BritainThinks: Complete transparency, complete simplicity
Updated 21 June 2021
1. Executive summary
Participants started this research from a point of very limited awareness about algorithmic transparency. Awareness of two of the central elements of this topic – algorithms and broader transparency in the public sector – is very low. There is almost no awareness of the use of algorithms in the public sector (except for a few participants who remember the use of an algorithm to award A-level results in 2020), and no spontaneous understanding of why transparency would be important in this context.
Once introduced to examples of potential public sector algorithm use participants became more engaged but still felt that, in their daily lives, they would be unlikely to make use of transparency information about the use of an algorithm in the public sector. Algorithmic transparency in the public sector is not a front-of-mind topic for participants. They expect to only be interested in transparency information if they were to personally have a problem or concern about the use of an algorithm, and struggle to foresee other circumstances when they would want to access this sort of information.
Trust in the use of algorithms in the public sector varied across different scenarios in which they might be used. The setting had a stronger influence on trust than prior knowledge of or trust in technology and was influenced by:
-
Perceptions of the potential risk created by deploying an algorithm in a use case – the likelihood of an unfavourable outcome occurring
-
Perceptions of the potential severity of impact should an unfavourable outcome occur
Despite their lack of personal interest in the information, participants felt that in principle all categories of information about algorithmic decision making should be made available to the public, both citizens and experts. For something to be meaningfully transparent, participants concluded, as much information as possible should be made accessible. Making all categories of information about an algorithm available somewhere is seen as the baseline for a transparency standard. Participants typically expect that this would be available on demand, most likely on a website. A central online repository is considered a sensible way of achieving this. They also expect that experts – such as journalists and researchers – would examine this information on their behalf and raise any potential concerns.
Making information available via transparency standards has the potential to slightly increase public understanding and trust around the use of algorithmic decision making in the public sector. However, this is ultimately a very low engagement topic and simply having information about the algorithm available is unlikely to have a substantial impact on public knowledge and trust as few members of the public would know to seek it out. More active communications (such as signs, letters, or local announcements) which notify the public about the use and purpose of algorithms would be more likely to have an impact and would signpost people to transparency information.
Taking more active steps to signpost people to information about the algorithm in use cases that might be perceived as higher risk and / or higher impact could do more to build understanding and trust, which is a key aim for the Centre for Data Ethics and Innovation (CDEI). Where the public are likely to feel there is more at stake, based on the perceived likelihood and severity of negative outcomes from the use of the algorithm, presenting transparency information before or at the point of interaction with the algorithm is felt to be more important. For use cases where participants are more accepting about the use of technology, and where they perceive a lower potential risk or impact, expectations around the availability of information about the algorithm are lower. Through the research processes, as participants explored the realities of transparency information some participants became more pragmatic about what information they would realistically engage with as individuals.
Most of all, participants want to know what the role of the algorithm will be, why it is being used, and how to get further information or raise a query. These are the categories of information participants would expect to see in active communications and form a first tier of information. While making all categories of information about the algorithm available somewhere is important in principle, this is less of a priority for active communication, forming a second tier or layer of information, as shown in the model below.
Figure 1: Tier one categories of information shown in overall order of importance for use cases that are high risk or impact when considering findings from across the research.
Information categories | Channels |
---|---|
1. Description of the algorithm 2. Purpose of the algorithm 3. How to access more information or ask a question (including offline options) |
Active, up-front communication that the algorithm is in use, to those affected. A more targeted and personalised approach. |
Figure 2: Tier two categories of information shown in overall order of importance across all use cases when considering findings from across the research.
Information categories | Channels |
---|---|
1. Description 2. Purpose 3. Contact 4. Data privacy (N.B. Data privacy was not tested as a category with participants but emerged during the research as a separate category of information from the data sets an algorithm is based on.) 5. Data sets 6. Human oversight 7. Risks 8. Impact 9. Commercial information and third parties 10. Technicalities |
Passively available information that can be accessed on demand, open to everyone. |
Reassurance about the ‘quality’ of public sector algorithms is also important. There is an assumption among participants that the public sector is unable to afford ‘the best’, and that this would extend to its use of algorithms. They expect that this would mean public sector algorithms are more likely to be inaccurate or ineffective. This is fuelled in part by a lack of understanding about what an algorithm is and the technology that sits behind it. Reassurance around the effectiveness of the algorithm within the information made available would be beneficial for improving trust.
Ensuring that transparency information about algorithm use in the public sector is accessible and understandable is a priority for participants. This refers both to communicating information in a digestible, easy to understand manner, as well as to making it possible for different groups to find the information, for example those without an internet connection.
2. Methodology
2.1 Purpose and aims of research
This research asked a diverse group of citizens to consider how the public sector can be meaningfully transparent about algorithmic decision-making.
Our core research questions were:
Which algorithmic transparency measures would be most effective to increase:
-
Public understanding about the use of algorithms in the public sector
-
Public trust in the use of algorithms in the public sector
Because of the low levels of awareness about the topic among the public we used a deliberative process. This involved providing information about algorithm use in the public sector, listening to the experiences of participants, and discussing their expectations for transparency. We worked collaboratively with participants to develop a prototype information standard that reflected their needs and expectations regarding transparency.
2.2 Sample
We recruited 36 members of the public, of whom 32 participants completed the research in full. We focused on having a diverse sample, rather than a representative sample, including people with different views on technology. To achieve this, participants were recruited to represent a mix of:
-
Age, gender, ethnicity, and socioeconomic group
-
Trust in institutions including an even spread of ‘High’ and ‘Low’ trust
-
Digital literacy- those who are highly familiar with technology and those less so, including their awareness of algorithms
12 of the respondents were recruited because they had experience of one of the three use cases (examples of algorithms in use by the public sector) that we focused on in the research. We recruited 4 participants who were aware that they had used an artificial intelligence (AI) parking system (e.g. where an automatic number plate recognition tool – ANPR – had been used), 4 who had participated in a video job interview and 4 who had interacted personally with the police or court system, all within the last six months. Participants with previous experience of each scenario were mixed with those without for all three use cases so they could hear each other’s views.
We recruited participants from a wide range of geographical locations including:
-
6 participants from Scotland
-
3 participants from Northern Ireland
-
4 from Wales
The remaining participants from England were recruited from a mixture of regions.
Due to the online nature of the research, these participants were also spread across the discussion groups.
All participants were paid an incentive on completion of each phase as a thank you for their time.
2.3 Method
In line with the COVID-19 restrictions, fieldwork was carried out remotely using secure, tested approaches with personal support for those with lower digital literacy to ensure they could participate fully. We used two formats to collect participant views: an online community and video discussion groups.
We set up an Online Community called “Tech in the Public Sector”, hosted on a web-based platform called ‘Incling’. The platform is simple, accessible and most participants used it with ease. Personal support was given when necessary. The platform featured questions and gathered responses in a range of formats, including video, images and text.
We moderated the platform, engaging with participants as they completed the tasks, probing them for further response where necessary and answering any questions and concerns participants had throughout the process. In addition to the online community, we conducted discussion groups (90 minutes) via Zoom in which groups of 6 participants discussed specific scenarios, areas of transparency and algorithms. The discussion sessions were moderated by BritainThinks researchers and attended by members of the CDEI team as observers.
The research involved three phases, shown in the timeline below.
Figure 3: Timeline
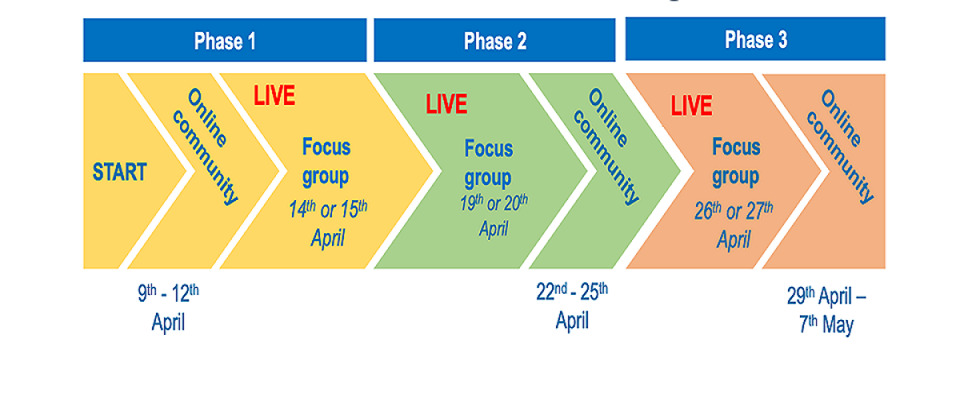
Phase 1
-
An introductory phase where participants were presented with one of the three algorithmic decision-making use cases to explore in their focus groups and on the online community, giving spontaneous, unprompted responses to transparency information.
-
The focus was on their initial understanding of and trust in algorithms in the public sector and the level of information they expected to be made available to people when in use.
Phase 2
-
This phase was more deliberative. In the online community, participants responded to stimuli that outlined different categories of transparency information and ways of presenting this information, assessing their importance and necessity. They also provided their own personal examples of complex information being presented well, discussing particular features with each other.
-
The focus group continued these conversations, discussing different transparency models, what information should be provided and how personal information should be stored.
Phase 3
-
This was an in-depth design phase where participants worked collaboratively with the moderators in focus groups to develop prototype transparency information about each of the three scenarios. They specified what information they would like to see regarding algorithm use in each case, how they would like it to be displayed and established a tier system for the information available.
-
The finished prototypes were then posted on the online community, with each participant reviewing and commenting on a prototype for each scenario.
Figure 4: An example final prototype describing algorithm use in policing.
