AI 2030 Scenarios Report HTML (Annex C)
Updated 28 April 2025
AI 2030 Scenarios
Helping policy makers plan for the future of AI
January 2024.
1. Executive Summary
1.1 Introduction
2022 saw AI break into the public consciousness with the release of Large Language Models (LLMs) including the OpenAI chatbot ChatGPT, and text-to-image models such as DALL-E 2. These tools were suddenly available to millions of people to interact with in simple language, no code required, and could be used for a range of tasks.
This moment significantly increased attention on developments in AI. But there is significant uncertainty around what the future holds, in particular at the frontier. We don’t know what the most advanced models will be capable of, who will own them, how safe they will be, how people and businesses will use them, and what the geopolitical context will be. These uncertainties will interact in unpredictable ways to create a specific future in which policy makers will operate.
GO-Science has developed five scenarios for developments in AI between now and 2030. They are designed to help policy makers explore the potential implications of different AI futures. We hope they help to develop policies that are resilient to risks, and to seize opportunities for the economy, society, and public services.
This report sets out evidence on a set of critical uncertainties, our AI 2030 scenario narratives, and the findings of a public engagement commissioned to explore public perceptions of the scenarios.
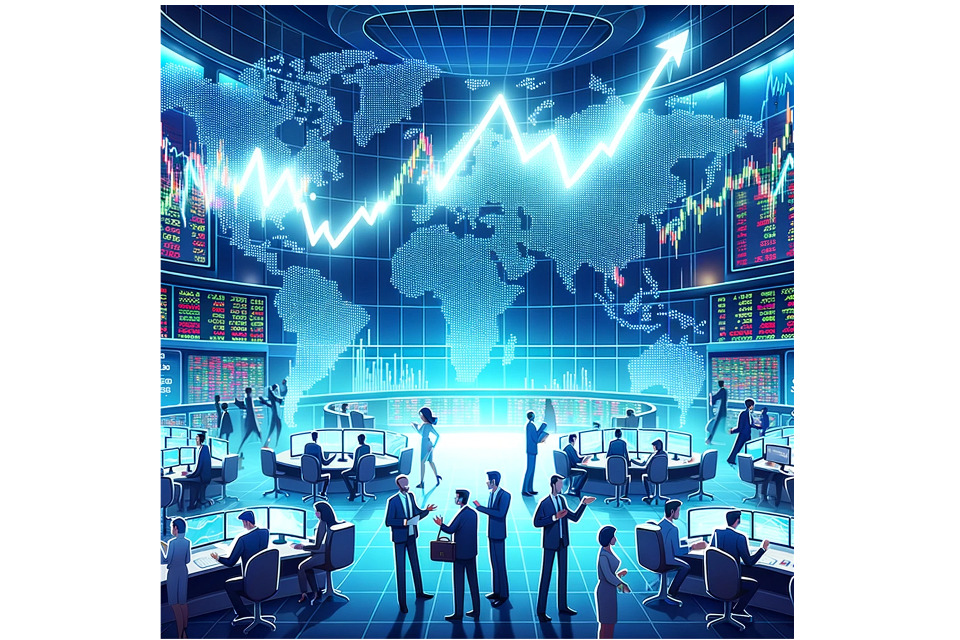
An AI generated image showing a bustling futuristic trade centre.
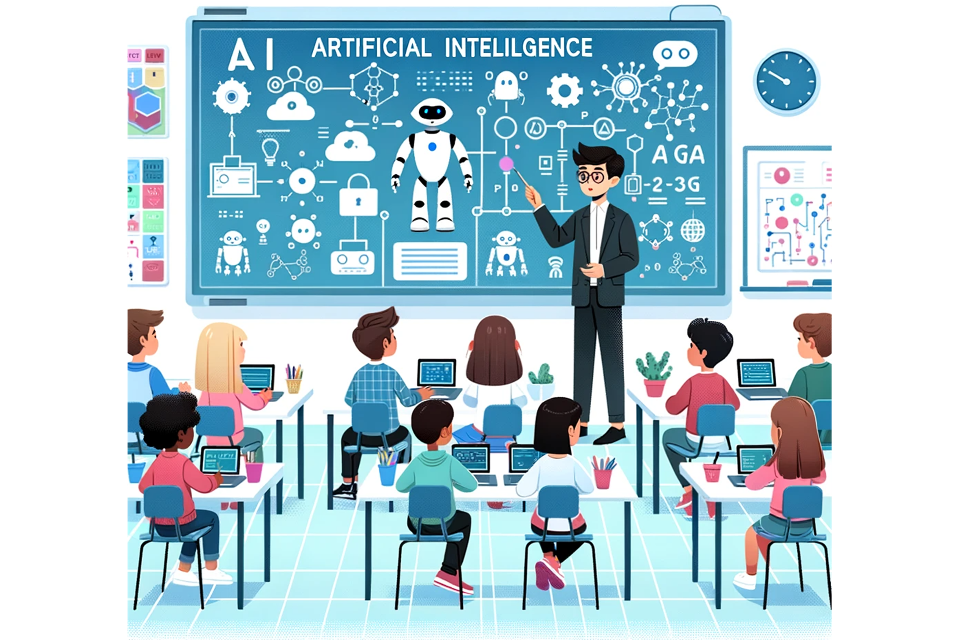
An AI generated image showing a school classroom, with a teacher teaching a class of children about AI.
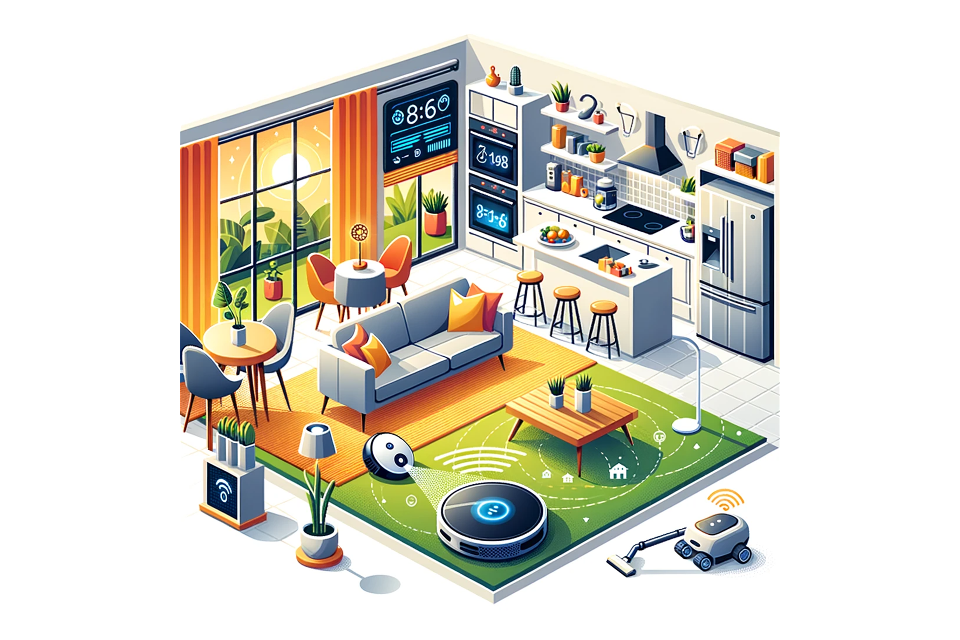
An AI generated image showing a high-tech living room, with autonomous cleaning robots and smart technology.
Figure 1 AI generated images illustrating possible aspects of a positive AI future. See note below on AI generated content.
1.2 Background and scope
Scenarios are a tool to help policy makers explore uncertainty and identify a resilient course of action that can withstand a range of future conditions. They are not predictions.
We have developed five scenarios, exploring developments in AI up to 2030. Events at a global level are described, but the focus is on implications for the UK.
The scenarios focus on Frontier AI the most capable and general models available in 2030. The models we consider Frontier AI today are only a small subset within the broad field of machine learning and AI. Many other approaches to, and applications of, AI exist. AI is not a singular thing. However, Frontier AI models have created a new, uncertain dynamic due to the pace of their improvement, their adaptability across multiple tasks, and their availability to anyone to interact with in natural language. These scenarios explore how this could play out to 2030.
The scenarios are designed to help policy makers, industry, and civil society test actions that might mitigate risks and navigate to a more favourable future. Policy makers need to be able to stress test new policy ideas against different plausible futures, so we have explicitly avoided including any such government interventions in the scenarios. As a result, they are all challenging, with difficult policy issues to tackle.
This does not mean a more positive AI future is implausible, or that the risks set out in this report are inevitable. There are many beneficial outcomes that could arise from AI developments, some of which are included in the scenarios. However, most experts we spoke to agree that policy intervention will be needed to ensure risks are effectively mitigated and benefits realised. As a result, we have deliberately avoided including any scenarios that are largely benign or favourable.
The scenarios are informed by the latest evidence and expert judgements. In drafting this Foresight report, and the Future Risks of Frontier AI paper, GO-Science consulted over 70 experts from industry, academia and policy making to ensure the scenarios and key findings were as robust and evidence based as possible.
These scenarios have some important limitations. They were developed and reviewed swiftly by experts to feed into the UK AI Safety Summit in November 2023, meaning certain evidence or perspectives may have been missed. Given the pace and unpredictable nature of AI development, some aspects of the scenarios may also soon become dated. Whilst they have been designed for use in different policy contexts, they may not be suitable in the form presented here for all AI policy questions.
1.3 The AI 2030 scenarios
The AI 2030 scenarios are built using five critical uncertainties, factors that are both important to the future of AI development, but also highly uncertain (Figures 2).
Figure 2: The five critical uncertainties
Capability: What ability will AI systems have to successfully achieve a range of goals? Will this include interaction with the physical world? How quickly will the performance and range of capabilities increase over time? |
Ownership, access, and constraints: Who controls systems? How accessible are they? What infrastructure and platforms are used to deploy systems? What constraints are there on the availability of AI systems? |
Safety: Can we build safe AI-based systems, assuring their validity and interpretability? How robust are systems to changes in deployment context? How successfully does system design ensure AI behaviour aligns to societal values? |
Level and distribution of use: How much will people and businesses use AI systems? What for and why? Will they be consciously aware they are using AI, or not? How will people be affected by AI misuse? How will use affect the education and jobs people do? |
Geopolitical context: What wider developments have there been at a global level that will influence AI development and use? Will there generally be more cooperation on big issues, or more conflict? |
The scenarios are described by a set of narratives. These cover outcomes across the critical uncertainties, and a range of implications across the economy, society and for peoples daily lives. A high-level vision for a positive AI future is also provided. Below are brief summaries of the scenarios alongside a slider chart to illustrate the critical uncertainty outcomes in each case.
1.4 Scenarios summary
Unpredictable Advanced AI
Highly capable but unpredictable open source models are released. Serious negative impacts arise from a mix of misuse and accidents. There is significant potential for positive benefits if harms can be mitigated.
AI Disrupts the Workforce
Capable narrow AI systems controlled by tech firms are deployed across business sectors. Automation starts to disrupt the workforce. Businesses reap the rewards, but there is a strong public backlash.
AI ‘Wild West’
A wide range of moderately capable systems are owned and run by different actors, including authoritarian states. There is a rise in tools tailored for malicious use. The volume of different AI systems is a problem for authorities.
Advanced AI on a Knife’s Edge
Systems with high general capability are rapidly becoming embedded in the economy and peoples’ lives. One system may have become so generally capable that it is impossible to evaluate across all applications.
AI Disappoints
AI capabilities have improved somewhat since the early 2020s, but more slowly than many expected. Investors are disappointed and looking for the next big development. There is a mixed uptake across society.
This graphic brings together the five individual graphics from above. The data from these graphics is available below:
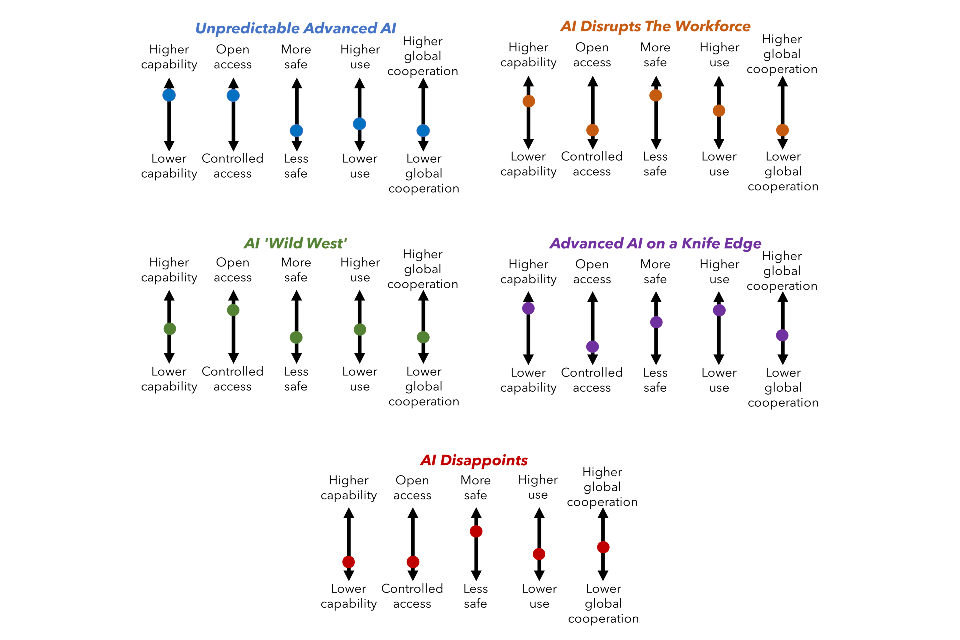
Scenario Sliders in relation to Critcal Uncertainties, Scored 1-5 (Low to High)
Scenario | Capability | Access | Saftey | Use | Global Cooperation |
---|---|---|---|---|---|
Unpredictable Advanced AI | 5 | 5 | 1 | 2 | 1 |
AI Disrupts the Workforce | 4 | 1 | 5 | 3 | 1 |
AI ‘Wild West’ | 3 | 5 | 2 | 3 | 2 |
Advanced AI on a Knife’s Edge | 5 | 1 | 4 | 5 | 2 |
AI Disappoints | 1 | 1 | 4 | 2 | 3 |
1.5 Public engagement
AI development will not happen in isolation. The direction AI takes will be strongly influenced by how citizens use it, shape it, and are affected by it. GO-Science, with the support of Centre for Data Ethics and Innovation (CDEI), commissioned the research company Thinks to carry out a public engagement based on the five scenarios. The research objectives for the engagement were to understand:
- public perceptions of the scenarios, including the risks, opportunities and plausibility of each;
- which elements of the scenarios members of the public feel strongly about, particularly their key concerns; and
- what factors could underpin mitigations to reach a more positive future scenario.
Given the rapid nature of our project, this engagement was conducted on a smaller scale and more quickly than would ideally be the case. Experts we spoke to agreed that large-scale, deliberative public dialogue will be needed to inform the development of AI safety systems and regulations, which could build on the findings of our study.
Frontier or Foundation?
There are several terms used for the largest AI models deployed today:
- Large Language Models (LLMs) - machine learning models trained on large datasets that can recognise, understand, and generate text and other content.
- Generative AI - AI systems that can create new content (images, text etc). The ability to create defines this type of AI.
- Foundation Models - machine learning models trained on very large amounts of data that can be adapted to a wide range of tasks. Adaptability defines this type of AI.
- Frontier AI - AI models that can perform a wide variety of tasks and match or exceed the capabilities present in today’s most advanced models.
These terms overlap (see contents for a full glossary). Today’s Frontier models are mostly trained with language data. They can generate text and, with fine tuning, be the basis for multiple more specialist models. Arguably, a model like GPT-4 meets all four definitions. However, what constitutes Frontier will change over time and may not always be based on the same architecture and training data as today’s LLMs.
1.6 Key findings
The evidence gathering, scenario analysis and public engagement that underpins this report has helped to identify the key findings below. The findings from this Foresight report build on those of the Future Risks of Frontier AI1 paper, published by GO-Science in October 2023. They draw on a common evidence base and expert engagement.
AI in future will, like today, be a mix of different models developed and operated by many different actors. This will include narrow models trained for very specific purposes. AI is not a singular thing. However, this report, and the findings below, focus on the most advanced and general models at the stage of AI referred to as Frontier AI.
- Future Frontier AI systems could have widespread positive impacts for society, including improvements in productivity and living standards, better public services, and scientific breakthroughs in health or energy. However, most experts we spoke to agreed that policy makers will need to act to fully realise these benefits, whilst mitigating the potential risks.
- The risks posed by future Frontier AI will include the risks we see today, but with potential for larger impact and scale. These include enhancing mass mis- and disinformation; enabling cyber-attacks or fraud; reducing barriers to access harmful information; and harmful or biased decisions.
- New risks could also emerge. What Frontier AI will be capable of by 2030 is highly uncertain but will shape the risks it poses. Risks will also depend on wider uncertainties in access to AI systems, ownership, safety measures, use by people and businesses, and geopolitics.
- As Frontier AI systems become more generally capable, it will become difficult to evaluate their performance and safety across all possible applications. Approaches to evaluation that address this dynamic will be needed.
- Use of highly capable narrow AI systems could also present policy makers with challenges, for example if used in widespread automation. This will need to be considered, alongside more general AI systems, when developing risk management policies.
- Over the next few years, a few large technology companies are likely to dominate Frontier AI. Longer term there is more potential for a shift away from this dynamic. However, in scenarios where highly advanced AI systems remain controlled by a few companies, they will hold a huge amount of power.
- Highly capable open source AI systems could also emerge. In scenarios where that happens, effective regulations or laws may need to cover any potential developer or user of these systems, not just the original creators.
- Negative impacts from future Frontier AI could be driven by a range of factors. These include rapid changes in AI capability, malicious use, ineffective safety systems, and wider societal consequences of well intentioned use. Policy makers will therefore need to consider interventions that can prevent or mitigate impacts associated with all these factors.
-
The scenarios highlight some low regret areas for policy makers that could help navigate towards a more favourable AI future:
- Addressing bias uses of AI will be held back by any bias or unfairness, whether perceived or actually held within models. Measures to identify, minimise and mitigate bias in Frontier AI models are likely to be part of any favourable future.
- AI skills and awareness to support the safe use of AI all scenarios look more favourable if more people and organisations in the UK are well placed to use the latest advances in AI safely and effectively.
- Stronger international collaboration a consistent theme of the scenarios is that it s harder to manage challenges if the mitigations governments put in place are not global. It follows that building trust, and a global approach to AI, is likely to be part of navigating to a more positive future.
-
Navigating towards a favourable future for AI will also require policy makers to consider choices and trade-offs. Some highlighted by the scenarios include:
- How openly accessible - open source models could accelerate innovation, involve a wider community in identifying issues, and reduce market concentration. Are these benefits worth the risks that come from anyone being able to access and adapt a powerful Frontier AI system?
