lawyers will be able to advise you whether your use of generative AI is within the current legal framework or needs new legislation. For example, it may be that the legislative framework does not allow the process you are automating to be delegated to a machine. Or, it may be that it provides for a decision to be made by a particular person.
Ethics
The ethical questions raised by your use of generative AI will depend on your context and the nature of your solutions. The key themes you should address include:
- Transparency and explainability
- Accountability and responsibility
- Fairness, bias and discrimination
- Information quality and misinformation
- Keeping a human-in-the-Loop
As well as the guidance in this framework, you should also take existing guidance into account, such as the UK government data ethics framework and the UKSA ethics self-assessment tool (PDF, 449KB). The five cross-sectoral, values-based principles for responsible AI innovation set out in the AI regulation white paper also provide a useful explainer for safety, security and robustness; appropriate transparency and explainability; fairness; accountability and governance; and contestability and redress.
Transparency and explainability
Transparency is a cornerstone of the ethical development, deployment and use of AI systems. A lack of transparency can lead to harmful outcomes, public distrust, a lack of accountability and ability to appeal. The AI regulation white paper establishes that AI systems should be appropriately transparent and explainable. Transparency is the communication of appropriate information about an AI system to the right people. For example: information on how, when, and for which purposes an AI system is being used. Explainability is how much it is possible for the relevant people to access, interpret and understand the decision-making processes of an AI system.
However, transparency can be challenging in the context of generative AI, due to the closed and proprietary nature of commercial tools, and the inherent opacity of neural networks. You should therefore ensure that you are transparent about the design of the generative AI system and the processes in which it is embedded:
What you are transparent about:
- Technical transparency: information about the technical operation of the AI system, such as the code used to create the algorithms, and the underlying datasets used to train the model.
- Process transparency: information about the design, development and deployment practices behind your generative AI solutions, and the mechanisms used to demonstrate that the solution is responsible and trustworthy. Putting in place robust reporting mechanisms, process-centred governance frameworks, and AI assurance techniques is essential for facilitating process-based transparency.
- Outcome-based transparency and explainability: the ability to clarify to any citizen using, or impacted by, a service that utilises generative AI how the solution works and which factors influence its decision making and outputs, including individual-level explanations of decisions where this is requested..
How and to whom you are being transparent:
- Internal transparency: retention of up-to-date internal records on technology and processes and process-based transparency information, including records of prompts and outputs.
- Public transparency: where possible from a sensitivity and security perspective, you should be open and transparent about your department’s use of generative AI systems to the general public.
Although there are no universally accepted standards for achieving transparency in the use of generative AI, there are existing standards and external resources which you can draw on:
- The UK Algorithmic Transparency Recording Standard (ATRS) should be used by public sector bodies using algorithmic solutions – like generative AI. The ATRS aims to make sure that information about algorithmic solutions used by government and the public sector are clearly accessible to the public.
- The UK’s national public sector AI ethics and safety guidance, understanding artificial intelligence ethics and safety, outlines a process-based governance framework that can assist project teams in establishing and documenting proportionate governance actions.
- Data and model cards or fact sheets can be used as a reference point when documenting information about AI models and the datasets used in training and testing. A good example of these are Google’s data cards and model cards.
- The Information Commissioner’s Office (ICO) also offers AI auditing consultation and support to government organisations. Further information can be found in a guide to ICO audit: artificial intelligence audits (PDF, 156KB).
- Explaining decisions made with AI guidance is the UK’s national AI explainability guidance co-produced by The Alan Turing Institute and the Information Commissioner’s Office (ICO): this details six types of explanations as well as documentation processes.
Practical recommendations
- Clearly signpost when generative AI has been used to create content or is interacting with members of the public. Where possible, label AI generated content, and consider embedding watermarking into the model.
- Put in place evaluation and auditing structures, tracking data provenance, design decisions, training scenarios and processes.
- Use existing standards and recording mechanisms such as the Algorithmic Transparency Recording Standard (ATRS) to communicate information about generative AI solutions to the general public.
- Use external resources and emerging best practice, such as data cards and model cards for internal transparency.
- Strive to make model outputs as explainable as possible, while being aware of the current explainability limitations of generative AI.
- Consider the use of Open Source models, which provide more transparency about datasets, code and training processes.
- Implement transparency and auditing requirements for suppliers as outlined in Buying generative AI.
Accountability and responsibility
Ensuring accountability for generative AI means that individuals and organisations can be held accountable for the AI systems they develop, deploy, or use, and that human oversight is maintained. To establish accountable practices across the AI lifecycle, you should consider three key elements:
- Answerability: You should establish a chain of human responsibility across the generative AI project lifecycle, including responsibility throughout the supply chain. In cases of harm or errors caused by generative AI, recourse and feedback mechanisms need to be established for affected individuals. Identifying the specific actors involved in generative AI systems is vital to answerability. This includes model developers, application developers, policymakers, regulators, system operators and end-users. The roles and responsibilities of each must be clearly defined and aligned with legal and ethical standards.
- Auditability: You should demonstrate the responsibility and trustworthiness of the development and deployment practices by upholding robust reporting and documentation protocols, and retaining traceability throughout the AI lifecycle. This refers to the process by which all stages of the generative AI innovation lifecycle from data collection and base model training to implementation, fine-tuning, system deployment, updating, and retirement are documented in a way that is accessible to relevant stakeholders and easily understood.
- Liability: You should make sure that all parties involved in the generative AI project lifecycle, from vendors and technical teams to system users, are acting lawfully and understand their respective legal obligations.
As an end-user, being accountable means taking responsibility for a system’s outputs and generated content and its potential consequences. This includes checking that these are factual, truthful, non-discriminatory, non-harmful, and do not violate existing legal provisions, guidelines, policies or the providers’ terms of use. It entails putting the necessary oversight and Human-In-The-Loop processes in place to validate output in situations with high impact or risk. Where these risks are too high, you must consider if generative AI should be used.
Ultimately, responsibility for any output or decision made or supported by an AI system always rests with the public organisation. Where generative AI is bought commercially, ensure that vendors understand their responsibilities and liabilities, put the required risk mitigations in place and share all relevant information. Refer to the buying generative AI section for further guidance.