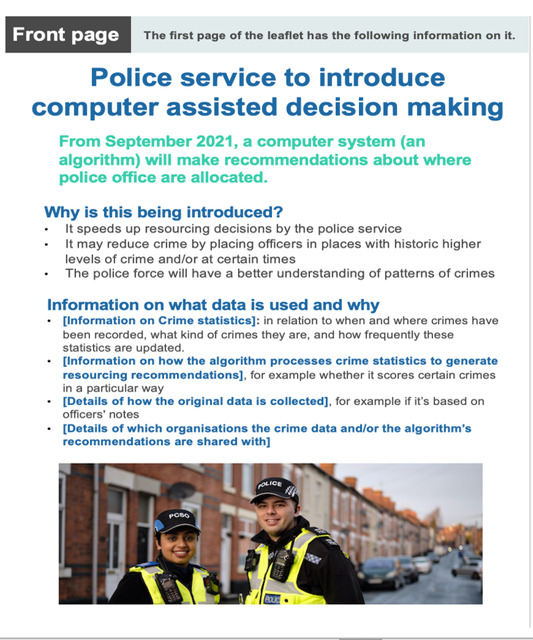
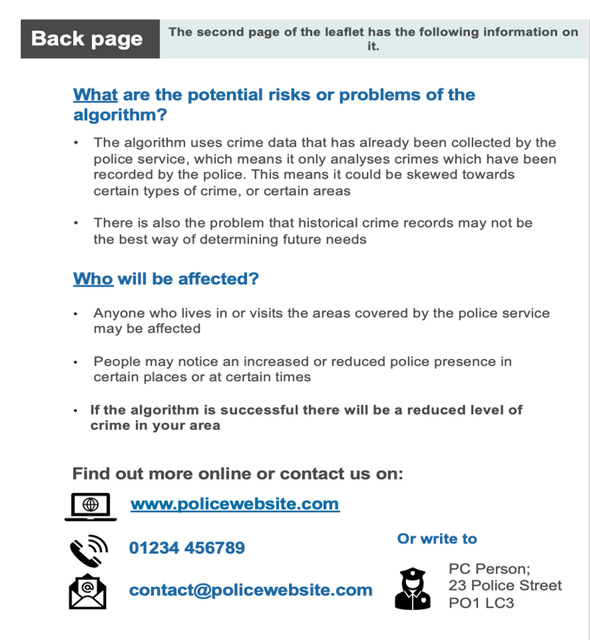
2.4 Timings
Our fieldwork began 9th April and ended 7th May when the final online community activities were taken down. During this time, Covid-19 government guidelines were in place meaning the focus groups were unable to take place in person and instead were conducted via Zoom. During the research, there were no major news stories regarding algorithmic or other data transparency issues raised by participants, however there were a number of news stories about transparency of government procurement decisions, which were referenced by some participants.
2.5 Data collection and analysis
Discussion groups were recorded, and detailed notes taken. Additionally, all responses to the exercises from the online community were exported from the platform. The findings of this report are based on this collated data, which was analysed by the research team.
Where possible, quantitative data has been exported from the online community and inserted throughout the report often in the form of charts. It should be noted that whilst this helps to provide a general impression of levels of trust and expectations for transparency of participants, the low sample size means findings are not statistically significant and care should be taken in generalising.
Quotes are used throughout this report, with comments made in the online community (and therefore in an individual setting) provided with the demographics of the participant. Demographics are not provided for quotes from discussion sessions which were in a group setting.
Within the report there are also three case studies which explore how the attitudes of individual participants changed across the research. These were created by comparing a participant’s individual responses in the online community, along with their focus group contributions, across the research period.
2.6 Participant feedback
Despite low levels of awareness and understanding of the subject matter at the start of the research project, when we asked participants in the final stage how far they felt they understood the content of the research on a scale of 0-10 (0 being no understanding, 10 fully understood) the average score was 7.9, suggesting that there was fairly high confidence about the purpose of the research by the end.
When we asked participants to what extent they understood the purpose of this research from 0-10 (0 being no understanding,10 being fully understood) they answered positively, again with an average score of 7.9. Participants frequently commented that they thought it was important research like this took place, and citizens were made aware of how public services were changing, even if they had been initially disinterested or completely unaware.
I would say that, even though I would not be particularly interested in how a particular algorithm worked, the fullest information must be readily available for people to look at if they want to, especially if they think they have been unfairly dealt with.
Female, 65+, Online community
3. Starting points: attitudes to algorithms and transparency
3.1 Summary
Most participants had low spontaneous understanding of the concept of algorithmic decision making, and low awareness of examples of it in practice. When given a description of what algorithmic decision-making is, commercial and low risk examples from everyday life are most front of mind, but there is little to no awareness of algorithm use in the public sector.
When thinking about transparency in the public sector, participants see this as important and equate more transparency with higher levels of trust in the government. However, this is a low salience topic for participants and they struggle to spontaneously identify examples of public sector transparency, whether good or bad. Without examples of public sector algorithmic decision making, participants are unclear about how this relates to the need for transparency.
However, with prompting of specific examples of public sector algorithmic decision making, there is wide acknowledgement that it is important for the public sector to make information accessible at the point of an individual encountering an algorithmic decision-making system. They prioritise information which flags that an algorithm is being used and why. Although ideally participants want all information to be accessible somewhere (complete transparency), they expect that they would only want or need to access this information if they encounter a problem in the scenario where the algorithm is being used.
3.2 Algorithms: awareness and understanding
Before being introduced to the term ‘algorithm’, we asked participants about their awareness of existing ‘computer assisted decision-making’ systems. This is not a top-of-mind topic, and not something participants could spontaneously apply to their daily lives. Similarly, when initially introduced to the term algorithm’, many participants had not heard of it before and were unsure exactly what it referred to and meant. Only a small number associate this term with decision making assisted by technology and based on data input.
Participants were then provided with the following definition: ‘algorithmic decision making, machine learning and artificial intelligence are all types of computer-assisted decision-making. This is where tasks are automated by computers, either to make suggestions based on past behaviour, to carry out simple and repetitive tasks or to analyse very big and complex data’. With this prompting, participants identify some examples in their own lives. However, it is worth noting that while some participants were able to identify commercial and low-risk examples for algorithmic decision-making, other participants gave examples where there is no algorithmic decision making (e.g. supermarket self-check-outs). This reflects participants’ overall limited understanding of the term, and suggests it may be used as a proxy for modern technology in general.
Examples given by participants include:
-
Algorithmic recommendations in their buying/viewing/search histories (e.g. Netflix and Amazon)
-
Text recognition and predictive text, responses, auto-completes
-
Self-checkouts
-
Credit card and other financial applications
-
Driverless vehicles
-
Robotics in manufacturing
One of my favourite examples [that] makes my life happier currently while in lockdown is Netflix, they use AI & algorithms to decide from my past watching history what I will enjoy next.
Female, 45-54, online community
Outside their own day-to-day experiences, some participants also recall having heard or read about computer assisted decision making systems being used in the healthcare sector (e.g. vaccine development) and the financial sector (e.g. stock markets and credit scores).
There is very little to no awareness of computer assisted decision making systems being used in the public sector. A very small number of participants spontaneously mention the A-level algorithm used by the Department for Education to give A-level results in 2020, and raise concerns about the use of an algorithm in this particular scenario. However, after discussing this example, participants remain open to algorithm use in other instances.
It’s that word algorithm which just makes me think back to last year and thousands of stressed-out students receiving inaccurate/unjustifiable grades based on algorithmic decision making as any algorithm can only be as good as the data that is inputted - millions of terabytes in even the simplest of human-human interactions.
Male, 35-44, online community
Please see Section 8, Appendix 1 for more information about participants’ spontaneous attitudes to algorithmic decision-making, including attitudes towards the use of algorithms in general, attitudes towards algorithms in different settings and attitudes towards algorithms in the public sector.
3.3 Transparency: initial views and awareness
Transparency in the public sector
Spontaneously, participants do not have strong top-of-mind associations with the concept of transparency in the public sector. There is some confusion about what both transparency and the public sector refer to; these are not terms or concepts many people regularly engage with in their daily lives.
However, once explained, the notion of transparency in the public sector and in public sector decision making is seen as an important and fundamental component of trust in government. Participants feel transparency is necessary to provide evidence and reassurance that the government is working in the best interest of the public. This is especially true for circumstances and decisions which are either likely to have a significant direct impact on individuals or wide ranging/ high stakes impacts on society as a whole.
Figure 5: Participants’ responses to a question across all Phase 2 focus groups: “What three words come to mind when you think about transparency in the public sector?”.
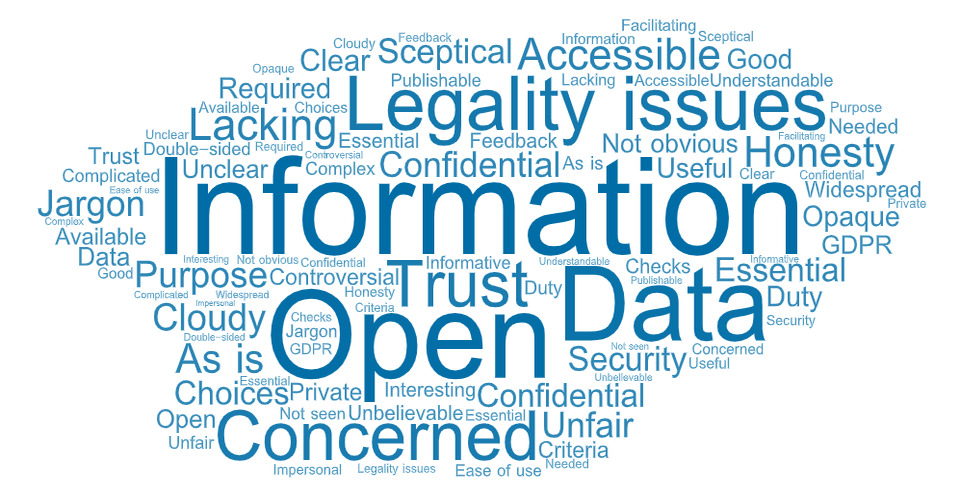
I think that people being able to…openly see what taxes are spent on is essential to trust the government. It’s currently lacking because there’s been a scandal around PPE contracts, people don’t have the full trust in what’s being spent.
Focus group participant
I think it’s important because you don’t read everything but it’s important to be there if you do need to find it, so if something happens you have the information there so you can see if you have the right to complain or not.