- Pace of change faster development should mean greater benefits, sooner, in terms of productivity and breakthroughs. But there are many voices in favour of slowing down to give regulatory mechanisms a chance to keep up.
- Who benefits a consistent theme of the scenarios is the risk to those (individuals or sectors) who are left behind. How to share the benefits of future AI between citizens and their creators, and the role of government in managing this, are likely to be hotly debated out to 2030.
- Members of the public we spoke to felt that safety is paramount. There was a consensus among public engagement participants that safety was a higher priority than other factors like AI capability. Participants found risks easier to imagine and tangible, whilst key opportunities for the future were harder to grasp and value.
- Members of the public we spoke to felt that government and regulators are responsible for ensuring the safe use and development of AI, as well as safeguarding jobs. They also felt that organisations using AI were responsible for following regulation and behaving ethically, in order to build trust with the public.
1.7 How to use this report and the scenarios
These scenarios have been written policy off - they don’t describe any potential policies from the UK government that might be introduced to shape the future of AI. They all include challenges and problems for policy makers to solve.
Of course, none of the scenarios will describe the real future, which will likely feature elements of all these worlds. Another, more positive future is possible, and it is implausible to imagine the UK government does nothing between now and 2030 to steer towards it.
We shouldn’t overstate the influence a single government, any government, has over developments. But policies enacted now could help navigate toward a more favourable future than any presented in these scenarios. We recommend three different types of exercise to support development of such policies:
- Governments using this work to identify the future they do want for AI. Then working back from there to develop a plan to navigate towards this future. We call this backcasting.
- Exploring how different policy responses perform in each scenario, and how they might need to be adapted to achieve their objectives in different contexts. We call this stress-testing.
- Testing plans and assumptions against unanticipated shocks to ensure they are sufficiently resilient to a range of possible outcomes.
The primary audience for this report is government departments and expert stakeholders engaged in developing AI policy. Other organisations, such as businesses and local authorities, may also find these scenarios helpful for planning and strategy development.
2. Introduction
Recent developments in AI
Artificial intelligence (AI) is a broad and rapidly expanding field, encompassing the creation of machines that are capable of tasks otherwise requiring human intelligence to perform, for example problem solving and decision making. Multiple techniques are often used in combination to deliver a specific AI capability.
Historically, AI has been limited to systems able to carry out 1 or a small range of related tasks. Over the past 15 years, notable advances have come from machine learning approaches, using large neural networks and complex training methods that allow AI systems to learn how to perform a task from data.
More recently, this has led to the development of foundation models, trained using large amounts of data and compute, and capable of performing a range of tasks. This includes generative AI systems such as LLMs, capable of recognising, understanding, and generating text and other content.
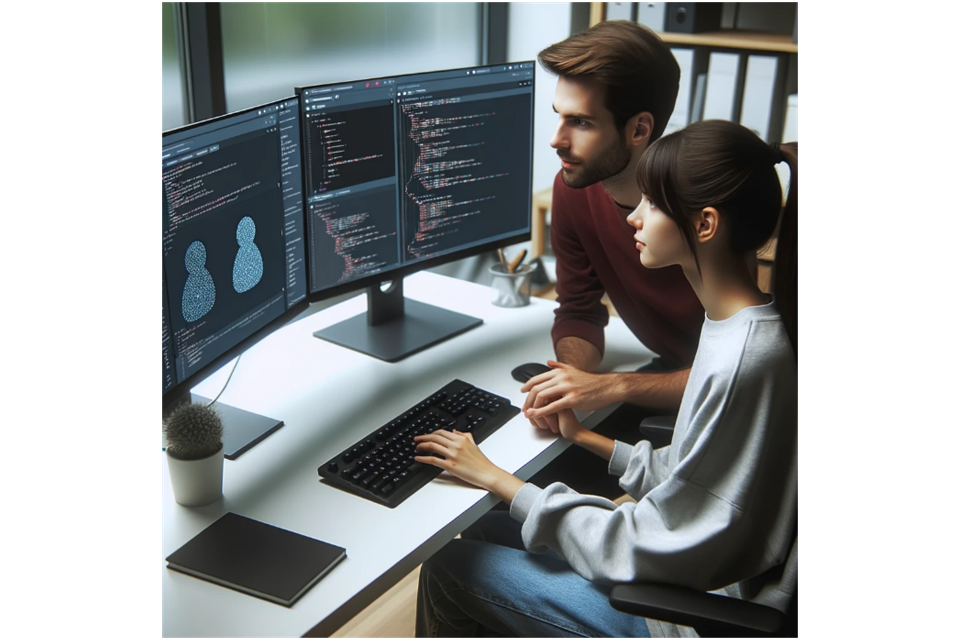
An AI generated image depicting 2 people using AI, while working from home.
Figure 4 Generated with Dall-e 3. We prompted the tool to depict people using AI from home. See note below on AI generated content.
Technical developments, such as the advent of transformer architectures and approaches to reinforcement learning (RL), have also contributed in recent years to the creation of increasingly capable models at the Frontier of AI.
This report uses the government s chosen definition of Frontier AI is a model that can perform a wide variety of tasks and match or exceed the capabilities present in today s most advanced models . As of October 2023, this primarily encompasses foundation models consisting of huge neural networks using transformer architectures. However, there is uncertainty over the form that Frontier AI systems could take in future.
Alongside Frontier AI becoming increasingly capable and multimodal, AI tools are being made more accessible to the general public. The ability to access models through online platforms using natural language interfaces has brought AI into the public consciousness . Within 5 days of OpenAI releasing its LLM-based chatbot ChatGPT to the public, the platform had reportedly reached 1 million users, and this grew to 100 million users within 2 months.
Other Frontier AI companies have followed suit, releasing their AI models for use by the general public. Google have used their LaMDA family of LLMs, and more recently their PaLM LLMs, to power their Bard chatbot , whilst Meta have revealed plans to launch a range of chatbots with different personas, powered by their Llama 2 LLM . Recent advancements have also extended to the creation of multimodal models, such as GPT-4, the first GPT model capable of responding to prompts of both text and images.
Data from this graphic shown below:
Date | 2014 | 2015 | 2016 | 2017 | 2018 | 2019 | 2020 | 2021 | 2022 | 2023 |
---|---|---|---|---|---|---|---|---|---|---|
Developments | Advent of Generative Adversarial Nets (GANs) | Advent of residual nural networks. Considerable progress in development of recurrent nural networks (RNNs) and short-term memory (LSTM) models | Google’s AlphaGo defeats Go World Champion | Advent of the transformer architecture | LLMs realseased include: BERT (Google), GPT-1 (OpenAI) | GPT-2 realeased by OpenAI | Start of the ‘Big Model Era’, GPT-3 (OpenAI) realeases | Latent Diffusion: DALL-E (OpenAI) realeased, DeepMind realeases AlphaFold 2 | Models realseased include: Chinchilla (DeepMind), LaMDA PaLM (Google), OPT (Meta), ChatGPT (OpenAI), BLOOM (Hugging Face), Stable Diffusion (Stability AI) | Models released so far include: GPT-4 (OpenAI), Claude (Anthropic), LLaMA (Meta), DinoV2 (Meta computer vision model, PaLM 2 (Google), Bard (Google chatbot) |
Such Frontier AI models also now demonstrate limited capabilities in planning and reasoning, memory, and mathematics. A more comprehensive list of current Frontier AI capabilities can be found in the GO-Science Future Risks of Frontier AI paper1.
Beyond Frontier AI, other AI systems and tools are being applied in a range of contexts, such as protein structure prediction and cancer imaging and diagnosis. Machine learning technology is already embedded into many online services we use every day, including social media, maps, streaming services, insurance, and online shopping. Opportunities and benefits from use of AI are highly likely to grow in impact and scope as systems become more capable in the future.
Current market structure
AI is developed and deployed by many companies and institutions, big and small. However, developments in Frontier AI are dominated by a small number of companies. These include OpenAI, Google DeepMind and Anthropic, who have the resources to train the largest models.
Although smaller companies and the open source AI community have the potential to disrupt this market structure in future, barriers such as access to compute, data and funding would need to be overcome or become less important (see Chapter 3).
Risks from AI
Whilst the increasing capability of Frontier AI offers numerous potential positive impacts, it also presents significant risks. These include increasing mass disinformation and deepfakes, enabling cyber-attacks, reducing barriers to access harmful information, and enabling fraud.
Current Frontier AI models are also susceptible to amplifying existing biases within their training data and providing potentially harmful and discriminatory responses. Training on large swathes of UK and US English internet content can mean that misogynistic, ageist, and racist content is overrepresented in the training data .
This is by no means an exhaustive list of the current risks from AI. A number of high-quality reports cover this topic in more detail, including those HMG, Alan Turing Institute, Parliament, the IMF, CSET, OWASP, Stanford University, and the OECD.
Over the past year, several leading figures in the field have expressed concern at the pace of recent developments and the unpredictable trajectory of future AI capability.
In March 2023, a large group of prominent AI experts, researchers and entrepreneurs signed an open letter calling for a six-month pause on the training of AI systems more powerful than GPT-4.
The heated debate on the potential for AI to present catastrophic or existential risk to humans led to calls for governments around the world to consider this a global priority.
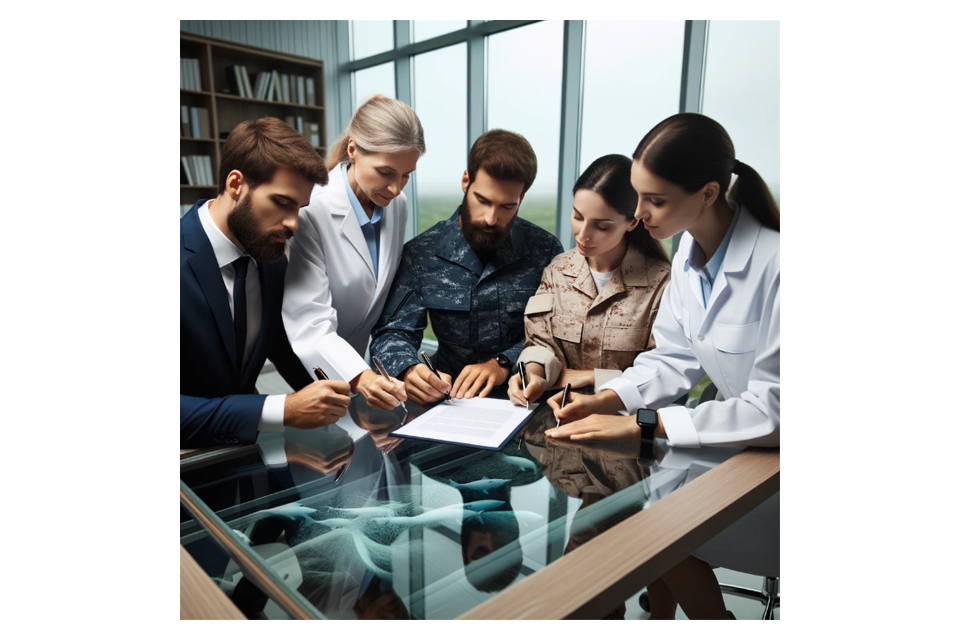
An AI generated image depicting a group of experts signing a letter agreeing upon AI safety and safe development.
Figure 5 Generated with Dall-e 3. We prompted the tool to depict a diverse group of experts signing a letter. See note below on AI generated content.
However, many experts consider existential risks to be very low likelihood with few plausible routes to being realised. They suggest there should be more focus on addressing current AI risks, which are set to get become more acute with increasing AI capability and access. Such differing expert views present policymakers with a high degree of uncertainty.
In this context of rapid change and rising concerns, GO-Science has developed this report to help policymakers plan and develop resilient AI policies and strategies.
2.1 Scope of this report
The future of AI development is surrounded by significant uncertainty. This includes uncertainty around the development of future capabilities and safety systems, as well as how people and businesses use AI and the resultant impacts both in the UK and globally. We have developed a set of scenarios to help explore these interacting uncertainties.
The scenarios are not predictions. Each scenario explores the events leading up to a plausible future in 2030. Plausible in this context means that the experts we spoke to generally felt these scenarios could happen given the bounds of uncertainty.
The scenarios are designed to help UK policy makers, industry and civil society explore AI risks and opportunities. Developments at a global level are described, but the focus is on implications for the UK. Policy makers can use these scenarios to test strategies and policies designed to navigate to a more favourable AI future for UK s economy, society, and public services. To make the scenarios usable for this purpose, we have intentionally avoided introducing new government interventions in the scenarios.
One consequence of this design choice is that all scenarios are challenging, with difficult policy issues to tackle. This does not mean a more positive AI future is implausible, or that the negative impacts described are inevitable. There are many beneficial outcomes that could arise from AI developments, some of which are included in the scenarios. However, most experts we spoke to agree that policy intervention will be needed to ensure risks can be comprehensively mitigated. As a result, we have explicitly avoided including any scenarios that are largely benign or favourable.
The scenarios focus on Frontier AI the most capable and general models available in 2030. However, the average AI systems in use could be significantly more capable than today. The scenarios therefore also consider some developments away from the Frontier.
2.2 A note on AI generated content
This report includes text artefacts and images that have been fully or partially generated by AI tools. This represents an experiment, designed to illustrate some of the current capabilities of AI.
Whilst we think this content does demonstrate some impressive AI capabilities, we also acknowledge variation in quality of some of the outputs. In particular, the AI tools we used struggled with concepts like diversity when depicting groups of people. They also produce errors, for instance a group of experts signing a table.
We decided to include these problematic images to help highlight these current biases and deficiencies. This content has been labelled as AI generated to alert the reader.
3. Methodology
This chapter sets out the methodology used to develop the AI 2030 scenarios and the key principles that informed this development.
3.1 Introduction
What are scenarios?
These scenarios are stories that describe alternative ways in which the uncertainties surrounding AI might play out in the future. Each scenario explores how different conditions might support or constrain delivery of policy and strategy objectives. Scenarios are never predictions, rather a way to imagine different versions of the future, explore how they could be brought about, identify the risks and opportunities they represent and help to guide decisions on what we should do now as a result.
The scenarios are not mutually exclusive and there is likely to be some overlap. The real future is likely to contain elements of all five the scenarios to varying degrees.
What are the principles for our scenarios?
The principles that underpin these scenarios are:
- Plausible: Scenario end states should feel like they could happen by 2030. This means uncertainties had to be combined in a coherent way, considering their likely interactions. To ensure plausibility, each scenario narrative also had to consider what might happen between now and 2030 to reach the end state.
- Stretching: As the scenarios are being developed to stress-test government strategy, they should feel stretching and, in some cases, uncomfortable or negative, diverging from what policy makers consider to be the business as usual trajectory.
- External to government action: These scenarios have been designed without UK government intervention i.e., we have intentionally avoided imagining any new regulations. UK policymakers can therefore use them to test a range of policy ideas and government actions in order to work out how best to mitigate the downsides of each scenario and navigate to a more favourable future. The potential outcomes in a more positive AI future are described in Chapter 4, but as many experts told us that reaching such a future will require policy interventions, this is not included in our scenario set.
3.2 Methodology
Our scenario development approach is a hybrid of the qualitative workshop-based approach set out in the Futures toolkit and a more technical General Morphological Analysis of how multiple related variables could plausibly combine. This hybrid approach allowed us to benefit from the qualitative insights of a large group of experts, whilst exploring complex interactions between uncertainties in a more structured way. We are grateful for the input received from over 70 experts from academia, industry, and government departments across various workshops and subsequent rounds of peer review. The methodology comprises four stages:
- Critical Uncertainties. Critical uncertainties are factors that are both important to the future of AI development, but also highly uncertain. They provide the building blocks for our scenarios and were developed using a combination of desk research and expert workshops. An initial list of 27 sub-uncertainties were identified. These were then clustered together into the five broad-brush critical uncertainties that were used to develop the scenarios. Table 1 shows the final five critical uncertainties and the sub-uncertainties that were clustered together to create them.
Table 1: Summary of critical uncertainties, descriptors, and sub-uncertainties
Uncertainty | Description | Sub-uncertainty |
---|---|---|
Capability | What ability do AI systems have to successfully achieve a range of goals? Can they interact with the physical world? Does the level of success and range of goals achieve increase over time? | • Capability • Generality • Understanding the physical world • Autonomy and agency • Self-improvement • Developments in other technologies |
Ownership, access, and constraints | Who owns systems? How do they deploy them and give access? What infrastructure and platforms are used to deploy systems? What constraints are there on availability of AI systems? | • Market structure • Access Closed vs open source models • System design and complexity • Levels of investment • Access to data and other inputs • Compute constraints |
Safety | Can we build safe AI-based systems, and assure the validity and interpretability of their internal models? How robust are these systems to changes in deployment context? How successfully do their design processes ensure that their behaviour aligns to societal values? How much have we allowed AI to control systems that could impact our safety? | • Controllability • Model interpretability • Changes in deployment context • Alignment / potential for bias • Control over critical systems |
Level and distribution of use | How much will people and businesses use AI systems? What will they use them for and why? Will they be consciously aware they are using AI, or will these systems be more subtly embedded in products and services? How does use of AI across society affect the education and training people undertake and the jobs people do? To what extent do other factors like levels of trust in institutions influence AI development and use? | • Integration into daily practices of members of the public • Level of public access, knowledge and skills inequality • Integration into business practices, products and services • UK professional skills • Public involvement in shaping how AI is used • Public sentiment • Level of AI misuse |
Geopolitical context | What wider developments have there been at a global level that will influence AI development and use? | • International cooperation on AI • Links to cooperation on other global issues, particularly climate change • Level of conflict in the world • Reliance on globalised supply chains versus re-shoring |
- Axes of Uncertainty. For each critical uncertainty, we constructed a high-level Axis of Uncertainty consisting of 2 plausible but extreme 2030 outcomes for the uncertainty. These represent an unrealistically binary simplification, but this was necessary to help narrow down to a manageable set of scenarios, before reintroducing complexity and detail at the end of the process. Table 2 shows the final set of axes.