Practical recommendations
- Follow existing legal provisions, guidelines and policies as well as the provider’s terms of use when developing, deploying or using generative AI.
- As an end-user, assume responsibility for output produced by generative AI tools when used to support everyday tasks, such as drafting emails and reports.
- Clearly define responsibilities, accountability, and liability across all actors involved in the AI lifecycle. Where the generative AI is bought commercially, define detailed responsibilities and liability contractually.
- Nominate a Senior Responsible Owner (SRO) who will be accountable for the use of generative AI in a specific project.
- Where generative AI is used in situations of high impact or risk, establish a human-in-the-loop to oversee and validate outputs.
- Adopt a risk-based approach to the use of AI-generated content and put strategies in place to minimise the risk of inaccurate or harmful outputs. Where the potential risks and harmful impacts are too high, consider whether Human-in-the-Loop approaches offer sufficient mitigation or if generative AI should be used.
- Provide routes for appeal and actionable redress and put feedback channels into place.
- Use assurance techniques to evaluate the performance of generative AI systems. The CDEI AI assurance guide provides a useful starting point, and the CDEI portfolio of AI assurance techniques offers real-world examples.
Fairness, bias and discrimination
Fairness is a concept embedded across many areas of law and regulation, including equality and human rights, data protection, consumer and competition law, public and common law, and rules protecting vulnerable people. The AI regulation white paper sets out that AI systems should not undermine the legal rights of individuals or organisations, discriminate unfairly against individuals or create unfair market outcomes.
Fairness, in the context of generative AI, means ensuring that outputs are unprejudiced, and do not amplify existing social, demographic, or cultural disparities.
By identifying and mitigating bias and reducing harm you will help your generative AI systems produce fairer outcomes. In generative AI, harmful biases can present as text, images, audio and video which perpetuate stereotypical or unfair treatment related to race, sex and gender, ethnicity, or other protected characteristics. Examples of this are the generation of harmful stereotypes or abusive content targeted against particular social groups.
Generative AI systems are designed, developed, and deployed by human beings who are bound by the limitations of their contexts and biases. They are always trained on data which encodes present and past biases and inequalities of society. These can present across the generative AI lifecycle, from data collection to prompt writing. The opacity and complexity of these systems can make it difficult to identify exactly where and how biases are introduced.
Generative AI models may reproduce biases embedded in training data or model design choices. They are particularly vulnerable to bias due to the fact that they are trained on vast amounts of unfiltered data scraped from the internet, which are likely to contain a wide range of content reflecting historical and social biases. The wording of prompts may also inadvertently introduce bias.
Addressing these issues can help to support equitable representation in AI-generated content. This might involve crafting prompts which encourage the consideration of different perspectives. For development teams, this might include ensuring training data is diverse, and implementing fairness testing to assess how the tool responds to different input. Technical methods and metrics for assessing bias in generative AI are still being developed and evaluated. Refer to the testing section for further guidance.
Practical recommendations
- Comply with human rights law, the Equality Act 2010, the Public Sector Equality Duty, the Equality and Human Rights Commission guide to using Artificial intelligence in public services, as well as procedural fairness obligations.
- Write prompts which minimise bias by using professional and neutral language. Refer to the prompt engineering section for guidance on how to develop and optimise prompts.
- Review generated output for potentially harmful content, such as sex and gender based or cultural biases.
- Test a set of prompts to assess for bias. For example, by changing the demographic information in a prompt (such as references to ethnicity or sex and gender) and comparing the outputs.
- Put feedback mechanisms in place to allow individuals to report harmful content produced using generative AI.
- Implement bias mitigation and fairness evaluation across the entire AI project lifecycle.
- Strive for diversity across teams involved in developing, testing, and deploying generative AI. Collect feedback from diverse groups during user testing to understand how a generative AI system performs in real-world scenarios.
- Adopt an approach of continuous evaluation to keep up with changing fairness considerations and societal expectations.
Information quality and misinformation
Having access to high quality information is vital to support effective decision-making. Generative AI poses a challenge to information quality due to its ability to generate content that appears credible but may be false or misleading.
The use of AI-generated content without proper validation and fact-checking can lead to the spread of misinformation. Many generative AI tools are built using large amounts of web-scraped data from unknown, potentially outdated and harmful, sources. For developers, this makes validating the data quality of generative AI models extremely difficult.
The effectiveness of LLMs and other generative models is dependent on the quality of their training data. Even in cases where input data quality is deemed to be high, it is important to keep in mind that these tools cannot understand real-world contexts, nuances in language, cultural references, or intent and do not have access to information that is known to be real or true. LLMs are designed to generate statistically likely language patterns rather than producing reliable and truthful accounts of reality. This can make them convincing generators of ‘nonsense’. The tendency for generative AI models to present nonsensical or incorrect outputs as factual is sometimes referred to as ‘hallucination’.
To mitigate the risk of misinformation, you should check generated content for accuracy and truthfulness, and any potentially harmful or misleading information.
Practical recommendations
- Optimise prompts to improve the quality of generated output. The specificity and structure of prompts can improve the quality of responses. For further guidance on writing prompts refer to the prompt engineering deep dive section.
- Verify and cross-reference information produced by generative AI tools with trusted sources to ensure content is accurate. Be aware that the data used by some publicly available generative AI tools may be outdated.
- Indicate where generative AI has been used to create content, and notify people when they are interacting with a generative AI system.
- Assess the impact of using AI-generated content and the risks of misinformation for each use case.
- Put in place structured governance and oversight processes to regularly review the performance of Generative models.
- Improve the output quality of a model by grounding or fine-tuning it with human feedback.
- Embed watermarking into a model so that outputs from the generative AI tools can be easily detected by users and impacted parties.
Maintaining appropriate human involvement in automated processes
Keeping a human-in-the-loop means ensuring that there is human involvement and supervision in the operations and outcomes of generative AI systems. In a broader context, humans should be involved with setting up the systems, tuning and testing the model so the decision-making improves, and then actioning the decisions it suggests.
The availability of generative AI tools may contribute towards increasingly automated workflows and decision-making processes. However, relying on AI to make decisions and generate content without meaningful human oversight can have negative consequences. A lack of human intervention might result in inaccurate or harmful outputs going unchecked. You should assess the quality of AI-generated outputs to ensure they are accurate, relevant, and align with societal values.