Focus group participant
The sense among participants that transparency in the public sector is important and to be encouraged sits in tension with the fact that some participants also associate public sector information with jargon and complex information that is hard to understand. Some participants feel that organisations in the public sector deliberately make it difficult to find or understand the decisions that they make. On the other hand, some suggest that there may be limits to the degree of transparency possible and feel that ‘too much’ transparency information may confuse the public and ultimately be unhelpful.
I think a lot of it is just jumbled with jargon, it’s not easy for people to understand. People have differing levels of experience and sometimes it’s done on purpose.
Focus group participant
If you put all the info out there not only, are you going to confuse people, the general population aren’t going to understand. I do think things are withheld. Overall, I don’t think they are hiding too much. I do think there is a reason for it.
Focus group participant
Transparency about public sector use of algorithmic decision making
Given the lack of awareness about algorithm use, participants do not have spontaneous expectations about what level of transparency is appropriate. In particular they find it difficult to identify the consequences of a lack of transparency, and so find it hard to form a view.
However, when presented with the three use case examples of algorithmic decision making in the public sector (including parking, recruitment, and policing - see section 4 for more on the use cases), and having detailed discussions, participants formed strong views about the level, categories and format of information that should be made available (more detail is provided on this in the following sections).
Ultimately, participants feel that being provided with transparency information will help to increase both public understanding and trust in public sector algorithmic decision making. At the same time, it is important to note that while participants feel it is important to be provided with this information, they only expect to need or want to access this information if they encounter a problem in the situation where the algorithm is being used.
During the research, we also asked participants about the relative importance of individual citizens and experts having access to information, and preferences for the level of detail the information would have. Overall, in instances where participants are directly affected, participants prioritise making simple transparency information available for individual citizens over journalists and experts (see fig. 6). In circumstances where the outcome of an algorithmic decision making has a direct impact on them, participants feel it is important and ‘their right’ to have access to information about an algorithm. Further, given the perception that too much detail would be overwhelming and hard to navigate or understand, there is also a preference for this information to be simple rather than detailed technical information.
However, among a small number of participants there is also an expectation and a desire that experts, journalists and civil society organisations like charities have access to wider, more detailed information about algorithms used by the public sector so that they are able to scrutinise and examine the impacts of new technology. There is a sense that these experts will go through the details on behalf of the public and would raise any concerns that might impact citizens.
This became even more important for participants in later research sessions where we asked people to design information standards. During this process, when confronted with realities of transparency information specific to use cases, some participants became more pragmatic about what information they would realistically engage with as individuals. Given some participants were unlikely to engage with the information, these participants began to feel it was more important that experts had access to this information than individual citizens.
Figure 6: Table showing different types of information provision regarding algorithmic decision-making in the public sector, ranked by the number of participants selecting each as ‘most important’.
Phase 2 Online Community Q1.3. ‘Based on the discussions so far, what do you think is most important for algorithmic decision making in the public sector?’ |
Percentage that selected each option. Base: All participants who responded in the online community (n=32). |
---|---|
That individual citizens have access to simple information about decisions that affect them directly | 38% |
That individual citizens have access to detailed technical information about decisions that affect them directly | 31% |
That individual citizens have access to simple information about algorithms in use more generally (including those that don’t affect them personally) | 16% |
That experts, journalists and civil society organisations like charities, etc have access to detailed information about algorithms in use | 9% |
That individual citizens have access to detailed technical information about algorithms in use more generally (including those that don’t affect them personally) | 6% |
If the info isn’t being scrutinized by an independent party, then an untrained eye will just assume it’s right… [it’s important] the government should have someone there challenging them [with information].
Focus group participant
I personally wouldn’t [look at information on the algorithm]. I suppose it would depend on what the algorithm is and if it will make a difference on my everyday life, I would like to see it in that case.
Focus group participant
4. Expectations for transparency
4.1 The tension between total transparency and simplicity
Unknown unknowns: initial desire for high levels of transparency
As participants start to build their knowledge of algorithmic decision making and its uses, they move from low engagement and interest, to a desire for detailed information. This is a common reaction: as we become aware of a topic we realise how much more there is to learn. Once introduced to example use cases (policing, recruitment and parking in this case) participants are keen to gain more knowledge about the use of algorithms in the public sector and expect that other members of the public would feel the same.
This sentiment comes through most strongly in the higher risk, higher impact use cases: recruitment and policing. In particular, participants spontaneously express a desire for:
-
Evidence from pilot studies or previous uses to illustrate the effectiveness of algorithmic decision making, including specific locations, contexts and consequences.
-
Details of when and where humans would be involved in the process if an algorithmic decision-making system is being used, especially whether they would have the option to contact somebody if they want to know more about the algorithm or to challenge a decision.
-
In addition, participants welcome information on what personal data is used and how it is stored across all use cases, consistent with a generally high level of awareness of ‘data protection’.
Participants also felt strongly at this initial stage that anyone who was affected by use of an algorithmic system should be made aware of it, to be told explicitly that an algorithm is being used so they can understand what this means for them.
Maybe there should be some statistics or examples of how it [the algorithm] is being used and a pilot study to show how it’s working. If you put it out and it doesn’t work, there is going to be backlash. Maybe they should include some views of the locals in the areas that it’s being used in – they need to provide evidence that it is working.
Focus group participant
This initial desire for more information translates into an expectation that all transparency information for a particular use case should be made available somewhere. This view was held consistently throughout the research by many participants who see transparency as making as much information accessible as possible. The exception to this is where there is concern about possible risks associated with publicly sharing some types of information (e.g. the data used by the algorithm in the policing use case), and in these instances participants expect less transparency information to be available.
You definitely need to know, it’s your personal information. I always read stuff especially if it’s to do with my personal information. You need to know why they are taking it and if they are going to keep it and how long. I think they should make all of the information available if somebody wants it.
Focus group participant
Known unknowns: increasing focus on the importance of simplicity
As they move through the research, participants consider how they would encounter this information in their day-to-day life, and also become more comfortable with the idea of an algorithm being used in the public sector. As this happens, participants become more selective about the volume of information that they expect to see (although with the continuing expectation that “all” of the information would be available somewhere). This resulted in a common desire for transparency information – whether basic or more detailed - to be presented clearly and simply.
In general.. [it needs to be] explained clearly and concisely - it’s a lot of stuff that you might not even pay attention to, but there needs to be a brief going over everything and just bullet-pointing the most important things. Especially for people not able to process information as easily as others, it can all be overwhelming. A lot of people aren’t really interested but you do have a right to know what’s going on. There is a risk to giving too much information, but you have to find that balance to what we need to know and what we might be better off not knowing.
Focus group participant
Resolution: Two tiers of transparency information
In phase three of the research, where participants worked together to design a prototype information format, this tension between transparency and simplicity was resolved by allocating information categories to different tiers. Participants expect the information in tier one to be immediately available at the point of, or in advance of, interacting with the algorithm, while they expect to have easy access to the information in tier two if they choose to seek it out.
Figure 7: Overview of the two tiers of transparency information.
Tier one | Tier two |
---|---|
A summary of what the algorithm does, and where to get more information Provided proactively |
All categories of information available Easy to find but not shared proactively |
Tier two information is generally what is expected as a bare minimum across use cases; at the very least, it is felt that individuals should be able to access all of the transparency information if they would like or need to. NB: A few participants expect to see information about how personal data is used in tier 1 for the parking use case, while some feel information about data should be held back in case criminals take advantage of this information.
I think they’re [transparency categories] all important. If you want it, this will give you the info you need, a link will give you an option if you have questions about the process.
Focus group participant
The two-tiered approach balances participants’ expectation that all transparency information is available to access on demand, whilst also ensuring that transparency information shared at the point of interacting with the algorithm is simple, clear, concise and unlikely to overwhelm individuals.
Do people need to know all the detail? Just have a basic leaflet and then make the detail available online clearly and without any jargon!
Focus group participant
4.2 The importance of context
During the research we presented participants with three different use cases that involve public sector algorithmic decision making to understand overall trust, as well as expectations around public sector transparency. We found that the different use cases had a significant influence on the degree to which participants feel comfortable and trust an algorithm. This in turn impacts the level of transparency information they feel is appropriate in each instance.
Figure 8: An overview of the three use cases tested with participants.
Recruitment | A public sector organisation is using an algorithmic tool to identify the most promising candidates to interview based on their applications. The system transcribes a video interview and scores it against pre-determined criteria. |
---|---|
Policing | The local police service is using an algorithmic decision making system to help decide when and where to allocate its officers. The system assesses historic crime data to make predictions about where and when officers are most likely to be needed. |
Parking | The local car park is using an algorithmic decision making system to notify the traffic warden to check your vehicle if you do not have a valid local parking permit or have not paid for parking online. An automated number plate recognition tool reads the car number plate and it is checked against a register. |
We found recruitment to be the most divisive use case, with concerns about a technologically- rather than human-driven application process translating to discomfort about the use of an algorithm. Conversely, participants’ low emotional engagement with and relatively high levels of familiarity with the use of technological solutions in car parking means they are more accepting of an algorithm in this instance.
There are two main factors that seem to influence how participants view the use cases and their level of comfort and trust in an algorithm being used:
-
Risk – the perceived likelihood that the scenario will lead to an unfavourable outcome for them as an individual or society more broadly. This is typically driven by the degree to which participants trust the efficacy of an algorithm to make a decision in each scenario. For example, many were sceptical about the ability of an algorithm to make correct recruitment decisions.
-
Impact – the severity of impact an unfavourable outcome would have directly on them as an individual or society more broadly.
Figure 9: Chart showing perceived impact and perceived risk of each of the use cases.