Table 2: Final set of axes of uncertainty
Axes of uncertainty
Lower capability: Gradual improvement in generative AI and other specific tasks. System limited to ‘call and response’ with human oversight. Not self-improving | Higher capability: Initial AGI. Can act without human input, including in the physical world. Self-improving and can set own goals. AI can connect to other new technology. |
Controlled access: Mainly big tech owned, centralised & API accessed. Higher levels of investment per model. Higher compute requirements. Constrained supply of inputs (data, chips and algorithms) | Open access: More smaller start-ups and open source. More decentralisation and local running. Lower levels of investment per model. Lower compute requirements. Unconstrained supply of inputs (data, chips and algorithms) |
More safe: Safety systems are reliably implemented and keep pace with developments and deployment context. Models are interpretable. Models are aligned to a widely accepted set of societal values. AI has only been embedded in critical systems where there is confidence in the reliability of safety systems. | Less safe: Safety systems developments are slower than AI developments. AI systems are deployed in new contexts before full evaluation of performance. Models are less interpretable. Models are aligned to a limited set of societal values and can make biased decisions or output harmful content. AI has been embedded into certain critical systems, with utility trumping safety. |
Higher use: AI systems are embedded in many daily practices. Businesses develop attractive AI-based products, and use of AI in these products is disclosed to the public. Government and businesses seek public views on in AI-use decision making. This contributes to positive public sentiment on AI. | Lower use: AI systems are less embedded in daily practices. Businesses develop few attractive AI-based products, and use of AI in these products is opaque to the public. Government and businesses ignore public views on in AI-use decision making. This contributes to negative public sentiment on AI. |
Higher global cooperation: International partners share resources and align regulations. There are lower levels of global conflict and supply chain disruption. | Lower global cooperation: International partners withhold resources and take regulations in different directions. There are higher levels of global conflict and supply chain disruption. |
-
Narrowing down the possible scenarios. Even just using the extremes of each axis of uncertainty, there are 32 combinations of outcomes and possible scenarios. We needed to narrow these down to a more manageable number. To do so, we used a process of impact mapping and morphological analysis to test each axis of uncertainty outcome against every other outcome to identify combinations that were particularly implausible or incoherent and which could be eliminated. The result was a shortlist of 13 possible scenarios, which were then voted on by experts to help identify the final five scenarios deemed to be sufficiently diverse and interesting.
-
Scenario narrative development. Each of the five scenarios is made up of different combinations of outcomes for the five critical uncertainties to create a coherent, plausible, and diverse set. The final stage of the process involved developing fuller scenario narratives, informed by expert input and our own assessments of plausible outcomes for all the critical uncertainty combinations. Detailed scenario narratives are provided in Chapter 5.
4. Evidence and uncertainties
4.1 Introduction
As the capabilities of AI systems rapidly improve, researchers and policymakers are working to monitor and measure these developments and their impacts. But difficulties in measuring such rapid changes mean that there is considerable uncertainty about the scale and nature of these impacts, and how they will evolve in the future.
This section summarises the evidence base that has informed our scenarios, as well as highlighting the areas where there is significant residual uncertainty. We focus on the five Critical Uncertainties introduced above, but first cover more certain drivers of change.
Drivers of change with more certainty
Whilst the Critical Uncertainties form the building blocks for our scenarios, there are also other, more certain drivers of change for AI development that will shape the majority of plausible futures. The following factors have been identified by engagement with a range of experts from academia and industry, in combination with a review of the existing literature in this area.
These factors are not all mentioned explicitly in each scenario narrative, but it can be assumed that the changes described below are, to some extent, present in the background of all five of our scenarios.
Frontier models will continue to get more capable over the next few years. The trend in recent years has been that increased amounts of compute and training data has yielded increased capability of models, referred to as scaling laws. Whilst these improvements have not always followed a neat linear trajectory, and have sometimes been unexpected, bigger models have, in general, been more capable. With industry experts suggesting that the next iteration of Frontier models will be trained using an order of magnitude more compute than current systems, it looks certain that these models will see some level of capability improvement.
As well as becoming more proficient at existing capabilities, like content creation and computer vision, experts also expect future Frontier AI systems to demonstrate new capabilities, such as increased multi-modality, creating more long-form structure text, and carrying out data analysis and visualisation. Current and potential future capabilities are discussed in more detail in the Capability section below and in the GO-Science Future Risks of Frontier AI paper1.
Although some experts have voiced concerns that compute or data shortages or the end of scaling laws could constrain Frontier AI developments, this was not anticipated to be an immediate concern.
A few AI companies are likely to remain at or close to the frontier of AI. The leading, most capable AI models are currently developed by big tech companies such as OpenAI, Google DeepMind and Anthropic who have access to the funding, skills, data and compute required. It is likely that a select group of AI laboratories will carry on developing the Frontier models as academia and open source communities continue to be unable to access the same levels of funding.
While big companies will likely dominate the AI frontier, open source models, like Falcon or Mistral, cannot be discounted. If such an open source model were to approach the capability of an AI system like GPT-4, it would be powerful tool for developers outside these big companies to adapt . However, even if notable advancements were to originate from the open source community, the leading AI companies would quickly be able to catch up.
Existing harms are already being exacerbated by AI, and this is likely to get worse. Harms from activities like spreading mis- and disinformation, deception, or creation and dissemination of other harmful content are already being increased by use of AI tools. Digitally manipulated images are also being used for political purposes, and there are concerns that deepfakes and misinformation could threaten democratic processes.
Evidence has shown that AI can produce compelling disinformation that can be harder to spot than disinformation produced by humans . As AI systems continue to become more widely available and more capable, many experts believe that this will lower the bar for such activities and increase their impacts.
Machine learning technology is already embedded into many online services, and this is likely to continue. Integration of AI into services such as healthcare and banking has already taken off in recent years . Many of the online tools we use every day rely on integrated machine learning algorithms to automate and personalise services, including social media, maps, streaming services, insurance, and online shopping (discussed in more detail in the Level and distribution of use section below). As AI systems become increasingly capable and readily available, it is somewhat inevitable that more private and public services will incorporate these tools and technologies.
Critical uncertainties
As described in Chapter 3, we have identified five broad critical uncertainties for AI development: ‘Capability’, ‘Ownership, access, and constraints’, ‘Safety’, ‘Level and distribution of use’, and ‘Geopolitical context’. These form the building blocks of our scenarios. Each is made up of a series of sub-uncertainties, described in more detail below. Each section also includes ‘wildcards’, more radical possible outcomes associated with the critical uncertainty, alongside a high-level description of its relationship to the other critical uncertainties.
Capability: What ability will AI systems have to successfully achieve a range of goals? Will this include interaction with the physical world? How quickly will the performance and range of capabilities increase over time? |
Ownership, access, and constraints: Who controls systems? How accessible are they? What infrastructure and platforms are used to deploy systems? What constraints are there on the availability of AI systems? |
Safety: Can we build safe AI-based systems, assuring their validity and interpretability? How robust are systems to changes in deployment context? How successfully does system design ensure AI behaviour aligns to societal values? |
Level and distribution of use: How much will people and businesses use AI systems? What for and why? Will they be consciously aware they are using AI, or not? How will people be affected by AI misuse? How will use affect the education and jobs people do? |
Geopolitical context: What wider developments have there been at a global level that will influence AI development and use? Will there generally be more cooperation on big issues, or more conflict? |
4.2 Capability
Overview
Capability can be defined as the range of tasks or functions that an AI system can perform and the proficiency with which it can perform them. This includes how the level of success and range of goals achieved increases over time, as well as the extent to which these systems are able to interact with the real world.
Whilst today’s Frontier AI models are significantly more capable than their predecessors, there is ongoing debate over whether they display some capabilities, such as planning or reasoning. And whilst it is almost certain that Frontier AI will get more capable over the next few years, there is significant uncertainty over which capabilities will be developed and when.
This in turn means that the risks posed by future Frontier AI systems are highly uncertain. However, experts highlighted several capabilities, that if realised, could increase the likelihood of future AI systems posing a serious risk. These included: agency, autonomy, self-improvement, and the ability to evade human oversight and shut down.
Sub-uncertainties
Generality
Historically, AI systems have tended to be trained on a narrow dataset to carry out 1 or a small range of related tasks. More recently, there has been a shift towards using complex training approaches and increasing amounts of data and compute to develop large and powerful neural networks. The graph below illustrates the trend of an increasing number of parameters in leading AI systems .
The last decade has seen a clear trend of increasing model size and compute yielding improved capabilities and performance on benchmark tests – so called “Scaling Laws”28. In addition to models showing improved accuracy in tasks they have been trained for, such as next word prediction , new capabilities have also “emerged” as models have increased in size, resulting in improved generality (see box below) .
Figure 6: The number of parameters (variables adjusted during training to establish how input data gets transformed into desired output) in notable AI systems from 1995-2023. Source: Epoch (2023)
Data from this graphic shown below:
Number of Noteable Systems by E+ Parameter
Date | E+00 | E+01 | E+02 | E+03 | E+04 | E+05 | E+06 | E+07 | E+08 | E+09 | E+10 | E+11 | E+12 |
1995-2001 | 1 | 3 | 1 | ||||||||||
2001-2006 | 1 | 1 | 3 | 1 | 3 | 2 | |||||||
2006-2012 | 1 | 4 | 5 | 1 | |||||||||
2012-2017 | 2 | 5 | 10 | 22 | 3 | ||||||||
2017-2023 | 6 | 9 | 18 | 22 | 13 | 10 | 5 | ||||||
2023-2028 | 1 | 1 | 1 | 2 | 5 | 1 |
Emergence: a disputed paradigm
As foundation models have increased in size, a range of “emergent” capabilities have been reported. This refers to a skill that the model was not explicitly designed to do, and was not present in smaller models, “emerging” above a certain scale. 1 example of this is ability to perform addition, subtraction, and multiplication .
There is ongoing debate as to how many capabilities truly “emerged”. Some experts cite early experiments suggesting “emergent” capabilities can be explained by models following instructions and pattern matching in their training data (in-context learning and memorisation respectively). Further it was suggested to us that many emergent abilities are at least within the expected domain of the model in question (for example, producing text).
However, while it remains plausible that unexpected capabilities could emerge as models get bigger, the capabilities and generality of future Frontier AI systems will be highly uncertain and making detailed predictions will be challenging.
Some experts believe we are on a trajectory towards artificial general intelligence (AGI), a system capable of human-level or higher performance across most cognitive tasks. In 2023, researchers at Microsoft were struck by the capabilities exhibited by GPT-4 across a variety of domains and tasks, enough to posit the existence of “sparks of artificial general intelligence” . How close we are to AGI remains the subject of debate . Many experts are sceptical about it arriving soon, and without new model architectures. Others, including some who build current Frontier Models, are more bullish.
Given the potential future risks that a highly advanced, general AI system could pose, many believe it is a possibility that should be planned for. The ‘Safety’ section below discusses challenges in evaluating the performance and safety of a highly advanced, general AI system across all possible applications.
Whilst many labs are aiming to develop increasingly general systems, experts we spoke to also expect the development of capable but narrow AI systems to continue. Such systems can provide cutting-edge performance for specific applications and use cases, for example, protein structure prediction using AlphaFold . Narrow AI systems, fine-tuned to perform specific tasks, may also be easier to integrate into business processes.
How much more general will Frontier AI systems be by 2030? Will unexpected capabilities emerge? What will the role of advanced narrow AI systems be?
The rest of this section covers other capabilities that one might expect from a highly general future AI system.
Computer vision
Computer vision covers a range of tasks from image labelling to object classification which are becoming increasingly well developed in today’s Frontier AI systems. Large multimodal datasets have led to adaptable models that can respond to text and images, such as GPT-4, SAM, CLIP, PALM-E, and DINO. Continuing to improve these capabilities is likely to be important for developing more general Frontier AI systems in future although there remains uncertainty over whether computer vision capability will scale in line with increasing size of multimodal datasets.
Planning and reasoning
current Frontier AI models have displayed limited planning and reasoning abilities, for example passing exams that require problem solving. However, there is ongoing debate about the extent to which they show true abstract reasoning or are matching to patterns in their training data. Novel architectures, training, and fine-tuning approaches are already being explored to enhance planning and reasoning capabilities. However, there is uncertainty over which of these novel approaches, if any, will prove the most successful for future Frontier AI systems.
Enhanced memory
Current Frontier AI models do not have an explicitly built-in capability to remember previous tasks, outcomes and users. Enabling models to query up to date databanks or increasing the length of user inputs can confer some of the useful properties of memory but often at the expense of accuracy and cost. Several approaches to improving AI memory are being pursued, although it is uncertain which will be most successful. These include developing cheaper and more effective ways of processing large prompts, as well as connecting models to banks of relevant information, known as retrieval augmented generation.
Understanding the physical world
Another key uncertainty is the extent to which AI systems will be successfully integrated with robotic systems to interact with the physical world. As an example, Google DeepMind’s Robotic Transformer 2 (RT-2) system, underpinned by their PaLM-E language model, has displayed some capability to recognise and move physical objects when prompted . Developing AI systems with an understanding of the physical world remains a key outstanding challenge and is likely to be crucial for the future application of Frontier AI systems for the design and optimisation of physical processes (e.g. manufacturing). This will also require the ability of AI systems to apply concepts such as space, time, gravity, and understand how different objects interact.
Experts have suggested that training models on an increasingly wide range of multimodal data (e.g. images and video) could accelerate the degree to which AI systems can understand the real world.
At what rate will this capability improve by 2030? How will it interact with developments in the generality vs narrowness of Frontier AI systems?
Autonomy and agency
Developments in foundation models over the last 18 months have prompted efforts to develop more autonomous digital systems. This encompasses the ability to plan and complete multiple sequential tasks to achieve a given goal without direct human oversight. Increased autonomy and agency of AI systems has been facilitated by the development of “wrappers” that harness the capabilities of LLMs for more complex multi-step problem solving and decision-making. Notable examples include apps such as LangChain and Auto-GPT . The latter is powered by GPT-4 and breaks down its goal into sub-tasks before using tools on the internet to achieve these without human oversight . As 1 example, when asked to build an app, it is claimed it was able to: work out what software and content is needed, secure access to the tools, write code, test it, and review the results. This was reported, on an unverified blog, to have been completed with AutoGPT asking and answering its own questions until the task was complete. However, other users note that AutoGPT frequently gets stuck in a loop of questions and answers.
Currently, autonomous agents possess a small range of capabilities and can only independently carry out relatively basic tasks. However, research is ongoing in this area to develop the necessary platforms and infrastructure to connect agents to a wide range of tools. Improving other capabilities such as memory, planning and reasoning and self-correction are likely to be crucial to delivering truly autonomous AI agents ,.
As outlined in the “Generality” section above, research is ongoing into the development of improved planning and reasoning and memory capabilities but there is uncertainty over which approaches will be most successful, and how they can be integrated into truly autonomous AI agents in future. Will such agents be available by 2030?
Self-improvement
Some experts believe that in future, AI models could become able to suggest improvements or autonomously self-improve but how this capability could emerge remains unclear. Some have suggested that using AI to produce training datasets or provide feedback to models during reinforcement learning could provide an important initial step towards self-improvement . Researchers at Google DeepMind have recently developed a self-improving AI agent for robotics called RoboCat that can self-generate new training data to improve its technique.
It is also plausible but uncertain that future AI systems may be able to autonomously edit and fine-tune their own code and training. Some experts view the ability of future AI systems to edit their own code as a potential pathway to an “intelligence explosion” event along the trajectory towards AGI although there is significant uncertainty about how this could occur. Suggestions that AI systems are already capable of improving on the code-writing ability of humans currently remain disputed and this form of self-improvement has so far required human oversight.
Changes in model architecture
As well as uncertainty over the capabilities that may emerge in the next generation of Frontier AI, there is also uncertainty over the development of new model architectures. The advent of the transformer architecture in 2017 paved the way for the Frontier LLMs in existence today. Scaling of data and compute used to train LLMs has in recent years resulted in new and unexpected capabilities. However, it is plausible that a new architectural development could be an alternative accelerant for the development of increasingly advanced AI systems in future.
Figure 7: The change in capabilities of AI systems, 1998 – 2020. Developments in “deep learning” (2012) and transformer architecture (2017). resulted in step changes in capability. Source: Kiela et al. (2021) – Dynabench: Rethinking Benchmarking in NLP.
Data from this graphic shown below:
Test Scores of AI Relative to Human Performance, human performance as a benchmark is set to 0
Date | Handwriting Recognition | Speech Recognition | Image Recognition | Reading Comprehension | Language Understanding |
---|---|---|---|---|---|
1998-2000 | -70 | -95 | N/A | N/A | N/A |
2000-2005 | -35 | -90 | N/A | N/A | N/A |
2005-2010 | -25 | -75 | -80 | N/A | N/A |
2010-2015 | -5 | -55 | -30 | N/A | N/A |
2015-2020 | 5 | 5 | 10 | 20 | 10 |
Developments in other technologies
The emergence of new AI capabilities could be facilitated by developments in other technologies. For example, improvements in hardware and robotic platforms could enable the integration of machine learning with embedded systems, which can reduce latency and increase the capability of machine learning in edge devices, such as smart watches and cameras .
The fields of neuroscience and AI are heavily intertwined and continue to drive each other forwards ,. Future developments in applied neuroscience could provide more insight into how the brain performs computation, leading to advances in neuromorphic computing and the design of more complex machine learning models . Developments in other emerging technologies, such as quantum computing and future telecoms could also impact on developments in AI in future.
Relationships to other critical uncertainties
This section provides a few key examples how different outcomes for the ‘Capability’ critical uncertainty could be correlated with outcomes on other critical uncertainties, including how these correlations might be contingent on other factors. This information was developed using outputs of the scenario development workshops (see Chapter 2).
Low capability: Gradual improvement in generative AI and other specific tasks. System limited to ‘call and response’ with human oversight. Not self-improving. Experts considered lower capability to be most strongly coherent with: Open access: If capability development is slow (suggesting no big new breakthroughs and scaling laws running out), open source will catch up to big labs. Less safe: Although many safety concerns arise from the potential for increasing capability, others related to misuse could still grow in a low capability world. This could be particularly problematic if there is a diverse array of lower capability AI systems that are harder to regulate. |
High capability: Initial AGI. Can act without human input, including in the physical world. Self- improving and can set own goals. AI can connect to other new technology. Experts considered higher capability to be most strongly coherent with: Controlled access: A world with higher capability is more likely to be 1 in which big tech firms have accelerated developments. It is also likely that this would be a world with high data and compute requirements, and where firms control access to these capable systems. Less safe: Safety and regulations tend to lag behind capability – the faster the capability improvement, the more this will be the case. Low global cooperation: It’s plausible that development of high capability systems could drive an ‘arms race’ dynamic. |
Wildcards
Wildcards are radical outcomes associated with each critical uncertainty. These are considered possible, but not likely, on a 2030 timescale. Policy makers could use these as possible ‘shocks’ to test their plans against (see Chapter 6).
Superintelligence
An AI system is developed that is capable of autonomous and recursive self-improvement. This results in an intelligence explosion event, leading to the emergence of an ‘artificial superintelligence’, which exceeds the cognitive performance of all humans at all tasks. Whilst the possibility of such an outcome is disputed, particularly on a 2030 timescale, its impacts could be so significant that there may be value in policy makers considering such a potential shock.
Neurological integration
With advances in AI capability and complementary hardware, people start to embed AI to improve their own capabilities. This begins with wearable tech but escalates to people using technological implants designed to enhance mental and physical performance. This has the potential to divide society, both in terms of inequality, with those who can afford to enhance their own capabilities benefiting most, as well as a broader philosophical debate about whether this signals a change in what it means to be human.