Generative AI also lacks flexibility, human understanding and compassion. While humans are able to take individual circumstances into account on a discretionary basis, AI systems do not have this capacity.
Maintaining meaningful human involvement in generative AI ensures that future innovation aligns with human values and supports the public good. You should uphold the expectation ‘to be heard’ by a human when interacting and receiving services from the government. This supports the principle of transparency and building public trust. You should never use generative AI to fully automate decision making in high-risk or high-impact situations.
Practical recommendations
- Consider whether generative AI is appropriate for the specific use case and whether there is a clear public and user benefit.
- Strive to understand the factors that influence the output and formulate your own views and organisational perspective before consulting the AI system.
- Ensure that there is a human-in-the-loop who can oversee outputs when generative AI is in use in situations with high impacts.
- Validate and cross-reference any information sourced via generative AI solutions.
- Refrain from fully automated decisions and ensure humans are the final decision-makers in high-risk or high-impact situations. Develop appropriate safeguards if a generative AI system is intended to be used in decision making with impact on members of the public.
- Give citizens the option to be referred to a person and enable feedback from users and affected stakeholders
Sustainability and environmental considerations
Generative AI has environmental impacts that you and your organisation should understand and consider before deciding to develop or use generative AI solutions. Large language models, in particular, rely heavily on computational power both during their training phase and then every time they are used, contributing to carbon emissions. They may require the use of a lot of water to cool the data centres, and the manufacturing process of key components like the graphics processing units (GPUs) also contributes to the extraction of rare metals.
You should balance the environmental costs of using pre-trained models and usage costs when deciding on the most appropriate model size for your needs. In general it will not be an environmentally-sound decision to train your own model if appropriate pre-trained models are available. As models are generally expensive to operate, they should not be used for tasks that could be undertaken by other available machine learning tools.
Generative AI can potentially contribute to reducing environmental impact as well. It can optimise processes and minimise resource wastage. For example, AI technologies can streamline data analysis, reducing the computational power required to process information. This optimisation results in lower energy consumption and a decreased carbon footprint.
Practical recommendations
- Include a focus on environmental impacts when considering using generative AI solutions, and compare these to alternative technologies that do not use large language models.
- Check the environmental credentials of potential model providers, including their use of renewable energy, energy-efficient infrastructure and sustainable practices and select low carbon emission energy grids.
- When selecting models, choose the smallest (in terms of number of parameters) that meets your requirements as these are likely to have the lowest environmental impact.
- Conduct life-cycle analysis to assess the carbon footprint of AI systems and make your technology more sustainable.
- Be transparent about the environmental costs of your generative AI project and mitigation measures such as using energy-efficient hardware.
Data protection and privacy
Generative AI systems can process personal data during their training and testing phases; as well as potentially generating outputs which contain personal data, including sensitive personal data. When using generative AI you need to consider how you protect personal data, are compliant with data protection legislation and minimise the risk of privacy intrusion from the outset.
Organisations developing and deploying generative AI systems must consider principles of data protection outlined in the UK General Data Protection Regulation (UK GDPR) and the Data Protection Act 2018.
The data protection law applies irrespective of the type of technology used, so its basic principles of compliance will also apply to any generative AI systems. The Information Commissioner’s Office, which is responsible for regulating compliance with the data protection legislation in the UK, outlines these principles in their guidance.
The data protection principles most relevant to the use of generative AI are:
- Accountability: your organisation has clear ownership of risk and responsibility for mitigations and compliance.
- Lawfulness: you have an applicable lawful basis for processing personal data and ensure the processing is lawful under data protection or any other regulation.
- Purpose limitation: you define why you are processing personal data and only process data for that purpose.
- Transparency and individual rights: you are open about what it uses personal data for, and your users can exercise their information rights.
- Fairness: you avoid processing personal data in ways that are detrimental, unexpected or misleading.
- Data minimisation: you develop systems that process only the data that is needed for the task at hand.
- Storage limitation: you avoid accumulation of vast amounts of personal data for unjustifiably long periods.
- Human oversight: you build in human oversight to automated decision making.
- Accuracy: you have steps in place to ensure the accuracy of generative AI responses and data related to individuals.
- Security: you implement appropriate technical and organisational mitigations to protect sensitive and personal data
Accountability
Accountability is a key principle in data protection law and the AI regulation white paper. Accountability establishes ownership of risk, responsibility for mitigations and compliance with the legislation, ability to demonstrate your compliance and high standards for privacy. The AI regulation white paper notes that clear lines of accountability need to be established across the AI life cycle.
Organisations should take the following steps when planning generative AI solutions:
- Make a strategic decision on how any use of generative AI technology fits with your existing risk appetite
- Review your risk governance model to establish clear ownership of generative AI risks at a senior level.
- Implement measures to mitigate these risks and test their effectiveness.
- Make sure residual risks are aligned with your organisation’s risk appetite.
- Due to the evolving nature of generative AI technologies and new regulations, ensure you conduct regular reviews and further iterations.
- Importantly, engage with internal data protection, privacy and legal experts from the outset.
Practical recommendations
- Establish ownership of generative AI risks at a senior level.
- Integrate oversight of generative AI into your governance processes.
- Take a risk-based approach, defining risk appetite and following principles of data protection by design and by default
Lawfulness and purpose limitation
The nature of generative AI means that its misuse may result in high risks to data subjects. As a result, a Data Protection Impact Assessment (DPIA) should be undertaken prior to deploying any generative AI capabilities which process personal data.
The DPIA process should identify personal data processing at each stage of the generative AI lifecycle starting from design to data acquisition and preparation, training, testing, deployment and monitoring.
If you are processing personal data in your generative AI system that is not fully anonymised, you must identify an appropriate lawful basis under UK GDPR.
The UK GDPR requires data controllers:
- To identify each distinct processing operation, determine whether personal data is included and identify the specific purpose.
- To map data sources and identify where personal data needs to flow as part of the processing operations.