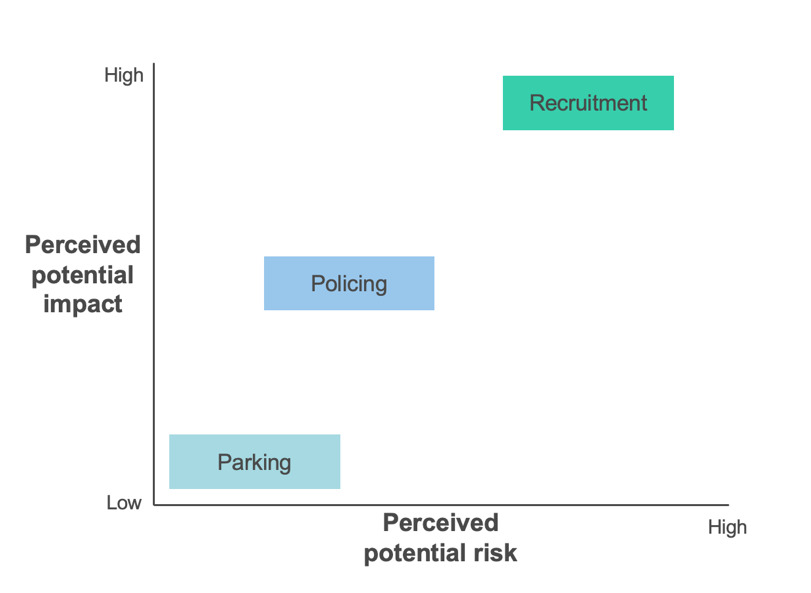
The degree of perceived potential impact and perceived potential risk influences how far participants trust an algorithm to make decisions in each use case, what transparency information they want to be provided, and how they want this to be delivered.
For lower potential risk and lower potential impact use cases, passively available transparency information – in other words, information that individuals can seek out if they want to – is acceptable on its own. This could, for example, be information available on a website. It is also more acceptable for passive information to be held as part of a centralised system, rather than being targeted to those affected.
For higher potential risk and higher potential impact use cases there is a desire not just for information to be passively available and accessible if individuals are interested to know more about the algorithm, but also for the active communication of basic information upfront to notify people that the algorithm is being used and to what end. As part of this, it is felt that information should be more targeted and personalised. For some use cases expectations around transparency information are very high (for example, for the policing use case, some expect to see door drop campaigns announcing the use of algorithms).
4.3 Use case deep-dives
Recruitment
This scenario is seen as having both high potential impact and high potential risk. There is less confidence in an algorithm being able to perform this task than a person, and therefore limited trust in an algorithm being used in this scenario.
Initial views
Figure 10: The recruitment use case with algorithmic decision-making information shown to participants.
Step 1 | You’re looking for a job online and decide to apply for a position within the public sector. |
---|---|
Step 2 | You’re asked to record a video of yourself answering a series of interview questions. |
Step 3 | Once you have answered the questions, you are asked to submit your video recording online. |
Step 4 | You submit your video recording and are notified that your application has been received. |
Step 5 | You wait for an email to let you know whether you have been invited to a face-to-face interview. |
Step 6 | You receive an email informing you if you have made it through to the next stage of the interview process. In the email, the organisation explains that they have used a computer assisted decision making system to identify the most promising candidates to invite for an interview. |
Participants have mixed views when shown the initial recruitment use case. Those with previous experience of an online job interview also report mixed experiences, and while they were able to complete the process and found it straightforward, some felt that the lack of face-to-face human interaction was unhelpful for rapport and easing nerves.
Recruitment cannot be automated there are too many variables to consider when choosing the perfect candidate.
Female, 45-54, Online community
Some participants expect that this type of process would make it more efficient and easier for some people to apply for jobs, and the ability to record a response in their own time would make some feel more comfortable. This is especially the case for those who are more familiar and comfortable using technology.
I am confident about doing videos and think it gives the employer a better idea of who you are as a person. It seems like an efficient way of doing things.
Female, 35-44, Online community
However, others feel that some people may not come across as well in a video response, and that their performance may suffer from the lack of human interaction. There is also some concern that people may be able to ‘cheat’ the system, for example by having someone else script the response for them, or having more time available to practise their response.
I think that all job interviews, even preliminary ones should be conducted in person. You can’t tell a lot about someone on video and you can’t get a feel for their personality, they may end up excluding some people that could be really good workers based on the fact they don’t video well!
Female, 18-24, Online community
Trust in algorithm use
The recruitment use case is where participants have most doubts about the overall effectiveness of an algorithm being used. Specifically, there is scepticism that a computer can assess candidates as effectively as a human. Some participants are suspicious about why a video is needed, as opposed to a written response, and express concerns about the purpose of a visual and the potential for this to lead to biased decisions. This use case is felt to have a high potential impact as a negative outcome would not only have ramifications for the individual applying for the job, but could also have broader societal impacts, for example if cultural biases were built into the algorithm preventing certain groups from getting employed.
The table below (fig. 11) summarises the perceived benefits and drawbacks of using an algorithm in the context of public sector recruitment.
Figure 11: Summary of perceived benefits and drawbacks of the recruitment use case.
Perceived benefits | Perceived drawbacks |
---|---|
* Increasing the efficiency of the process * Potential for reduced human bias and discrimination |
* Unsuitable applicants getting through the process through ‘cheating’ the system * Some suitable candidates might be missed if technology fails * Removes ‘human factor’ and potential for emotional connection and rapport to be built, which is felt to be important for a work environment * May exclude people from the process if they are not confident with technology * Potential inability to receive feedback on application |
When asked on a scale of 1-10 (where 1=not at all, 10= totally trust) how much they trust an algorithmic decision-making system to be used in this context, participants give an average score of 3.9. Broadly participants feel that it is important for humans to be involved in this process to remove potential technological issues and errors and maintain human element of recruitment.
Transparency information
The perceived high stakes of this use case, combined with a lack of familiarity and trust in technology being used this way, mean that participants feel it is especially important for transparency information to include how exactly the algorithm works. As part of this, participants are particularly keen understand what criteria are being used to identify the most promising candidates so that they understand the process fully and can ensure their response and application is well-prepared. Understanding the criteria being used by the algorithm is also seen to help applicants understand why they have or haven’t been successful, and a fundamental aspect of the feedback process.
Given doubts and concerns about algorithms being used in a process that is seen to require human and emotional intelligence, transparency information about the role of human involvement in this process is also felt to increase trust in this algorithm being used. As part of this, participants would like clarity on where exactly algorithmic decision making ‘begins and ends’, as well as understanding where human decision-making fits into this. Further, participants also want to see information provided about where they can go if they have any questions about the process or the outcome.
Due to the highly personal nature of the application and significant amount of personal information given, participants also feel it is important for transparency information to include reference to data security and privacy, so that applicants understand exactly how their information will be used and how long it will be stored for, before applying.
Overall, desire for transparency information in this use case is higher than other use cases tested. With the algorithm playing a significant role in determining whether they get through to the next stage of an employment process, this use case is felt to have higher potential risk and higher potential impact, especially on an individual level. Given the emotional stakes of this use case, and an unfamiliarity with technology being used in this way, participants feel candidates should be informed about the fact an algorithm is being used and to what effect at the point of deciding whether or not to apply, and proactively throughout the process.
The case study of Randeep below (Fig. 12) highlights some of the specific concerns some participants have about algorithmic decision making in the recruitment use case.
Figure 12: Case study of Randeep (male, 55-64, recruitment use case), a participant who remained sceptical.
Start point: sceptical | End point: sceptical |
---|---|
In the beginning Randeep has little trust in institutions and sees CADMS as a threat but he believes technology can be useful seeing it as instructive and informative. He thinks using algorithms in recruitment could be positive giving the company a speed advantage but that it is impersonal. Initially he does not trust algorithms to be used in this scenario, 2/10. Randeep believes the most important types of transparency information are knowing why the algorithm is in use, how data is used, impact, and risks. |
He sees knowing information about how the algorithm works, the technicalities and third-party information as less important but is still concerned about how third parties could extract information and use it. He remains skeptical throughout the process with an even lower trust score of 1/10 in the final stage mainly due to his dislike and distrust in algorithms being used for this specific use. He is now very likely to look up more information about the algorithm upon knowing it is in use (5/5 likelihood). |
“Without human contact in these decisions [it] would not give you an exact profile. It would only cover the basic requirements. There are things computers are great for. This is not one of them” |
Policing
Overall, this scenario is seen as being low risk and medium impact. Participants generally feel comfortable with an algorithm being used in this use case, as they recognise the potential value in terms of efficiency, and it is felt to have less direct personal impact on them than other use cases. However, there is some concern that publicly available information about police decision-making processes may enable potential criminals using this information to their advantage. This was a strong concern for some participants, even when it was explained that this would be an unlikely outcome of the scenario proposed.
Figure 13: The policing use case with algorithmic decision-making information shown to participants.
Step 1 | You are online reading local news stories. |
---|---|
Step 2 | You see a local news story about a new way of allocating police in your area. |
Step 3 | You click on the article and it explains that your local police force is using a computer assisted decision making system to decide when and where to allocate its officers. At the end of the article there is a link to the local police force website for more information. |
Initial views
In this use case, participants are interested to find out more about how the way of allocating police had changed and the impact this could have on their local areas.
The key positives related to this use case are understood as the potential for increased crime prevention and reduced crime levels, while others are pleased to learn more about an issue impacting their local area.
More police officers will be better and the neighbourhood will feel safer.
Female, 25-34, Online community
Among participants there is also some concern around how a potential increase in police presence would be funded, and others wonder whether information about police allocation can be harmful to public safety, and lead potential criminals to use the information to their advantage.
Could it be abused by people by sending the police to different areas deliberately?
Male, 65+, Online community
Trust in algorithm use
Most participants believe that there are clear benefits to algorithmic decision making in this use case. The fact that this scenario feels more removed from participants’ daily lives, also increases acceptance of an algorithm being used, as participants feel that it is less likely to have a direct negative impact on them.
The table below (fig. 14) summarises the perceived benefits and drawbacks of using an algorithm in the context of policing.
Figure 14: Summary of perceived benefits and drawbacks of the policing use case.