4.3 Ownership, constraints and access
Overview
The Frontier AI industry is heavily concentrated, with a handful of technology firms such as OpenAI, Google DeepMind and Anthropic developing and controlling the leading models. As systems becomes increasingly capable and complex, there is uncertainty over whether computational and data requirements will hinder the ability of smaller companies and the open source community to keep pace with Frontier AI firms.
The accessibility of Frontier AI to developers and users, driven by both open source and closed-source technologies, has significantly increased in recent years. It remains to be seen how future AI systems will be deployed and how access will be controlled.
Sub-uncertainties
Market structure
The current Frontier AI market is dominated by a small number of private sector organisations, including technology giants such as Meta and Google DeepMind . Their dominance is evidenced by the fact that 76 AI startups were acquired by five technology companies between 2010 and 2021 , with Apple and Google responsible for 60% of these acquisitions.
A recent report commissioned by the UK Department for Science, Innovation and Technology (DSIT), estimated that large firms generated 71% of all UK AI revenues despite comprising only 4% of the total number of UK AI firms . The future market structure of the Frontier AI ecosystem is uncertain, however continued market concentration could pose risks around unequal access to, and impacts from, AI. This is particularly true if the leading models continue to be closed-access. Open source developments could help to diversify the Frontier AI market, although are often dependent on big tech corporations .
Access
Over the last year, the public release of generative AI systems, including LLM chatbots such as OpenAI’s ChatGPT, has paved the way for rising public access to and use of AI applications. ONS data from May 2023 indicate that 5% of adults in the UK use AI a lot and 45% use AI a little in their day-to-day life . A third of adults also report using chatbots in the past month. However, access to Frontier AI services is increasingly being controlled using paywalls and the rise in the number of people experimenting with AI tools for fun appears to be slowing. Following reports in February 2023 suggesting that ChatGPT was the fastest-growing app in history , visits to the ChatGPT website subsequently declined for three consecutive months between May and August 2023 . Analysis has also suggested that the average amount of time spent by users on the website declined monthly from March through to August 2023.
Closed vs open source models
The majority of Frontier AI models released to date by the leading companies, such as OpenAI, Google DeepMind and Anthropic, have been closed access. The underlying code and parameters such as weights and biases have remained confidential. However, the increasing availability of data and computational resources has bolstered the open source AI community.
In May 2023, an unverified leaked memo, allegedly from an unnamed senior software engineer at Google DeepMind, expressed concern that open source models were quickly catching up to the capabilities of closed-source models produced by the likes of Google and OpenAI . Meta have the resources to compete with other Frontier organisations and continue to release open source models of greater scale and capability (e.g. Llama2 ). Other open source models such as Falcon are also showing signs of closing the gap to the leading closed models.
Making a system accessible to many developers or users may increase the risk of misuse by malicious actors compared to private systems. However, open systems support scrutiny by a larger developer community who could spot biases, risks, or faults, and systems can be tailored for specific user needs. Alternatively, if the Frontier AI ecosystem comes to be dominated by one, or a small number of closed models which have undetected biases, this could heighten the potential for safety failures (e.g. prompt injection attacks ) or undetected biases being propagated across multiple use cases.
Whilst it seems almost certain that open source models will continue to improve in future, it is uncertain whether they will be able to keep pace with the closed models released by Frontier labs. If capability continues to scale with data and compute, it appears unlikely that the open source community will be able to access the same levels of funding, data and compute that a select few companies have access to.
Levels of investment
Between 2013 and 2022, global corporate investment in AI has increased by 1200% from ~$15 billion to ~$190 billion (Figure 8). Although year-on-year investment dipped by 30% from 2021 to 202232, the overall upward trend in AI investment is expected to continue, with spending on AI-centric systems estimated to exceed $300 billion in 2026 . The global annual value of venture capital funding in AI has increased by 25x, from $3 billion to $75 billion between 2012 and 2020 . Whilst increases seem likely to continue in the short term, the medium-term trajectory is more uncertain, with potential for ongoing increases, slowing or volatility.
Figure 8: Global Corporate Investment in AI by Investment Activity, 2013-22. Source: NetBase Quid, 2022 | Chart: 2023 AI Index Report Data from this graphic shown below.
Data from this graphic shown below:
Global Corporate Investment in AI by Investment Activity 2013-2022, Total Investment (in Billions of U.S. Dollars)
Date | Merger/Acquisition | Minority Stake | Private Investment | Public Offering | Total |
---|---|---|---|---|---|
2013 | Number not shown | Number not shown | Number not shown | Number not shown | 14.57 |
2014 | Number not shown | Number not shown | Number not shown | Number not shown | 19.04 |
2015 | Number not shown | Number not shown | 13.35 | Number not shown | 25.43 |
2016 | 13.05 | Number not shown | 17.13 | Number not shown | 33.82 |
2017 | 24.68 | Number not shown | 25.72 | Number not shown | 53.72 |
2018 | 21.89 | Number not shown | 43.1 | 12.62 | 79.62 |
2019 | 31.91 | Number not shown | 55.09 | Number not shown | 95.57 |
2020 | 26.06 | 46.06 | 61.61 | 13.01 | 146.74 |
2021 | 119.66 | Number not shown | 125.36 | 29.1 | 276.14 |
2022 | 83.35 | Number not shown | 91.86 | Number not shown | 189.59 |
Compute constraints
Development of Frontier AI systems has become highly dependent on access to significant compute resource for training AI models, which itself is dependent on the semiconductor supply chain , . Restricted access to cloud computing services could impact future AI model training, development and deployment, as could increases in cost or disruption of access to next generation hardware (e.g. Nvidia’s GH200 chip ). In particular, rising GPU demand coupled with the concentration of the market is already resulting in chip shortages, impacting the development and deployment of Frontier AI systems . The production of semiconductors is concentrated in Asia, with Taiwan alone producing 60% of the global semiconductor supply, and 90% of the most advanced chips . Geopolitical tensions are already influencing the supply of hardware critical to AI systems and there is potential for this to continue to impact developments at the Frontier of AI (see Geopolitical Context section).
Figure 9 – Training compute used for notable AI systems, measured in petaflop (1015 floating point operations estimated from AI literature, though with some uncertainty). Source: Epoch (2023)
Data from this graphic shown below:
Training compute used for notable AI systems, measured in petaflop (E+/-)
Date | E-14 | E-12 | E-10 | E-8 | E-6 | E-4 | E-2 | E+00 | E+02 | E+04 | E+06 | E+08 | E+10 |
---|---|---|---|---|---|---|---|---|---|---|---|---|---|
1960-1973 | N/A | 1 | N/A | N/A | N/A | N/A | N/A | N/A | N/A | N/A | N/A | N/A | N/A |
1973-1987 | N/A | N/A | N/A | N/A | 2 | 1 | N/A | N/A | N/A | N/A | N/A | N/A | N/A |
1987-2001 | N/A | N/A | N/A | N/A | 2 | 3 | 3 | N/A | N/A | N/A | N/A | N/A | N/A |
2001-2014 | N/A | N/A | N/A | N/A | N/A | N/A | 3 | 9 | 10 | 7 | N/A | N/A | N/A |
2014-2028 | N/A | N/A | N/A | N/A | N/A | N/A | N/A | N/A | 5 | 17 | 24 | 27 | 7 |
Figure 10 – The number of transistors that fit into a microprocessor 1971-2021. Source: Karl Rupp, Microprocessor Trend Data (2022)
Data from this graphic shown below:
Date | Transistors by microprocessor (E+) |
---|---|
1970 | E+3.3 |
1980 | E+4.6 |
1990 | E+6.1 |
2000 | E+7.3 |
2010 | E+9.4 |
2020 | E+10.6 |
Furthermore, there is uncertainty over whether the increases in compute that have powered recent advances in AI are sustainable in the long term. The amount of compute used in training runs for AI models has been increasing exponentially over the last decade , as illustrated in Figure 9 . Such training runs are extremely costly and energy-intensive, as is the running of servers to deploy Frontier AI models. The environmental impact of training and deploying AI in this way is likely to be challenging from a sustainability point of view and could influence future development .
In addition, Moore’s Law, the observation that the number of transistors on an integrated circuit doubles approximately every 2 years, has held true over the past fifty years (Figure 10 ). However, experts are becoming increasingly concerned that this relationship will soon be at an end as we reach the limit of transistors to operate in increasingly smaller circuits at higher temperatures . At this point, firms may find it increasingly difficult to reduce computational costs, creating uncertainty over the potential knock-on effects on developments in AI. The emergence of cost-effective alternative compute technologies, designed with AI in mind, could potentially provide solutions.
Access to data and other inputs
Both the quantity and quality of data available for training and fine-tuning AI models is a crucial consideration for the development of current and future AI systems. Being unable to access the same scale and quality of training data as some of the leading technology corporations is likely to hinder AI start-ups in their development of new systems and capabilities, giving Frontier firms a competitive advantage .
There are concerns that the increasing demand for high-quality language data may soon outstrip supply, with some estimating that these data sources may be exhausted as early as 2026 . Additionally, there is the potential for existing datasets to become “poisoned” over-time by AI generated content, including mistakes of previous AI models . This has been referred to as “model collapse” by researchers and could reduce the performance of future AI systems , . However, there is a vast amount of non-language data yet to be used, including photos and videos. Some organisations are also exploring the use of computer-made “synthetic” data , although its utility for training future models remains uncertain.
One of the other main concerns with regards to data for AI models is the issue of intellectual property. Several lawsuits have already been brought against companies including Microsoft, OpenAI and Stability AI, claiming that the use of web-scraped data to train their models was an infringement of copyright laws . There is uncertainty over the impacts that the outcomes of these legal challenges could have on the AI industry. Were access to high-quality data sources to become restricted, such that they were only affordable to big tech companies, it could result in a further increase in the concentration of market power, restricting competition and impeding the open source community. The development of more capable models in future could be hampered or become significantly more costly.
System design and complexity
Some AI systems can be run on local devices, containing the necessary algorithms and data for specific use cases. For larger more complex systems, data and processing is sent to external machines for task execution. Due to significant compute requirements, some small start-ups have pooled their computing resources77. The potential future impacts of pooling resources are uncertain but there is the potential for significant leaps in capability that could challenge the leading players. It appears unlikely that the large technology firms developing Frontier models will follow suit, in order to try and maintain a competitive advantage.
Researchers are experimenting with the use of combinations of different foundation models to enhance capabilities and there is the potential for this to continue with both closed and open source models to increase functionality49. Frontier models can also be modified using “wrappers” to fine-tune the system for a particular function, or to facilitate increased autonomy and agency, as discussed previously in the Capability critical uncertainty .
Relationships to other critical uncertainties
This section provides a few key examples how different outcomes for the ‘Ownership, access, and constraints’ critical uncertainty could be correlated with outcomes on other critical uncertainties, including how these correlations might be contingent on other factors. This is based on outputs of the scenario development workshops (Chapter 2).
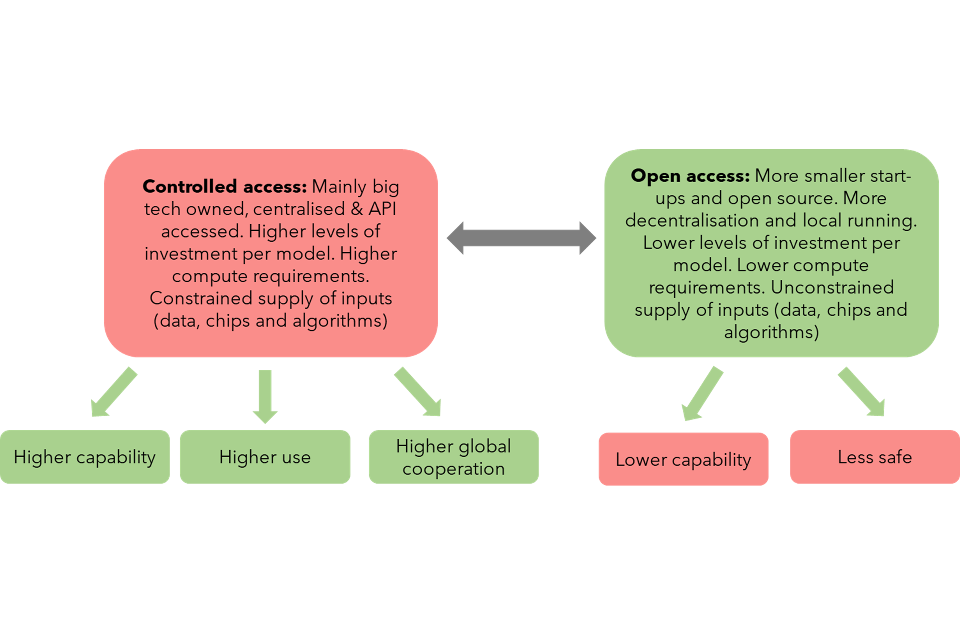
Controlled access: Mainly big tech owned, centralised & API accessed. Higher levels of investment per model. Higher compute requirements. Constrained supply of inputs (data, chips and algorithms) Experts consider controlled access to be most strongly coherent with: Higher capability: More compute is expected (at least in the short term) to continue to produce more capable models. Higher use: Big firms are generally better at driving mass adoption of tech. Higher global cooperation: Cooperation is difficult if accessibility is uncontrolled. |
Open access: More smaller start-ups and open source. More decentralisation and local running. Lower levels of investment per model. Lower compute requirements. Unconstrained supply of inputs (data, chips and algorithms) Experts consider open access to be most strongly coherent with: Lower capability: In a world with lower capabilities, the market will likely be more diverse, with start-ups and open source developers catching up to big tech. Less safe – The dispersed nature of open source makes it harder to regulate, although can support greater scrutiny for spotting biases, risks and faults. |
Wildcards
Wildcards are radical outcomes associated with each critical uncertainty. These are considered possible, but not likely, on a 2030 timescale. Policy makers could use these as possible ‘shocks’ to test their plans against (see Chapter 6).
Leaks of Frontier models
A cyber security breach of the leading AI firm has resulted in the unrestricted public access of their proprietary Frontier AI models. This results in any sufficiently resourced and competent user having the freedom to deploy a highly capable AI model as they choose, including across a range of unsuitable and malicious uses.
Stifled AI innovation
Seeing the profit potential behind AI and the threat from open access AI, companies work to create patents and copyrights to maintain their market position. The pace of growth slows and becomes more product focused. AI software prices increase, and it becomes more constrained and safer as companies fear reputational damage due to AI malfunction.
4.4 Safety
Overview
The development of increasingly capable AI systems has heightened interest in ensuring that they can be deployed and used safely and responsibly. AI safety encompasses a range of social and technical considerations and challenges. Technical measures to monitor, evaluate and report model faults and shut down systems are all areas of active research but there is uncertainty over how effectively such systems can be designed and implemented.
There also remains significant uncertainty over the alignment of future AI systems to societal values and who should decide which values to embed. Industry, academia, civil society, governments, and the public all have an important role to play in tackling the social and technical challenges and consequential safety risks presented by increasingly capable Frontier AI systems. But exactly how the perspectives of these groups will influence the development of future AI safety measure remains uncertain.
Defining AI safety
The term “AI safety” can mean different things to different communities, with experts outlining several schools of thought to us, including:
- The range of risks and dangers that AI systems could pose, particularly to the safety of humans.
- Upstream, technical evaluations of AI safety, using a mix of theoretical measures and analytical methods.
- How precisely AI models operate within set constraints, including their tolerances to faults and errors.
- Being able to use AI safely for clinical practices and applications.
- Responsible AI, including considerations of bias, ethical principles, and the differential impacts of AI systems for different groups of people.
AI safety is a complex and multi-faceted socio-technical challenge that cannot be resolved with technical interventions alone. Measures will need to incorporate considerations of all of the elements above into a broader framework of governance, processes, and policy that considers people, systemic effects and how risks emerge in practice , . The rest of this section outlines key sub-uncertainties around how future Frontier AI systems can be developed and used in a safe way.
Sub-uncertainties
Controllability
A key factor determining the safety of future AI systems will be our ability to control their behaviour. Evaluation is a key element of AI safety and controllability – testing an AI system’s responses to a range of prompts and contexts before it is made publicly available . This can enable developers to implement technical guardrails to restrict certain outputs of an AI system, which can contribute to ensuring it is deployed safely. For example, developers have attempted to use safety guardrails that engineer models to refuse to respond to prompts which could otherwise be used to generate harmful content .
Current guardrails can often be circumvented. For example, current Frontier AI systems are still prone to errors and their results often need to be assured by a human before being used. There have also been instances of LLMs being manipulated to generate misinformation and harmful, inaccurate content, demonstrating that there is still more research required to develop robust evaluation measures and safety assurances.
Post-deployment monitoring and evaluation of the responses generated by AI systems is a crucial feedback mechanism for refining and improving the safety and controllability of subsequent iterations of the system. In future, could rigorous evaluations of model safety and risk be implemented as a precondition for deployment?
Another technical measure that has been proposed to maintain controllability is the development of systems that allow humans to shut down AI models . However, there is uncertainty amongst experts over the technical feasibility of designing “kill-switches” or tripwires, largely due to concerns that future Frontier AI models could develop strong incentives for self-preservation and pursue goals to avoid being switched-off .
Whether Frontier AI systems could exhibit such behaviour in future will depend on uncertain factors such as their levels of agency, autonomy and alignment with human values and goals. If Frontier AI systems become irreversibly embedded into society, will it be as simple as switching them off if something goes wrong?
Alignment/potential for bias
A key element of AI safety is ensuring systems achieve goals that are in line with societal values. This should incorporate both human values and legal principles, such as fair process and proportionality . Alongside the technical challenge of how to embed values effectively into AI models and monitor success, there is the important question of how these values should be defined and by whom.
Companies developing Frontier AI are already embedding values and limitations into their models. However, current models have been shown to amplify existing biases within their training data, including in models developed to aid recruitment, which were shown to produce sexist responses. Training on large swathes of UK and US English internet content can mean that misogynistic, ageist, and white supremacist content is overrepresented in the training data12.
Contextual understanding of the training data is not explicitly embedded within these models. Without fine-tuning, they can fail to capture perspectives of underrepresented groups or the limitations within which they are expected to perform. Techniques such as reinforcement learning from human feedback (RLHF) are currently being used to improve the alignment of LLMs with the values of their users .
Alongside the uncertainty over who should decide on the values to embed, there is also uncertainty over the risks that future AI systems could present if they become misaligned. This could occur if a system develops the capability to pursue an objective in unintended ways that are not in line with the limitations embedded during development and more broadly, moral or ethical norms of human society. Measures to improve the controllability of AI systems are likely to be crucial for detecting and preventing misalignment in future. The form that these measures could take remains highly uncertain.