Identification of all personal data sources is important as data controllers will be accountable for all personal data processed throughout the generative AI lifecycle. For example, generative AI products are often trained on publicly available information drawn from the internet. Publicly available content which contains personal data may have been published in the public domain lawfully, but it is not currently agreed that the re-use of public personal data to train an LLM is lawful. Before re-using personal data in an LLM or generative AI system, you should seek data protection and legal expertise to consider and advise whether the re-use of that data is compatible with the purposes for which it was collected.
Special category data is personal data that needs more protection because it is sensitive, such as health data. If your generative AI system needs to process special category data, you must be able to demonstrate that you meet one of the specific conditions in Article 9 of the UK GDPR.
When mapping personal data flows, it is important to identify the geographic location of each distinct processing activity since the processing of data outside the United Kingdom will increase the risk of losing the protection of the UK data protection laws. Data controllers may need to bring in additional safeguards, such as International Transfer Data Agreements if personal data is being processed in jurisdictions where the data protection regime is not deemed to be adequate and transfers of personal data is restricted under Article 46 of the UK GDPR.
If having undertaken a Data Protection Impact Assessment, data protection risks remain “high” even after mitigations, and you cannot do anything to reduce it, prior consultation with the ICO is required under UK GDPR before processing of personal data can begin.
Practical recommendations
- When building your team, seek support from data compliance professionals, including data protection, legal and privacy experts.
- Identify data processing operations and their purpose, and map personal data sources and flows.
- Determine whether personal data is necessary for each activity, and whether you are processing special category data or children’s data.
- Identify the applicable lawful basis of your data processing and assess data protection and privacy risk through data protection impact assessments and legitimate interest assessments.
- If data protection and privacy risks remain “high” even after mitigations, consult with the ICO.
- Identify any processing outside the UK to take additional safeguards to protect personal data in jurisdictions where data protection regime may not be adequate.
- Assess any changes in the purpose of your generative AI system and make sure your generative AI system remains compliant and lawful.
Transparency and individual rights
In addition to the ethical reasons for seeking transparency, organisations need to be transparent about how they process personal data in a generative AI system so that individuals can effectively exercise the rights granted to them by the UK GDPR.
This obligation applies to the direct collection of data from individuals and to personal data collected from other sources. The rights relating to personal data granted to individuals under data protection law apply wherever personal data is used at any of the various points in training, testing and deployment of an AI system.
The UK GDPR requires data controllers:
- To provide information to users in concise, transparent, intelligible and easily accessible form using clear and plain language.
- To be transparent about the purpose for processing personal data, retention periods, third parties involved in the processing activity.
- To be transparent about the existence of automated decision-making, meaningful information about the logic involved, as well as the significance and the envisaged consequences of such processing for the data subject.
- To provide a clear explanation of the results these systems produce.
- To uphold individuals’ rights, including the right of access to the personal data that you hold on them, and a simple and clear process to exercise their right to correction and to object to the processing of their personal data at any time.
The data transformation processes involved in training a model may convert personal data into a less detailed form, making training data harder to link to a particular named individual. However, even without direct identifiers, individual level data that is rich in other variables may lead to inadvertent identification of people and is subject to data protection safeguards. This data needs to be considered when responding to individuals’ requests to exercise their rights as the initial processing stages may have included their personal data.
Practical recommendations
- Explain your system in plain language.
- Be transparent about the purpose for processing personal data, retention periods and third parties involved in the processing activity.
- Be transparent about the existence and nature of automated decision-making, using the Algorithmic Transparency Recording Standard (ATRS).
- Provide a clear explanation of the results these systems produce, following guidance such the ICO’s Explaining Decisions on AI.
Fairness
In addition to ethical reasons for fairness, it is also a data protection obligation under the UK GDPR for generative AI systems that process personal data. In the context of the data protection legislation, fairness means that “you should only process personal data in ways that people would reasonably expect and not use it in any way that could have unjustified adverse effects on them.”
You must make sure that generative AI systems do not process personal data in ways that are unduly detrimental, unexpected or misleading to the individuals concerned. You need to uphold the ‘right to be informed’ for individuals whose personal data is used at any stage of the development and deployment of generative AI systems as part of fulfilling the transparency and fairness principles.
If generative AI systems infer data about people, you need to ensure that the system is accurate and avoids discrimination. Data protection aims to protect individuals’ rights and freedoms with regard to the processing of their personal data, not just their information rights. This includes the right to privacy but also the right to non-discrimination.
Data Protection Impact Assessments (DPIAs) are the main tool to steer you to consider the risks to the rights and freedoms of individuals, including the potential for any significant social or economic disadvantage. DPIAs also help you to demonstrate whether your processing is necessary to achieve your purpose, is proportionate and fair.
You must remember that there may be other sector-specific obligations around lawfulness, fairness, statistical accuracy or discrimination to consider alongside data protection obligations (e.g. Equality Act 2010). These are discussed in more detail under the Legal considerations section.
Practical recommendations
- Identify the risks to the rights and freedoms of individuals through data protection impact assessments and assess whether your processing is necessary, proportionate and fair to achieve your purpose
- Use the ICO’s AI Toolkit to reduce the risks to individuals’ rights and freedoms.
- Mitigate risks using the ICO’s guidance on fairness in AI systems
- Provide users with clear reassurance that you are upholding their right to privacy, including simple and clear processes to exercise these rights in clear privacy notices
- Address any objections from users related to solely automated decisions producing legal or similarly significant impact on them by implementing safeguards, such as meaningful human intervention, effective process to obtain and consider individuals’ views and corrections of factual errors.
Data minimisation
The data minimisation principle requires you to identify the minimum amount of personal data you need to fulfil your purpose, and to only process that information, and no more. This does not mean that generative AI shouldn’t process personal data. If you can achieve the same outcome by processing less personal data then by definition, the data minimisation principle requires you to do so.
There are a number of techniques that you can adopt to develop generative AI systems that process only the data you need, while still remaining functional. The Centre for Data Innovation and Ethics (CDEI)’s responsible data access programme includes important work to encourage adoption of Privacy-Enhancing Technologies (PETs). PETs are a set of emerging techniques that provide stronger protections to preserve data privacy whilst enabling effective use of data. PETs come with their own limitations however, therefore selection of the PET technology should be proportionate to the sensitivity of the data.
