Learning ladders: the role of adult training in supporting progression from low pay
Updated 13 November 2020
Learning ladders
The role of adult training in supporting progression from low pay
Research report
November 2020
1. Executive summary
Low pay and lack of pay progression remain entrenched in the UK labour market. Although increases in minimum wages have helped to lift many people out of relative poverty, in 2019, 15% of workers were still in low paid jobs. Research shows that relatively few low paid workers achieve long-term progression into higher pay. More commonly, they remain permanently stuck in low pay or cycle in and out of higher pay. A lack of pay progression can have long-term impacts on social mobility, with the children of low paid workers themselves more likely to work in low paid jobs.
Low pay is unevenly spread across UK workers, with many demographic groups, regions and sectors disproportionately hit. It is likely that the Coronavirus pandemic will only exacerbate these issues. The drastic fall in job vacancies already experienced and the expected rise in unemployment as the Coronavirus Job Retention Scheme ends will create increased competition for jobs, reducing the opportunity for pay rises. The full long-term economic impacts of the pandemic are still to be seen, but there will likely be a strong hit on both pay and pay progression.
Workplace training and adult education have long been seen as a route to higher wages and better opportunities, both in and outside of work. They can allow adults to upskill and retrain for better-paid occupations, enabling upwards social mobility and providing knock-on effects down the generations.
However, not all training is equal. Research on earnings returns has tended to find differences in the impact of different types and levels of qualifications. There are also sectoral differences, with engineering, construction and business tending to give rise to the highest returns while subject areas such as caring, childcare and catering the lowest. However, evidence on the impact of training on low pay and progression is still incomplete, and it is not fully clear what types of training lead to earning returns and for how long they remain.
This report presents the findings of an analysis of 2 large-scale longitudinal datasets: the British Household Panel Survey/Understanding Society (BHPS/USOC), and Longitudinal Education Outcomes (LEO). It investigates the personal characteristics associated with pay progression and access to adult education, and examines the relationship between training and progression from low pay over an 8-year period.
1.1 Key findings
A range of factors influence the chances low paid workers will escape low pay
Workers are more likely to escape low pay if they are younger, live in London, have a more privileged background, work in a professional occupation and are a white international migrant.[footnote 1]
After accounting for interactions between different characteristics, the key factors that had a significant influence on pay progression were:
- total days of training
- no children in the household
- being younger
- holding Level 2 qualifications
- living in London
Geographical mobility is low among low paid workers, with almost all remaining within the same broad region.
Workers with the very lowest earnings were more likely to be stuck in low pay: those who were stuck in low pay had substantially lower earnings during periods of low pay than those who escaped or cycled in and out of higher pay.
Training plays a role in supporting low paid workers to progress
Having a higher total number of training days significantly increases the chances of escaping from low pay. Our analysis suggests that the chances of escaping low pay are 8 percentage points higher for those with 150 training days over 8 years compared to those with no training days over that period.
People with higher level qualifications are generally more likely to progress from low pay. However, only 1% to 4% of people in the surveys we analysed improved their qualifications over the time period. This suggests a case for increasing the number of adults improving their skills, so more people can benefit from pay progression.
But the likelihood that low paid workers participate in training varies
The 3 significant predictors of participation in training after accounting for interactions between different characteristics were: higher prior qualification levels, being female and living outside of London.
Individuals with parents from more privileged backgrounds are substantially more likely to participate in training – reinforcing inequalities and limiting social mobility.
And the impacts differ according to the type of training
Individuals undertaking higher levels of learning, particular subjects (such as engineering and manufacturing), and longer courses are more likely to escape from low pay.
Level 3 courses (A-level or equivalent) were found to significantly increase the earnings of low paid workers by an average of 5%. No other statistically significant positive returns were found for any other qualification level.
1.2 Policy implications
Ensure the new ‘Lifetime Skills Guarantee’ benefits low paid workers
To ensure the benefits of the new Lifetime Skills Guarantee in England are shared by low paid workers, policymakers should:
- consider how to encourage individuals to participate in courses that have demonstrable benefits for pay progression
- provide support for other costs associated with learning
- ensure there are flexible learning opportunities available
- find ways to inspire and engage low paid workers in learning and give support to progress to Level 3 from entry level onward, using our complimentary research on behavioural insights in work to engage employers in this effort[footnote 2]
Address inequalities in apprenticeships to help combat low pay
The results suggest that the key training characteristics identified in the research (Level 3 qualification, guided learning hours and subject) are important to consider when designing or choosing apprenticeship programmes. We need to make sure apprenticeships match these characteristics, that there are learning ladders to reach them where workers are not ready to progress straight to them (including basic skills learning and Level 2 where needed), and that everyone has fair access to the best apprenticeships, regardless of background. Our earlier research on apprenticeships identified that the current system is not achieving its potential to improve social mobility, but with strategic intervention, it could do so.
Develop an overall lifelong learning strategy for England with a focus on progression
The government needs to align existing funding streams under an overall strategy for lifelong learning and create a unified system. Aligning investment in skills with investment in jobs is likely to maximise the benefits in the short and longer term. A lifelong learning strategy should:
- increase investment by both government and employers at all levels – including basic skills and Level 2 to create progression routes
- ensure lifelong learning is a central part of industrial strategies to improve growth, and involve employers and trade unions in the design of training and qualifications
- focus on the outcomes of learning and its role in wider policy
- empower local government to join up support
Tailor employment and progression support to individuals
Training was one factor that can affect the chances that workers escape low pay. Interventions to increase pay progression should take account of contexts and barriers for different groups. Targeted interventions may be beneficial for groups most at risk of becoming stuck in low pay.
Find and test ways to improve support for low paid workers
Government should support combined and local authorities to trial different approaches based on best available evidence and local economic contexts. This should be accompanied by rigorous evaluation and mechanisms for sharing best practice. In addition, the current crisis strengthens the case for finding ways to improve outcomes from remote and blended learning.
2. Introduction
Many people from disadvantaged backgrounds work in low paid jobs, with limited opportunities to improve their prospects or those of their children. Workplace training and adult education have long been seen as a route to higher wages and better opportunities, both in and outside of work. But is this really the case, and if so, for whom does it work?
2.1 Low pay and progression
Low pay and limited pay progression are consistent features of the UK labour market. Although the prevalence of low pay has fallen in recent years, in 2019 15% of workers were still in low paid jobs.[footnote 3],[footnote 4],[footnote 5] Low pay is not equally spread across UK workers. In 2018, women comprised 60% of low paid workers. In 2018, there was a 3.8% ethnicity pay gap in Great Britain with White employees earning more on average than employees from Black, Asian and minority ethnic (BAME) backgrounds. There is also a substantial class pay gap, with individuals from a working class background earning an average of 24% less a year in 2018 than those with parents in professional occupations.[footnote 6] In 2019, fewer than 9 in 10 (8.7%) jobs in London were low paid compared with 16.2% for workers in the rest of the UK.[footnote 7] There are also substantial occupational and sector variations in the prevalence of low pay.[footnote 8] Many workers experience additional disadvantage in pay from the intersection of different characteristics. For example, in 2018 women from working class backgrounds earned an average of 36% less than men from professional backgrounds. Individuals who are BAME and from professional backgrounds are less likely to gain a professional role than White individuals from professional backgrounds (56% and 60% respectively).[footnote 9]
For many low paid workers there is a strong risk of remaining in low pay. Research conducted by the Resolution Foundation found that a quarter of individuals who were low paid in 2006 remained stuck in low pay 10 years later. Only 1 in 6 (17%) had escaped low pay and sustained this.[footnote 10] Research suggests that a range of demographic and work-related characteristics are associated with being stuck in low pay, such as being female, increased age, being a lone parent with young children, having a work-limiting health condition, working part- time, working in a small workplace, working in certain sectors and living outside of London.
There is also a close relationship between low skill levels and low pay.[footnote 11],[footnote 12]
Lack of long-term pay progression can have profound effects on social mobility: a substantial proportion of the population are stuck in low paid elementary occupations and this effect can reverberate down the generations. Children of low paid parents tend to do less well at school, are less likely to attend university, more likely to be unemployed, less likely to work in a professional occupation and more likely themselves to remain stuck in low paid work.[footnote 13]
2.2 Adult education and skills
There is a close relationship between low skill levels and low pay.[footnote 14] This is particularly important given the persistent problem of low basic skills levels among UK adults.[footnote 15],[footnote 16] Adult education can provide a second chance for adults to gain the qualifications and skills they need for secure and stable employment. It can also have a wider role enabling social mobility, allowing adults to upskill and retrain for better-paid occupations, with knock-on effects on their children’s opportunities and prospects.
However, the relationship between specific courses or qualifications and pay progression can be more complex. Studies have tended to show mixed results, with some qualifications yielding positive wage returns and others having no effect. Returns can vary significantly by characteristics of learning, such as qualification level, subject and sector.[footnote 17],[footnote 18],[footnote 19] Recent research using LEO data found positive returns for most qualifications and levels compared with workers holding qualifications at one level lower. Returns were particularly strong for vocational qualifications at Level 4, NVQs at Level 2 and 3, and apprenticeships.[footnote 20]
Access to training varies considerably across different groups, with those from higher socio- economic groups generally the most likely to access it. For example, the long-running survey of adult participation in learning conducted by Learning and Work Institute (L&W) has consistently shown that learners are more likely to be younger, to have left full-time education at a later age and to be in a higher socio-economic group.[footnote 21] Work-related learning is more likely to be accessed by those with higher skill levels in better paid jobs.[footnote 22],[footnote 23],[footnote 24] Research conducted for the Social Mobility Commission in 2019 reinforces this, finding that graduates are 3 times as likely to access training as those with no qualifications, and people in professional occupations to be twice as likely to do so as those in intermediate or working-class occupations. These issues are exacerbated by the substantial decline in public funding of training since 2010. There has also been a corresponding flatlining of employer-funded training – only 30% of UK employees receive continuing vocational training compared with 41% across the EU, with expenditure per employee on a training course half the EU average.[footnote 25]
Access to adult education and training is becoming ever more important due to long term economic changes. Over the coming decades, technological developments and demographic changes are likely to substantially alter the nature of work and demand for skills. Although it is difficult to quantify the actual impact of increasing automation and technological change, it is clear that technological advances will progressively alter the nature of the workplace. Low skilled workers are particularly at risk of automation, as are women, young people and part-time workers.[footnote 26] Longer working lives will also result in a need for many workers to retrain throughout their careers to keep pace with a changing labour market.[footnote 27]
2.3 Policy context
In recent years there have been substantial policy changes in the adult education and training sector. In the autumn 2017 Budget, the government announced the development of the National Retraining Scheme (NRS) in England.[footnote 28] The NRS aimed to help adults retrain into better jobs, by combining information, advice and guidance with functional skills and in-work vocational training.[footnote 29] The Government says it is now building lessons from the NRS into its National Skills Fund, worth £3 billion over the current parliament. The fund is intended to provide matched funding for adult upskilling and retraining, with a proportion reserved for strategic investment in skills, though full details are yet to be published.[footnote 30] In September 2020 the government announced the Lifetime Skills Guarantee, paid for by the National Skills Fund. The guarantee offers fully-funded Level 3 courses to all adults in England not yet qualified to that level.[footnote 31]
Recent years have seen fundamental reforms to the apprenticeship system, with the government’s clear priority an increase in both the number and quality of apprenticeship opportunities.[footnote 32] In 2017, the government introduced the apprenticeship levy across the UK, a mandatory levy of organisations with an annualised payroll of £3 million or more. In England, employers can reclaim this funding to spend on apprenticeships via digital accounts. As of April 2019, non-levy-paying employers can receive up to 95% of the cost of an apprenticeship through public funding.[footnote 33] In addition to training new recruits, apprenticeships are designed as an effective way to upskill or retrain existing employees.
In 2019 the government conducted a consultation to inform a review of post-16 qualifications at Level 3 and below in England. The review was intended to streamline the currently complex collection of approved qualifications to ensure that all qualifications that receive public funding are of high quality and serve a clear purpose. The review has also looked at routes into and through post-16 education and at strengthening the link between qualifications and the world of work.[footnote 34]
In the context of these recent and ongoing changes to the adult education system, it is important to identify the links between learning and social mobility, and how learning can most effectively be used to encourage pay progression for low paid workers. These findings are particularly important in light of the coronavirus crisis, the uneven impact of which is exacerbating existing inequalities and having the greatest impact on low paid workers.[footnote 35]
2.4 This report
This report presents the findings of research to investigate what forms of adult education and training can support adults to progress from low pay. It investigates the characteristics associated with pay progression and access to adult education and training and examines the relationship between the two. It then analyses the actual earnings returns of adult education at different levels and subjects. The report concludes by considering the implications of these findings for policy, practice and future research.
Box 1: Methodology
The principle aspect of the research was the analysis of 2 large-scale longitudinal datasets: British Household Panel Survey/Understanding Society (BHPS/USOC) and Longitudinal Education Outcomes (LEO).
- BHPS/USOC forms a combined 27-year longitudinal panel survey, first interviewed in 1991 and comprising 10,000 cases.[footnote 36] The UK-wide survey includes earnings questions and contains a very large set of questions covering respondents’ current activities, their family and work histories and details of any training they have undertaken.
- The LEO dataset links employment and earnings records from HMRC with adult learner data for England from the Individualised Learner Record (ILR). The LEO dataset is extremely large, with approximately 6 million adult learners observed each year, roughly half of whom are in the study age group (aged 25 and above) and a significant number of whom are affected by low pay. This analysis focused on learners from the 2013/14 academic year, with earnings data from 2006/07 to 2016/17.
The 2 datasets were used in combination in order to enable triangulation of results and to offset the limitations of each dataset. BHPS/USOC provides a wealth of contextual data about individual respondents which can be analysed alongside earnings data. It also includes data on any training undertaken, rather than just publicly funded training (as in LEO). LEO is limited to publicly funded training but provides a vast sample size alongside detailed information about course characteristics. It also includes actual earnings data rather than self-reported data (as in BHPS/USOC).