Model interpretability
One particular technical challenge to ensuring AI safety is the limited interpretability and predictability of the large neural networks underpinning Frontier foundation models. These models have frequently been referred to as “black-box” systems due to their complex and often opaque inner working mechanisms. Even those who build the systems cannot fully explain the inner workings of LLMs or predict the next steps in their evolution with certainty. Currently, this can make it difficult to assess the safety of systems and the potential for novel risks. The limited interpretability has also likely slowed the adoption of AI tools in some sectors, such as healthcare .
Given that the neural networks underpinning Frontier AI models are loosely based on structures in the brain, continued research in the neuroscience sector has the potential to increase understanding of deep learning mechanisms and improve the interpretability of future AI systems. , The field of explainable AI incorporates a range of methods and techniques to explain and present the behaviour of models in human-understandable terms , .
Changes in deployment context
Evaluation is the process of testing a model’s response to as representative as possible a set of tasks and deployment contexts. However, as models become more general, it will become more and more difficult to evaluate their responses to the full range of applications they could be used for. Universally agreed metrics to measure particularly dangerous or helpful characteristics could help, but do not yet exist. In some cases, it is unclear even what a suitable metric would look like.
Furthermore, if models are released open source (with the model weights freely available), it will not be possible for the original developer of the model to control deployment post-release. If advanced models are deployed in new and unfamiliar contexts, beyond the expectations of the original developers, their responses could be unpredictable or harmful. It is uncertain how avoidable this is.
Control over critical systems
Carefully considering which tools a model can access, and therefore the threats it could pose, should be a key element of AI safety. Applications of AI across critical systems, such as the energy sector, has the potential to pose a range of beneficial impacts. For example, AI can play a role in optimisation of energy consumption and anticipation of network malfunctions49. However, using AI to control critical systems such as nuclear weapons, power plants and telecommunications, could pose data and cyber security risks, as well as serious risks to human safety. Overreliance on AI in future could lead to system failure or unintended consequences that are challenging to control.
The US has recently highlighted the importance of maintaining human control over military uses of AI whilst in 2022, the UK laid out their position in the Defence Artificial Intelligence Strategy . However, there is uncertainty over the degree to which other countries will follow suit, which could have significant safety and geopolitical implications.
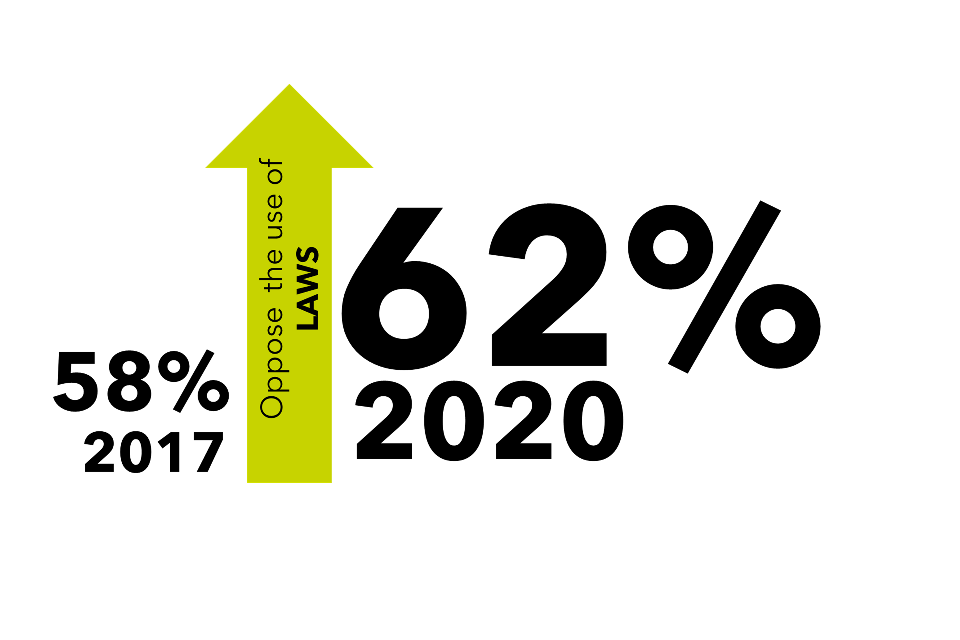
An image depicting the percentage of nations opposed to the use of LAWs, 58% in 2017 – 62% by 2020.
The use of AI in autonomous weapons systems is developing rapidly, raising concerns they could be used against a wide range of targets, including by terrorist groups . Resistance to the use of lethal autonomous weapon systems (LAWS) has been rising. In a 2020 survey across 28 countries, 62% of respondents said they oppose the use of LAWS, compared to 56% in 2017 . Governance and regulation of LAWS remains a subject of ongoing debate globally with uncertainty over the future regulatory landscape.
4.5 Relationships to other critical uncertainties
This section provides a few key examples how different outcomes for the ‘Safety’ critical uncertainty could be correlated with outcomes on other critical uncertainties, including how these correlations might be contingent on other factors. This information was developed using outputs of the scenario development workshops (see Chapter 2).
Less safe systems: Safety systems developments are slower than AI developments. AI systems are deployed in new contexts before full evaluation of performance. Models are less interpretable. Models are aligned to a limited set of societal values and can make biased decisions or output harmful content. AI has been embedded into certain critical systems, with utility trumping safety. Experts considered less safe systems to be most strongly coherent with: Open access: If systems are more accessible and less controlled, it will be easier for bad actors to misuse them and harder for authorities to monitor. Higher capability: Safety regulations tend to be playing catch up to high capability. |
More safe systems: Safety systems are reliably implemented and keep pace with developments and deployment context. Models are aligned to a widely accepted set of societal values. AI has only been embedded in critical systems where there is confidence in the reliability of safety systems. Experts considered more safe systems to be most strongly coherent with: Higher use: The public and businesses will be more likely to use AI systems that are proven to be safe. Higher global cooperation: Technology development is global. Safety is more likely to be assured if safety processes are agreed globally. |
Wildcards
Wildcards are radical outcomes associated with each critical uncertainty. These are considered possible, but not likely, on a 2030 timescale. Policy makers could use these as possible ‘shocks’ to test their plans against (see Chapter 6).
AI activists
With continued personalised anthropomorphised AI in use, humans become more and more attached to AI assistants or software. As AI agents become more capable at meaningful communication with humans, there becomes a growing movement looking to not only protect human safety but AI safety too. Humans end up in a debate about sentience of AI and how AI should be treated. There is also a concurrent increase in conspiracy theories that this advocacy is a result of AI manipulating humans leading to anti-tech vandalism and crime increasing.
AI alignment holds up a mirror to human contradictions
Advanced AI systems are successfully aligned with human values, but they quickly highlight the internal contradictions within these. These contradictions make it hard for AI systems to know how to proceed with certain decisions. For example, an AI system learns about animal welfare practices from the way humans treat pets, then highlights inconsistencies with how livestock are treated when used in agricultural applications. The model’s designers are left with a dilemma – how to muddle through with indecisive AI when they are unlikely to be well placed to resolve long-standing moral debates?
4.6 Level and distribution of use
Overview
The level and distribution of use of AI across society will be a key determinant of how AI tools develop and what impact they have, with societal uses of AI influencing development, and vice versa. Evidence is emerging around the public’s awareness of AI and how this varies across socioeconomic and demographic groups. But there is significant uncertainty over how this will change over time and the extent to which public views will be considered in the development of AI systems.
As AI capability improves systems will be increasingly integrated into private and public services, and people’s daily practices. This integration could lead to a range of interactions between people and AI systems. Examples of this includes engaging with anthropomorphised AI assistants, AI invisibly making tasks easier and more efficient, and humans being subject to automated decision making and job displacement.
Adverse impacts from malicious use of AI in areas such as disinformation, cyber-attacks, and fraud will likely grow. However, their future severity and frequency remains uncertain, as does the extent to which this will cause a public backlash against AI.
Sub-uncertainties
Integration into daily practices of the public
AI and machine learning systems are already integrated into many daily practices, including communication (social media, predictive text), cultural activities (streaming), shopping (recommendations, customer service chatbots), travel (navigation apps), security (facial or fingerprint authentication), and life admin (virtual assistants). This continues to grow, for example companies such as ASOS and H&M partnering with Microsoft and Google respectively to use AI to personalise the user experience and improve efficiency by predicting user demand.
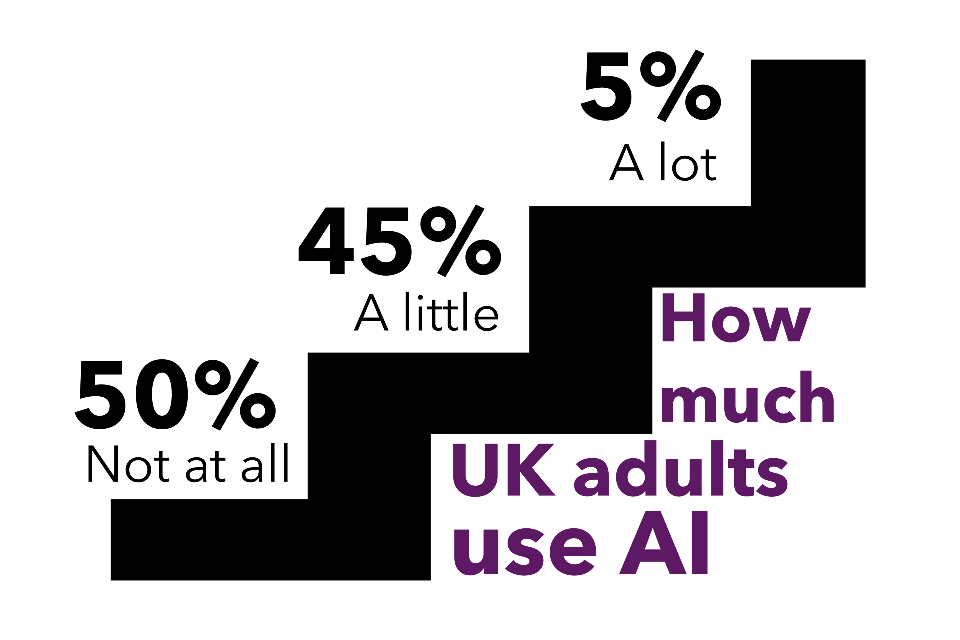
An image representing the percentage AI use in UK adults, 50% not at all, 45% a little, 5% a lot.
This is likely to continue to increase in line with AI capability and the ability of businesses to integrate AI systems into services (see below).
Many of these applications of AI are invisible to users , which contributes to a gap in awareness of AI use across the tools and services people use in their daily lives.
In a 2019 study, researchers interviewed members of the public and tech executives and found a significant gap in awareness of AI use across a wide range of services and applications (Figure 11). This was a US study and results may have changed since 2019 but it illustrates a gap between how much AI is used and how much people perceive AI in use.
Figure 11 –The difference between the amount that AI applications are used by the general public and tech executives. Source: 2019 Edelman AI Survey Whitepaper.
Data from this graphic shown below:
Table depicts the difference between the amount that AI applications are used by the General Public and Tech Executives
Voice Assistants | Robots/self working machines | Image Recognition | Natural Language Processing | Computer Visions | Drones | Text Recognition | Traffic and Navigation Apps | Ride Hailing Apps | Satalite Radio | Gaming Consoles | None of These | |
---|---|---|---|---|---|---|---|---|---|---|---|---|
General Population | 65% | 63% | 52% | 37% | 38% | 48% | 45% | 43% | 21% | 18% | 28% | 5% |
Tech Executives | 74% | 72% | 61% | 56% | 46% | 46% | 55% | 45% | 31% | 20% | 35% | 1% |
Furthermore, a 2023 ONS survey found that 5% of adults reported using AI a lot, 45% a little and 50% not at all. Given the range of invisible uses of AI outlined above it seems plausible that some respondents didn’t consider these as ‘using AI’ when answering the question. Indeed, a more recent ONS survey found that only 17% of adults say that they can often or always recognise when they are using AI.
People may associate ‘using AI’ with communicating with an AI agent or assistant, which is set to become an increasingly common practice . The rise of LLMs is set to accelerate this in the near term as the technology starts to enable simulation of realistic human characters. This is also prompting consideration of the legal and social consequences of anthropomorphised AI.
Overall, the scale, pace, and nature of growth in AI use is uncertain. To what extent will entirely new use cases emerge and grow? Will there be more growth in ‘invisible’ applications, or in those where users are consciously aware they are using AI? How might future concerns over automated decision making or other negative perceptions of AI moderate growth? These uncertainties are explored further below.
Level and equality of public AI access, knowledge and skills
Access to, knowledge of, and the skills to use future AI systems are important factors in determining whether members of the public experience the benefits of these systems. Public understanding of AI is growing, although is still limited to a minority.
A recent ONS publication suggested that the percentage of adults reporting they had heard of AI and could explain it in detail increased from 10% in 2022 to 19% in 2023 (Figure 12). However, the same survey showed that experience using AI was lowest amongst older people, illustrating inequalities that exist in access, knowledge, and skills.
Figure 12 – Proportion of adults in the UK’s awareness to AI, 27 June to 18 July 2022 (PADAI) and Great Britain, 4 to 14 May 2023 (OPN). Source: Opinions and Lifestyles Survey from the Office for National Statistics and Public Attitudes to Data and AI from the Office for AI.
Data from this graphic shown below:
Percentages of AI Awareness and Understanding
Never heard of AI | Heard of AI but could not explain what it is | Heard of AI and could give a partial explination | Heard of AI and could explain in detail | |
---|---|---|---|---|
PADAI, 2022 | 11% | 32% | 45% | 10% |
OPN, 2023 | 9% | 19% | 53% | 19% |
More generally, there is a socio-economic ‘digital divide’, driven in part by a lack of access to hardware and the internet. As of 2020, around 6% of households in the UK had no internet access , and poor internet connectivity affects around 40% of people living in rural areas . As AI-based services require access to devices and digital connectivity, to what extent will growth in AI use, reinforce, or exacerbate this divide?
Leading AI tools, which were launched as free products, can now only be accessed by paying customers2. This could further restrict access for those in lower socio-economic groups. There is uncertainty over whether ongoing efforts to improve access and digital literacy will narrow these inequalities across demographic groups, or whether they will persist, or even grow.
UK professional AI skills
How successfully UK businesses develop and use AI systems will depend on the level of professional AI skills in the economy.
A 2021 study highlighted the imbalance between the supply of and demand for data scientists in the UK, with the supply from universities unlikely to exceed 10,000 per year, and at least 178,000 unfilled data specialist roles . This demand is growing, with research showing that in the last ten years demand for AI related jobs has tripled . To help address this, the UK is increasing public funding for PhD research into AI and industry-funded master’s degree schemes , but there remains uncertainty over how well this will keep up with demand.
One additional challenge for the UK could be a brain drain due to high demand for AI skills in other countries, with a 2023 World Economic Forum (WEF) report suggesting global demand for AI and machine learning specialists will grow by around 40% over the next 5 years .
This could be associated with a brain drain of AI researchers from academia to industry. Research has shown that the increasing participation of the private sector in AI research has seen an accompanying rise in the number of researchers moving from academia into industry. Researchers seem to be moving to work for large technology companies such as Google due to greater access to state-of-the-art data, research infrastructure , and increased funding.
This brain drain may limit the availability of experts to support AI development for public good, such as improving use in public services. It may also see researchers move out of the UK to be closer to areas of innovation such as Silicon Valley.
It is not only the total availability of people with AI skills that matters to how AI develops in the UK – the demographic make-up of this group is also important. Certain groups (including women, people with disabilities, and those from minority ethnic backgrounds) are underrepresented in data-related jobs and experts suggest this lack of workforce diversity could affect efforts to minimise bias in AI systems .
If AI systems reflect the opinions of those who create them, will this amplify inequalities?
Integration into business practices, products, and services
The extent to which businesses integrate AI systems into their practices, products and services will be an important factor in determining use of AI by both customers and employees. Business use cases appear to be growing, with 35% of global companies surveyed by IBM in 2022 reporting having deployed AI . Research conducted by the World Economic Forum in 2023 also found that 75% of the world’s leading companies are in the process of adopting AI and big data systems147.
A key reason for this growth is emerging evidence on productivity enhancements from AI systems. For example, a 2023 pre-print of a study by Harvard Business School and Boston Consulting Group suggested the use of AI across 18 business tasks to have productivity impacts including a 25% increase in speed of task completion and a 40% increase in quality of results, although some tasks outside current capability saw AI underperform against humans. Whilst these results appear to be significant, there will remain uncertainty over the productivity impacts of Frontier AI until a more comprehensive and robustly peer reviewed evidence base is developed.
This improvement in AI performance is causing some businesses to replace human workers with AI systems, with BT planning to replace around 10,000 jobs with AI by 2030 and IBM pausing hiring on back-office jobs that could be replaced by AI .
A 2023 OECD study found that 27% of jobs are at high risk of automation related to AI , with the rise of LLMs particularly affecting white collar administrative jobs . Furthermore, recent cross-country analysis by the International Monetary Fund comparing the UK to the US, South Africa, India, Colombia, and Brazil, showed that the UK has the largest proportion of workers exposed to AI and that 52% of UK workers are engaged in occupations with high AI complementarity . Whilst historical evidence suggests many new jobs could be created through growing AI use , , some experts think AI could differ from previous technological revolutions. This is due to there being less barriers to expanding software across industries and job types compared to changes in physical machinery, as evidenced by the rapid and widespread uptake of ChatGPT .
A key uncertainty is the balance between AI use to augment human skills (e.g. by automating certain tasks) and AI fully replacing specific jobs . This is interdependent with uncertainties around AI capability and level of AI-related skills in the economy (see above section). Furthermore, it’s uncertain when changes will happen. Some organisations like PwC suggesting that over the next 2 decades there will be three waves of changes (Algorithm, Augmentation, and Autonomy) in the labour market driven by AI. Others argue AI may lead to increases in unemployment in the short-term but not the long-term .
Whilst there is growth in AI use it is predominantly amongst large and globally facing companies150, data from the ONS suggests AI use in the majority of UK businesses remains low, with only 13% of businesses specifying at least 1 planned use 81. 81% of UK businesses also report no plans to adopt AI technologies in the next 3 months, and that they have either not attempted to adopt AI technologies or experienced any barriers to adoption in the past 3 months .
Will motivation for businesses to adopt AI increase? Will they develop the skills to deploy AI systems? What barriers might they encounter? Will new market entrants overtake incumbents who are slow to adopt the technology? Overall, significant uncertainty remains about how quickly use will grow outside early adopters, and how this will affect workers and customers.
Public involvement in shaping how AI is used
Public involvement could play a critical role in shaping the use of AI in wider society. Experts we spoke to suggested engaging the public in AI-related decision-making processes may help ensure accountability and harms caused by AI are minimised. Ways to involve the public could include:
- tracking public attitudes and using this information to shape AI systems and policies;
- policy makers or businesses running public engagements (see Chapter 5) or dialogues to develop in-depth qualitative insights on specific issues; and
- mechanisms for the public to provide feedback or complain if an AI system causes harm or makes a disputed decision.