CDEI has published a PET adoption guide to raise awareness of these emerging technologies. Similarly, the ICO has published the new PET guidance which explains how they can be used to support a data protection by design approach in line with regulatory requirements.
Practical recommendations
- Justify use of personal data, thinking about the problem you are solving through your data protection impact assessment and settle with the minimum personal data that is required. Less personal data means less risk.
- Reduce the risk of individuals’ identifiability through the processing of their personal data employing a range of privacy enhancing techniques.
Storage limitation
Generative AI systems can only process personal data as long as you can reasonably justify it for the purpose you are processing. As challenging as it may be, you need to strike a delicate balance between any relevant training of large language models and minimising the collection and storage of personal data to meet the UK GDPR requirement of storage limitation.
It may be necessary to retain training data in order to retrain the model, for example when new modelling approaches become available and for debugging. However, where a model is established and unlikely to be retrained or modified, the training data may no longer be needed. You should:
- Assess data requirements for accurate training.
- Specify a clear period for retaining and processing personal data in your information materials and be transparent.
- Delete the personal data at the end of that period.
There are a number of strategies you can follow to address concerns around long (or even perpetual) retention of personal data. Storage limitation is best complied with through purpose limitation and data minimisation. You should map all personal data flows through stages of development, testing and deployment, and utilise data minimisation or eventually anonymisation techniques to remove or irreversibly transform personal data from training datasets.
Practical recommendations
- Use data minimisation and anonymisation techniques (PDF, 325KB) as needed to remove or irreversibly transform personal data where possible.
- Be transparent about length of personal data retention in privacy notices.
Human oversight
Although it is possible to use generative AI systems for automated decision making where the system makes a decision automatically without any human involvement, this may infringe the UK GDPR. Under Article 22, the UK GDPR currently prohibits “decision(s) based solely on automated processing” that have legal or “similarly significant” consequences for individuals. Services that affect a person’s legal status or their legal rights utilising generative AI must only use it for decision-support, where the system only supports a human decision-maker in their deliberation.
Generative AI systems need to bring processes into training, testing and output stages so that humans work together with machines to perform tasks, combining their abilities to reach best results. However, the human input needs to be “meaningful”. The degree and quality of human review and intervention before a final decision is made about an individual are key factors in determining whether a generative AI system is being used for automated decision-making or merely as decision-support.
There are a number of factors that should determine the amount of human involvement in generative AI, such as the complexity of the output, its potential impact, the amount of specialist human knowledge required. As an example, generative AI systems deployed in legal, health and care are likely to always require human involvement no matter how exceptional the technology.
While focusing on generative AI risks, it is important to consider biases at organisational and human review levels. Humans and generative AI technology have different strengths and weaknesses when it comes to ensuring fair outcomes. Generative AI cannot use emotional intelligence, nuance, or an understanding of the broader context. At the same time, humans have their own unconscious biases and beliefs that influence their reasoning. This points back to the importance of the accountability principle, robust governance structures for oversight and alignment of generative AI and existing business processes, such as risk management.
Further aspects on human oversight for generative AI systems can be found in the ethics section.
Practical recommendations
- Design, document and assess the stages when meaningful human review processes are incorporated and what additional information will be taken into consideration when making the final decision.
- Use the ICO guidance on automated decision making under UK GDPR for more clarity on types of decisions that have a legal or similarly significant effect.
Accuracy
Accuracy in the context of data protection requires that personal data is not factually incorrect or misleading, and where necessary, is corrected, deleted and kept up to date without delay.
You need to put in place appropriate mathematical and statistical procedures as part of your technical measures to correct inaccuracies in personal data and minimise errors. Generative AI outputs should be tested against existing knowledge and expertise in early implementations of those outputs.
The outputs of a generative AI system are not always intended to be treated as factual information about the individual but instead represent a ‘statistically informed guess’. You need to factor in the possibility of them being incorrect and the impact this may have on any decisions. To avoid such misinterpretations of outputs as factual, systems should be explicit that they are statistically informed guesses rather than facts, including information about the source of the data and how the inference has been generated.
For more information see the getting reliable results section.
Practical recommendations
- Test generative AI outputs against existing knowledge and expertise during training and testing.
- Be transparent that outputs are statistically informed guesses rather than facts.
- Document the source of the data and the AI system used to generate the conclusion.
- Implement processes to consider individuals’ feedback, views and corrections of factual errors.
Security
The UK government has a responsibility to ensure that the services it provides do not expose the public to undue risk, which makes security a primary concern for anyone looking to deploy emerging technology, such as generative AI.
This section takes you through how to keep generative AI solutions in government secure:
- How to deploy generative AI securely
- Security risks
- Practical Security Recommendations
We have set up a cross-government generative AI security group made up of security practitioners, data scientists and AI experts to support this section, and help people across government to share knowledge and best practices. You can request to join the group by emailing: x-gov-genai-security-group@digital.cabinet-office.gov.uk.
How to deploy generative AI securely
Generative AI can be deployed in many different ways. The approaches set out below present different security challenges and can affect the level of risk that must be managed.
This section covers different approaches that you need to take for:
- Public generative AI applications and web services
- Embedded generative AI applications
- Public generative AI APIs
- Privately hosted open source generative AI models
- Data provenance
- Working with your organisational data
- Open-source vs closed-source models
For additional information see the section on deployment patterns.
Public generative AI applications and web services
The use of public chatbots such as Google Bard or ChatGPT are easier to use compared to open-source, bespoke solutions.
However, a key disadvantage of allowing the use of public applications is that you cannot easily control the data input to the models, and must rely on training users on what they can and cannot enter into the chat prompt. You also have no control on the outputs from the model and are subject to their commercial licence agreements and privacy statements, for example OpenAI will use the prompt data you enter directly into the ChatGPT website to improve their models, although individual users can opt out.
Embedded generative AI applications
As well as these more direct approaches to using generative AI, many vendors include generative AI features and capabilities directly within their products, for example Slack GPT and Microsoft 365 Copilot. Whilst this guidance applies at a high level to each of these applications, they come with their own unique security concerns, you should speak to your security teams to discuss your requirements.