Analysis of both datasets has enabled this research to explore low pay progression in greater detail than previous studies. The combination of actual earnings data and granular course characteristics from LEO with the wealth of contextual data on individuals and their family background in BHPS/USOC has enabled a broad and detailed analysis of factors associated with progression from low pay.
Full methodological details are given in the technical annex.
Definitions
The study population included adults aged 25 and over residing in the UK (BHPS/USOC) or England (LEO) who had at least one episode of low pay recorded in the dataset.[footnote 37] All cases in LEO will have undertaken at least one episode of publicly funded training. Cases in BHPS/USOC may or may not have participated in any training.
The following definitions were used:
- Low pay: hourly earnings below two-thirds of the median hourly wage (BHPS/USOC), or weekly earnings below two-thirds of the median weekly wage (LEO).[footnote 38],[footnote 39]
- Adult education and training: any education or training undertaken after completing formal full-time education. BHPS/USOC contains data on any type of training undertaken, including employer provided, while LEO contains data only on publicly funded training.
- Progression: 2 definitions of progression were used. Firstly, the analysis of earnings returns used a simple definition of a significant growth in earnings. Secondly, due to the volatility of earnings at the lower end of the distribution, the remainder of the analysis used progression categories developed by the Resolution Foundation: cases were divided into ‘escapers’, ‘stuck’ and ‘cyclers’. Categories are based on 8-wave (year) sequences of earnings data – individuals must therefore have been in work and have earnings data available for 8 consecutive waves.[footnote 40],[footnote 41]
The definitions for each progression category are:
- Escapers: individuals with a period of low pay, followed by at least 3 years of consecutive higher pay at the end of the 8-wave sequence
- Stuck: individuals who are low paid for each wave of the 8-wave sequence
- Cyclers: individuals who are low paid in the first and last wave of the 8-wave sequence, with at least one wave of higher pay in between
3. Who progresses out of low pay?
3.1 Key findings
Progression from low pay differs according to workers’ characteristics and background. Age, gender, caring responsibilities, prior qualification level and occupational class are all associated with different levels of pay progression, as is residence in London.
Workers from a professional background are more likely to escape low pay than their working- class peers, with a particularly strong relationship identified between mother’s occupation and pay progression. 70% whose mother was in a professional occupation escaped (vs. 37% of those whose mother was in a working class job).
After accounting for interactions between different characteristics, the key factors that had a significant influence on pay progression were:
- the likelihood of escaping from low pay is increased by participating in more training days, having no children in the household, being younger, holding Level 2 qualifications, and living in London.
- the likelihood of being stuck in low pay is increased by being a woman, having no children in the household, being older, and living outside London.[footnote 42]
- the likelihood of cycling in and out of low pay is increased by being a woman, being older, and coming from a working-class background
Participating in more training significantly increases the chances of escaping from low pay. The results show that the chances of escaping low pay are 8 percentage points higher for those with 150 training days over 8 years compared to those with no training days over that period.
This chapter identifies which groups escape low pay, cycle in and out of low pay, or get stuck in low pay. It describes how the likelihood of these outcomes varies between different groups and analyses the factors that significantly predict progression from low pay.
3.2 Differences in pay progression across characteristics
This section examines differences in pay progression according to individual and job-related characteristics. Although this does not take account of underlying factors (as the regression analysis set out below), it explores the actual difference in progression between specific groups. Breakdowns of each characteristic by progression category are shown Tables 1 and 2.
Progression from low pay differs according to workers’ individual characteristics and background
Individuals from working class backgrounds are more likely to be in low pay and least likely to escape it, highlighting the intergenerational impacts of low pay. Individuals are most likely to escape from low pay, and least likely to be stuck, if their parents have a professional occupation. The differences are particularly marked for mother’s occupation: 70% of those whose mother had a professional occupation escaped from low pay, compared with 37% of those whose mother had a working-class occupation.
Younger adults were more likely to escape from low pay and less likely to be stuck compared to older workers. The LEO analysis splits respondents into a younger age group (aged 25 to 40 years[footnote 43]) and an older age group (aged 40 and above). In total, 45% of the younger age group escaped low pay compared with 37% of the older age group. Young people were also less likely to be stuck in low pay than the older age group (11% versus 17%). This is explored further in the regression analysis but is expected, as many young people start their careers in lower paid jobs and progress as they gain more work experience.
Women make up over three-fifths of all low paid workers[footnote 44] and are more likely than men to get stuck in low pay, with respective figures of 18% and 9% in the LEO data and 30% and 14% in BHPS/USOC. The BHPS/USOC analysis shows a substantially higher proportion of men escaping low pay, with 65% so doing compared with 36% of women.[footnote 45] Again, these differences are explored further below but are likely to occur, in part, as women tend to work in less productive, lower paying industries, such as retail and hospitality.[footnote 46]
The division of caring responsibilities within households is also likely to impact on women’s ability to progress out of low pay.[footnote 47] Our analysis shows that low paid workers in households with no children are more likely to escape low pay (53% compared to 42% in households with dependent children). The division of childcare means that most part-time work continues to be done by women (and that over half of UK workers on zero-hours contracts are women) which offers fewer prospects for wage progression. [footnote 48],[footnote 49]
Regional differences in pay progression are relatively small, with the exception of London. The proportion escaping from low pay is 45% in the northern regions of England (see footnote), 45% in the midlands and south-west, 49% in the south and east, and 40% in the devolved nations.[footnote 50] A substantially higher proportion of low paid workers in London (72%) escaped low pay. The data suggests that this is due to differences in the labour markets within these regions rather than people relocating, as most respondents stayed within the same broad region. [footnote 51],[footnote 52] Recent analysis has also shown that pay gaps within regions are often greater than those between regions.[footnote 53] London’s ‘escalator effect’ reflects the prevalence of higher-paid jobs in the capital.[footnote 54]
This matches previous studies of pay progression, although it has been argued that once occupational composition is taken into account there is no evidence for faster wage growth among low paid workers in London.[footnote 55],[footnote 56] The London labour market, especially in central London, is markedly distinct from that outside the capital in terms of the nature of the jobs it provides.
There is little difference in progression outcome between different ethnic groups. The exceptions are the ‘White Other’ and Chinese ethnic groups, which has the highest and lowest proportions of escapers, at 47% and 31% respectively. 1 in 10 in the ‘White Other’ group got stuck in low pay compared to 1 in 5 in the Chinese ethnic groups. ‘White Other’ is likely to include a relatively high proportion of Western European and North American migrants who tend to have higher level qualifications and above average earnings.[footnote 57]
People with higher level qualifications are generally more likely to progress from low pay. Although slightly different groupings are used, both LEO and BHPS data show a marked increase in the proportion of people escaping low pay as the prior qualification level rises to Level 3 or A-level. For example, BHPS shows that 52% with A-levels escaped low pay compared with 25% with no prior qualifications. While only 5% of low paid workers had degree- level qualifications, 71% of them escaped low pay.[footnote 58]
The vast majority (96% to 99%) of respondents did not increase their overall qualification level over the study period. Therefore, although having higher prior qualifications made someone more likely to escape from low pay, actually increasing their overall qualification level was not the route to pay progression. The returns to different qualification levels are explored in the next chapter.
People in professional occupations are more likely to escape low pay. While just 11% of low paid workers started the 8-wave period in professional occupations, more than two-thirds (67%) of them escaped low pay. This compares with 40% in working-class occupations (which 75% of low paid workers started in). Almost 3 in 10 in working-class occupations were stuck in low pay (29%), compared with 1 in 10 (11%) in professional occupations. This suggests that professional occupations may offer greater opportunity for earnings progression.
Job switching and moving occupational class plays an important role in progression from low pay.[footnote 59],[footnote 60] Escapers were more likely to change their current occupational class over the course of the 8-wave sequence than those in the other 2 categories: 88% of those in the stuck category remained in the same occupational class, compared with 80% of cyclers and 64% of escapers.[footnote 61] There is a mixed relationship between changes in occupational class and pay progression, however, which has implications for progression studies. Although for many respondents an increase in current occupational class occurred alongside escaping from low pay, others increased their occupational class yet remained stuck and some escaped low pay while decreasing occupational class.
Escapers participated in more training on average compared to those who were stuck on low pay. Low paid workers who progressed on to higher pay participated in an average of 5 training days per year compared to 3 days among those who were stuck in low pay. These differences may be due to other factors, for example, those participating in more training may also work in occupations with more opportunities for progression or already hold higher level qualifications, which opens up access to higher paid roles. The regression analysis below controls for these factors in an attempt to isolate the impact of training – and other factors – on different progression outcomes.
Table 1: Descriptive statistics on outcome by individual characteristic based on BHPS/USOC analysis
Escapers (%) | Stuck (%) | Cyclers (%) | |
---|---|---|---|
Father’s occupational class | |||
Professional | 53% | 22% | 25% |
Intermediate | 48% | 27% | 24% |
Working | 42% | 27% | 31% |
Mother’s occupational class | |||
Professional | 70% | 11% | 20% |
Intermediate | 55% | 22% | 23% |
Working | 37% | 28% | 35% |
Gender | |||
Male | 65% | 14% | 22% |
Female | 36% | 30% | 34% |
Caring responsibilities | |||
Dependent children | 42% | 26% | 32% |
Non-dependent children | 41% | 30% | 29% |
No children | 53% | 23% | 24% |
Region | |||
London | 72% | 15% | 13% |
South and East | 49% | 26% | 25% |
Midlands and South West | 45% | 28% | 27% |
North | 45% | 24% | 32% |
Devolved nations | 40% | 28% | 32% |
Prior qualifications | |||
Degree | 71% | 14% | 14% |
Other higher degree | 62% | 15% | 23% |
A-Level or equivalent | 52% | 19% | 29% |
GCSE or equivalent | 48% | 23% | 29% |
Other qualifications | 41% | 30% | 30% |
No qualifications | 25% | 42% | 33% |
Occupational class | |||
Professional | 67% | 11% | 21% |
Intermediate | 56% | 17% | 26% |
Working | 40% | 29% | 30% |
Source: British Household Panel Survey/Understanding Society
Table 2: Descriptive statistics on outcome by individual characteristic based on LEO analysis
Escapers (%) | Stuck (%) | Cyclers (%) | |
---|---|---|---|
Age | |||
25 to 40 | 45% | 11% | 45% |
40 and above | 37% | 17% | 45% |
Gender | |||
Male | 39% | 9% | 51% |
Female | 40% | 18% | 43% |
Ethnic group | |||
White British | 39% | 16% | 45% |
Other white | 47% | 10% | 44% |
Mixed | 42% | 12% | 46% |
African Caribbean | 42% | 10% | 48% |
Asian | 39% | 18% | 43% |
Chinese | 31% | 21% | 48% |
Other | 36% | 14% | 50% |
Prior qualifications | |||
Level 6 to 7 | 33% | 19% | 48% |
Level 4 to 5 | 39% | 11% | 50% |
Level 3 | 48% | 9% | 43% |
Level 2 | 46% | 11% | 43% |
Level 1 | 40% | 13% | 47% |
Entry level | 37% | 18% | 46% |
Missing | 34% | 21% | 45% |
Source: Longitudinal Education Outcomes
3.3 Factors that predict progression from low pay
This section presents the regression analysis that identifies which factors predict an outcome (in this case, being an ‘escaper’, a ‘cycler’ or ‘stuck’) when all observable factors are considered.
For example, are women less likely to get stuck in low paid work once occupation and household type are considered? Or, as discussed above, are low paid workers who undertake more training less likely to escape low pay once prior qualifications are taken into account?
Figures 1 to 3 show the factors that were found to predict which category a low paid worker falls into.[footnote 62] An odds ratio of below one indicates the factor is associated with a decreased likelihood of being in a particular category and an odds ratio of above one shows an increased likelihood, and statistically significant findings are denoted by stars.[footnote 63] Full details of the analysis can be found in the technical annex.
Gender, age, childcare responsibilities, prior qualifications, location and training all have a significant effect on progression outcomes
Five factors were found to be significant positive predictors of escaping from low pay. These are:
- the total number of days’ training undertaken over the course of the 8-wave sequence[footnote 64])
- having no children in the household
- being younger
- having Level 2 qualifications and residence in London
Three factors were found to be significant positive predictors of being stuck in low pay. These are:
- being female
- having no children in the household
- being older.[footnote 65]
In contrast, residence in London was associated with a significantly decreased risk of being stuck.