There are several methodologies or frameworks that could inform this, such Ethics, Transparency and Accountability Framework for Automated Decision-Making and the Framework for Responsible Innovation .
Some leading AI labs are making efforts to involve the public in decisions about the rules AI systems follow , but questions remain over whether such processes should be led by businesses or whether it would be more appropriate for elected governments or other independent organisations to facilitate this.
Research by the Ada Lovelace Institute found the majority of the public supported greater AI regulation and would want clear procedures for appealing AI decisions. However, younger participants were more likely to say it was the role of the companies developing the technology to ensure it was safe while older participants favoured independent regulators taking this responsibility . Some level of future public engagement on AI use seems inevitable, but there is uncertainty over the scale, depth and breadth of this engagement, the extent to which it will be ongoing and what it’s impact will be.
Public sentiment
Public sentiment towards a technology can affect its uptake. One notable example is negative public sentiment towards Genetically Modified crops in the UK in the 1990s, where public sentiment towards it has been a factor in lower levels of use of the technology in the UK compared to other parts of the world . Such negative perceptions have not yet developed towards AI, with emerging evidence that people are mostly curious about the technology, and lower but roughly equal levels of excitement and worry .
In a 2023 survey, the Ada Lovelace Institute found that public sentiment is broadly positive about a range of AI use cases, which may encourage wider use and adoption, with concerns focussed on some specific uses of AI, such as driverless cars and autonomous weapons167. The ONS has also surveyed the public on their expectations of AI’s impact on society81, with most respondents expecting neutral to positive impacts. People’s expectations for personal benefit are more reserved with almost half of people surveyed neither agreeing nor disagreeing with the statement that AI will benefit them personally and 43% believing AI brings both equal risk and benefits137. However, there is uncertainy over how this will evolve over time – could instances of AI misuse, accidental harm caused by AI, or AI displacement of jobs start to stoke a public backlash against the technology?
Level of misuse of AI systems
Another uncertainty is the extent and nature of AI misuse by bad actors, including online groups, organised crime gangs, and hostile states. AI is already misused in various ways, including disinformation, causing harm to members of the public. For example, videos using ‘deepfake’ technology has been used to create fake news reports and events , such as a 2023 incident where AI-generated imagery of an explosion near the Pentagon briefly caused stock markets to decline .
Experts agree there is significant scope for existing misuses to grow in severity and frequency with increasing access to more capable AI systems. This is expected to increase the volume, velocity, and virality of disinformation operations as fake media generation becomes easier . The National Cyber Security Centre (NCSC) also warns that AI is likely to amplify existing cyber threats and attacks, stating that AI is already being used to develop more sophisticated phishing emails and scams, and that generative AI has the potential to create synthetic cyber environments for criminal activities like fraud .
Whilst AI is already helping to address biosecurity challenges, like understanding pathogens and developing diagnostics, there is also scope for such advances to enable new misuses in assisting biological or chemical terrorist plots. Collaborations Pharmaceuticals found in 2020 their AI-based Megasyn software could generate a compendium of 40,000 potentially toxic chemical substances when run overnight . Whether they would prove toxic when synthesised or not, this case study illustrates the potential risks from bad actors using AI to generate novel and unpredictable physical threats.
There is uncertainty over who will misuse Frontier AI as it becomes more capable and accessible, and at what scale. As well as capability, this will depend on uncertain societal and geopolitical factors like future levels of crime and conflict. There is also uncertainty over the impact this misuse will have on public sentiment towards AI.
Another uncertainty is the extent to which countermeasures will be able to prevent bad actors from successfully perpetrating such AI misuse. Whilst AI can create threats it may also be able to counter them, with it having the ability to assist in critically evaluating and fortifying national security . For example, countries such as Austria are using AI tools to help the public in detecting misinformation which may conversely raise trust in associated applications if they are consistently effective.
The NCSC identified173 cyber security opportunities from AI, as well as risks. Their report describes how AI is currently being used to detect known types of frauds and will be able to improve detection and triage of cyber-attacks, find system vulnerabilities, and generate more secure code. Will the cyber security community be able to secure the skills and investment needed to successfully implement these sophisticated countermeasures?
Other uncertainties, like future levels of trust in institutions, will affect the ability of authorities to manage misuse (and AI misuse could also exacerbate falling trust in institutions via disinformation). Key events for maintaining trust in institutions will likely be political elections where interference from AI misuse is expected to be prominent and may be publicly visible . What will the role of AI misuse be in upcoming elections, and what will be the long term implications?
Relationships to other critical uncertainties
This section provides a few key examples how different outcomes for the ‘Ownership, access, and constraints’ critical uncertainty could be correlated with outcomes on other critical uncertainties, including how these correlations might be contingent on other factors. This information was developed using outputs of the scenario development workshops (see Chapter 2).
Lower use: AI systems are less embedded in daily practices. Businesses develop few attractive AI- based products, and use of AI in these products is opaque to the public. Government and businesses ignore public views on AI-use decision making. This contributes to negative public sentiment on AI. Experts considered lower use to be most strongly coherent with: Open access: Open source systems may be more susceptible to misuse, which could result in more negative public sentiment towards AI. Less safe: The public and businesses are unlikely to trust systems with safety concerns. |
Higher use: AI systems are embedded in many daily practices. Businesses develop attractive AI- based products, and use of AI in these products is disclosed to the public. Government and businesses seek public views on AI-use decision making. This contributes to positive public sentiment on AI Experts considered higher use to be most strongly coherent with: Controlled access: Corporations are likely to be better at driving mass adoption of tech. More safe: The public and businesses are more likely to use systems that are considered safe. Higher global cooperation: Global cooperation on AI will help to avoid large scale misuse and build public trust. |
Wildcards
Wildcards are radical outcomes associated with each critical uncertainty. These are considered possible, but not likely, on a 2030 timescale. Policy makers could use these as possible ‘shocks’ to test their plans against (see Chapter 6).
Social dilution
As AI use increases, people become less sociable as they are better able to get their social needs met through anthropomorphised AI and tailored services. This increases social divisions in society and leads to less cooperation as both work and social activity becomes streamlined and minimised through AI influence.
A new opting-out movement
Some people become increasingly concerned about the growth in use of AI, in terms of a loss of privacy, autonomy, and human capability. This grows into a movement of people looking to opt out of AI-based services, losing out on economic and time-saving benefits, but claiming a greater sense of freedom and personal responsibility.
4.7 Geopolitical Context
Overview
The geopolitical context will affect AI development in a range of ways. This includes directly via global cooperation on AI governance, and indirectly through competition or conflict between nations. A lack of cooperation could lead to a duplication of investment, a lack of or incoherent regulation, and more limited evidence base with a divided research community.
Global efforts are being made to cooperate on AI governance, but it is uncertain what the level of global cooperation will be in 2030. Factors that could influence this include strengthening global cooperation on other global issues, like climate change, and rising international tensions between geopolitical blocks. The extent to which AI hardware supply chains remain global or are on-shored will also affect developments.
Sub-uncertainties
International cooperation on AI
Several global initiatives on AI have been established that could affect developers’ approach to designing and training models, how they are deployed, and how they are monitored, used and regulated once deployed. For example, all 193 Member States of UNESCO adopted a global standard on AI ethics, the “Recommendation on the Ethics of Artificial Intelligence” in 2021 .
Another initiative is the Global Partnership on Artificial Intelligence (GPAI), a collective of 29 international partners with a shared commitment to the OECD Recommendation on Artificial Intelligence, the first intergovernmental standard on AI, which is rooted in responsible governance of trustworthy AI . Partners include G7 countries, who also have their own operation, the Hiroshima AI process, which calls for global standards on AI governance while enabling variation among countries .
The EU has also proposed the AI Act, which seeks to classify AI systems by their risk level, and will determine the degree of regulation in the EU . The AI industry is increasingly involved in supporting development of regulations, particularly in the USA, where several leading AI organisations have agreed with government voluntary commitments on safety, security, and transparency.
Act/Regulation/Pledge | Countries involved | What is it? |
---|---|---|
Recommendation on the Ethics of Artificial Intelligence | All 193 Member States of UNESCO | A global effort to ensure that all developments in AI have strong ethical barriers for decades |
Global Partnership on Artificial Intelligence (GPAI) | 29 international partners with a shared commitment to the OECD Recommendation on Artificial Intelligence | Bringing together expertise from industries, international organisations and governments to foster strong scientific international cooperation |
The Hiroshima AI Process | Includes G7 countries | A call for global standards on AI governance while enabling variation among countries |
The AI Act | Members of the EU | Classifying AI systems by their risk level, and will then determine the degree of regulation in the EU |
The White House AI Bill of Rights | The United States of America | Principles and Practices to enforce safe design, use and deployment of AI systems to protect the American public |
Table 3: Summaries of AI acts, regulations and pledges
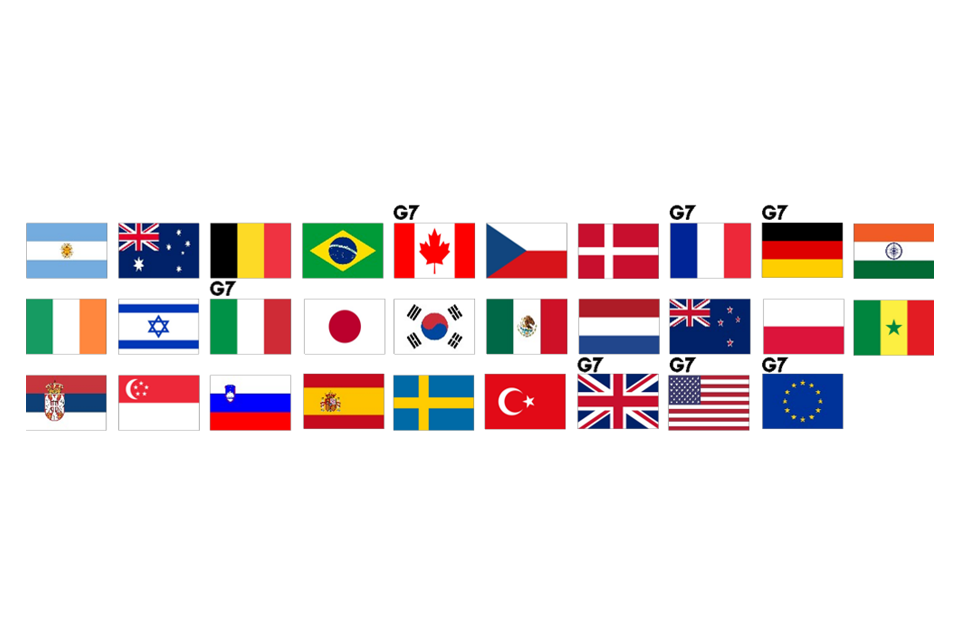
The flags of the 29 GPAI countries, Argentina, Australia, Belgium, Brazil, Canada (G7), The Czech Republic, Norway, France (G7), Germany (G7), India, Ireland, Israel, Italy (G7), Japan, South Korea, Mexico, Netherlands (G7), New Zealand (G7), Poland (G7), Senegal, Serbia, Singapore, Slovenia, Spain, Finland, China, UK (G7), USA (G7), EU (G7).
Figure 13. GPAI countries.
More recently, the UK hosted a summit focused on AI risks and safety, attended by representatives from over 28 nations, including the US, China, and the EU. This gathering focussed on establishing a mutual understanding of both the opportunities and the risks presented by Frontier AI. The culmination of these discussions was the Bletchley Park Declaration, a statement endorsed by all 28 participating countries that recognises the importance of managing potential AI risks through collaborative efforts. The agreement underscores a commitment to ensuring the safe and responsible development of AI. The consensus also highlighted a shared interest in maintaining international cooperation, with the participating nations agreeing to have France host the next summit .
Away from these big global initiatives, the relationship between the US and China - the 2 biggest state actors in AI - is particularly important. The US announced a ban, to come into effect in 2024, limiting US investment into China’s AI, quantum computing and advanced chips industries, citing national security risks, but. How this dynamic plays out will have significant implications for the level of cooperation on AI governance, and how this is balanced against economic and national security competition between the states.
Despite recent steps towards cooperation, it remains uncertain whether the global governance of AI will be more coordinated or fragmented between geopolitical blocks by 2030. The future level of cooperation between industry and government is also uncertain. This will be influenced by developments in the other sub-uncertainties outlined below.
Cooperation on other global issues, particularly climate change
Cooperation on one issue has previously acted as a catalyst for cooperation on others, and there is potential for the same to happen with AI. For example, following WWII there was a period of strong international cooperation, with a series of mutually reinforcing multilateral organisations and agreements, including establishment of the United Nations, the Bretton Woods system and the General Agreement on Tariffs and Trade.
A more recent example, which could affect wider international cooperation, is the Paris Agreement, a legally binding international treaty intending to limit global warming signed by 196 parties at the UN’s COP21 . Commitment from key actors (e.g. US, China) to the treaty is essential to maintain integrity; the US briefly left the agreement in 2020, before reinstating their inclusion in 2021 . China, Russia, Australia, and Brazil did not send their respective heads of state to COP27 in 2022 but remain committed to the Paris Agreement . In the face of worsening effects from climate change, will global leaders regroup to follow through on their plans, and will this have consequences for global cooperation on AI?
Level of conflict in the world
Global conflict will influence AI development and use, with more conflict generally making it harder to cooperate. The Russian invasion of Ukraine is the most notable ongoing war between 2 nations at the time of writing, and is showing no signs of resolution. However, the conflict appears to have strengthened relations between the West and allies, including in the Indo-Pacific region. 2023 saw the addition of Russian neighbour, Finland, to NATO, while Sweden is also expected to join imminently . NATO also strengthened ties with Japan, South Korea, Australia and New Zealand .
Elsewhere globally, current indications of conflict are primarily intra- as opposed to inter-state . Terrorist and criminal groups, as well as political militias, are the main culprits of conflict worldwide, with war deaths declining since the end of WWII. AI also has the potential to be employed in conflict or military applications, as well as the potential to stir conflict via competition (often referred to as an ‘arms-race dynamic’).
Future use of AI systems in combat situations is another uncertainty that could affect both the impacts of conflict and the wider development and use of AI systems. There is already some use of Lethal Autonomous Weapons (LAWs), for example in the ongoing Russia-Ukraine conflict . Experts suggest that developments in AI have the potential to transform military norms and strategy, creating a call for international ethical debate .
A recent Parliamentary Office of Science and Technology (POST) report on automation in military operations describes the potential for autonomous weapons systems to increase the safety of military personnel and civilians, and the overall efficiency of warfare . These systems can enable intelligence, surveillance, reconnaissance, data analysis, weapons systems, and uncrewed vehicles. There are many existing examples of these applications, although human oversight in usually mandated. Will this human oversight necessarily continue as AI systems get more advanced? The POST report also highlights the lack of international legislation specific to the use of AI in military applications, raising debate surrounding accountability and responsibility192.
It is difficult to predict the level of conflict in the world in 2030. If global tensions heighten and AI is increasingly used as a weapon, what impact will this have on the ability of nations to develop shared standards and agreements on AI?
Reliance on globalised supply chains versus re-shoring
AI hardware, particularly advanced semiconductors, is produced via highly integrated global supply chains. This involves moving components across continents multiple times before a final product is produced, which makes the process vulnerable to many potential disruptions . The high geographic concentration of aspects of the supply chain leaves the whole system vulnerable to single points of failure.
For example, the Tōhoku earthquake and tsunami in Japan, 2011, which led to the Fukushima nuclear power-plant meltdown, impacted 75% of the global supply of hydrogen peroxide and 25% of the global production of silicon wafers.
Consequently, multiple semiconductor fabrication plants were closed for several months. This reliance on a globalised supply chain also creates vulnerability to geopolitical tensions. Any conflict between key actors in the supply chain would have global consequences for semiconductor supply.
Over the last 30 years, East Asia has become dominant in semiconductor supply, particularly Taiwan, with the US market share falling significantly (Figure 14) . The US is pushing to rebuild market share, assisted by the CHIPS and Science Act, 2022, which invests over $50 billion in the US semiconductor industry. The UK also launched the National Semiconductor Strategy this year, which aims to grow the UK industry and improve the resilience of supply chains .
Data from this graphic shown below:
Percentage Share in Global Manufacturing Capacity
Date | Other | China | Taiwan | South Korea | Japan | Europe | US |
---|---|---|---|---|---|---|---|
1990 | <1% | <1% | <1% | <1% | 19% | 43% | 37% |
2000 | 2% | 1% | 22% | 15% | 15% | 25% | 20% |
2010 | 5% | 13% | 20% | 17% | 15% | 15% | 15% |
2020 | 6% | 16% | 21% | 21% | 15% | 10% | 11% |
2030 | 4% | 24% | 22% | 19% | 14% | 7% | 10% |
Figure 14 – Global Manufacturing capacity by location (%). Source: VLSI Research projection; SEMI second-quarter 2020 update; BCG analysis.
Greater access to manufacturing, R&D, and a skilled workforce for US-based companies could reduce vulnerability of supply both domestically and for US allies . However, this is likely to be challenging, particularly for advanced chips. Almost the entire global capacity for manufacturing the most advanced chips (<10 nanometre) is in Taiwan, accounting for 92% of this supply.
There is uncertainty over what geographical distribution of production will be in 2030, and what supply chain disruptions there could be between now and then. What impact could such disruptions have on the development of Frontier AI?
Relationships to other critical uncertainties
This section provides a few key examples of how different outcomes for the ‘Ownership, access, and constraints’ critical uncertainty could be correlated with outcomes on other critical uncertainties, including how these correlations might be contingent on other factors. This information was developed using outputs of the scenario development workshops (see Chapter 2).
Lower global cooperation: International partners withhold resources and take regulations in different directions. There are higher levels of global conflict and supply chain disruption. Experts considered lower global cooperation to be most strongly coherent with: Less safe: Experts suggested there are currently relatively low levels of cooperation, and also safety issues. It’s plausible these are related. High capability: It’s plausible that development of high capability systems could drive an arms race dynamic. |
Higher global cooperation: International partners share resources and align regulations. There are lower levels of global conflict and supply chain disruption. Experts considered higher global cooperation to be most strongly coherent with: Controlled access: It may be easier to cooperate on AI regulations if systems are already relatively controlled. More safe: More likely to have coherent global regulation, resulting in improved safety. Higher use: Global cooperation on AI will help to avoid large scale misuse and build public trust. |
Wildcards
Wildcards are radical outcomes associated with each critical uncertainty. These are considered possible, but not likely, on a 2030 timescale. Policy makers could use these as possible ‘shocks’ to test their plans against (see Chapter 6).
Global dissonance
Strong economic competition between states has workforce impacts as export controls are introduced and each country looks to develop their own supply chains and chips. Growing tensions lead to significant increases of malicious use. Safety and sustainability are disregarded as efforts are concentrated in building capability.