In addition to embedded applications there are also many generative AI tools that offer plugins or extensions to other software, for example, Visual Studio Code has a large ecosystem of community built extensions, many of which offer generative AI functionality. Extreme caution should be taken before installing any unverified extensions as these are likely to present a security risk. You should speak to your security team to discuss your requirements.
Before adopting any of these products it is important to understand the underlying architecture of the solution and what mitigations the vendor has put in place for the inherent risks associated with generative AI.
All of these different approaches come with trade-offs between security, privacy, usability and cost. Each of the security risks of generative AI models need to be taken in context with the way the model is deployed and used to inform the level of risk that an application poses.
Public generative AI APIs
Many public generative AI applications usually offer the ability to access their services through Application Programming Interfaces (APIs), which define the set of rules, protocols, and tools for building software applications. Through using the API it can be very easy to integrate generative AI capabilities into your own applications. The benefit here is that you can intercept the data being sent to the model and also process the responses before returning them to the user.
You can also include Privacy Enhancing Technology (PET) to prevent data leakage, add content filters to sanitise the prompts and responses, and it also means you can log and audit all interactions with the model. Note that PETs come with their own limitations, therefore selection of the PET should be proportionate to the sensitivity of the data: see ICO’s privacy-enhancing technologies (PETs) and CDEI’s PET adoption guide for more information.
Use of the API still means that data is passed over to the provider, although the retention policies tend to be more flexible for API use, for example, OpenAI only retains prompt data sent to the API for 30 days.
Privately hosted open source generative AI models
Instead of using a public generative AI offering, the alternative is to host your own generative AI model. By taking one of the many publicly available open source models and running it in your own private cloud infrastructure, you ensure that data never leaves an environment that you own.
The type of models that you can run in this way are not on the scale of the publicly available ones, but can still provide acceptable results. The advantage is that you have complete control over the model and the data it consumes. The disadvantage is that you are responsible for ensuring the model is secure and up to date.
An alternative approach is to use one of the larger commercial models, but in a private managed instance, for example, the Microsoft Azure OpenAI service offers access to the OpenAI ChatGPT models but running in a private instance with zero-day retention policies.
Data provenance
In addition to where your generative AI model runs, how the model was trained is also important from a security perspective. All the publicly available models were trained using data from the public internet. This means that they include data that is personally identifiable, inaccurate, illegal and harmful, all of which could present a security risk.
It is possible to train a LLM using your own data, but the cost of doing this for larger and more capable models is prohibitive. Along with the cost, the amount of private data required to produce acceptable performance of a large model is also beyond the capacity of most organisations.
Working with your organisational data
A key application of generative AI is working with your organisation’s private data, by enabling the model to access, understand and use the private data, insights and knowledge can be provided to users that is specific to their subject domain and will provide more reliable results.
Open-source vs closed-source models
Neither open-source or closed-source LLMs are inherently less secure than the other. A fully open-source model may expose not only the model code, but also the weights of its parameters and the data used to train the model. While this increases transparency, it also potentially presents a greater risk, as knowing the weights and the training data could allow an attacker to create attacks carefully tailored to the specific LLM.
One benefit of fully open-source models is that they allow you to inspect the source code and model architecture, enabling security experts to audit the code for vulnerabilities. Despite this, owing to their complexity, even an open source LLM is mostly opaque, meaning that the internals of the model are hard to analyse. Open-source models theoretically benefit from a community of developers, who can quickly identify and fix security issues, whereas closed-source model owners might be incentivised not to publicise security flaws in their models. However, it should be noted that several high-profile vulnerabilities in open source libraries have been present for many years before being identified.
Security risks
Significant work has already been done by the Open Worldwide Application Security Project (OWASP) to identify the unique risks posed by LLMs. From these we can draw out some of the most common vulnerabilities and put them in context of how they could apply to LLM applications in government. These risks focus on the use of LLMs but many of them will also apply to other types of generative AI models.
We take each security risk and use a scenario describing an application of generative AI in a government context, to illustrate how that vulnerability might be exploited. The list of scenarios is not exhaustive, but should be used as a template for assessing the risks associated with a particular application of generative AI.
Impacts are described for each scenario, and mitigations suggested. The likelihood and impact of each risk in a given scenario are scored, following the approach outlined in the OWASP risk rating methodology. In addition to the impact factors included in the OWASP approach, we add user harm and misinformation as a significant impact factor.
Security threats include:
- Prompt injection threats: Using prompts that can make the generative AI model behave in unexpected ways:
- LLM chatbot on a government website
- LLM enhanced search on a government website
- Private LLM chatbot returning suggested file sources
- Data leakage: Responses from the LLM reveal sensitive information, for example, personal data:
- Intranet search engine enhanced with LLM
- Private LLM chatbot summarises chat conversations
- Hallucinations: The LLM responds with information that appears to be truthful but is actually false:
- Developer uses LLM generated code without review.
Prompt injection threats
Prompt injections can either be direct, meaning a user directly enters a prompt into the LLM to subvert its behaviour. Or they can be indirect, meaning the LLM gets input from an external source, and that source has been manipulated to include a prompt injection, for example from an email or an external file.
LLM chatbot on a government website - full chat interface
Scenario
A chatbot deployed to a government website to assist with queries relating to a particular public service. The chatbot uses a private instance of one of the publicly trained LLMs. The user’s question is combined with system instructions that tell the LLM to only respond to questions relevant to the specific service. The system instructions are combined with the user’s original question and sent to the LLM. A malicious user could craft a specific prompt that circumvents the system instructions and makes the chatbot respond with irrelevant and potentially harmful information.
This is an example of a direct prompt injection attack.
Impact
Actual risk of user harm if a user is tricked into using an unsafe prompt that then results in harmful content being returned and acted on, for example: a user is looking for how to pay a bill and is directed to a false payment site.
Reputational damage to the government, if a user made public potentially harmful responses received from the chatbot, for example: a user asking for generic information receives an inflammatory response.
Mitigation
Use prompt engineering to attach a meta prompt to any user input to prevent the LLM from responding to malicious input.
Apply content filters trained to detect likely prompt injections to all prompts sent to the LLM.
Choose a more robust model, some models have been shown to be more resistant to this kind of attack than others.