Perceived benefits | Perceived drawbacks |
---|---|
* Fairer, data-based way of allocating resources * Saves time for police if they are allocated appropriately * Might lead to better safety for local residents |
* Errors, data inaccuracies or poor source data leading to certain areas being under-resourced * Good police resourcing requiring more than data, and needing to be informed by human knowledge, understanding and experience * Unforeseen consequences, such as the potential for criminals to trick the system by changing where they are active |
When asked on a scale of 1-10 (where 1=not at all, 10= totally trust) how much they trust an algorithmic decision making system to be used in this context, participants give an average trust score of 4.7. While participants feel positively about the potential for algorithmic decision making to increase the efficiency and the impact of police resourcing, there are some concerns about how effective this would be in practice, and potential ‘unforeseen consequences’ of under-resourced areas and people tricking the system.
Transparency information
Given the perception that good police resourcing should be informed by human experience, there is some desire for transparency information to provide reassurance on the human input and support going into this decision making. Participants also want to know that the algorithm is working appropriately and is being checked.
However, in this use case participants feel strongly that any transparency information should not include the actual data which the algorithm uses, as it is felt that potential criminals could use this information to their advantage and to the detriment of public safety. In fact, some participants are concerned that any degree of transparency information may inadvertently lead to the system being ‘tricked’ and therefore also want reassurance that any and all transparency information has been deemed ‘safe’ to share more widely by experts. This was a particular concern for one participant in Northern Ireland, who felt that information about policing needed to be secure.
Overall, similar to other use cases, participants do not expect to actively search for or access transparency information on this use case themselves unless they have personally had a negative experience directly related to police resourcing. However, given the potential for any errors in police resource allocation to have a significant negative impact on wider public safety, participants express a desire to be actively notified upfront that ‘policing is changing’ e.g. via public information campaigns. This also reflects the fact that among participants, there is a strong sense that algorithms being used in this way is new, and a significant development.
The case study of Peter below highlights how becoming more informed about public sector algorithmic decision making, in this case when used in policing, can increase trust in algorithms being used.
Figure 15: Case study of Peter (male, 65+, policing use case), a participant who became more trusting.
Start point: sceptical | End point: trusting |
---|---|
At the beginning Peter views technology as a threat and identifies no positives to using an algorithm, he focuses on reasons not to use the algorithm. He consistently says proof the algorithm works is needed to make him trust it and is initially sceptical, 4/10. Peter believes the most important types of transparency information are knowing how the algorithm works, its impact, why it’s in use and risks. |
However, as he becomes more informed his trust increases in use of algorithms in policing, 7/10. He sees the technical details as less important to display, as well as how the data is used and commercial information. He believes information in the prototypes is clearly presented but would still like to have feedback on the success of the algorithm later down the line. With his improved confidence and trust he is very likely to look up more information about the algorithm knowing it is in use, 5/5 likelihood. |
“At the beginning I felt a bit overwhelmed by the fact that most people knew more than me on the subject but once into it, all of it became much clearer and my confidence grew.” |
Parking
This scenario is seen by all participants as being both low risk and low impact. Participants generally have high levels of confidence in an algorithm to be able to perform this task and are less concerned about the impact of a negative outcome for them.
Figure 16: The parking use case with algorithmic decision-making information shown to participants.
Step 1 | You park your vehicle in a car park. |
---|---|
Step 2 | There is a sign instructing you to pay for your parking space online if you do not have a valid local parking permit or have not paid for parking at a ticket machine. |
Step 3 | A camera at the entrance reads the number plate and a computer system then checks to see if the vehicle has a valid permit or online parking payment. |
Step 4 | If the system doesn’t identify a permit, a traffic warden will visit the vehicle to check for a paper ticket. |
Step 5 | The traffic warden will issue a ticket if they cannot see a paper ticket in the windscreen of the vehicle. You see a notice that states that a computer assisted decision making system is used to ensure each vehicle has a valid local parking permit or online parking payment. Drivers have the option to scan a Quick Response code (QR code) in the car park (at step 2) to find out more. |
Initial views
When shown an outline of the initial parking use case, without explicit information regarding the use of algorithm (see Figure 16), participants are broadly happy and comfortable with this scenario. There is familiarity with ANPR technology, and several have used this type of system before and had positive experiences.
Participants associate a range of benefits with this scenario, including it being an easy, quick and efficient way of paying for parking, the ability to have evidence of online payment, and facilitating fairness by ensuring everyone pays for parking.
I do actually prefer this method as years ago you would have to make sure you had change for the machine and often I would not have the right money…So having the camera at the entrance reading your number plate and you ringing a number to pay for your parking I feel is a good idea as you have proof that the payment has been taken from your debit card in case you do get a ticket.
Female, 55-64, Online community
Key issues associated with this scenario include having issues with phone and/or internet signal, not having a credit card, and ensuring the signage is clear enough for everyone to understand it.
That’s all well and good, assuming you have an internet enabled phone to pay online.
Female, 55-64, Online community
Trust in algorithm use
On the whole, participants are comfortable with the use of algorithmic decision making in this context. Ultimately, participants do not see the use of an algorithm as likely to have a significant impact on them. An unfavourable outcome would mean getting a ticket, which in participants’ view is ‘how parking has always worked and always will.’
The table below (Fig. 17) summarises the perceived benefits and drawbacks of using an algorithm in the context of parking.
Figure 17: Summary of perceived benefits and drawbacks of the policing use case.
Perceived benefits | Perceived drawbacks |
---|---|
* Ensures fairness by ensuring that everyone pays for parking * Increases the efficiency and ease of the traffic warden’s role * May improve the security of cars |
* Reliability and accuracy of the technology * Security and data breaches related to number plate scanning * Availability of humans to intervene or provide support in cases of issues or errors * Increase in unemployment related to a loss of demand for traffic wardens |
Overall, participants are positive about the use of an algorithmic decision making in the parking use case; when asked on a scale of 1-10 (where 1=not at all, 10= totally trust) how much they trust an algorithmic decision-making system to be used in this context, participants give an average score of 7.5. There is a general sense that in this parking scenario, the use of an algorithm is straightforward and efficient, and the most significant issue would be the reliability of the technology. Those who lacked trust were often sceptical about parking fees and ticketing generally, as much as about the algorithm.
I do feel that this is a process in which computer assisted decision making is relatively straight forward to apply and drives up efficiency and enables cost-savings which can be used to better purposes.
Male,35-44, Online community
Transparency information
Given the fact that this use case involves personal data, participants also feel that it is important for transparency information to include data security and privacy information. Participants generally want to know what data is being held, for how long and why. Participants often link this to awareness of General Data Protection Regulation, and a sense that they have a right to know how their data is handled.
[I’d like to have] confirmation that your information is kept private and that your details are not shared with any third parties.
Female, 55-64, Online community
As with other use cases, participants also feel that information about who they can contact in case they encounter any problems or want to challenge a parking ticket is particularly important, and gives them reassurance that help is at hand in case there are any technological issues.
However, overall, desire for transparency information in this use case is lower than other use cases tested. In general, participants feel strongly that it is their right to know an algorithm is being used and expect this to be explicitly stated at the car park entrance. Yet, beyond this, participants expect that people can seek out further information (including contact and data security and privacy information) if they want it, rather than expecting any active communications.
As long as it’s very clear that this is in operation, I would trust it.
Male, 18-24, Online community
The case study of Thomas below highlights some of the specific advantages participants recognise when algorithmic decision making is used in the parking use case.
4.4 Figure 18: Case study of Thomas (male, 35-44, parking use case), a participant who is informed about technology.
Start point: informed | End point: situationally trusting |
---|---|
Thomas enters the research with a good understanding of AI, spontaneously mentioning examples of machine learning and algorithm use. He thinks technology is exciting, and innovative but can be dangerous. He can see positives and negatives of using algorithms in parking believing it would make paying faster and more efficient but would make you reliant on having a charged mobile with signal. He is trusting of algorithm use, 7/10, but is clear that this is specific to this low-risk use case and in some situations it would be inappropriate. |
For Thomas, the most important types of transparency information are its impact, details of how data is used, and how the algorithm works. He is less concerned about technicalities, information on third parties and why the algorithm is in use. His opinion is informed by his desire to know how successful an algorithm is, his need to ensure it is not biased and that it doesn’t have disproportionate negative effects. He remains trusting 8/10 about using algorithms in parking but is clear it is situationally dependent. |
“There is a fine line that could very easily and rapidly see this computer aided decision process lead to one which is fully automated where the human element is removed entirely” |
5. Standards for transparency information
5.1 Categories of transparency information
In Phase 1 of the research, where participants were initially introduced to the three use cases (recruitment, policing and parking), they were also shown basic transparency information about how and why an algorithmic decision-making system is deployed in each setting. In Phases 2 and 3 of the research, participants were presented with a total of nine categories of transparency information, which were based on discussions with CDEI, the Cabinet Office, and a review of the transparency standards used internationally. Across the research, a description of the algorithm and its purpose remained the most important categories of transparency information.
The table below outlines participants’ prioritisation of the nine information categories during the Phase 2 online community. This prioritisation remained largely consistent when asked generally and when discussed in relation to each of the use cases in turn. The main exception to this is contact, which came out as a higher priority during the Phase 3 focus group discussions and prototype development. The other exception is data which, when interpreted as personal data, was considered a higher priority to include.
Figure 19: Overview of importance of transparency categories in relation to algorithmic decision making in the public sector Phase 2, Q1.1. “Please rank the following categories of information in order of how important you think they are when it comes to being transparent about algorithmic decision making in the public sector.’ Base: All participants who responded in the online community (n=33).
Order of importance | Category of information regarding transparency | Average weighted rank (where 9 points are assigned to position 1 and 1 point is assigned to position 9) |
---|---|---|
1st | Description: how does an algorithm work? | 7.21 |
2nd | Purpose: why is an algorithm used? | 7.18 |
3rd | Risks: what are the potential risks or problems with the algorithm? | 5.82 |
4th | Impact: who will be affected by the algorithm? | 5.7 |
5th | Data: what data is used and why? | 5.12 |
6th | Contact: how can I ask questions or raise concerns about the algorithm? | 4.42 |
7th | Human oversight: who is responsible for the algorithm? | 3.88 |
8th | Technicalities: what are the technical details of the algorithm? | 3.52 |
9th | Commercial information and third parties: who else is involved in the algorithm? | 2.15 |
While one of the lower ranked categories in the online community, in the focus groups contact emerged as one of the most important categories. In a two-tier system, details about where to get more information or ask questions is elevated and prioritised as tier one information, alongside description.