Three factors were found to be significant positive predictors of cycling in and out of low pay. These are:
- being female
- being older
- having a working-class background
Figure 1: Younger workers and people with no children are most likely to escape low pay
Regression analysis of escapers (odds of being an escaper controlling for factors shown)
Odds ratios | |
*** Total training days | 1 |
Male | |
Female | 0.74 |
Dependent children | |
Non-dependent children | 1.68 |
*** No children in household | 2.15 |
*** Age at date of interview | 1.77 |
*** Age-squared | 0.99 |
Degree | |
Other higher degree | 0.79 |
A-level and equivalent | 1.53 |
* GCSE and equivalent | 2.10 |
Other qualification | 1.82 |
No qualification | 0.63 |
Job: professional | |
Job: intermediate | 0.92 |
Job: routine | 0.89 |
White | |
BAME | 0.53 |
North | |
Midlands and SW | 1.06 |
* London | 2.10 |
South and East | 1.19 |
Devolved nations | 0.91 |
Father’s job: professional | |
Father’s job: intermediate | 1.50 |
Father’s job: routine | 1.15 |
Source: British Household Panel Survey/Understanding Society
Figure 2: Women and older workers are most likely to get stuck in low pay
Regression analysis of those stuck (odds of being stuck controlling for factors shown)
Odds ratios | |
Total training days | 1.00 |
Male | |
*** Female | 4.77 |
Dependant children | |
Non-dependant children | 0.99 |
* No children in household | 1.91 |
*** Age at date of interview | 1.71 |
*** Age-squared | 0.99 |
Degree | |
Other higher degree | 0.23 |
A-level and equivalent | 0.95 |
GCSE and equivalent | 1.05 |
Other qualification | 1.25 |
No qualification | 0.96 |
Job: professional | |
Job: intermediate | 1.13 |
Job: routine | 1.94 |
White | |
BAME | 1.33 |
North | |
Midlands and SW | 1.19 |
* London | 0.27 |
South and East | 0.81 |
Devolved nations | 0.66 |
Father’s job: professional | |
Father’s job: intermediate | 1.45 |
Father’s job: routine | 1.69 |
Source: British Household Panel Survey/Understanding Society
Figure 3: Women and people with no children are most likely to cycle in and out of low pay
Regression analysis of cyclers (odds of being a cycler controlling for factors shown)
Odds ratios | |
Total training days | 1.00 |
Male | |
*** Female | 4.79 |
Dependant children | |
Non-dependant children | 0.89 |
No children in household | 1.13 |
*** Age at date of interview | 2.16 |
*** Age-squared | 0.99 |
Degree | |
Other higher degree | 0.86 |
A-level and equivalent | 1.42 |
GCSE and equivalent | 1.41 |
Other qualification | 1.58 |
No qualification | 0.85 |
Job: professional | |
Job: intermediate | 1.01 |
Job: routine | 1.75 |
White | |
BAME | 1.39 |
North | |
Midlands and SW | 1.07 |
London | 0.45 |
South and East | 0.63 |
Devolved nations | 0.90 |
Father’s job: professional | |
Father’s job: intermediate | 1.28 |
* Father’s job: routine | 1.80 |
Source: British Household Panel Survey/Understanding Society
Table 3: Summary of significant factors associated with each category
Stuck:
- Female
- No children in household
- Older
Cyclers:
- Female Older
- Working-class background
Escapers:
- More training days
- No children in household
- Younger
- Holding Level 2 qualifications
- Living in London
Age was the only factor found to significantly predict membership of all 3 categories, with a younger age associated with escaping from low pay and an older age with being stuck or cycling. It may also mean that early intervention is important to prevent workers getting stuck in low pay. Other factors identified may help to target these individuals. The strong influence of age on likelihood of progressing from low pay indicates that more intensive support may be required by older workers.
In contrast, previous qualification level was mostly found not to be predictive of membership of any of the pay progression categories. The only significant predictor identified was that Level 2 qualifications were associated with an increased likelihood of escaping from low pay. For father’s occupational class, the only significant predictor was that a working-class background was associated with an increased likelihood of cycling in and out of low pay. No significant associations were found between respondents’ own occupation and pay progression, or between ethnicity and pay progression.
Participating in more training appears to have a modest impact compared to other factors. The chances of escaping low pay increase slightly (with an odds ratio of close to 1) with one extra training day. Scaling that up, our analysis suggests that the chances of escaping low pay are 8 percentage points higher for those with 150 training days over 8 years (an average of 18.75 days a year) compared to those with no training days over that period. There is no significant relationship between number of training days and cycling between or getting stuck in low pay (although the relationship is negative with the latter).
Box 2: Fixed-effects regression analysis
The regression analysis identifies that training is associated with being an escaper from low pay. However, we cannot exclude the possibility that the same factors that influence the availability of training also influence being an escaper from low pay – in other words, that people who are more likely to receive training are also more likely to escape from low pay.
The fixed-effects regressions are an attempt to approach this problem by counting all the individual-level factors together, and so accounting for the combined individual characteristics.[footnote 66] A description of the fixed-effects method can be found in the technical annex. Charts summarising the results are also shown in the technical annex (Figures 1 to 3).
These analyses found no significant effect of the number of training days on likelihood of membership of any of the 3 groups.[footnote 67] This could suggest that the likelihood of progression is affected by underlying factors not included in the model, rather than by training. However, the findings for this model are subject to a very wide degree of uncertainty: the analyses for those who are stuck and cyclers identify central estimates for some of the qualification levels, but do not successfully estimate 95% confidence intervals around these particular estimates.[footnote 68] We therefore do not consider the results of the fixed-effects model as robust.
This means that rather than concluding that escaping from low pay is due to factors that jointly predict both undertaking training and escaping low pay, we favour the interpretation from the individual-level model that training truly has an independent positive effect on escaping from low pay.
3.4 Summary
This chapter has investigated the groups which escape from low pay, and the factors that predict progression from low pay. The results show substantial differences in the chances of escaping low pay across different groups, with characteristics such as age, gender, caring responsibilities, prior qualification level and occupational class all associated with different levels of pay progression. Much of this difference between groups may be down to structural trends in the labour market. For example, women are more likely to work in industries with less scope for pay progression, such as retail and hospitality. These findings highlight the differing impact of low pay across the population, and that certain groups - such as women or those from working class backgrounds - may need specific support to help them to progress.
The results also show that low paid workers often face multiple barriers to progressing from low pay. Our analysis highlighted that, once interactions between different characteristics are taken into account, there are several key factors – such as age, gender and occupational background - that influence likelihood of pay progression. Again, it is vital for these characteristics to be taken into account in any attempt to encourage pay progression.
Finally, participating in more training was found to have a modest positive impact on pay progression when considered alongside other individual and job-level characteristics. Although the association between training and pay progression will be considered in more detail in the following chapters, this finding begins to show the benefits on pay progression that training can have.
4. How does training influence low pay?
4.1 Key findings
Low paid workers with low qualifications, particularly those with no qualifications, are significantly less likely to undertake training. Workers at different ages, occupational classes and with different levels of caring responsibilities were also found to have differing likelihoods of participating in training.
The 3 significant predictors of increased participation in training (after accounting for interactions between different characteristics) were: higher prior qualification levels, being female and living outside of London.
Individuals undertaking higher levels of learning, particular subjects (such as engineering and manufacturing), and longer courses are more likely to escape from low pay.
When analysed alongside training characteristics, many personal characteristics are not significant predictors of pay progression. The only exceptions are specific categories of age, ethnicity[footnote 69], gender, prior qualifications and disability.
This chapter examines how different types of training are associated with escaping from low pay, with a specific focus on the types of publicly funded training that low paid individuals aged 25 and over undertake. It describes how participation varies across different progression categories and types of training, and analyses training characteristics found to significantly predict progression when all available information is taken into account. It first explores the variation in training participation between different groups and what factors predict participation.
4.2 LEO
A large part of the analysis in this chapter is based on the LEO dataset. LEO provides a vast sample size alongside detailed information about course characteristics, enabling a detailed analysis of the interaction between training characteristics and pay progression. However, LEO is limited to data on publicly funded training and is therefore focused on a narrower cohort than the full study population. See the technical annex for a full discussion of the pros and cons of each dataset.
Lower qualified low paid workers, particularly those with no qualifications, are significantly less likely to undertake training
Participation in training among low paid workers differs according to individual characteristics:
-
Low paid workers with a parent in a professional occupation were the most likely to participate in training (70% and 72% based on father’s and mother’s occupation respectively). Individuals with parents in working-class occupations were the least likely to undertake training (57% for father’s occupation and 62% for mother’s occupation).[footnote 70]
- Low paid workers are less likely to participate in training as they get older, with the average number of training days declining rapidly from the age of 40 onwards.[footnote 71] Research has found that older workers are often less willing to participate in training and career development, and less likely to report that training improves their workplace skills and practices or results in a pay rise.[footnote 72],[footnote 73]
- Low paid workers in professional occupations were the most likely to have undertaken training (65%). This was followed by intermediate occupations (52%). Individuals from working-class occupations were the least likely to have undergone any training episodes (45%).
- Low paid workers with higher levels of prior qualifications are more likely to participate in training. Individuals with degrees were the most likely to have undertaken training (80%), followed by other higher degrees (73%), qualifications at A level or equivalent (71%), qualifications at GCSE or equivalent (63%) and those with other qualifications (63%). Workers with no qualifications were the least likely to participate in training (33%).
- Individuals with dependent children were the most likely to have participated in training (58%). This was followed by respondents with no children in the household (48%). Individuals with non-dependent children were the least likely to participate in training (37%).
4.3 Factors that predict participation in training
Factors that predict participation in training were identified through a regression analysis. This analysis was based on BHPS/USOC data, and hence includes any type of training undertaken.[footnote 74]
Prior qualification levels, gender and region were found to be significant predictors of participation in training (see Figure 4).
The strongest predictor is prior qualification levels, with lower qualifications significantly associated with less training. Modelling to control for other factors found that respondents with a degree had the highest mean number of training days (6.7 days), closely followed by those with an ‘other higher degree’ (5.8 days). Number of days training continued to drop with qualification level – respondents with no qualifications had the lowest mean number of training days (0.8).
See Figure 4 in the technical annex for further detail.
In addition, women receive significantly more training than men. Modelling to control for other factors found that on average, women did 2.8 days training per year compared to 1.7 days for men.[footnote 75] See Figure 5 in the technical annex for further detail. This is partly due to women being over-represented in occupations, including professional roles and care, with high rates of participation in training. The context for training is important. For example, women participating in training provided by employers are more likely to be completing mandatory training rather than training that may lead to career progression, according to previous research.[footnote 76]
Finally, geographic region is important, with living in London associated with undertaking significantly less training than other regions. Modelling to control for other factors found that respondents in London participated in an average of 1.7 training days per year, compared to a high of 3.1 for the Midlands and south-west. See Figure 6 in technical annex for further detail.
No significant association was found between having children in the household, age, current occupation or father’s occupation and mean number of training days.
Figure 4: Low paid workers with no qualifications are significantly less likely to undertake training compared to graduates
Factors predictive of mean training days per year
Odds ratios | |
Male | |
*** Female | 1.29 |
Dependant children | |
Non-dependant children | -0.63 |
No children in household | 0.38 |
Age at date of interview | -0.22 |
Age-squared | 0 |
Degree | |
Other higher degree | -0.47 |
** A-level and equivalent | -3.07 |
*** GCSE and equivalent | -4.78 |
*** Other qualification | -4.94 |
*** No qualification | -5.71 |
Job: professional | |
Job: intermediate | 0.3 |
Job: routine | -0.04 |
White | |
BAME | 2.98 |
North | |
Midlands and SW | 0.18 |
* London | -1.25 |
South and East | 0.35 |
Devolved nations | -0.71 |
Father’s job: professional | |
Father’s job: intermediate | 0.03 |
Father’s job: routine | -0.11 |
Source: British Household Panel Survey/Understanding Society
Differences between predictors of training and progression
Some of the characteristics associated with an increase in training were found to have an opposite association with progression from low pay (as set out in the previous chapter). For example, women are more likely to participate in training but less likely to progress from low pay. Residents of London are more likely to progress but less likely to participate in training.
These findings show that, while training can help pay progression, there is not a direct relationship between the two. The type of training is important (as discussed in the next section), but also the labour market context of the worker – some groups are more likely to work in roles or sectors with fewer opportunities for pay progression. This highlights the need for training to be considered alongside industrial strategies and linked to work design.
4.4 Differences in pay progression across training characteristics
This section examines the proportion of learners with different characteristics who progress from low pay. Although this does not take account of other underlying factors (as the regression analysis that follows does), it explores the actual difference in progression between specific groups.
Individuals undertaking higher level of learning, particular subjects, and longer courses are more likely to escape low pay
Individuals undertaking higher levels of learning are more likely to escape low pay. The LEO data shows a clear difference in the proportion of learners who escape from low pay based on the level of their course (Figure 5). In particular, those aiming for Level 3 and Level 4+ qualifications are most likely to leave low pay after achieving their qualification (55% and 54%). In contrast, individuals aiming for entry level or Level 1 qualifications show the lowest proportion of individuals escaping from low pay (25% and 27% respectively). In total, 21% of the entry level group are stuck in low pay, 3 times the proportion for Level 4+ qualifications. The proportion of cyclers is highest for those with missing information, with a figure of 60%. Missing information often represents programmes that are not classified at particular levels.
Figure 5: More than half of low paid adults undertaking degree level or A-level qualifications escaped low pay
Level of learning by progression category
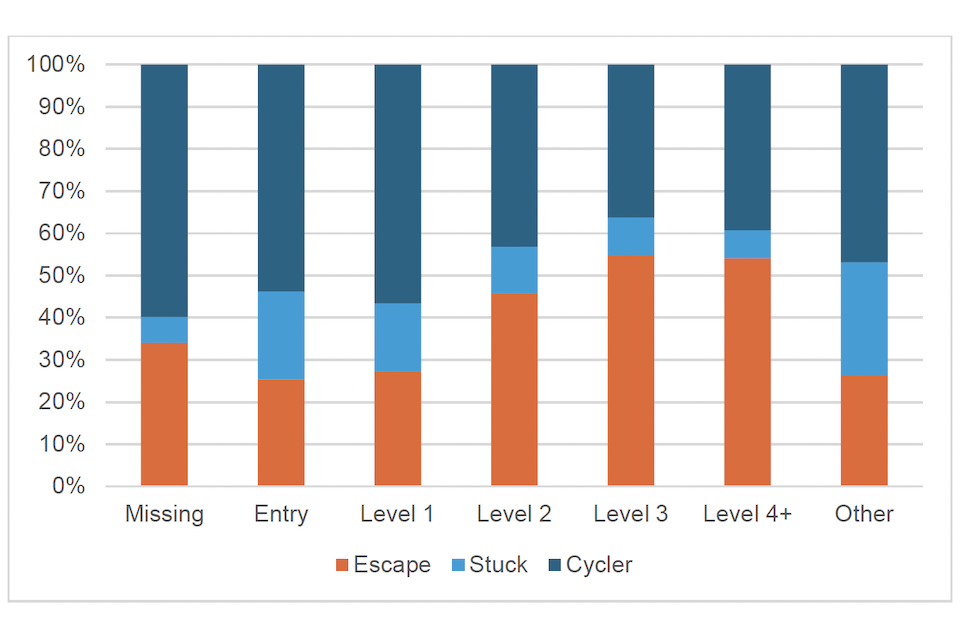
Source: Longitudinal Education Outcomes
The likelihood of workers escaping low pay varies according to subject area, with those who studied engineering and business-related subjects most likely to progress. The LEO data shows substantial differences in the proportion of learners who escape low pay based on the subject of their main learning aim (Figure 6).[footnote 77] For example, over half of those who studied engineering and technology or business-related subjects escaped from low pay.