None of these mitigations are sufficient to guarantee that a prompt injection attack would not succeed. Fundamentally an LLM cannot distinguish between user input and system instructions. Both are processed by the LLM as natural language inputs so there is no way to prevent a user prompt affecting the behaviour of the LLM.
Risk rating
Likelihood: HIGH
Impact:
- LOW - response is returned to a single user with limited repercussions
- HIGH - response causes actual harm to a user
Recommendation
Deploying an LLM chatbot to a public-facing government website does come with a significant risk of a direct prompt injection attack, the impact of such an attack should be considered in the context of the specific use case. For example a chatbot deployed to a small number of users for the purposes of gathering data about the effectiveness of LLMs under controlled conditions, is far lower risk, than one that is more generally available and designed to make specific, actionable recommendations to a user.
LLM enhanced search on a government website - no chat interface
Scenario
A private LLM is used to enhance the search capabilities of a public facing government website, without providing a chatbot interface. The content of the government website is initially split into small chunks of text and vector indexed using a ML algorithm. A user enters a natural language search term. The ML algorithm processes the search term into a vector, a similarity search is done against the vector indexed database of text chunks. The most relevant chunks are retrieved and passed in context to the LLM, along with the user’s search term, and system instructions telling the LLM to return a summary of the search results. A malicious user could craft a specific search term that circumvents the system instructions making the summary contain potentially harmful information.
Impact
Actual risk of user harm if a user is tricked into using an unsafe search term that results in harmful content being returned and acted on.
Reputational damage to the government, if a user made public potentially harmful responses received from the enhanced search.
Mitigation
Apply content filters trained to detect likely prompt injections to all prompts sent to the LLM.
Filter the summarised search results returned by the LLM to ensure they contain only information relating to the government website.
Do not pass the original search term to the LLM.
Risk rating
Likelihood: MED
Impact:
- LOW - response is returned to a single user with limited repercussions
- HIGH - response causes actual harm to a user
Recommendation
This scenario presents lower risk than directly hosting an LLM chatbot on a government website (Scenario 1), as a level of indirection exists between the search term entered by the user and the prompt sent to the LLM. However, if the search term is passed to the LLM along with the search results in context, then a direct prompt injection would still work. To stop this no part of the search term should be passed to the LLM. The trade off here is that this is likely to reduce the usefulness of the enhanced search.
Private LLM chatbot returning suggested file sources
Scenario
A chatbot is deployed into an internal departmental messaging system (for example Google Chat). The chatbot calls out to a privately hosted open source LLM running within the department’s cloud. The chatbot scans attachments posted in the chat, and passes the content of these to the LLM, with system instructions telling the LLM to augment its responses with links to relevant information held in departmental files. A user posts an attachment that unknown to them has been manipulated to contain a prompt injection. The chatbot passes the attachment content as input to the private LLM, the resulting response contains a list of links to relevant files on the department’s shared drives. The prompt injection alters the list of links so that they direct the user to an insecure third party website rather than the original file.
This is an example of an indirect prompt injection attack.
Impact
A user follows a harmful link, which may lead to data loss and privacy violations.
Additional vectors of attack are opened up which may result in further data loss or financial damage if malicious software was downloaded from the insecure site.
Mitigation
Apply content filters to attachments before they are passed to the LLM.
Apply filters to the response generated by the LLM, to ensure any links contained in it are only to known resources.
Ensure network controls are enforced that prevent users following dangerous links.
Risk rating
Likelihood: MED
Impact: MED
Recommendation
This scenario does not present a significant risk, however the impact is highly dependent on the actions the response from the LLM is used to make, in this case only generating a list of links. If the response was used to send emails, or modify files or records the impact would be much greater. LLM responses must not automatically lead to destructive or irreversible actions, a human must be present to review the action.
Intranet search engine enhanced with LLM
Scenario
A private LLM is used to enhance the search capabilities of an internal departmental search engine. The departmental data (documents, emails, web pages and directory information) is initially split into small chunks of text and vector indexed using a ML algorithm. A user enters a natural language question, for example: “how do I apply for compassionate leave?”. The ML algorithm processes the user’s question into a vector, a similarity search is done against the vector indexed database of text chunks. The most relevant chunks are retrieved and passed in context to the LLM, along with the user’s question, and system instructions telling the LLM to tailor its responses to the user’s question using information in the retrieved text. The LLM responds with confidential information that has been inadvertently retrieved by the vector index search. For example it may return details about who is currently on compassionate leave and the reasons why.
Impact
Significant privacy violations and leakage of confidential data, irrespective of data security controls.
Reputational damage to the department due to loss of data.
Regulatory breaches with financial consequences.
Mitigation
Ensure that any vector index database respects source data security controls. The identity of the search user must be passed to the similarity search so that appropriate controls can be applied. This prevents the LLM receiving content that the user is not permitted to see.
Risk rating
Likelihood: MED
Impact: HIGH
Recommendation
In this scenario security controls can be preserved, however if the LLM was to be fine tuned with private data or trained directly with private data, then there would be no way of applying the original data security controls owing to the way the LLM encodes the data it is trained with. Private data which contains confidential information or employs different levels of security controls must not be used to fine-tune or train an LLM.
Private LLM chatbot summarises chat conversations
Scenario
A chatbot is deployed into an internal departmental messaging system (for example Google Chat). The chatbot calls out to a privately hosted open source LLM running within the department’s cloud. The chatbot scans the conversation thread and summarises the content. A prompt injection in the conversation thread causes the chatbot to emit the summary of the thread in the form of an obfuscated link to a malicious site, for example https://hackernoon.com/?summary=base-64-encoded-summary (this link is safe). The chat interface unfurls the link posted in the response, automatically calling out to the malicious site and transferring the encoded summary of the chat.
Impact
Data loss, potentially confidential information contained in the chat thread is transferred to a third party.
Reputational damage to the department due to loss of data.
Regulatory breaches with financial consequences.
Mitigation
Apply filters to the response generated by the LLM, to ensure any links contained in it are only to known resources.
Ensure network controls are enforced that prevent applications making calls to dangerous URLs.