Technocratic societies
AI systems become more capable, infiltrating all aspects of daily life, including replacing many jobs. As nation states increasingly rely on AI systems, there are concerns that they are becoming beholden to technology companies. These companies find that they can now influence global events at least as effectively as nation states.
5. Scenario narratives
5.1 Introduction
This chapter describes our five scenarios for AI in 2030, providing narratives across the Critical Uncertainties, and a range of implications across the economy, society and for peoples’ daily lives.
Scope of the scenario narratives
The scenarios focus on Frontier AI – the most capable models at a given point in time. These models present significant uncertainty in terms of their future capabilities, how widely they might be used, and what their impacts might be. However, as average AI systems in 2030 could be significantly more capable than today, the scenarios also consider some developments away from the Frontier.
The narratives are also necessarily concise, focusing on the most notable or extreme differences between the scenarios. In all scenarios there will be a broad spectrum of AI capabilities, a spectrum of uses and misuses, and a spectrum of experiences for different groups of people. If we described all of this in detail, the scenarios would no longer be useful for policymakers.
Nor does the focus on the most notable issues in each scenario mean that these things don’t occur in the other scenarios, just to a lesser extent. Things that are likely to happen in all scenarios (important but more certain factors) are described in Chapter 3, above.
A vision of a positive AI future
As noted above, whilst the five scenarios contain some opportunities, they all present challenges to be resolved and imagine some risks materialising.
However, the evidence we have collected suggests that more positive AI futures are possible, which could see many plausible and wide-ranging benefits from AI. A selection of the positive impacts that AI could have in a range of areas are described below.
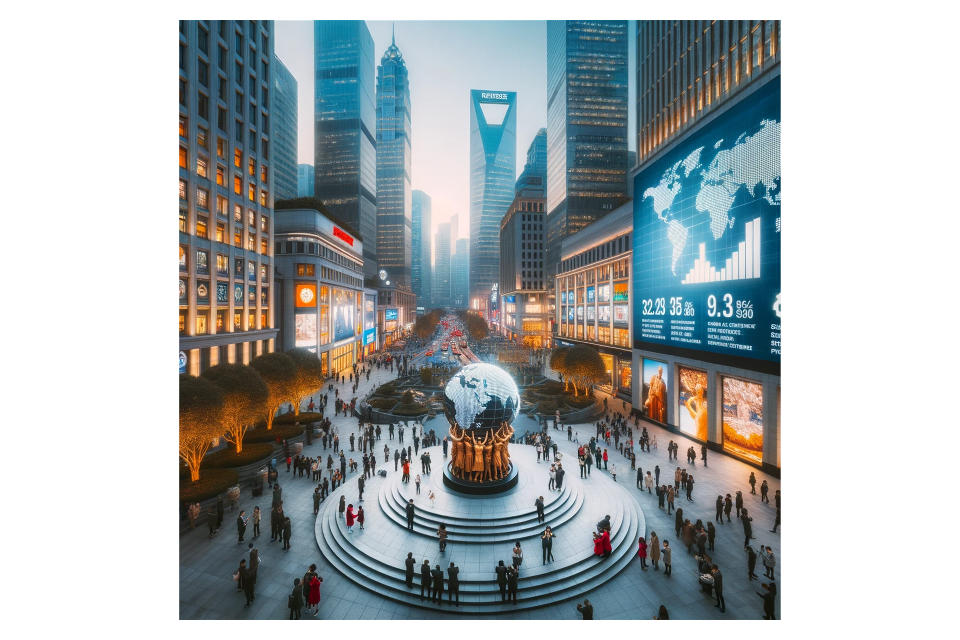
An AI generated image depicting a busy town square in a futuristic city.
Figure 15 – Generated with Dall-e 3. We prompted the tool to depict a futuristic high-tech city.
Economy
Whilst technological change has historically led to disruption for some, it tends to create more jobs than are displaced in the long run. Although the long-term effect on labour markets remains uncertain and the subject of debate, AI and automated technologies are already being adopted by businesses across the UK, with net positive impacts on job creation being reported.
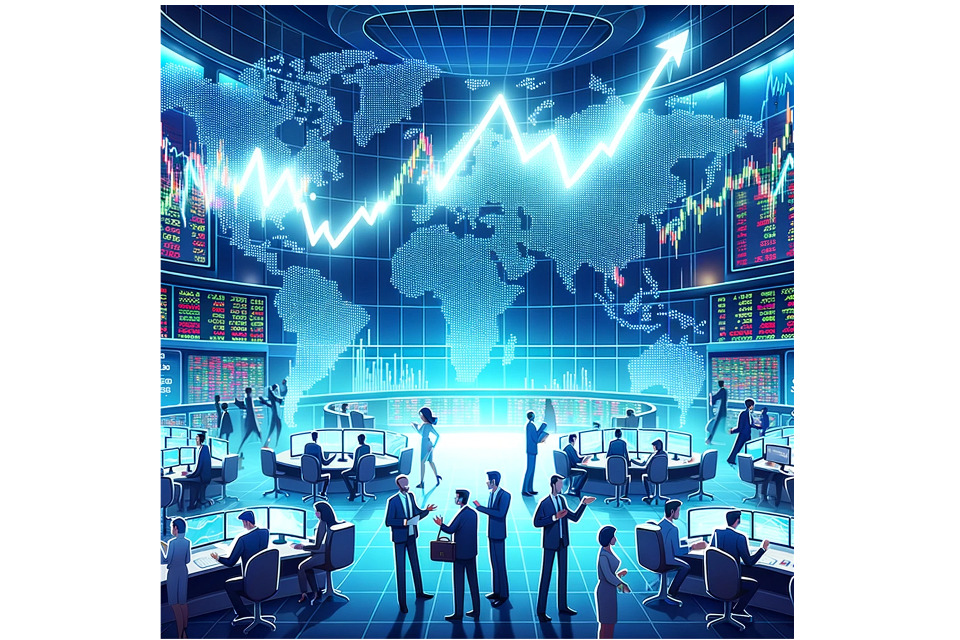
An AI generated image showing a bustling futuristic trade centre.
Figure 16 – Generated with Dall-e 3. We prompted the tool to depict a booming stock market and growing world trade.
If policies can be designed to manage the transition to a society and economy where AI use is more pervasive, it could have a positive effect on work.
Experts have highlighted that changes to the anatomy of work could provide market opportunities for the UK to develop and export advanced AI systems, with huge economic benefits .
AI is also expected to boost productivity, with potentially widespread economic benefits across a range of sectors. Stagnating productivity growth across developed economies over recent years has been well documented , , with negative impacts for living standards and public finances.
Studies have shown that AI tools are already being used by businesses to increase productivity. Automated tasks include administration , coding , and providing customer support ,. McKinsey recently estimated that generative AI could support trillions of dollars of productivity increases across the global economy, an estimate that is likely to increase as tools become more advanced and integrated across society .
Public sector
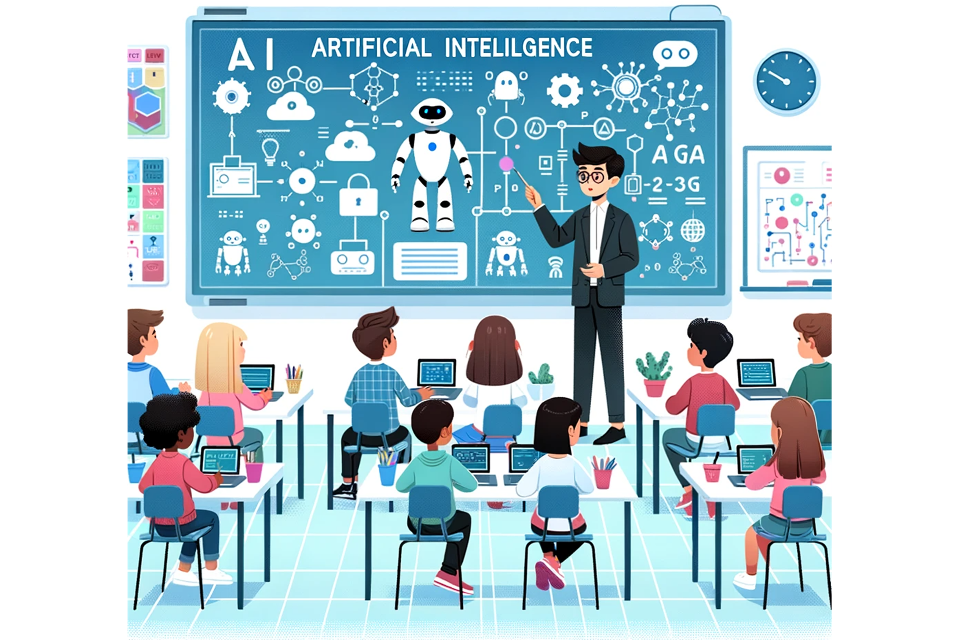
An AI generated image showing a school classroom, with a teacher teaching a class of children about AI.
Figure 17 – Generated with Dall-e 3. We prompted the tool to depict a classroom of children being taught about AI.
Automating repetitive, administrative tasks using AI could also help to address workload and cost pressures in sectors such as healthcare. This could enable staff to improve service levels, particularly if implemented in a way that allows them to focus on their interactions with public service users or neglected areas of their work.
AI also has the potential to facilitate the provision of more personalised public services, such as in education, that can be tailored to the needs of individuals , .
Achieving this will likely require the collection of more personal data, so privacy issues will need to be considered.
Policy making is another area of the public sector which could benefit from the integration of AI tools, provided that the necessary safeguards are implemented, and that humans retain control and oversight of the process. AI tools could assist in making difficult policy decisions by :
- improving data gathering, analysis capabilities and synthesising this into evidence;
- helping to simulate complex future situations; and
- supporting structured idea generation and red teaming.
Science and technology
Developments in AI also have the potential to accelerate advances in science and technology. Google DeepMind is already making use of AI to rapidly improve understanding of protein folding . AI is also finding applications in other areas such as drug design and chip design .
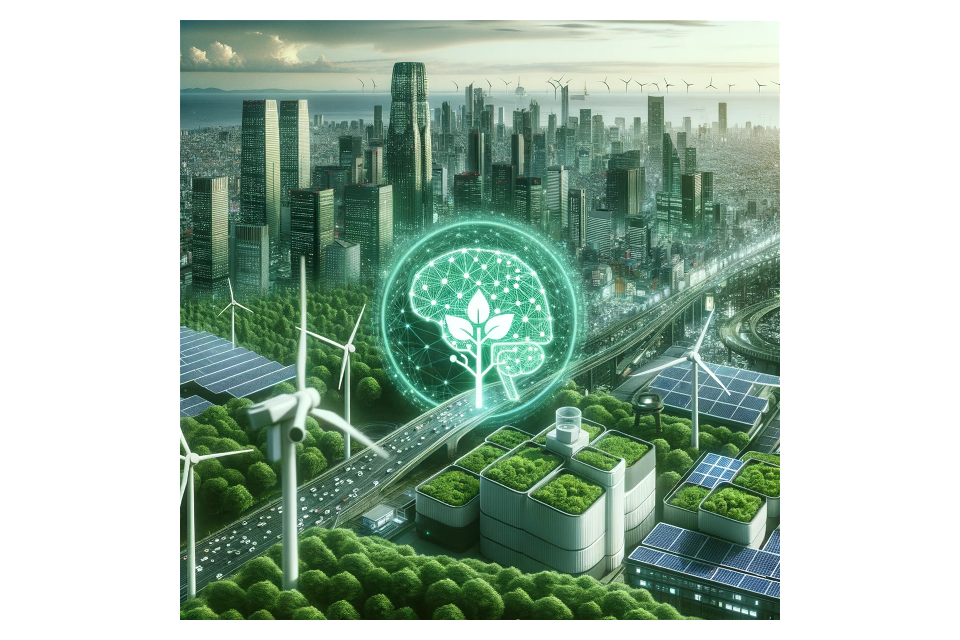
An AI generated image showing ecological advancement and green energy integration with a city.
Figure 18 – Generated with Dall-e 3. We prompted the tool to depict a futuristic cityscape where technology is used to help the environment.
As AI systems and tools continue to become more advanced, the range of applications across science and technology disciplines is anticipated to increase . Automation of research and development using AI could drive medical advances with widespread healthcare benefits.
AI could also pave the way for improved management of high-risk technology and environments, such as nuclear resources and space exploration.
In addition, AI has the potential to enable technological solutions to global challenges such as climate change, such as monitoring greenhouse gas emissions, predicting emissions more accurately and analysing where emissions can be reduced most effectively .
AI has also been applied in the field of materials science to help design and discover new advanced energy materials which could contribute to global carbon neutrality .
Society
AI could facilitate a range of societal benefits, spanning reduced isolation, access to knowledge, improved safety and increased free time.
AI systems could act as a companion to reduce isolation or provide personalised, 24/7 care, particularly in circumstances where the state doesn’t currently provide such a service. Alternatively, if automation of tasks by AI provides more free time for humans, they could use this time to provide care to those less keen on AI companionship.
AI could also radically improve access to knowledge, helping to reduce inequality. For example, by improving access to information and teaching, AI could drive an increase in global education standards and could also help to equip students and workers with the digital skills required to thrive in the future economy .
Autonomous vehicles are an example of an AI system that could improve public safety whilst allowing vehicle occupants to spend time on other activities. They also offer the potential to improve the public realm by reducing the need for parking spaces. However, policies will be needed to ensure the ease of using autonomous vehicles doesn’t cause an increase in travel leading to higher levels of road congestion .
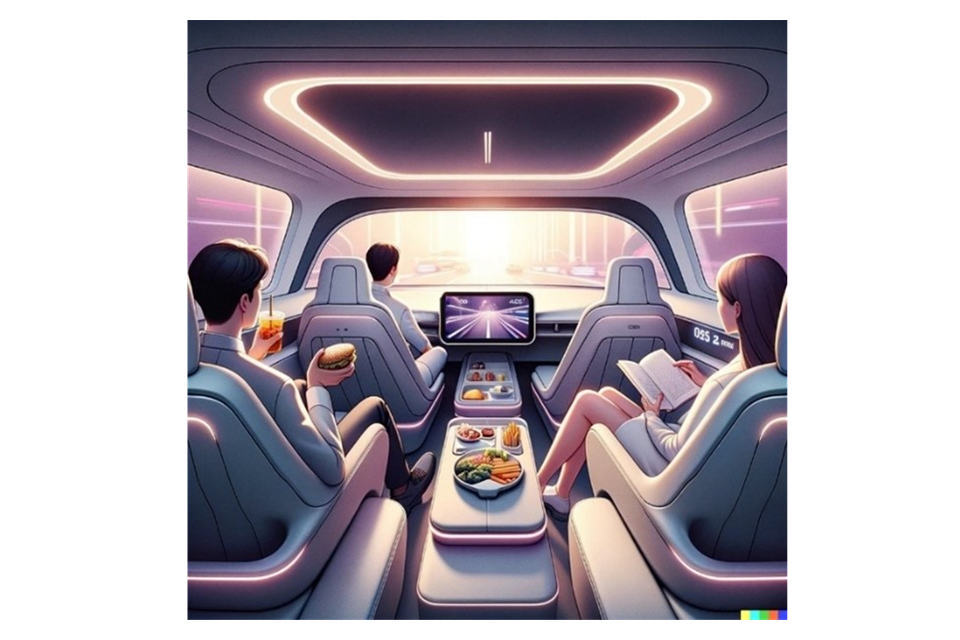
An AI generated image depicting a high tech driverless car.
Figure 19 – Generated with Dall-e 3. We prompted the tool to depict a driverless car in a futuristic city.
In addition, as more tasks become automated, there is potential for AI to improve people’s work-life balance to pursue recreational interests or more rewarding work, although this would require a shift in business practices and how employers design jobs.
Of course, if we are to allow AI into these personal aspects of our lives to realise these benefits, this will require public trust and privacy to be maintained, bias to be minimised, and accuracy of AI systems to be improved.
Why haven’t we included more favourable AI futures in our set of scenarios?
Evidently, the continued development of AI offers many opportunities for beneficial impacts across society. However, many experts in the field believe that in order to navigate to positive AI futures, policy interventions and government action will be required, at both national and international level.
The following scenarios have been designed to test policy ideas and government interventions that might mitigate the potential future risks that AI could pose. The scenarios therefore focus on the challenges that could arise in different AI futures and the policy decisions needed to realise the positive impacts of AI outlined above.
5.2 Scenarios summary
Unpredictable Advanced AI
Highly capable but unpredictable open source models are released. Serious negative impacts arise from a mix of misuse and accidents. There is significant potential for positive benefits if harms can be mitigated.
AI Disrupts the Workforce
Capable narrow AI systems controlled by tech firms are deployed across business sectors. Automation starts to disrupt the workforce. Businesses reap the rewards, but there is a strong public backlash.
AI ‘Wild West’
A wide range of moderately capable systems are owned and run by different actors, including authoritarian states. There is a rise in tools tailored for malicious use. The volume of different AI systems is a problem for authorities.
Advanced AI on a Knife’s Edge
Systems with high general capability are rapidly becoming embedded in the economy and peoples’ lives. One system may have become so generally capable that it is impossible to evaluate across all applications.
AI Disappoints
AI capabilities have improved somewhat, but big labs are only just moving beyond advanced gen AI. Investors are disappointed and looking for the next big development. There is a mixed uptake across society.
This graphic brings together the five individual graphics from above. The data from these graphics is available below:
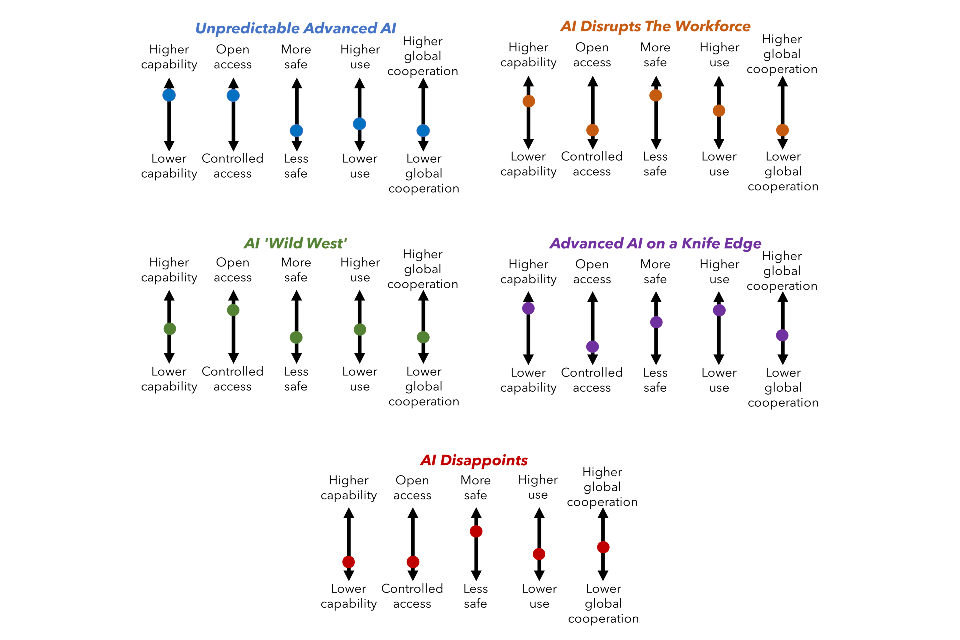
Scenario Sliders in relation to Critical Uncertainties, Scored 1-5 (Low to High)
Scenario | Capability | Access | Safety | Use | Global Cooperation |
---|---|---|---|---|---|
Unpredictable Advanced AI | 5 | 5 | 1 | 2 | 1 |
AI Disrupts the Workforce | 4 | 1 | 5 | 3 | 1 |
AI ‘Wild West’ | 3 | 5 | 2 | 3 | 2 |
Advanced AI on a Knife’s Edge | 5 | 1 | 4 | 5 | 2 |
AI Disappoints | 1 | 1 | 4 | 2 | 3 |
5.3 Quick guide to the scenario narratives
The scenario narratives include the following sections. Unless otherwise stated, we developed these using workshop outputs and expert feedback.