Substantially fewer individuals who studied subjects with fewer direct links to occupations escaped from low pay, such as arts, media and publishing (24%) and languages, literature and culture (28%). The subject group with the lowest proportion of escapers was history, philosophy and theology, with a figure of 17%.
Adults learning information and communications technology (ICT) subjects – a subject relevant to many jobs – were the second least likely to escape low pay, with 1 in 5 moving on to higher pay. This suggests that some courses may have been undertaken not just for labour market value, but also to establish basic ICT skills more generally (for example, ICT citizenship, digital inclusion).
Figure 6: More than half of those who study business-related or engineering subjects escape low pay
Subject area by progression category
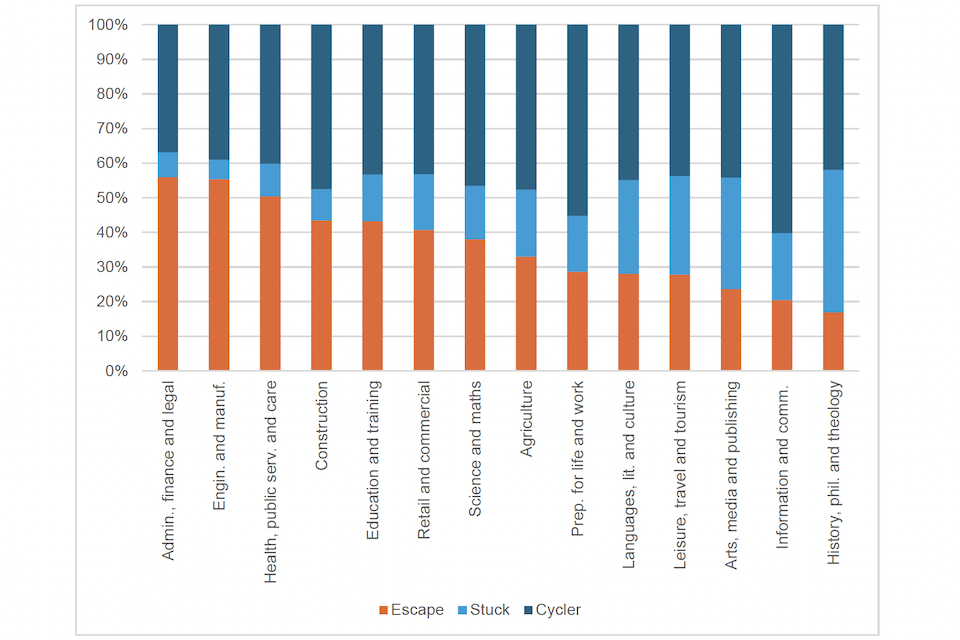
Source: Longitudinal Education Outcomes
The proportion of learners who escape low pay increases with time spent training. The LEO data shows a clear increase in the proportion of learners who escape from low pay as number of guided learning hours (GLHs) – or the expected number of hours a course involves – increases (Figure 7).[footnote 78] The percentage of escapers is small for programmes with 0–50 GLHs – around 30%, compared with 54% for people with 200–300 GLHs. The lowest percentage of people escaping from low pay is for those on courses where GLH information is missing. This often represents programmes that are not classified at particular levels (see Figure 5 for a similar effect).
Figure 7: Low paid workers studying courses with more than 200 guided learning hours are most likely to escape low pay
Guided learning hours by progression category
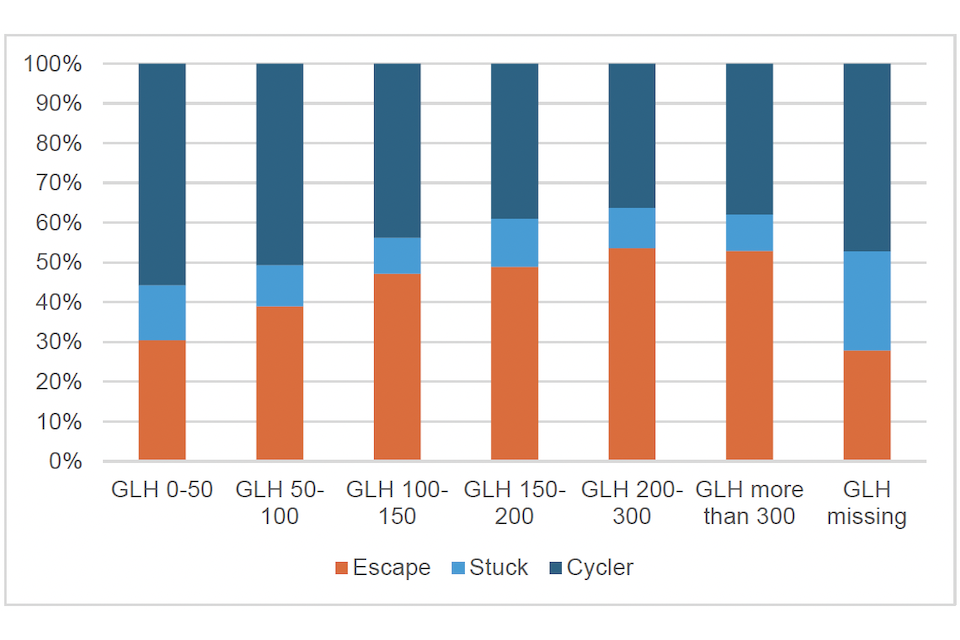
Source: Longitudinal Education Outcomes
As discussed in the previous chapter, the BHPS/USOC data also contains information about the length of time spent training. Figure 8 (a to c) shows respondents’ likelihood of belonging to each progression category by their total number of training days (over the 8-wave sequence), based on the regression analysis described in the previous chapter. Modelling to control for other factors found a clear relationship between increased number of training days and increased likelihood of escaping from low pay, but no clear association between training days and the other categories (being stuck or cycling). Figure 8a shows that training for 900 days is associated with a probability of greater than 75% of escaping low pay, for example.
Figure 8: Low paid workers are more likely to escape low pay as they participate in a higher number of training days when other factors are controlled for
Likelihood of escaping by training days:
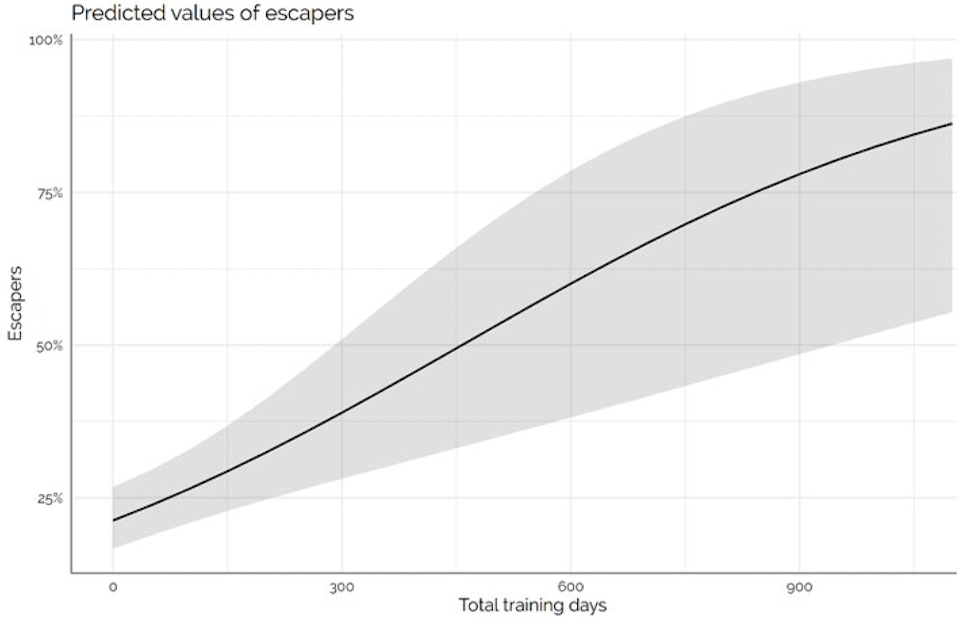
Likelihood of being stuck by training days:
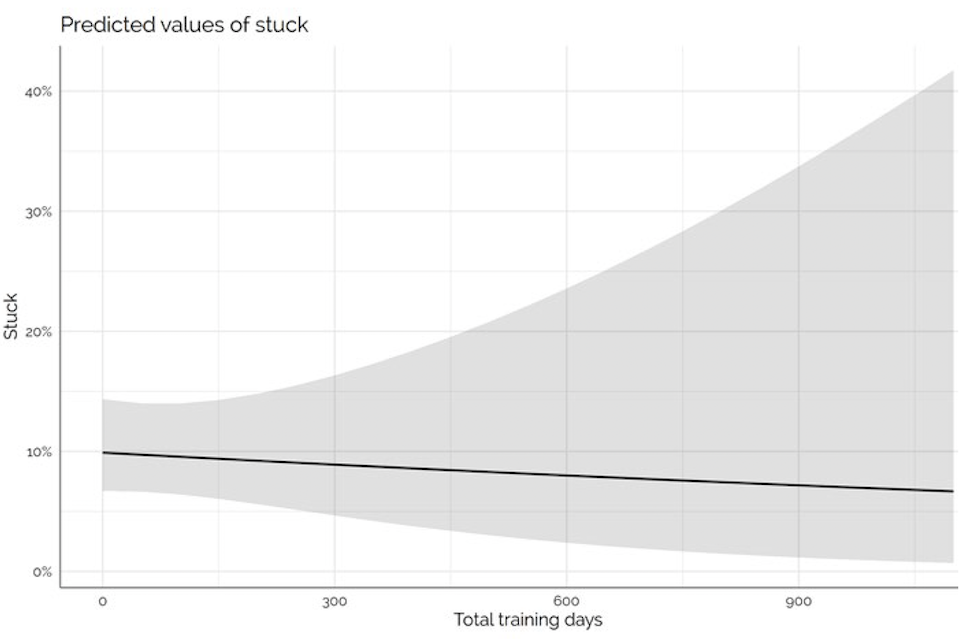
Likelihood of cycling by training days:
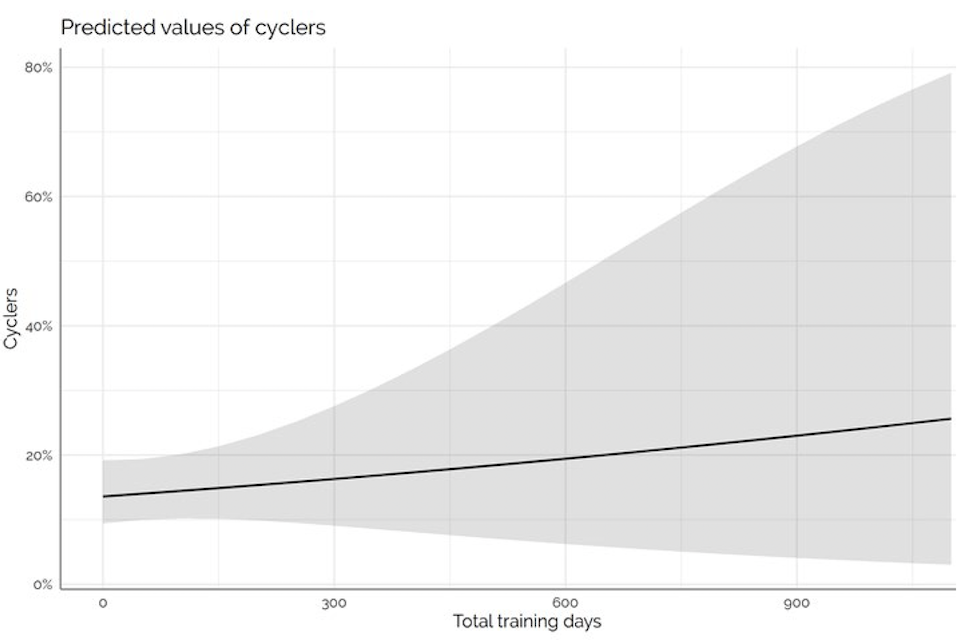
Source: British Household Panel Survey/Understanding Society. Notes: The charts show the central estimate as a dark line, with the 95% confidence intervals represented by the grey area. The scale on the left is the probability of being in the progression category. A 95% confidence interval shows the range of values of a parameter – in this case the probabilities of escaping/being stuck in/cycling in and out of low pay – for which there is a 95% chance that the value will fall within this range.
Adults who get stuck in low pay are more likely to undertake training though a college or via distance learning (Figure 9). Almost all respondents in each category received training through their employer. The next most common source of training for all low paid workers was a college or FE provider, with three-quarters (75%) referencing this. However, stuck individuals were substantially more likely to receive training through a college/further education provider (81% compared with 64% of cyclers and 61% of escapers) or through distance learning (79% compared with 54% of cyclers and 46% of escapers). Those who were stuck in low pay were also the least likely to receive training through a university, with a figure of 4% compared with 10% of cyclers and 15% of escapers. This does not imply a causal relationship between providers and outcomes for low paid workers but raise questions over the role providers can play in supporting more of their learners to progress.