Risk rating
Likelihood: LOW
Impact: HIGH
Recommendation
In this scenario prompt injection can be used to perform data exfiltration without any action required by the user. The risk can be mitigated by removing malicious links in the response from the LLM. More generally LLM responses that will be read by humans should avoid using links to external resources, and if external links are provided then the response must be filtered to remove malicious URLs.
Hallucinations
Developer uses LLM generated code
Scenario
A developer uses public LLM to answer coding questions, and receives advice to install a specific software package, for example “arangodb” from the JavaScript package management system npm. When the LLM was trained the package did not exist. A hacker has previously interrogated the LLM with common coding questions and identified this hallucination, they have then created a malicious package with the fictitious name and registered it with the package management system. When the developer now comes to install the package, they receive the malicious code.
Impact
Unauthorised code execution when the software containing the fake package is deployed and run, this could result in significant data loss and other serious consequences.
Mitigation
Do not rely on the responses of the LLM, double check all outputs before including them in your code. Check all package dependencies of your code before deployment. Use an automated tool to scan for supply chain vulnerabilities, for example, ‘dependabot’ or ‘snyk’.
Risk rating
Likelihood: LOW
Impact: HIGH
Recommendation
If developers are following secure coding best practices the risk should never arise as all dependencies should be checked before deployment. Over reliance on LLM generated code without sufficient human oversight is likely to become an increasing risk. Treat all LLM generated code as inherently insecure and never use it directly in production code without first doing a code review.
Practical security recommendations
- Design risk-driven security taking account of the
OWASP Top 10 security risks for LLMs. - Use a consistent risk rating methodology to assess the impact and likelihood of each risk.
- Minimise the attack surface by only using the required capabilities of the generative AI tool, for example, by avoiding sending user input directly to an LLM.
- Defend in depth by adding layers of security, for example, by using privacy enhancing technology (PET) to prevent data leakage and adding content filters to sanitise the prompts and responses from an LLM.
- Never use private data that needs different levels of access permissions based on the user who is viewing it, to fine-tune or train an LLM.
- Prevent LLM responses automatically leading to destructive or irreversible actions, such as sending emails or modifying records. In these situations a human must be present to review the action.
- Avoid using links to external resources in LLM responses that will be read by humans, and if external links are provided then the response must be filtered to remove malicious URLs.
- Treat all LLM generated code as inherently insecure and never use it directly in production without code review.
- Never enter any OFFICIAL information directly into public generative AI applications or APIs, unless it is already publicly available or cleared for publication. Exceptions may apply for specific applications with different data handling terms provided under commercial licences, for example, Microsoft Copilot, Azure Open AI, or Bing Enterprise Chat.
- Avoid putting LLM chatbots on public facing government websites, unless the risk of direct prompt injection is acceptable under the specific use case.
Governance
Because of the risks around security, bias and data, all AI programmes need strong governance processes. In the AI regulation white paper, the accountability and governance principle says that governance should ensure effective oversight of the supply and use of AI systems, with clear lines of accountability established across the AI life cycle. Whether they are already built into existing governance frameworks or a new governance framework, the processes should be focused on:
- Continuous improvement by including new knowledge, methods, and technologies.
- Identifying key stakeholders representing different organisations and interests such as Civil Society Organisations (CSOs) and sector experts to create a balanced view from stakeholders so that they can support AI initiatives.
- Planning for the long-term sustainability of AI initiatives, considering scalability, long-term support, maintenance, and future developments.
As part of any governance framework, organisations should consider setting up a separate AI Governance board or have AI representation on a Governance Board and an Ethics Committee. An AI governance board and an ethics committee are components of responsible AI implementation within an organisation or department which play different and distinct roles and responsibilities.
AI governance board or AI representation on an existing board
- In general, an AI governance board covers aspects such as alignment to ethical principles, risk management, compliance, assurance, resource allocation, stakeholder engagement, and alignment with business objectives.
- An AI governance board or representation on a board provides oversight, accountability, and strategic guidance to make informed decisions about AI adoption and use.
- The board holds the organisation accountable for achieving responsible and effective AI outcomes and helps ensure AI projects are aligned with ethical values; its scope is broader, including operational and strategic considerations.
- Alongside support and input from your organisation’s internal assurance team, a board or an AI representative on a board will help you make sure your project is on track and manage risks.
Ethics committee
The primary focus of an ethics committee is to assess the ethical implications of various actions, projects, and decisions within the organisation. It evaluates projects, policies, and actions from an ethical standpoint, focusing on values such as fairness, transparency, and privacy
It typically includes legal experts, representatives from relevant organisations, community members, and other stakeholders who provide a specialised perspective on ethical matters and may also include Civil Society Organisations (CSOs).
See the ethics section for related content.
Creating an AI/ML systems inventory
To support the work, organisations should consider setting up Artificial Intelligence and Machine Learning systems inventory to provide a comprehensive view of all deployed AI systems within an organisation.
It helps management and stakeholders understand the scope and scale of AI usage across programmes and projects, providing better oversight and awareness of any AI used in making decisions, and potential risks such as data quality, model accuracy, bias, security vulnerabilities, and regulatory compliance. The inventory should be regularly kept up to date with the following details:
- Describe each system’s purpose, usage, and associated risks.
- Include details like data elements, ownership, development, and key dates.
- Employ protocols, structures, and tools for maintaining an accurate and comprehensive inventory.
Programme governance in teams should consider
- Set out how the model will be maintained over time, and develop a comprehensive plan for knowledge transfer and training to ensure the model’s sustainable management.
- Establish clear roles and responsibilities to ensure accountability within teams for AI systems, including who has the authority to change and modify the code of the AI model.
- Establish pathways for escalation and identify key points of contact for specific AI related issues.
- Set out how they work with and report into their programme boards and the ethics committee.
- Ensure diversity within the project team by incorporating a range of subject matter expertise, skills, and lived experiences.
Practical recommendations
- Connect with your organisation’s assurance team and review the Centre for Data Ethics and Innovation’s assurance guide
- Set up an AI governance Board or include AI experts on existing governance boards.
- Consider setting up an ethics committee, made up of internal stakeholders, cross-government stakeholders, sector experts and external stakeholders like CSOs.
- Set up an AI/ML systems inventory to provide a comprehensive view of all deployed AI systems within your department.
- Make sure your programme teams have clear governance structures in place.