The main one for me is contact, I need to know who to contact if it goes wrong. I need to know who is responsible and their contact info so I can contact them by email or phone.
Focus group participant
Sharing information about risks is polarising among some participants and in some use cases due to a concern that it will cause additional and unnecessary anxieties among those already unsure about the use of an algorithm. In the focus groups this category was expected to appear in tier two but was prioritised below other types of information.
I would like to know more than just the descriptors but not the risks because your trust is lowered. You don’t want to know the risks because it’s going to happen anyway. I’d rather not know risks and just go with it. The risks would put me off as I am already anxious about it.
Focus group participant
In the focus groups, it became apparent that data is understood by some to refer to the use of their personal data and data privacy – participants expect this to be called out and is of higher importance to them than information on other data sources.
You just need to know where your information is going and who has that information. I think data is the most important for me [it is like] when you’re on Facebook… your information is being sorted and you don’t even know it.
Focus group participant
For some, there are concerns about sharing information on data when it comes to the policing use case particularly due to the fear of this information getting into the wrong hands.
You shouldn’t share too much data in case criminals get hold of it and start committing crimes in other areas or play the system.
Focus group participant
For the policing use case, some participants expect more information to be included in tier one. Commercial information is felt by some to be more important than other categories, and in some instances, participants feel this should be included along with tier one information. This is because the police are an institution that serves and protects the public and therefore making the public aware of other organisations that might be involved in delivering this service is felt to be important.
As policing impacts entire communities, some participants would also like to see information on risks and impact included in tier one. However, this should be at the expense of the information remaining simple and clear.
It’s important to know if it’s just the police that handle this information. I think it’s important to understand the impact for policing, as it impacts the community. They need to explain this so everybody can understand.
Focus group participant
5.2 Deep dive on the information categories in order of priority
For each category of information, participants were presented with the following details:
-
Heading
-
A question that explains the category
-
3-4 examples of specific types of information that could be included in that category
Breaking down the transparency information in this way is felt by participants to be clear and easy to digest. In particular, having the question that explains what each category of information covers is seen as a helpful aid to understanding.
Description
Figure 20: Stimulus used in Phase 2 focus groups.
How does the algorithm work? | * When the algorithm is used * How the algorithm works * How the result of the algorithm is used/interpreted |
---|
Consistently throughout the research, the description of how the algorithm works was seen as the most important piece of transparency information to be shared. Participants expect a description to be clear, simple and not overly technical, summarising the role of the algorithm rather than providing too detailed of an explanation. This category of information had high appeal when it was first presented in Phase 1, and this remained the case for the duration of the research process.
Participants expect that all three of the bullet points would be included within each of the three use cases. The first and second bullet points ‘when the algorithm is used’ and ‘how an algorithm works’ are felt to be particularly important, as participants expect that many individuals would have limited knowledge about what an algorithm is, and providing this information would help to address this. The information outlining ‘how the result of the algorithm is used/interpreted’ is felt to be particularly relevant for the higher risk and higher impact use cases (policing and recruitment).
Purpose
Figure 21: Stimulus used in Phase 2 focus groups.
Why is the algorithm used? | * Reasons why an algorithm is being used * Why algorithmic decision-making is better than human decision making * What non-algorithm alternatives have been considered |
---|
Similarly to the description outlining how an algorithm works, the information included within the purpose category is felt to provide further clarity about use of algorithms in the public sector. Participants often find it difficult to separate their feeling about whether an algorithm should be used in each use case, so information outlining ‘why an algorithm is being used’ is especially valuable.
The first two bullet points ‘reasons why an algorithm is being used’ and ‘why algorithmic decision making is better than human decision making’ are the most interesting and important. While the extra information is welcomed, the third bullet point is felt to be less relevant across all use cases.
Description and Purpose - I put these first as I think we should know exactly why this algorithm is being used. Having a basic understanding of why/how it’s used would benefit the person it’s being used on. I think it’s someone’s right to know why and how it’s being used.
Male, 25-34, Online community
Contact
Figure 22: Stimulus used in Phase 2 focus groups.
How can I ask questions or raise concerns about the algorithm? | * Individual responsible for use of the algorithm * Contact information of the responsible government organisation and a designated person responsible for the algorithm * Details on the appeal process for those affected by an algorithmic-assisted decision |
---|
Unlike description and purpose, this category of transparency information was not presented to participants until Phase 2 of the research. However, in Phase 1, participants spontaneously mentioned that the relevant contact details needed to be included in the transparency information.
The most important element of this information category is providing the public with clear details on how to get more information or get a query addressed. The last bullet point outlining ‘details on the appeal process’ is seen to be especially relevant to the parking and recruitment use cases. The first bullet point was felt to be less important for some.
You need to know who to contact if you have any concerns about it [the algorithm] – this needs to be available.
Focus group participant
Data
Figure 23: Stimulus used in Phase 2 focus groups.
What data is used and how? | * Data used in algorithm and sources of data * Data used to train algorithm (e.g. example data) * Information on data collection and sharing agreements and processes * Processes in place to ensure data quality |
---|
When discussing data participants typically think first of their personal data – what is being used and how it is being stored - which is considered to be more important than getting information on other data sets used by the algorithm. This is particularly true for participants who are more aware of General Data Protection Regulation (GDPR). This is most relevant to the parking and recruitment use cases where individuals have more direct interactions with the algorithm.
Particularly among those with a more advanced understanding of algorithms, knowing what data the algorithm is using is seen as very important, as this is seen as what will determine the effectiveness of the algorithm. However, these participants concede that not everyone will be interested in this information, and would expect it to fall within tier 2. For the policing use case, there is concern among some that too much information on data sources and data used to train the algorithm could lead to criminals ‘playing the system’.
I think there’s a danger that once people know the algorithm they can then game it…if I know the algorithm about police ending up in my street and I’m a criminal I know that this is the best time to commit a crime in my area because police are being sent elsewhere.
Focus group participant
Human Oversight
Figure 24: Stimulus used in Phase 2 focus groups.
Who is responsible for the algorithm? | * Who is responsible for the human oversight of the algorithm * Who is involved in development of algorithm and how * Who is reviewing and accountable for the algorithmic decision-making * Who intervenes if something goes wrong |
---|
This category of information resonates with participants, as they want reassurance that a human is also involved in the process in each use case. However, they are more interested in information about how and when humans would be involved in the process as opposed to the risk of human error. Overall, while relevant, this category of information is not felt to be as important as the others.
Would there be a human involved? When would they come in or would it be the algorithm throughout? This is important to know.
Focus group participant
Risks
Figure 25: Stimulus used in Phase 2 focus groups.
What are the potential risks or problems of the algorithm? | * The potential risks and bias associated with algorithm use * How any risks have been addressed/mitigated * Assessments in place to check and review the algorithm * Details on the process if any bias is found |
---|
This is the most polarising category of transparency information. Many participants welcome this extra level of transparency, especially when there is a lot at stake. For others, this is considered as being ‘too transparent’ leading to worries that sharing this information would lead to unnecessary anxieties and concerns about the use of algorithms in the public sector, which could inadvertently cause more work for public sector bodies as they have to manage this. This is mainly a concern in the higher risk and higher impact use cases where there is lower immediate acceptance of an algorithm being used. Ultimately, most feel that this is important information to make available within tier two.
I put risks as least important because any time you mention risks it will pre-emptively scare the reader because it’s putting the risks out there for all to see and causing some doubt in the decision where maybe there was none before.
Male, 25-34, Online community
The case study of Anita below highlights the worries that some have about including information about risks as part of algorithmic decision-making transparency information.
Figure 26: Case study of Anita (female, 25-34, policing use case), a participant who lost trust.
Start point: trusting | End point: sceptical |
---|---|
In the beginning Anita has a high level of trust in institutions and sees technology as an opportunity, describing it as intriguing and exciting. She is positive towards the use of algorithms in police allocation because it could increase police presence but announcing algorithms were in use would make her worry an incident has occurred. Her trust is initially high, 7/10 and her only concerns relate to practicalities of using technology. Anita believes the most important types of transparency information are, how the algorithm worked, why it was in use, its impact and contact information. |
She thinks details of commercial information, technicalities, oversight and data use are less of a priority and worries about displaying the risks. She believes too much information is off putting and that outlining possible risks could alarm the public and cause complaints. She became more sceptical towards the end of the research with her trust reducing to 5/10 in the same criminal justice scenario and is likely to look up more information about an algorithm like this in use (4/5 likelihood). |
“I think the risks will just cause upset and protest about algorithms. If I was to read this, I would wonder why there are risks to such an important change.” |
Impact
Figure 27: Stimulus used in Phase 2 focus groups.
Who will be affected by the algorithm? | * Groups affected by its use (directly and indirectly) * How the algorithmic system has impacted society *Impact reports for various demographic groups |
---|
Participants feel that information on impact is necessary for transparency. However, they are less interested in ‘impact reports for various demographic groups’, and more motivated by how the algorithm is directly impacting them as individuals.
I’d be interested to find out more to see how it would impact me, but I wouldn’t be interested in the technical details – just how it will work and why they are doing it.
Female, 45-54, Online community
Commercial information & third parties, and Technicalities
Figure 28: Stimulus used in Phase 2 focus groups.
Who else is involved with the algorithm? | * Third parties involved in the creation and running of the algorithmic system * Information on procurement details, including the cost and contract * Details on how open the procurement process was |
---|
Figure 29: Stimulus used in Phase 2 focus groups.
What are the technical details of the algorithm? | * Accuracy of algorithm / failure rates and cases * Frequency of reviews and checks * How long algorithm has been in use/how established it is |
---|
While participants feel details about commercial information and third parties and technicalities should be made available and accessible, they are less likely to be the main priority. Therefore, participants suggest that they should be available online if individuals are seeking extra information about the use of an algorithm in the public sector.