Figure 9: Workers who get stuck in low pay are more likely to participate in training through a college/FE provider or via distance learning
Training provider by progression category
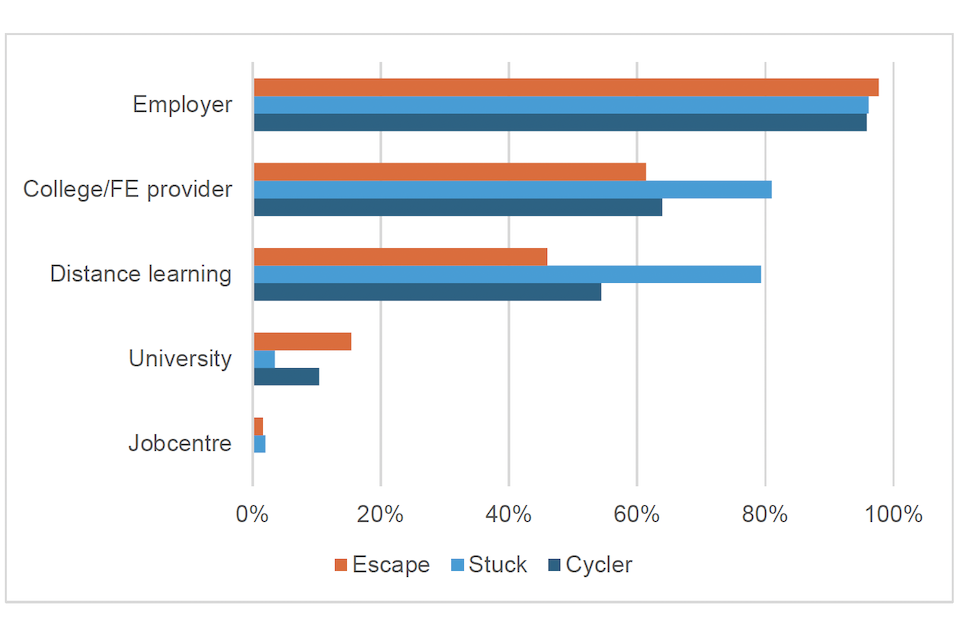
Source: British Household Panel Survey/Understanding Society
4.5 Training characteristics that predict progression from low pay
Regression analysis of training characteristics
We identified training characteristics that predict escaping from low pay using a regression analysis. As described previously, this identifies which factors predict an outcome (in this case, escaping low pay or not) when all available factors are taken into account. This analysis was based on LEO data, and hence includes only courses in receipt of public funding.[footnote 79]
Figure 10 shows the results. Characteristics are compared against base categories of:
- Level 2
- health and social care
- 0 to 50 learning hours
- Male
- White British
- south-east region
- unknown prior educational attainment[footnote 80]
Course subject, level of learning and hours play a significant role in the likelihood that workers will escape low pay. The results of the regression analysis show all training characteristics to be significant predictors of escaping low pay, whether positive (increasing likelihood of escaping) or negative (decreasing likelihood of escaping).[footnote 81]
Compared with Level 2, lower qualification levels decrease the probability of leaving low pay. Higher levels qualification levels (Level 3 and Levels 4 to 6) increase the probability of progressing.
The most common subject learnt by the study population is health and social care. Using this as a reference category, the regression analysis shows whether studying other subjects increases or decreases the probability of leaving low pay. The results show that most subjects significantly decrease the probability of leaving low pay relative to health and social care. However, studying engineering and manufacturing, administration or finance and legal are associated with an increased likelihood of progression.
The number of guided learning hours (GLH) is also a significant predictor of progression.[footnote 82] Compared with the base category of 50 hours, a higher number of guided learning hours is associated with an increased likelihood of escaping low pay. However, the benefits of increased learning hours start to diminish above 150 hours.
Compared with training characteristics, many personal characteristics are not significant (when analysed alongside training characteristics). On the one side the exceptions are being older, being female, having a disability and being of Asian / Chinese ethnic origins, which are all associated with a decreased likelihood of escaping from low pay. On the other, being of other White or African / Caribbean ethnic origins and having prior qualifications at Levels 3 to 5 were associated with an increased likelihood of escaping from low pay.
Figure 10: There is a wide variation in the effect of training characteristics on workers likelihood of escaping from low pay
Regression analysis of training and personal characteristics associated with escaping low pay
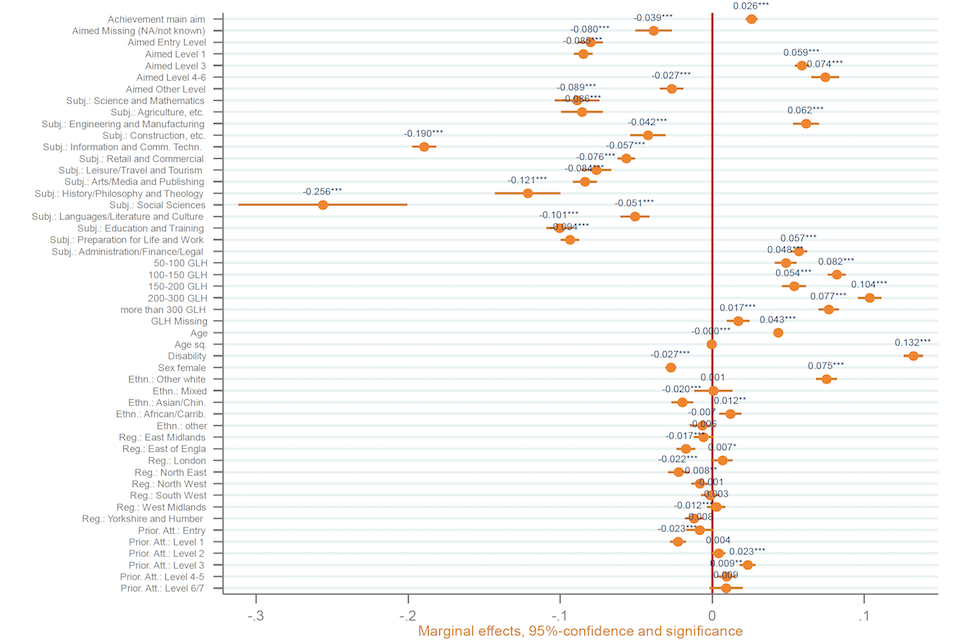
Source: Longitudinal Education Outcomes
4.6 Summary
This chapter has investigated the groups that participate in training, and the characteristics of training that are associated with progression from low pay. The results show substantial differences in the proportion of different groups who participate in training, with characteristics such as age, occupational class, caring responsibilities and prior qualifications all associated with different levels of participation in training. These results correspond with previous research for the Commission showing that poorer adults with lower levels of qualifications are the least likely to access training.[footnote 83] As with progression, the interaction of different characteristics plays a part. The results show that prior qualification levels, gender and region are key factors predicting participation in training once other factors are taken into account. These findings highlight the unequal access to the benefits of training among different parts of the population, and that certain groups may need specific support to help them to access training as part of any attempt to encourage pay progression.
Although the previous chapter identified an association between time spent training and progression from low pay, these findings show that not all training is associated with pay progression. Course subject, level of learning and hours spent learning all play a significant role in the likelihood that workers will escape low pay. These results show that for investment in training to be effective, there must be consideration of the interaction between training characteristics and pay progression.
Finally, the results show that when analysed alongside training characteristics, many personal characteristics of learners are not significant predictors of pay progression. Although there are some exceptions in relation to age, gender, ethnicity and disability, these findings demonstrate the benefits of effective training are similar for most groups of low paid workers.
5. The returns to adult education and training
5.1 Key findings
Training can have a positive effect on earnings but there is variation across different levels of learning.
The LEO analysis found that Level 3 courses (A-level or equivalent) significantly increase the earnings of low paid workers by an average of 5%. Small positive returns were also found for Level 2 and 4 courses but these were not statistically significant.
The BHPS/USOC analysis found a small positive impact on earnings from participation in training, but this was not statistically significant
This section investigates the earning returns of adult education and participation in training. It includes an analysis of adult education data in LEO, which includes only courses in receipt of public funding but is a substantially larger dataset with more detail on training characteristics. This is followed by an analysis of training recorded by BHPS/USOC, which includes any kind of training but is a smaller dataset.
Training can have a positive effect on earnings but there is variation across different levels of learning.
5.2 Impact of publicly funded adult education on earnings recorded in LEO
Earnings returns
A difference-in-differences approach was taken in order to analyse the effect of achieving qualifications on the earnings of people affected by low pay (see the technical annex for further details).[footnote 84] This approach means that time-constant differences (such as gender and ethnicity) between groups are controlled for in order to estimate the percentage effect on earnings of achieving a particular qualification.[footnote 85]
Level 3 courses (A-level or equivalent) significantly increase the earnings of low paid workers by an average of 5%, according to our analysis of different levels of publicly funded training.[footnote 86] The results of the models are shown in Tables 4 to 9. Positive returns were also found for Level 2 and 4 courses but these were not statistically significant. This finding contrasts with much of the previous literature which tends to find statistically significant and positive returns to qualifications, especially those at Level 2 and above.[footnote 87] However, this existing literature covered learners more generally and did not focus specifically on the low paid.
The results also suggest that achieving entry level qualifications is associated with significantly lower wages. This is not an uncommon finding in the literature on the effects of adult education on earnings.[footnote 88] However, testing suggests that the results are not robust and should therefore be interpreted with caution.[footnote 89]
Table 4: Difference-in-differences analysis for entry level qualifications
Model 1 | Model 2 | Model 3 | |
---|---|---|---|
Achievement | −0.046** | −0.058** | −0.050*** |
Standard error | 0.018 | 0.018 | 0.017 |
Controlling for characteristics | |||
Time | yes | yes | yes |
Learning | yes | yes | |
Learner | yes | ||
N | 100,316 | 93,392 | 89,144 |
R2 | 0.018 | 0.028 | 0.106 |
Source: Longitudinal Education Outcomes
Table 5: Difference-in-differences analysis for Level 1 qualifications
Model 1 | Model 2 | Model 3 | |
---|---|---|---|
Achievement | −0.018 | −0.020 | −0.019 |
Standard error | 0.013 | 0.013 | 0.013 |
Controlling for characteristics | |||
Time | yes | yes | yes |
Learning | yes | yes | |
Learner | yes | ||
N | 167,684 | 164,564 | 159,532 |
R2 | 0.016 | 0.030 | 0.085 |
Source: Longitudinal Education Outcomes
Table 6: Difference-in-differences analysis for Level 2 qualifications
Model 1 | Model 2 | Model 3 | |
---|---|---|---|
Achievement | 0.004 | 0.004 | 0.006 |
Standard error | 0.005 | 0.005 | 0.005 |
Controlling for characteristics | |||
Time | yes | yes | yes |
Learning | yes | yes | |
Learner | yes | ||
N | 611,280 | 610,160 | 598,532 |
R2 | 0.000 | 0.030 | 0.054 |
Source: Longitudinal Education Outcomes
Table 7: Difference-in-differences analysis for Level 3 qualifications
Model 1 | Model 2 | Model 3 | |
---|---|---|---|
Achievement | 0.052*** | 0.052*** | 0.055*** |
Standard error | 0.006 | 0.006 | 0.006 |
Controlling for characteristics | |||
Time | yes | yes | yes |
Learning | yes | yes | |
Learner | yes | ||
N | 274,260 | 273,812 | 269,180 |
R2 | 0.008 | 0.057 | 0.079 |
Source: Longitudinal Education Outcomes
Table 8: Difference-in-differences analysis for Level 4+ qualifications
Model 1 | Model 2 | Model 3 | |
---|---|---|---|
Achievement | 0.011 | 0.011 | 0.020 |
Standard error | 0.015 | 0.015 | 0.015 |
Controlling for characteristics | |||
Time | yes | yes | yes |
Learning | yes | yes | |
Learner | yes | ||
N | 52,772 | 52,668 | 51,308 |
R2 | 0.001 | 0.043 | 0.060 |
Source: Longitudinal Education Outcomes
Table 9: Difference-in-differences analysis for qualifications at other levels
Model 1 | Model 2 | Model 3 | |
---|---|---|---|
Achievement | −0.020 | 0.015 | 0.013 |
Standard error | 0.012 | 0.013 | 0.012 |
Controlling for characteristics | |||
Time | yes | yes | yes |
Learning | yes | yes | |
Learner | yes | ||
N | 366,268 | 336,868 | 330,236 |
R2 | 0.014 | 0.039 | 0.178 |
Source: Longitudinal Education Outcomes
Box 3: Changes in average pay
Individuals in this group are not only stuck in low pay but also have a lower level of pay than escapers or cyclers .
Figure 11 shows the (inflation-adjusted) median earnings for each of the progression categories: escapers from low pay, those stuck in low pay and cyclers in and out of low pay compared to the median earnings of those not in low pay. Median earnings were used in assessing the returns to adult education (as described above) and help to contextualise the findings.
Although the median earnings for individuals with no period of low pay rose from £46 to £53 per day prior to the 2008/09 recession, for those with low pay, earnings remained flat over this period. After the recession, earnings growth was generally very low in real terms for those not in low pay. Earnings grew by approximately £0.90 per day between 2009/10 and 2016/17 (from £52.25 to £53.16).
At the other end of the spectrum, the earnings of people stuck in low pay are much lower. Although they have had steeper growth since the recession than those with no period of low pay (from £10.37 to £12.40), their earnings are substantially lower than those of any other category.
Figure 11: Average earnings for those stuck in low pay remained much lower than other groups between 2006 and 2016
Development of median earnings across categories
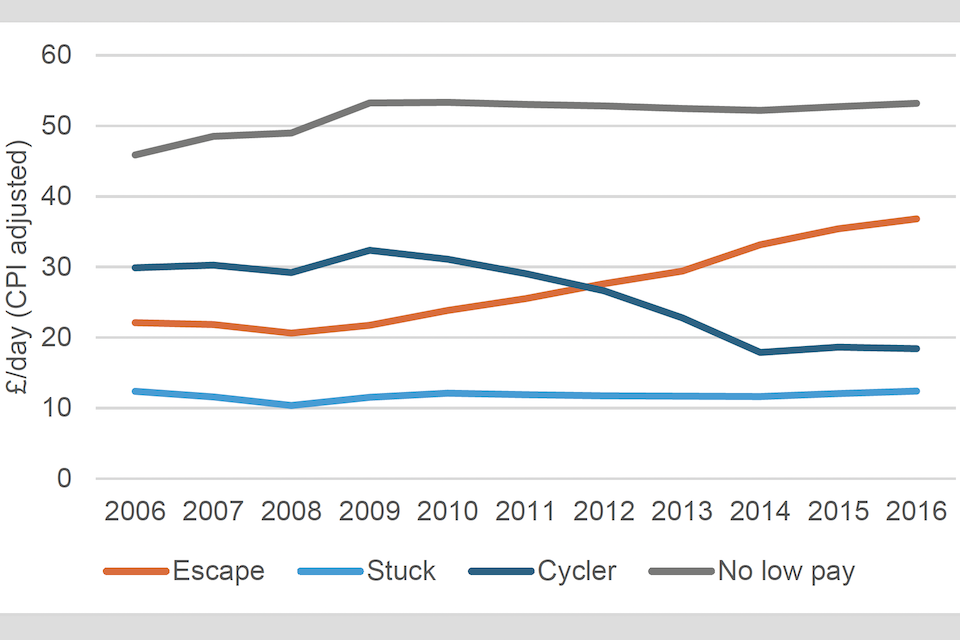
Source: Longitudinal Education Outcomes
5.3 Impact of learning and training on earnings as recorded in BHPS/USOC
Earnings returns
We used a fixed-effects approach to analyse the effect of training days on the earnings of low paid individuals. As with the previous analysis, this approach means that individual characteristics (such as gender and ethnicity) are taken into account.[footnote 90]
There is a small increase in annual earnings as the number of training days increases (Figure 12), although it is not statistically significant.[footnote 91] The rate of increase reduces as the number of training days increases. This suggests declining returns to undertaking additional training days within a year. For example, the impact on earnings is larger for an increase in the number of training days from 10 to 15 than it is for an increase from 20 to 25 days.