A few participants feel commercial information and third parties should be communicated upfront for the policing use case.
6. Information channels
6.1 Summary
Overall, participants want basic information about the use of the algorithmic decision-making system to be made immediately available when engaging with the information, or in advance, in tier 1 for all three use cases (refer to section 4) and would expect to be signposted to a website if they require additional information. However, many participants also feel this information should be provided in leaflets, the local newspaper, and on the radio to make it widely accessible to members of the public.
In terms of information design, accessibility for a wide range of people is a key consideration and impacts the sort of language and layout participants would expect to see. Crucially participants want to avoid jargon, to easily be able to find the information they are looking for, and for options to be offered to those who are not online (e.g. automated phone lines).
6.2 Active and passive information
In general participants default to the two tiers of information in relation to the preferred channels they expect for each use case (parking, policing, recruitment).
In tier 1 (across all use cases) participants expect active communication of basic information upfront to notify people that the algorithm is being used and to what end – this is either immediately at the point of interaction or in advance. For example, participants’ suggestions included signs before entering the car park (parking), door drops, or radio announcements, to notify people an algorithm is being used (policing), and a notification in the job description (recruitment).
In addition, for the use cases in which participants feel less comfortable with an algorithm being used (higher impact and higher risk) more personalised information is likely to be more effective than non-personalised information. For example, in the recruitment use-case, participants expect that they would receive a personalised letter informing them how algorithmic decision making was used to process their application.
For more detailed information (tier 2), participants express the need to be directed to a website and being provided the option to call an automated line (which will provide the same information) for those who are offline and do not have access to a computer or the internet.
6.3 Preferred channels for each use case
Recruitment
Overall, participants want to be notified that an algorithm is being used within the job application. Within the application, participants expect to be directed to the organisation’s website if they require more detailed information about the use of an algorithm to identify the most promising candidates (as well as the written content, participants express that an option should be provided to listen to short videos to ensure the information is accessible). To make the process more transparent, for those that continue with their application, there is an expectation for organisations to send the applicant an email with the outcome outlining how their application was assessed by the algorithmic tool.
Figure 30: Preferred channels to receive information that an algorithm is being used to identify the most promising candidates to invite for an interview.
1: Job application notifying that an algorithm will be used (tier one information) with a link for additional information if required. |
2: Company website with detailed information about the use of an algorithm to identify candidates provided in both written content and short videos (tier 2 information). |
3: Email from the organisation with information outlining how the applicant’s application was accessed by an algorithmic tool (tier 1 information). |
Policing Participants feel that a leaflet and radio would be the most suitable channels to receive basic information about algorithms being used to allocate police officers because it’s both accessible and personalised. In addition, to make it more accessible to a range of different audiences, participants recommend this information should also be announced on the radio. Within the leaflet, participants expect to be directed to their local police website, via a link, to access more detailed information.
Figure 31: Preferred channels to receive information explaining the local police service is using an algorithmic decision-making system to allocate police officers.
1: Leaflet and radio to be updated that an algorithm is being used to allocate police officers (tier one information) with a link for additional information. |
2: Local police website to obtain more detailed information about the allocation of police officers (tier 2 information). |
Parking
There was a widespread view that there should be a sign which clearly outlines that an algorithm is in use before people enter the car park. For additional information, participants propose that there should be a link and QR code on the sign, which directs individuals to their local council’s website. In addition, to ensure everybody has access to this information, participants also suggest including an automated number for members of the public to contact if they are offline or do not have access to the internet.
Figure 32: Preferred channels to receive information explaining the use of algorithmic decision-making systems in car parks.
1: Sign notifying people that an algorithm is being used before people enter the car park (tier 1 information) with a QR code or phone number for additional information. |
2: Local council’s website / automated line for more detailed information about the use of an algorithm (tier 2 information). |
6.4 Design principles
Participants highlighted a range of principles for the presentation of transparency information in any channel and format:
Language
Overall, participants expect the language that is included within the transparency information to be clear, concise and accessible (without the use of acronyms or jargon). Participants felt this was particularly important when they reflected on the fact that they originally had low understanding and awareness of algorithms at the beginning of the research process.
Information structure
To ensure that the content is user friendly, participants feel that both headlines and bullet points should be used to clearly group the content, both in paper form (leaflets/ local newspapers) and on websites. This is to ensure the information does not cause cognitive strain, especially because many members of the public are unfamiliar with what an algorithm is. In addition, drop down boxes and ‘hover overs’ that help to explain technical terms have high appeal to aid comprehension further.
Visuals
Although participants spontaneously mentioned using visuals and animations at the beginning of the research, this is not considered to be a priority at the end of the process and is therefore rarely mentioned in the design process in Phase 3. This is because simplicity is a key driver, and if the balance is correct between the headlines and the following content, visuals are not thought to be essential to aid understanding further.
Recognised symbol for algorithm use
Some participants can see huge potential for having a recognised symbol indicating when an algorithm is in use. While this will increase transparency in the public sector, participants also feel that this will lead to more awareness and familiarity about the use of algorithms in the public sector more generally.
Responses to international models
In the final phase of the research, participants were presented with two existing examples of algorithmic transparency information that are used in Helsinki and New York. Participants were asked to provide their views on the information and presentation style within both models.
The Helsinki model
Overall, the Helsinki model has high appeal amongst all participants. They are positive about the information being in a centralised register and assume that people would be able to locate this information easily. In addition, they appreciate the simple headlines and summaries that are provided on the home page, and value the option of being able to click on ‘read more’ if they require more detailed information.
Figure 33: Screenshot of Helsinki model. This can be found on the City of Helsinki AI Register website
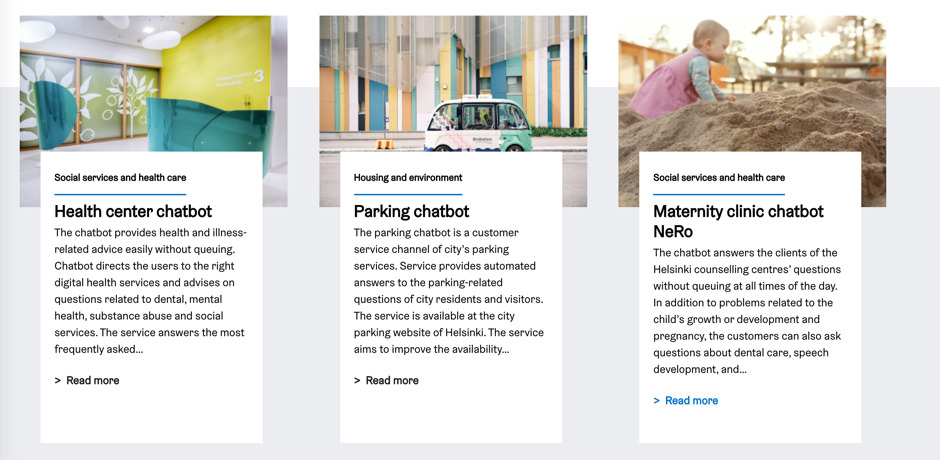
While the visuals are not spontaneously mentioned by most, those that notice them acknowledge that they capture the essence of the content well and contribute to an overall aesthetically pleasing and clear design. When exploring the additional information available, ‘contact information’ and ‘more detailed information on the system’ really stand out to participants as being important to cover.
Overall, this example is felt to be simple, user friendly and to have the right balance of information to increase transparency in Helsinki’s use of artificial intelligence. Participants express a desire for the public sector in the UK to have a similar centralised register for the more detailed content and feel that they as individuals and others would be able to engage with the information. The Helsinki example brings to life how participants expect and want tier two information to be made available.
If it’s laid out like this, it’s not too much, as you can pick out what you want to see as you can click through. It’s bright, it’s light and inviting – it definitely helps.
Focus group participant
The New York Model
Overall, this model is not particularly well received. On initial exposure to the New York Model, participants raise concerns about the length of the content and feel that it is not as user friendly as the first example. Participants like the clear headings, but without the filter for additional information (as in the Helsinki model), participants either feel this is the right level of information or too much.
Figure 34: Screenshot of New York model. This is a downloadable document from the NYC Algorithms Management and Policy Officer, available on the official website of the City of New York
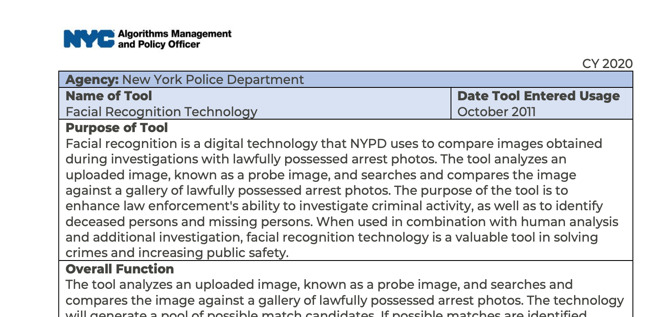
Most participants assume that this level of information is not targeted to the public, and is more likely to be suitable to academics or experts. In addition, a few participants raise concerns about the accessibility of the information because of its format and thought that some people would not be able to access it. There was some confusion about whether this would need to be downloaded from a website or whether it would open in a web browser.
I don’t think this is good, it’s a document academics would go to as it’s a more technical resource. Would the general public know all of the jargon being used?
Focus group participant
7. Conclusion
In summary, public understanding of and trust in the use of algorithms in the public sector is most likely to be improved through active communications that notify the public about where algorithmic decision-making is being deployed, with full information available on demand.
In terms of understanding, at present awareness of the use of algorithms in the public sector is low, as is awareness of transparency in the public sector. The low engagement nature of these topics means that people are unlikely to seek out information about the use of algorithms. Signposting people to the fact that there is information available if they are interested has the potential to increase engagement, and therefore understanding about the role algorithms play in the public sector. Presenting all information in a simple, clear and easily digestible way is also key for aiding understanding.
When it comes to trust in the use of an algorithm, the scenario in which it is being used is a highly influential factor. In scenarios where trust is lower (typically those which are perceived to carry a greater risk of a negative outcome, and where the potential impact of a negative outcome is felt to be higher) activities that build trust are more important. Therefore, active communications around the role of the algorithm, its purpose and how to access further information are most important in these instances.
Importantly, active communications need to be supported by a baseline of having all available categories of information about the algorithm accessible to the public somewhere in an on-demand way. Although participants do not generally expect to make use of this information, they feel that in principle it should be available to them, and that without this the public sector could not claim to be truly transparent around its use of algorithms. They also expect that experts may access this information on their behalf, raising any concerns which may be relevant to citizens.
In this report, we have summarised the need for different types of information in different scenarios or at different points in the journey of engaging with an algorithm through the two-tier framework. This model highlights the difference between the information about an algorithm that is expected to be passively available on-demand (tier two), and the types of information which, if actively communicated, would be most effective and increasing trust and understanding (tier one).
Our recommendation to the public sector would be to flex this two-tier model depending on the specific scenario in which an algorithm is being used. For scenarios where the public are likely to perceive higher potential risk and impact, a two-tier approach with full transparency information available alongside signposting is likely to be most effective for building trust and understanding. For scenarios which are felt to be lower stakes, just having tier two information available is likely to be sufficient.
8. Appendix 1: Additional detail on associations with and attitudes to algorithms
Associations with algorithmic decision making
When first asked about their associations with ‘computer assisted decision making’, participants have mixed views. While there is some positivity towards computer assisted decision making, participants also have a number of concerns.
Figure 35: Participants’ responses to: “What three words come to mind when you hear the word computer assisted decision making?” Question asked in Phase 1 focus group, responses across all focus groups.
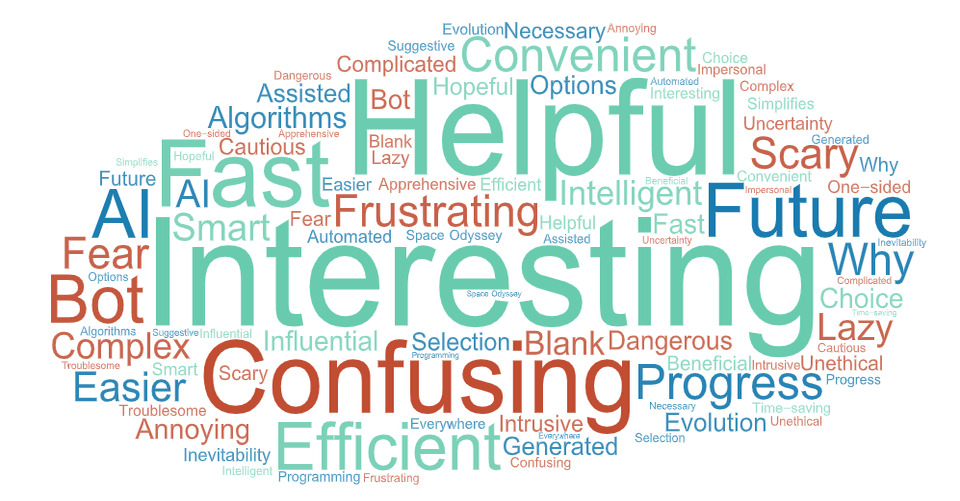
Where ‘computer assisted decision-making’ is viewed positively, it is associated with a sense of efficiency, optimism, and progress. For these participants computer decision making is seen as:
-
An important form of technology that can save resources, time and costs
-
An essential part of society and daily lives, and an inevitable and undeniable aspect of the future
You can see it really well with technology right now. It has almost become difficult to live without your phone. You can do a lot of things and it saves a lot of time. Right now you can chat to any part of the world with anyone in a short amount of time as if they are next door. Technology has made the world smarter and more efficient.
Focus group participant
Others, while able to recognise the benefits of computer assisted decision making, are more apprehensive about it. This is mainly driven by:
- Confusion and a lack of understanding around how algorithms work, and their impact
Sometimes it can be scary to people that don’t know much about assisted decision making. It can be scary because you don’t know if it will choose the right things, you just don’t know.
Focus group participant
-
Negative, frustrating, and invasive experiences with technological systems and processes in general
-
A sense that computer assisted decision making is not able to adequately account for ‘human’, emotional, and personal characteristics and circumstances
This I feel makes things harder for humans now for example buying a house with a mortgage an algorithm makes the decision for you whether you can have it or not and it takes away the personal aspect and I feel it’s too regimented!
Female, 18-25, online community
-
A fear that computer decision making may result in laziness or an inability for people to make decisions
-
A concern that computer assisted decision may lead to unemployment
I worry what it means for actual jobs and people, I don’t understand how it’s going to help people with jobs. You’re kind of wiping out loads of jobs and taking the human out of things.
Focus group participant
Attitudes towards the use of algorithms in general
The mix of views on algorithmic decision-making systems is reflected in the fact that the majority (60%) of participants believe that computer assisted decision making systems are as much of an opportunity as a threat (see Figure 36).
Figure 36: Overview of those who see computer assisted decision making systems as more of an opportunity or threat to our economy, security and society.
Online Community, Phase 1, Activity 1.8 ‘Do you see computer assisted decision making systems as more of an opportunity or a threat to our economy, security and society?’ |
Percentage of participants that selected each option. Base: Online community participants (n=35). |
---|---|
More of an opportunity than a threat | 26% |
As much of an opportunity as a threat | 60% |
More of a threat than an opportunity | 14% |
The demographic profiles of those who see computer assisted decision making as an opportunity are varied. However, of the 5 participants who see computer assisted decision making systems as more of a threat, 4 are within the 55-64 age range. Although the numbers are small and should be treated with caution, this provides some indication that older participants are more likely to see computer assisted decision making as a threat.
I think it’s more of an opportunity, it takes out human error, computers can make a decision in half a second. I have been to Singapore where everything is run on a system and it’s more efficient than the underground.
Focus group participant
I think it’s open to being misused, the majority of people in general are not up to speed.
Focus group participant
Although there is some indication that older people are more likely to see computer assisted decision making as a threat, there is no clear correlation between attitudes to computer decision making and attitudes towards technology more widely.
Algorithms in different settings
Across the dialogue we found that the degree to which participants feel comfortable with and trust algorithmic decision making depends most strongly on the specific area and scenario in which it’s being used.
Figure 37: Overview of scenarios and sectors where participants feel more and less comfortable with algorithmic decision making:
Areas where participants feel more comfortable with the use of computer assisted decision making: | Areas where participants feel less comfortable with the use of computer assisted decision making: |
---|---|
* Media and entertainment e.g. playlist and TV recommendations * Banking and economics, analysing supply and demand * Diagnosis and care of health issues * Remote navigation * Air travel and space exploration |
* Recruitment and employment * Benefits, loans, mortgages, and credit * Education * End of life health * Criminal justice system * Defence and weapons systems |
In general, participants are more comfortable with algorithms being used in instances where the decision making is perceived to be purely factual and data-driven, with no need for human or emotional insight. There is also a sense that algorithms work best for repetitive tasks and instances where speed is valued.
Computer assisted decision making is beneficial in many situations, including travelling in an aircraft where autopilot is used, GPS used to drive cars, medical equipment used to save lives by constantly analysing data and computers making decisions about right medication and care.
Male, 65+, online community
If these systems enhance the user experience by saving time and recognising the user’s probable needs and can then direct the user more specifically to the area they need to be, for example, in medical situations where there’s an online questionnaire to determine what treatment would be the right one or not.
Male, 35-44, online community
In contrast, participants feel less comfortable with algorithms being used in more complex, nuanced and high-stakes situations, where emotional insight and a detailed understanding of personal situations is perceived to be important. Among participants, there is a strong sense that algorithmic decision making is not able to adequately account for the emotional aspects of the human experience.
Interpersonal services which require empathy or emotional intelligence should not be replaced by computers.
Male, 45-54, online community
There are always going to be issues around the use of any type of artificial intelligence in weapons systems. When, if ever, should a drone be allowed to make an executive, autonomous decision on weapon deployment?
Male, 35-44, online community
Algorithms in the public sector
While there is limited awareness of specific instances where algorithms are currently being used in the public sector, among some participants there is a general assumption that algorithms must be already being used by the public sector to streamline some processes.
Given the perception that algorithmic decision making can help save resources, time and costs, participants are generally comfortable with the concept of algorithmic decision-making being used in the public sector. However, there is an assumption from some participants that algorithmic decision making may require expensive technology, and that the public sector may not be able to afford ‘high-quality’ systems. There is also a concern that algorithmic decision making in the public sector may also replace certain jobs, leading to unemployment.
The issue I have with that is I know they cost a lot of money for those systems that are that intelligent, I would worry that the public sector won’t be forking out the money for those machines - they’d be using the ones that don’t have those features
Focus group participant
My fear in the public sector where I work, will they not need human beings anymore and we won’t have jobs?
Focus group participant
Transparency in the public sector
Despite the perceived importance of transparency in the public sector, there are very few specific positive or negative examples of public sector transparency that are front- of-mind for participants. On further probing, some participants identify the Covid-19 vaccination rollout as an example of where the Government has been successfully transparent. They feel that the public have been well-informed about the key decisions regarding the timings and order of the roll-out of vaccines, and the reasons behind these decisions. As well as being easy to understand, there is also a sense that the information has been timely and widely accessible.
I think the COVID-19 vaccination plan is fairly understood by everyone. It was all very open and clear why the decisions were made the way they were. You can’t get away from all the news briefings.
Focus group participant
In contrast, some participants express concern about the lack of transparency and clarity around individual decisions, including universal credit decisions. Not being given clear reasons and explanations for the final decision leads participants to feel that these decisions are inconsistent and unfair. A small number of participants feel that there is a certain degree of ‘cronyism’ in the current government, which they associate with a lack of transparency.
I would like information on why they got it [financial support] and I didn’t. All you get is a yes or a no. You don’t get why. The government are not justifying their actions.
Focus group participant