The only factors found to significantly increase earnings were being younger and the survey year being prior to the 2008/09 recession (as wages stagnated in years following the recession).[footnote 92] Being in a working-class job was significantly associated with a decrease in earnings.[footnote 93]
Figure 12: Earnings returns diminish as the number of training days increases
Effect of training days on real earnings increases
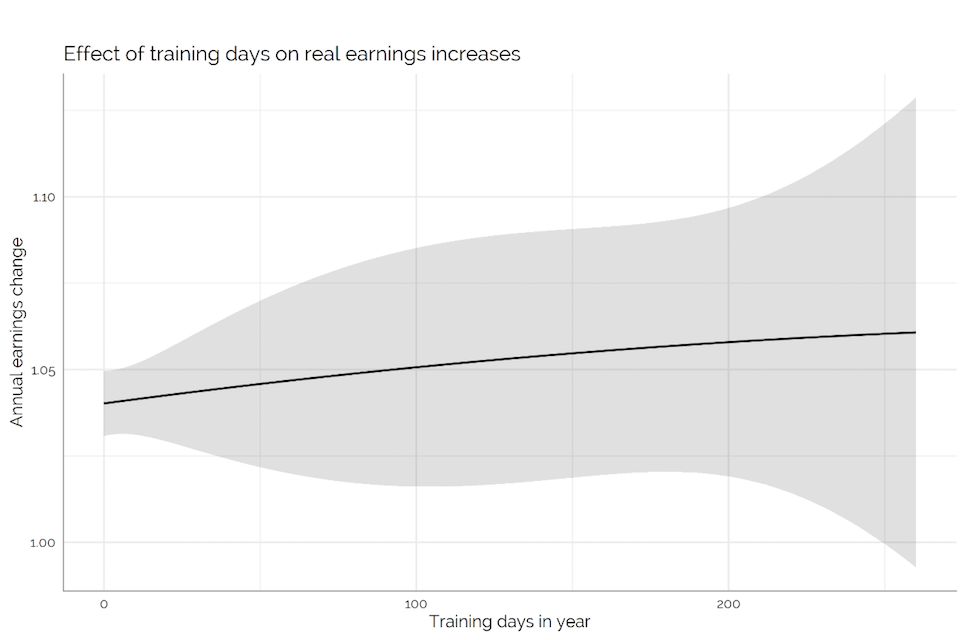
Source: British Household Panel Survey/Understanding Society
5.4 Summary
This chapter has investigated the earnings returns to adult education and participation in training. The results show that Level 3 courses (A-level or equivalent) significantly increase the earnings of low paid workers by an average of 5%. These findings demonstrate that learning at Level 3 is a key factor in enabling pay progression for this cohort of workers, and that adults should be encouraged to undertake qualifications with demonstrable earnings returns. It also indicates the importance of learning at lower levels (particularly basic skills and Level 2) that can provide a route to a higher-level course.
6. Policy implications
Low pay and a lack of pay progression have long been a stubborn feature of the UK labour market. Although workplace training and adult education have long been seen as key to addressing this, actual research findings have been mixed. This project set out to explore whether training leads to pay progression and, if so, for whom.
Low paid workers often face multiple barriers to progressing from low pay. There are substantial differences in the chances of escaping low pay across different groups, highlighting the wide range of barriers to progression individuals face. Age, gender, caring responsibilities, qualifications and training all impact on progression outcomes.
Adult education and training significantly increase the chances of escaping low pay. Those who escape low pay were likely to have participated in substantially more training than individuals who cycle in and out of low pay or are stuck.
Despite the potential benefits, many low paid workers do have access to or engage in learning. Individual characteristics such as parental background, age and gender can substantially influence someone’s chances of participating in training.
Not all training leads to higher earnings. Increased length of study (an increase in guided learning hours from 0 to 50, up to 100 to 150) and subject of learning (specifically engineering and manufacturing), were also found to be associated with escaping from low pay.
Effective investment in training has the potential to help break intergenerational cycles of low pay and support social mobility by enabling workers to progress. Many of those stuck in low pay are from working-class backgrounds with parents who were also low paid workers.
The case for investment in adult skills has strengthened in the current crisis as workers whose jobs have been affected – often low paid workers – need to retrain or upskill. It has grown over the longer term as the UK has seen weak productivity growth and automation changes the skills needed in work. But it must be allied to looking at the structure of the labour market and industrial strategies that aim to promote growth.
6.1 Ensure the new ‘Lifetime Skills Guarantee’ benefits low paid workers
Government investment should ensure that low paid workers have access to adult education and training that supports them to progress in work. The new Lifetime Skills Guarantee – giving those aged over 23 in England funding for a first Level 3 qualification – offers an opportunity for adults to develop their skills and climb the career ladder.
The new Guarantee follows a decade of decline in adult education and training. Adult participation in learning was at a 20-year low in 2019, partly due to a 45% cut in the adult education budget since 2010. As a result, the UK has relatively high concentrations of people with low skills and at the bottom of the pay distribution.
To ensure the benefits of the new Lifetime Skills Guarantee are shared by low paid workers, policymakers should:
-
Consider how to encourage individuals to participate in courses that have demonstrable benefits for pay progression. Level 3 qualifications have a significant positive effect on low paid workers earnings, but the returns vary according to subject and guided learning hours. The National Careers Service could play a role in disseminating this information to individuals seeking careers advice.
- Provide support for other costs and ensure there are flexible learning opportunities available. Low paid workers often face multiple barriers to training. Many face work and time pressures or have childcare responsibilities, for example. Low paid workers are also more likely to find meeting the additional costs associated with training difficult.
- Find ways to inspire and engage low paid workers in learning and give support to progress to Level 3 from entry level onward. Lack of awareness or interest in training also acts as a barrier to learning. It is important that low paid workers are made aware of the opportunities available by the Guarantee and how it might benefit them. Future support for union learning representatives should be considered as part of this.
- Work with employers to encourage low skilled workers to train. Our complimentary research on behavioural insights in work looks at how employers themselves can be convinced to target training and progression conversations to their low skilled workforce. It includes a behavioural model and includes case studies to help policymakers and employers find solutions.[footnote 94]
- Consider how course choice at age 16 impacts future outcomes. Our research on course choice in technical education and apprenticeship shows the impact of post-16 decisions on earnings. Addressing gender issues in low paid work requires focusing on entrenched gender issues in what courses young women chose. This and more is covered in this report.[footnote 95]
The same principles apply to activities that replace the National Retraining Scheme (NRS), which is being folded into the National Skills Fund. The NRS was designed to help low paid working adults in England to retrain, and involves a mix of information, advice and guidance with a mixture of functional skills and in-work vocational training. If NRS-related activities are to ensure progression into well-paid work, rather than participants being stuck in a different but still low paid job, then it must encourage adults to participate in training with demonstrable earnings (rather than just employment) returns (such as Level 3 qualifications), or at the least, start participants on a learning pathway to such qualifications.
6.2 Address inequalities in apprenticeships to help combat low pay
Apprenticeship schemes are designed to combine formal and on-the-job learning and are increasingly considered a successful route for individuals to both enter and progress within employment. However, research shows a wide variation in the returns to apprenticeships. For example, for both men and women, undertaking an apprenticeship before the age of 25 provides a greater wage return than doing so after 25.[footnote 96]
While some apprenticeships result in high salaries (for example, for advanced apprenticeships in engineering the wage premium at the age of 28 is similar to that of doing an engineering degree in university), there are subjects which they have a negligible or lower premium at the age of 28 compared with alternatives for people educated to the same level.[footnote 97] Although this project did not specifically consider apprenticeships, the results suggest that the key training characteristics identified in the research (Level 3 qualification, guided learning hours and subject) are important to consider when designing or choosing apprenticeship programmes.
It is also important to address inequalities in the take up of apprenticeships if low paid workers are to benefit from them. Previous research for the Commission has found substantial “disadvantage gaps” in each stage of the apprenticeship journey. Individuals from disadvantaged backgrounds are less likely to be offered an apprenticeship, to receive good quality training, to complete their apprenticeship or to progress into higher-level apprenticeships, further or higher education. They are also likely to have lower post- apprenticeship pay than their more privileged peers.[footnote 98] In addition, L&W has previously identified significant inequalities in access to apprenticeships for individuals from BAME backgrounds and those with health problems and disabilities. Gender segregation in apprenticeship subjects is also a significant challenge.[footnote 99] If apprenticeships are to be successful in improving the pay and career prospects of disadvantaged workers, then it is essential that inequalities in access, quality and outcomes are addressed.[footnote 100]
6.3 Develop an overall lifelong learning strategy for England with a focus on progression
The Lifetime Skills Guarantee will be paid for through the National Skills Fund (NSF). The government is integrating the National Retraining Scheme (NRS) into the NSF to reduce the complexity of the adult education system. The NSF and NRS sit alongside the Adult Education Budget and the Apprenticeship Levy, and the forthcoming UK Shared Prosperity Fund designed to replace EU Structural Funds. The government needs to align these funding streams under an overall strategy for lifelong learning in England and create a unified system.
A lifelong learning strategy that supports people to progress in their careers should:
- Increase investment by both government and employers at all levels – including basic skills and Level 2 to create progression routes. Investing in lower-level qualifications has its own benefits and can provide a route to higher level courses associated with earning returns.
- Ensure lifelong learning is a central part of industrial strategies to improve growth and involve employers and trade unions in the co-design of training and qualifications. Working with employers is essential to design learning that meets their needs and leads to earnings returns for low paid workers. The strategy will also need to find ways to involve employers as funders and providers of lifelong learning.
-
Focus on the outcomes of learning and its role in wider policy. Learning has a range of economic and social impacts. We should focus more on these and perhaps less on qualifications alone. This could allow learning and training to be aligned with industrial strategies, approaches to health and wellbeing and other public policy objectives.
- Empower local government to join up support. The Adult Education Budget is devolved to a number of combined authorities. Local leaders are often well placed to convene different organisations and employers to support low paid workers and address multiple barriers in ways that are difficult to do at national level. We should also consider the case for further devolution where this would benefit people and employers and help to ‘level up’ opportunities.
The economic fallout from the global pandemic means the UK government needs to find ways to protect existing jobs and support wider job creation. This is particularly important for low paid workers have been among the hardest hit. Aligning investment in skills with investment in jobs and support for retraining is likely to maximise the benefits in the short and longer term. The government’s investment in green jobs through home energy efficiency needs to be matched with adequate investment in training to enable disadvantaged adults to access and progress in jobs created.
Box 4: A message for employers
Studies show employers who invest in training can receive accrued benefits, such as increased profitability. One influential UK study found that the increase in productivity from training employees is around twice the size of the increase in their wages.[footnote 101] So businesses also gain from investment in training, with profits increasing by around the same amount as do workers’ wages. This finding of an approximate 50:50 split of the benefits of training between workers and employers is supported by earlier findings from the US and Belgium.[footnote 102],[footnote 103] These results demonstrate quantifiable benefits to business from investing in training. Thus, businesses have an enlightened self-interest to invest in training their employees.
Unfortunately, the percentage of employees receiving job related training has fallen in the last 20 years, especially for young workers aged under 25.[footnote 104]
Findings show that certain groups are substantially less likely to progress out of low pay, including those from lower socio-economic backgrounds. The route to escaping low paid work can be made more difficult by a lack of training opportunities from employers. Evidence shows that staff in low paid jobs often do not have opportunities to develop skills and that training is usually restricted to induction training.[footnote 105],[footnote 106] Evidence on how this problem can be addressed through behavioural interventions to encourage businesses to target training opportunities at low skilled workers will shortly be published by SMC.
Those in low-skilled repetitive work, in particular, often lack access to training that would develop their skills, which would allow them to improve their productivity, resulting in higher incomes.[footnote 107] Where training is available, certain groups can face particular barriers to accessing it. For example, women may struggle to fit in training around childcare or other caring arrangements. Older workers may consider themselves too old to learn.[footnote 108] Given the demonstrated return on training investment received by employers – including for workers in low-skilled jobs – increasing provision and access to training can benefit both workers and employers.
Employers should consider how to expand training opportunities to staff who currently have the least opportunity to access it. There should be a particular focus on low paid workers, particularly those from groups that are least likely to access training in general. This includes individuals from working class backgrounds and those with lower levels of prior qualifications. Demonstrable returns on investment in training ensure that both employers and employees benefit from increased access to training. Employers should consider how to remove barriers to accessing training – for example, through line management processes and developing a culture of learning.
6.4 Tailor employment and progression support to individuals
Training was one factor among a variety that can affect the chances that workers escape low pay. Interventions or approaches to increase pay progression should take account of contexts and barriers for different groups. There may be a need for targeted interventions for groups most at risk of becoming stuck in low pay.
Women often face multiple additional barriers, from caring responsibilities to lack of confidence, for example. Training may provide an effective route to enabling access to jobs with higher earnings, but these wider barriers will also need to be addressed. Older workers may also require more intensive support to progress in work.
With many low paid workers either being made redundant in the current crisis and others already accessing Universal Credit (UC), support delivered via UC also needs to be effectively tailored. Any training organised for UC claimants (for example, as part of their claimant commitment) will be more effective in raising individuals out of low pay if it is focused on qualifications with demonstrable earnings returns or is part of a learning pathway to such qualifications.
Box 5: Interventions for pay progression
The research literature on interventions for pay progression generally concludes that the subject of low pay progression is complex and that policies must be introduced that address the needs of a very varied group of workers.[footnote 109],[footnote 110] For example, recent evaluations by L&W have found that pay progression can be facilitated by personalised and flexible support based on an initial assessment of client needs, a sequenced action plan and wide ranging advisor support focused on employability skills, confidence and motivation.[footnote 111],[footnote 112] In addition, one of these evaluations found that participants undertaking training were 3 times more likely to increase their earnings than those who did not.[footnote 113]
Although trials assessing the impact of specific interventions on pay progression are limited, examples of successful approaches include:
- The UK Employment Retention and Advancement (ERA) Programme, which used a mixture of ‘post-employment’ job coaching and financial incentives in addition to job placement services.[footnote 114]
- DWP In-Work Progression Randomised Controlled Trial (IWP RCT), which found frequent advisor support for claimants to be more effective than moderate or minimal support. [footnote 115],[footnote 116]
- WorkAdvance, a US programme which provided pre-employment support, occupational skills training, job placement services and in work support.[footnote 117],[footnote 118]
- Quest, a US job training programme which provides support including remedial instruction in maths and English, counselling and job placement assistance.[footnote 119]
- Guidance and Orientation Interventions for Low-Educated Adults (GOAL), a European policy pilot project that developed educational guidance services for adults with low educational skills, with the objective of increasing participation in education and training.[footnote 120]
A recent evidence review by L&W’s What Works Unit found the key lessons from evaluations of pay progression interventions to be that:
- Interventions to support pay progression tend to have a greater effect on individuals furthest from the labour market and on the lowest incomes.
- Investments to enable low-income workers to achieve the ‘right job’ may have a greater impact than ‘work first’ approaches.
- It is important for interventions to respond to local demand based on an understanding of the local labour market and local opportunities, and to work closely with employers in design and delivery.
- Effective marketing and networking can be crucial in engagement participants in interventions.
- Individual-led support can be more effective when combined with needs assessment and tailored support from a trusted advisor.[footnote 121]
6.5 Find and test ways to improve support for low paid workers
Several US-based training programmes designed to support disadvantaged adults to find and progress in work have been found to have demonstrable earnings effects.[footnote 122] Yet evidence of these types of initiative working in a UK context remains weak.
The Government should support combined and local authorities to trial different approaches based on best available evidence and local economic contexts across the UK. This should be accompanied by rigorous evaluation and mechanisms for sharing best practice.
The current crisis strengthens the case for finding ways to improve outcomes from remote and blended learning. A relatively high proportion of workers stuck in low pay already train through distance learning and the current social distancing measures have led to a significant increase in online learning. While it is important to understand more about the distance learning low paid workers are undertaking, experience from adult basic education programmes, particularly for learners with the lowest skills levels, suggests the most effective models are hybrid ones with tutors delivering at least half of the instruction.[footnote 123] There are also questions about how to build people’s confidence in online learning and equip them with the necessary digital skills, and how online tools can improve learners’ motivation to persist with learning.
7. Glossary
Adult education and training: Any education or training undertaken after completing formal full- time education
Apprenticeships: A work-based training system, where apprentices earn a qualification after completing a blended mix of study and work. Apprentices must complete 20 per cent of their training off-the-job, be paid the apprenticeship minimum wage (£4.15/hr for those under 19 or aged 19 and over and in their first year) and pass an end point assessment.
BHPS/USOC: Linked survey data from the British Household Panel Survey (BHPS) and subsequent Understanding Society survey (USOC), to form a combined 27-year longitudinal panel survey. BHPS started in 1991, and from 2009 was expanded to become USOC.
Cycler: Individuals who are low paid in the first and last wave of the 8-wave sequence, with at least one wave of higher pay in between
Difference-in-differences approach: A difference-in-differences approach compares changes over time in a treatment group with changes over the same time period in a matched control group.
Eight-wave sequence: A sequence of earnings data for 8 consecutive survey waves. Individuals must have been in work and have earnings data for each wave.
Elementary occupations: Jobs with low skill requirements.
Escaper: Individuals with a period of low pay, followed by at least 3 years of consecutive higher pay at the end of the 8-wave sequence
Fixed-effects regression analysis: See regression analysis definition. A fixed-effects analysis counts all individual-level factors together (such as gender and age), and so accounts for the effect of combined individual characteristics.
Further education: Typically refers to classroom-based learning at Further Education (FE) colleges or providers. Colleges offer a wide array of offerings, including academic routes (such as basic maths and English courses, GCSEs and A levels), as well as hundreds of technical qualifications (such as BTEC), apprenticeships, English as a second language courses, adult education classes, and more. Students can start at age 14 or 16, depending on the college. Most colleges and providers are for-profit and receive significant state investment. Sixth form colleges are sometimes included in this definition, though traditionally they mainly offer academic qualifications and have fewer students enrolled compared to FE colleges.
Household status: Categorises individuals based on whether they have dependent children or non-dependent children in their household, or live in another type of household (for example, house share).
Intermediate occupations: These are defined as NS-SEC 3 and 4 occupations. Examples include: shopkeepers, paramedics, and police officers.
Longitudinal education outcomes (LEO): This is an experimental new data source that brings together administrative data on education, employment, benefits and earnings at an individual level. The analysis for this project used longitudinal economics outcomes data linked to data from adult learners available from the Individualised Learner Records (ILR).
Longitudinal panel survey: Repeated surveying of the same respondents at set periods over a long time frame.
Low pay: Hourly earnings below two-thirds of the median hourly wage (BHPS/USOC analysis) or weekly earnings below two-thirds of the median weekly wage (LEO analysis).
National living wage: The national minimum wage that an employer must pay its workforce. Rates vary by age but for those aged over 25, it is currently set at £8.72 as of April 2020.
Occupational class: Social class based on occupation type. For this study 3 groups of occupational class were used: working class occupations, intermediate occupations and professional occupations (see separate definitions).
Pay progression: An increase in average earnings (excluding bonuses). For this study 2 definitions of pay progression were used. Firstly, the analysis of earnings returns used a simple definition of a significant growth in earnings. Secondly, due to the volatility of earnings at the lower end of the distribution, the remainder of the analysis used progression categories developed by the Resolution Foundation: cases were divided into ‘escapers’, ‘stuck’ and ‘cyclers’ (see separate definitions).
Professional occupations: These are defined as NS-SEC 1 and 2 occupations – managerial and professional (as per standard reporting). Examples include: CEOs, senior police officers, doctors, journalists, barristers, solicitors, teachers and nurses.
Regression analysis: A regression analysis identifies which factors predict an outcome when all available factors are taken into account.
Social mobility: The chances of people from different backgrounds ending up in a different occupational group from their origin in comparison to others from different social backgrounds.
Socio-economic group: A combined total measure of an individual’s or a family’s economic and social position in relation to others based on income, education and occupation.
Stuck: Individuals who are low paid for each wave of the 8-wave sequence
Survey wave: Each separate survey in a survey series. For BHPS/USOC, waves were usually conducted once a year.
Vocational qualifications: Work-related qualifications combining the required knowledge and practical skills to meet recognised standards for different jobs and sectors.
Voluntary living wage: An hourly rate set by the Living Wage Foundation, which employers can voluntarily pay. It is based on a ‘minimum cost of living, based on a basket of household goods and services.’ It is currently £9.30 outside of London and £10.75 in London.
Working class occupations: These occupations are defined as NS-SEC 5, 6, 7 occupations – routine and manual. Examples include: receptionists, electricians, plumbers, butchers and van drivers.
8. About the Commission
The Social Mobility Commission is an independent advisory non-departmental public body established under the Life Chances Act 2010 as modified by the Welfare Reform and Work Act 2016. It has a duty to assess progress in improving social mobility in the UK and to promote social mobility in England.
The Commission board comprises:
- Sandra Wallace, Interim Co-Chair, Joint Managing Director Europe at DLA Piper
- Steven Cooper, Interim Co-Chair, Chief Executive Officer, C. Hoare and Co
- Alastair da Costa, Chair of Capital City College Group
- Farrah Storr, Editor-in-chief, Elle
- Harvey Matthewson, Aviation Activity Officer at Aerobility and Volunteer
- Jessica Oghenegweke, Broadcast and Digital Coordinator at The Roundhouse
- Jody Walker, Senior Vice President at TJX Europe (TK Maxx and Home Sense in the UK)
- Liz Williams, Group Director of Digital Society at BT
- Pippa Dunn, Founder of Broody, helping entrepreneurs and start ups
- Saeed Atcha, Chief Executive Officer of Youth Leads UK
- Sam Friedman, Associate Professor in Sociology at London School of Economics
- Sammy Wright, Vice Principal of Southmoor Academy, Sunderland
9. About Learning and Work Institute and NIESR
Learning and Work Institute (L&W) is an independent policy, research and development organisation dedicated to lifelong learning, full employment and inclusion. We research what works, develop new ways of thinking and implement new approaches. Working with partners, we transform people’s experiences of learning and employment.
The National Institute of Economic and Social Research (NIESR) is Britain’s longest established independent research institute, founded in 1938. Our mission is to carry out research into the economic and social forces that affect people’s lives and to improve the understanding of those forces and the ways in which policy can bring about change. The Institute is independent of all party political interests and is not affiliated to any single university, although our staff regularly undertake projects in collaboration with leading academic institutions. Our work with the Social Mobility Commission was part of NIESR’s research for the Centre for Vocational Education Research (CVER). CVER was launched in March 2015, funded by the Department for Education, to create a research institution that will advance our understanding of the requirements for vocational education in the UK.
L&W and NIESR take all reasonable steps to ensure the accuracy and quality of their publications but cannot take responsibility for any inaccuracies or the purposes to which the information is put. Any opinions expressed in the paper are those of the authors, and do not necessarily reflect the views of either of the 2 organisations.
-
Individuals from a White background, who do not identify as English, Welsh, Scottish, Northern Irish, British, Irish, Gypsy or Irish Traveller. ↩
-
Social Mobility Commission, Developing behavioural insights to in-work training and progression, 2020 ↩
-
Defined as an hourly rate of less than two-thirds of the median wage. ↩
-
Resolution Foundation (2020). A new settlement for the low paid. ↩
-
Resolution Foundation (2019). Low pay Britain 2019. ↩
-
Social Mobility Commission (2019). The state of the nation 2018-2019: social mobility in Great Britain. ↩
-
ONS (2019). Low and high pay in the UK: 2019. ↩
-
Lee, N, Green, N, Sissons, P (2018). Low-pay sectors, earnings mobility and economic policy in the UK. Policy and Politics 46(3). ↩
-
Social Mobility Commission (2019). The state of the nation 2018-2019: social mobility in Great Britain. ↩
-
Resolution Foundation (2017). The great escape? Low pay and progression in the UK’s labour market. ↩
-
Social Mobility Commission (2019). The state of the nation 2018-2019: social mobility in Great Britain. ↩
-
Social Mobility Commission (2019). The adult skills gap: is falling investment in UK adults stalling social mobility? ↩
-
Social Mobility Commission (2019). The state of the nation 2018-2019: social mobility in Great Britain. ↩
-
Social Mobility Commission (2019). The adult skills gap: is falling investment in UK adults stalling social mobility? ↩
-
Social Mobility Commission (2019). The state of the nation 2018-2019: social mobility in Great Britain. ↩
-
Department for Education (DfE) (2019). Level 2 and 3 attainment in England: attainment by young people aged 19 in 2019. ↩
-
Conlon, G., Hedges, S., McIntosh, S., Morris, D., Patrignani, P. (2017). The payoff to vocational qualifications: reconciling estimates from survey and administrative data. Centre for Vocational Education Research. ↩
-
Institute for Fiscal Studies (2020). The impact of undergraduate degrees on lifetime earnings. ↩
-
McIntosh, S., Morris, D. (2016). Labour market returns to vocational qualifications in the Labour Force Survey. Centre for Vocational Educational Research. ↩
-
Patrignani, P., Conlon, G., Hedges, S. (2017). The earnings differentials associated with vocational education and training using the Longitudinal Education Outcomes data. Centre for Vocational Educational Research. ↩
-
Smith, R., Eggleston, C., Jones, E., Aldridge, F. (2019). Adult Participation in Learning Survey 2019. Learning and Work Institute. ↩
-
Social Mobility Commission (2019). The adult skills gap: is falling investment in UK adults stalling social mobility? ↩
-
Zwart, S., Baker, M. (2018). Improving productivity and job quality of low-skilled workers in the United Kingdom. OECD Economics Department Working Paper No. 1457. ↩
-
Green, A. (2016). Low skills traps in sectors and geographies: underlying factors and means of escape. Institute for Employment Studies. ↩
-
Social Mobility Commission (2019). The adult skills gap: is falling investment in UK adults stalling social mobility? ↩
-
ONS (2019). Which occupations are at highest risk of being automated? ↩
-
Evans, S. (2019). Tomorrow’s world: Future of the labour market. Youth Commission Report 3. Learning and Work Institute. ↩
-
HM Treasury (2017). Autumn Budget 2017. ↩
-
Department for Education (2019). National retraining scheme. ↩
-
Conservatives (2019). Our plan: Conservative manifesto 2019. ↩
-
HM Government (2020). Major expansion of post-18 education and training to level up and prepare workers for post-COVID economy. ↩
-
HM Government (2015). English apprenticeships: our 2020 vision. ↩
-
Education and Skills Funding Agency (2019). Pay the apprenticeship levy. ↩
-
DfE (2019). Review of post-16 qualifications at level 3 and below in England. ↩
-
Learning and Work Institute (2020). Coronavirus and the labour market: impacts and challenges. ↩
-
A longitudinal panel survey involves repeated surveying of the same respondents at set periods over a long time frame. ↩
-
An episode of low pay is defined as a single wave (BHPS/USOC) or year (LEO) in which mean earnings are below two-thirds of the median low-pay threshold. ↩
-
Median earnings vary by year and dataset. For context, the Annual Survey of Hours and Earnings estimates that the median hourly earnings for all employees in 2019 were £13.27 (equating to £25,531.48 annually based on a 37-hour week). The low-pay threshold for hourly earnings in 2019 is therefore £8.85 (£17,027.40 annually). ONS. (2019). Low and high pay in the UK: 2019. ↩
-
Bonus payments are excluded from the definition. ↩
-
Resolution Foundation (2017). The great escape? Low pay and progression in the UK’s labour market. ↩
-
The original Resolution Foundation study used 10-year sequences, so we initially investigated the possibility of using 10-year sequences in our research. However, this resulted in too small a sample size for the BHPS/USOC analysis, and so it was reduced to an 8-year sequence. ↩
-
No children in the household predicts both escaping from and being stuck in low pay. This may appear contradictory, but likely indicates that cyclers are less likely than both groups to have no children. ↩
-
Age at start of most recent qualification. ↩
-
Tinson, A., Aldridge, H., Whitham, G. (2016). Women, work and wages in the UK: understanding the position of women in the UK labour market and the need for effective policy response. National Policy Institute. ↩
-
Although this pattern is not repeated in the LEO data, this may be due to population differences between the 2 datasets: LEO includes only those who have enrolled on a publicly funded further education course, so it is a narrower cohort than BHPS/USOC. ↩
-
Joyce, R. and Xu, X. (2019). The gender pay gap: women work for lower-paying firms than men. Institute for Fiscal Studies. ↩
-
Dias, M.C., Joyce, R. and Parodi, F. (2018). Wage progression and the gender pay gap: the causal impact of hours of work. Institute for Fiscal Studies. ↩
-
Plunkett, J. (2011). The missing million: the potential for female employment to raise living standards in low to middle income Britain. Resolution Foundation. ↩
-
Tinson, A., Aldridge, H., Whitham, G. (2016). Women, work and wages in the UK: understanding the position of women in the UK labour market and the need for effective policy response. National Policy Institute. ↩
-
’North’ combines the regions of North East, North West and Yorkshire and the Humber. ‘Midlands and South-West’ combines the regions of East Midlands, West Midlands and South West. ‘South and East’ combines the regions of South East and East of England. ‘Devolved nations’ combines Scotland, Wales and Northern Ireland. ↩
-
See Social Mobility Commission (2020). Moving out to move on: understanding the link between migration, disadvantage and social mobility. Institute for Employment Studies. ↩
-
99% of respondents in the stuck category, 99% of cyclers and 98% of escapers stayed within the same broad region. ↩
-
McCurdy, C. (2019). Election 2019: how Britain’s North-South divide is changing. ↩
-
Champion, T., Coombes, M., Gordon, I. (2013). Urban escalators and inter-regional elevators: The difference that location, mobility and sectoral specialisation make to occupational progression. SERC Discussion Paper 139, London: Spatial Economics Research Centre, LSE. ↩
-
CIPD (2014). Pay progression: understanding the barriers for the lowest paid. ↩
-
Velthuis, S., Sissons, P., Berkeley, N. (2019). Do low paid workers benefit from the urban escalator? Evidence from British cities. Urban Studies 56(8). ↩
-
Platt, L. (2019). Migration and inequality. Institute for Fiscal Studies. ↩
-
LEO data shows a peak in the proportion of escapers at level 3 (48%), with a lower proportion escaping as respondents’ highest qualification increases to levels 4 to 5 (39%) and to levels 6 to 7 (33%). This is a surprising finding, but as neither dataset provides information about the subject of prior qualifications, it is not possible to investigate whether this drop is caused by subject-specific factors. ↩
-
Kirkup, J., Corfe, S. and others (2018). Pay progression for low paid workers. Social Market Foundation. ↩
-
Resolution Foundation (2017). The great escape? Low pay and progression in the UK’s labour market. ↩
-
Occupation group is based on the 3-code classification. More nuance may have been identified through a 5 or 7-code classification. ↩
-
Categorical factors (such as gender) are shown in relation to a ‘base’ category. For gender, the category shown is Female, with Male as the base category (and therefore not included). The other base categories are: prior qualification level - degree, caring responsibilities - dependent children, occupation - professional class, father’s occupation - professional class, ethnicity - White, regions - North. ↩
-
One star = significant at the 10% level, 2 stars = significant at the 5% level, and 3 stars = significant at the 1% level. The odds ratio describes the change in the probability of an outcome (escape, stuck or cycler) associated with the presence of a characteristic. Odds ratios above 1 indicate an increased probability and below 1 a decreased probability. ↩
-
Training days have been included as a numeric rather than a categorical variable, and therefore the coefficient relates to the impact on the chances of being an escaper of just one extra training day over a sequence. ↩
-
’No children in the household’ covers all households with no dependent or non-dependent children, including childless couples and house shares. No children in the household was found to predict both escaping from and being stuck in low pay. This may appear contradictory, but likely indicates that cyclers are less likely than both groups to have no children. ↩
-
In this analysis, we have used an interview-level dataset, so the 8 waves in a sequence have 8 observations. ↩
-
No significant effects were found for any of the individual-level characteristics that were included in the model (those that change over time) either. ↩
-
The analyses for those who are stuck (and cyclers) identify central estimates for some of the qualification levels, but do not successfully estimate 95% confidence intervals around these particular estimates. ↩
-
These results for ethnicity contrast with those from the previous chapter which used data from the BHPS / Understanding Society. The results here are based on a different dataset: Longitudinal Education Outcomes (LEO) which only covers publicly funded training and also uses a different econometric specification because LEO includes a lot more information on the nature of the learning being done compared to the BHPS / Understanding Society. Given these methodological differences, some differences between the 2 sets of results are inevitable. ↩
-
These findings are similar to those of recent research conducted for the Commission, which found that the likelihood of having received training in the previous 3 months increases with parents’ occupational class. Social Mobility Commission (2019). The adult skills gap: is falling investment in UK adults stalling social mobility? ↩
-
The specification for the model was in quadratic form. Confidence intervals indicate that this was a suitable choice for the analysis. ↩
-
Unwin, L., Davey, G., Fuller, A., Leonard, P. (2015). Supporting an ageing workforce: implications for working life, training and skills policy in England – a literature review.. LLAKES Research Paper 51. ↩
-
Felstead, A. (2010). Closing the age gap? Age, skills and the experience of work in Great Britain. Ageing and Society 30(8). ↩
-
In this analysis, we have included people who did not train (with zero days training). ↩
-
This corresponds with findings from the Annual Population Survey. ONS (2019). Characteristics and benefits of training at work, UK: 2017. ↩
-
Aldridge, F., Egglestone, C. (2015). Learning, skills and progression at work: analysis from the 2015 Adult Participation in Learning Survey. National Institute of Adult Continuing Education (NIACE). ↩
-
Learning aim with the highest number of guided learning hours ↩
-
We only consider GLHs that relate to the main vocational learning aim. ↩
-
It should be noted that LEO contains limited data on personal characteristics, and so it is possible that some findings are influenced by unobservable characteristics (factors for which we don’t have data). ↩
-
These are the most frequent values for these categories. ↩
-
Significant predictors are those where the 95% confidence intervals do not cross the red (zero-percentage points) line. Therefore, the hypothesis that these values are equal to zero is rejected via statistical tests. ↩
-
Number of guided learning hours is a notional measure roughly associated with the number of hours spent under instruction. ↩
-
Social Mobility Commission (2019). The adult skills gap: is falling investment in UK adults stalling social mobility? ↩
-
A difference-in-differences approach compares changes over time in a treatment group with changes over the same time period in a matched control group. ↩
-
The regression analysis of the effects of training characteristics on progression (as described in the previous section) indicates that learning characteristics differentially influence the probability of leaving low pay. Therefore, the difference-in-differences analysis of the influence of learning characteristics on the wages of low-pay groups takes account of differences in levels and subjects undertaken. ↩
-
This finding holds across the specifications and is robust when tested using a placebo test (a test which looks for unobserved/unobservable variation between achievers and non-achievers that is not being controlled for). ↩
-
Conlon, G., Hedges, S., McIntosh, S., Morris, D., Patrignani, P. (2017). The payoff to vocational qualifications: reconciling estimates from survey and administrative data. Centre for Vocational Education Research. ↩
-
Conlon, G., Hedges, S., McIntosh, S., Morris, D., Patrignani, P. (2017). The payoff to vocational qualifications: reconciling estimates from survey and administrative data. Centre for Vocational Education Research. ↩
-
A placebo test found some significant differences between achievers and non-achievers in the population of people aiming for Level 1 qualifications in the period before they participate in adult education. ↩
-
A dummy variable was included in the model here to control for the fact that real earnings increases before the 2008/09 recession were on a different scale to real earnings increases afterwards. ↩
-
1.00 represents no increase in earnings, 1.05 an increase of 5% and 1.10 an increase of 10%. ↩
-
As a result of the major differences in the earnings distributions before and after the 2008/09 recession, we included time period (pre- and post-2008) as a factor in the analysis. This factor was found to be significantly associated with earnings, confirming (and controlling for) the ongoing impact of this recession. ↩
-
Different specifications were also tested using training days as a linear predictor and with a quadratic form, to take account of non-linearity in effects. ↩
-
Social Mobility Commission, Behavioural insights in work, 2020 ↩
-
Social Mobility Commission, The road not taken, 2020 ↩
-
Melville, D., Pintado, A. (2019). Economic returns to apprenticeships. Learning and Work Institute, unpublished memo. ↩
-
McNally, S. (2018). Apprenticeships in England: what does the research tell us? Centre for Vocational Education Research. ↩
-
Social Mobility Commission (2020). Apprenticeships and social mobility: fulfilling potential. ↩
-
Learning and Work Institute (2018). All change: where next for apprenticeships? ↩
-
Social Mobility Commission (2020). Apprenticeships and social mobility: fulfilling potential. ↩
-
Dearden, L., Reed, H., Van Reenan, J. (2005). The impact of training on productivity and wages: evidence from British Panel Data. Institute of Fiscal Studies Working Paper W05/16. ↩
-
Blundell, R., Dearden, L., Meghir, C., Sianesi, R. (1999). Human capital investment: the returns from education and training to the individual, the firm and the economy. Fiscal Studies 20(1). ↩
-
Konings, J., Vanormelingen, S. (2010). The impact of training on productivity and wages: firm level evidence. Institute for the Study of Labor (IZA) Discussion Paper No. 4731. ↩
-
Labour Force Survey data for April to June 2020 compared to April to June 2000. For workers aged 16 to 64 the percentage of those receiving job related training have fallen by 4 percentage points. For workers aged 16 to 17 and 18 to 24 respectively, the falls have been 14 and 9 percentage points respectively. ↩
-
Zwart, S., Baker, M (2018). Improving productivity and job quality of low-skilled workers in the United Kingdom. OECD Economics Department Working Paper No. 1457. ↩
-
Green, A. (2016). Low skills traps in sectors and geographies: underlying factors and means of escape. Institute for Employment Studies. ↩
-
Mohr, S., Troltsch, K., Gerhards, G. (2016). Job tasks and the participation of low-skilled employees in employer provided continuing training in Germany. Journal of Education and Work 29(5). ↩
-
Egglestone, C, Stevens, C., Jones, E., Aldridge, F. (2018). Adult Participation in Learning Survey 2017. Learning and Work Institute and DfE. ↩
-
Adam, D., Atfield, G., Green, A.E. (2017). What works? Policies for employability in cities. Urban Studies 54(5). ↩
-
Cort, P., Mariager-Anderson, K., Thomsen, R. (2018). Busting the myth of low-skilled workers: destabilizing EU LLL policies through the life stories of Danes in low-skilled jobs. International Journal of Lifelong Education 37(2). ↩
-
Colechin, J., Murphy, H., Stevens, C., Penacchia, J., Ray, K., and Vaid, L. (2017). Evaluation of the Skills Escalator Pilot. Learning and Work Institute ↩
-
Murphy, H., Bennett, L., Klenk, H., Ray, K and Stevens, C. (2018), Step Up: Trialling new approaches supporting low paid workers to progress their careers, Learning and Work Institute. ↩
-
Colechin, J., Murphy, H., Stevens, C., Penacchia, J., Ray, K., and Vaid, L. (2017). Evaluation of the Skills Escalator Pilot. Learning and Work Institute ↩
-
Hendra, R., Riccio, J.A., Dorsett, R. and Robins, P.K. (2015). Breaking the low pay, no pay cycle: the effects of the UK Employment Retention and Advancement programme. ↩
-
Department for Work and Pensions (2018). Universal Credit: in-work progression randomised controlled trial. ↩
-
Frequent support claimants met their Work Coach fortnightly to get support and review agreed actions, moderate support claimants met with their Work Coach for these purposes every 8 weeks, and minimal support claimants had an initial telephone appointment and a follow up telephone call 8 weeks after starting on the trial. ↩
-
Heinrich, C.J., Mueser, P.R., Troske, K.R., Jeon, K-S and Kahvecioglu, D.C. (2013). Do public employment and training programs work? ↩
-
Schaberg, K. and Greenberg, D.H. (2020). Long-Term Effects of a Sectoral Advancement Strategy: Costs, Benefits and Impacts from the WorkAdvance Demonstration. ↩
-
Roder and Elliott. (2018). Escalating gains: the elements of Project Quest’s success. ↩
-
OECD (2019). Getting skills right: engaging low-skilled adults in learning. ↩
-
Learning and Work Institute (2019). Evidence review: supporting progression from low pay. ↩
-
Learning and Work Institute (2019). Evidence review: supporting progression from low pay. ↩
-
Learning and Work Institute (2019). What Works to improve adult basic skills? ↩