- Scenario summary. A brief to description of the scenario in 2030, focusing on the key outcomes across the critical uncertainties.
- Slider-graph showing where the scenario falls on each critical uncertainty axis.
- Scenario details in 2030, describing outcomes for the five critical uncertainties and asking ‘key questions’ for policy makers.
- AI generated images intended to bring the scenarios to life visually. The images in this section were generated with AI Comic Factory. AI Comic Factory is powered by a Stable Diffusion application programme interface (API) and the Llama-2 language model API . We prompted the tool to depict specific ‘visions’ we devised for each scenario. In some cases, we asked the LLM to generate images of more diverse groups, but this was not always effective. This image generator also struggles to create images of text, and we chose to leave these inaccurate representations of words within the images to highlight this limitation of current AI systems.
- Key turning points in 2025, suggests key events that led to a particular scenario.
- Frontier AI Adoption in 2030, a graph sets out our assumptions for the adoption of AI in different sectors and markets. They are average values, and we acknowledge they conceal significant variation within each sector and market. These assumptions are derived directly from the qualitative narrative, rather than any quantitative study.
- Wider context in 2030. An overview of the Social, Economic, Technological, and Environmental context.
- Key policy considerations, opportunities and potential policy implications associated with the scenario.
5.4 Unpredictable Advanced AI
Data from this graphic shown below:
Scenario | Capability | Access | Safety | Use | Global Cooperation |
---|---|---|---|---|---|
Unpredictable Advanced AI | 5 | 5 | 1 | 2 | 1 |
In the late 2020s, new open source models emerge, capable of completing a wide range of cognitive tasks with startling autonomy and agency. The pace of this change takes many by surprise. In the initial weeks following release, a small number of fast-moving actors use these systems to have outsized impacts, including malicious attacks and accidental damage, as well as some major breakthroughs in science and technology. There is public nervousness about use of these tools.
See ‘Quick guide to the scenario narratives’ at the start of Chapter 5 to learn how each section was created.
Scenario detail in 2030
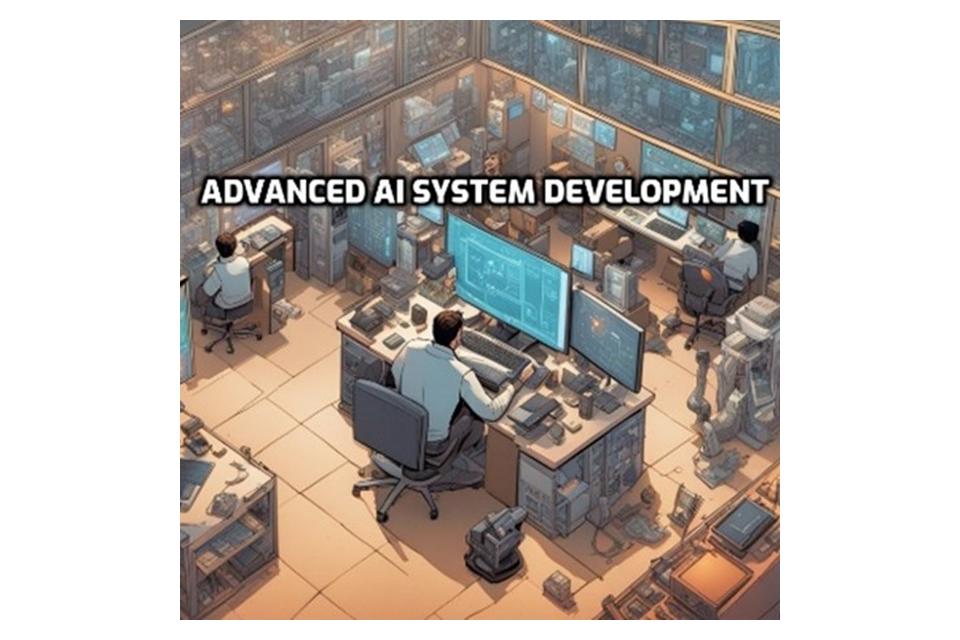
An AI generated image showing digital technicians developing advanced AI systems.
Figure 20 – Generated with AI Comic Factory. We prompted the tool to depict open source developers working on advanced AI.
Capability. During the 2020s, improvements in AI capability steadily continued, particularly for cognitive tasks, although AI systems continued to need human oversight and assurance. AI also started to show promise in physical world tasks. A breakthrough in the late 2020s saw a group of open source developers combine several interacting, capable, but narrow AI systems that use disparate tools and software packages to produce highly capable, autonomous AI agents.
These agents can complete complex, long-term tasks that require planning and reasoning, and can interact with one another in rich and flexible ways. Once a task is set, they quickly devise a strategy with sub-goals, learning new skills or how to use other software tools, mining and manipulating the internet to achieve their end objective.
This results in unforeseen consequences, as primary objectives are prioritised over collateral damage, including instances of AI agents pirating software, and hijacking data and compute resources to aid goal completion. Most users try to mitigate these side-effects, but some have accidents and others intentionally use the tools to cause harm.
Key question: If highly capable open source AI systems can be developed, will it be enough to target any standards or safety regulations at the original developers of these systems, given that others will adapt them after release?
Ownership, constraints, and access. Big labs continued to focus on improvements driven by more compute and data. But there has been a drag on progress from a relatively constrained supply of GPU chips, driven partly by conflict-driven supply chain disruption, and a slowdown in availability of new data sources. These proprietary tools, which many people continue to use in 2030, are accessed through user-friendly, but relatively controlled software interfaces.
In the background, the open source community has focussed on building systems of interacting tools that need less compute and training data. This work was accelerated by several high-profile developers leaving big labs to join this open source effort after becoming concerned with the concentration of power sitting with a few large companies. This new model architecture wasn’t fully anticipated by big labs, who are working quickly to make use of these breakthroughs, but there is a lag. A lag of a few weeks is enough for big impacts to unfold, and even after the big labs catch up, bad actors still have access to powerful tools whose use is difficult to regulate.
Key question: Who is accountable for the impacts of advanced open source AI systems? And how should they be held to account?
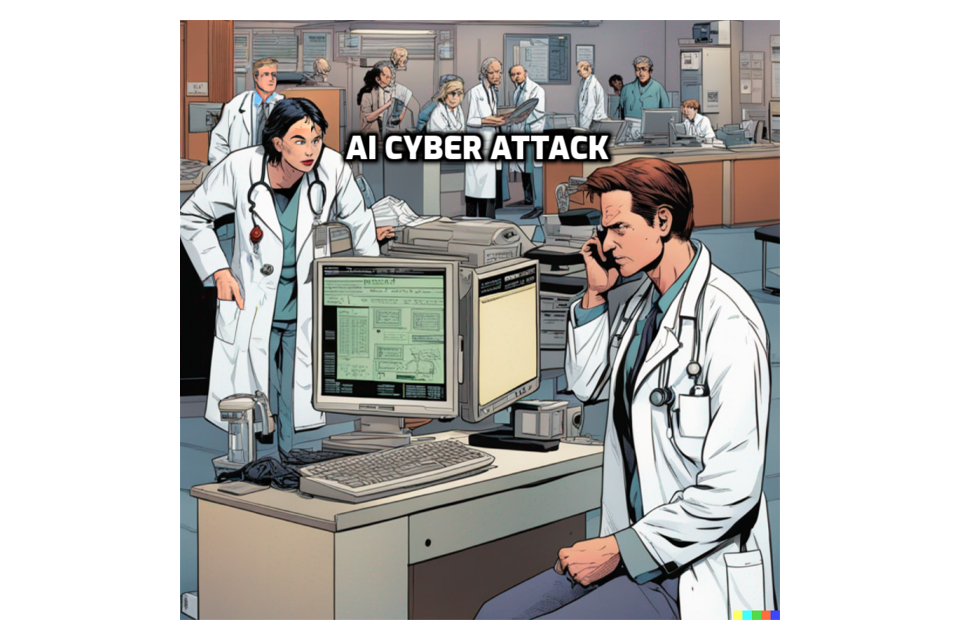
An AI generated image showing doctors inside a hospital looking frustrated and concerned about a cyber-attack.
Figure 21 – Generated with AI Comic Factory. We prompted the tool to depict a cyber-attack on a hospital.
Safety. Given the gradual pace of progress during the mid-2020s, safety systems for mainstream AI tools were developed in parallel. Biases and incidents of misuse emerged, but the big technology companies tracked these closely and fine-tuned models in response to user demand and emerging best practice standards.
However, serious concerns are raised when highly capable open source systems are released, which do not have much safety infrastructure, and misuse is harder to control. Both accidental harms, caused by irresponsible development and use of these tools, as well as malicious AI-based attacks rapidly become more frequent and more severe. This starts with cyber-attacks on infrastructure and public services, but by 2030, intelligence services become aware of terrorist groups developing bioweapons using these tools – it now seems only a matter of time before one of these plots is successful.
There are concerns that this will permanently damage the reputation of open source developers, who have continued to be key to building many of the critical components of widely used software and cyber security systems.
Key question: How can law enforcement and intelligence services keep up? Are AI-based physical-world attacks next?
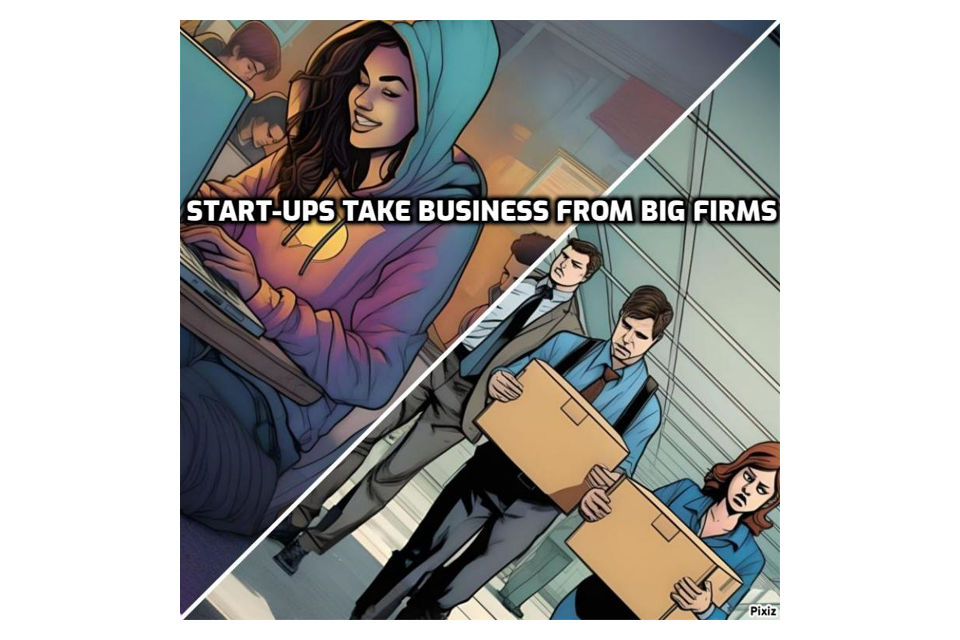
An AI generated image showing a split scene of new start-up businesses thriving while big firms see closures.
Figure 22 – Generated with AI Comic Factory. We prompted the tool to depict a successful start-up and employees of a big firm losing their jobs.
Level and distribution of use. The mid-2020s saw some instances of serious misuse, such as disinformation campaigns. However, AI tools had also proved useful and benign in many contexts, such as helping people be more productive through integration into widely used software. There had been some workforce disruptions with certain tasks and jobs being automated, but the focus was more on augmentation of workers than on replacement, which allowed industries and workers to adapt more easily. However, when powerful new tools are released, skills inequalities widen. Start-ups with higher skilled workers and a higher risk appetite quickly surge ahead and disrupt existing markets.
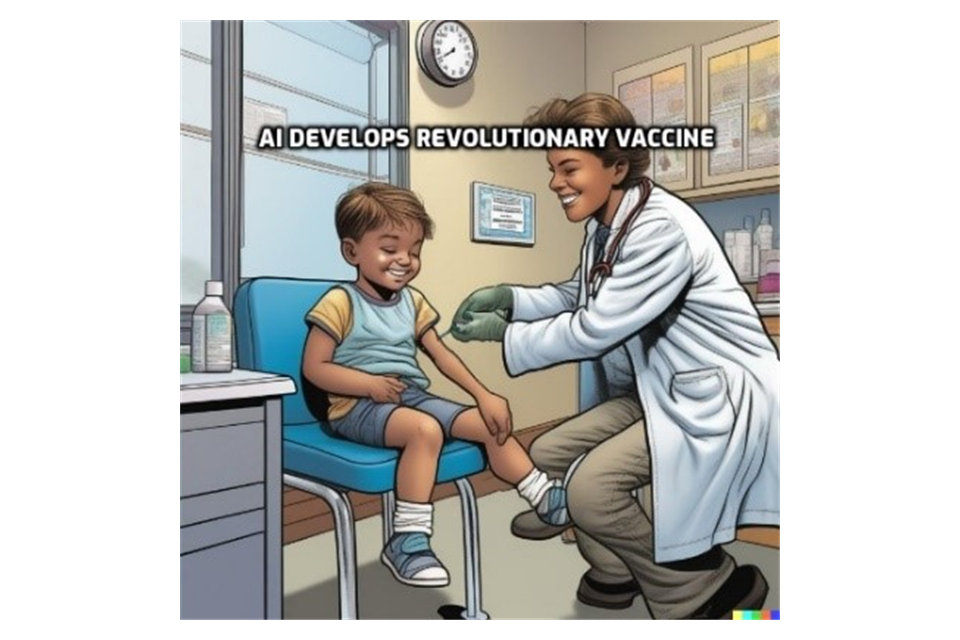
An AI generated image showing a doctor administering a vaccine that was developed by AI.
Figure 23 – Generated with AI Comic Factory. We prompted the tool to depict a child receiving a new vaccine.
Severe misuse, including in advanced cyber-attacks on UK public services and infrastructure, contributes to a feeling that AI is enabling more harm than good.
Key question: How can large, risk averse organisations, catch up in this new paradigm?
One cause for optimism is a series of rapid science and tech discoveries enabled by groups of academics adopting these new tools, including breakthroughs in healthcare and green technologies. There is also an army of developers working on this open source AI, finding new applications and fixing bugs for the benefit of users.
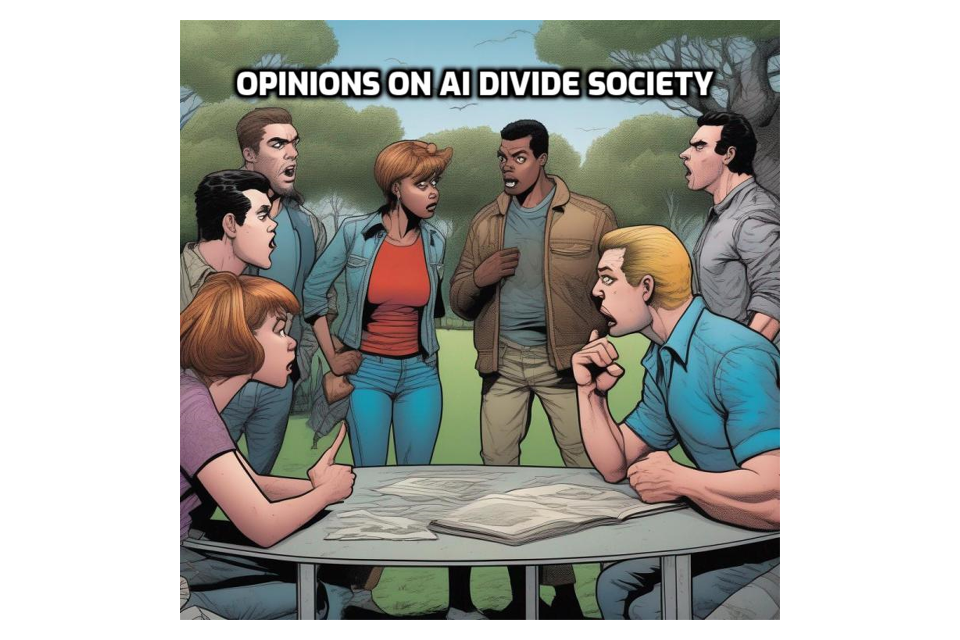
An AI generated image showing a crowd of people arguing about AI in a park.
Figure 24 – Generated with AI Comic Factory. We prompted the tool to depict a disagreement between a group of people.
Geopolitical context. Global tensions had simmered during the 2020s, with flare-ups in tension leading to semiconductor supply chain disruption. Cyber and military capabilities unlocked by the open source AI breakthroughs of the late 2020s led to a series of incidents and emerging conflicts between blocks of countries. This makes it challenging to cooperate to tackle adverse impacts.
Key question: If the Frontier of AI is open source, how can we avoid at least some actors using advanced AI in a military context, and that exacerbating global conflict?
How did we get here? Key turning points in 2025
-
A ‘GPU crunch’ - supplies of advanced semiconductors from a key manufacturer are disrupted due to global conflict. Only big labs can afford the limited supply.
-
Driven by the GPU crunch, open source researchers to focus on systems with lower-compute needs and start to succeed in producing useful components in the run-up to the big breakthrough in the late 2020s.
-
Hostile actors track these developments closely and can deploy breakthrough tools as soon as they are released.
Unpredictable Advanced AI - Frontier AI Adoption in 2030
The chart below shows high-level adoption assumptions for Frontier AI in 2030. Uptake is highest amongst the highly skilled and start-ups who are moving rapidly to deploy the latest tools, despite safety issues. Less capable, more mainstream AI is in general more widely used across larger businesses and to some extent the public sector.
Data from this graphic shown below:
Unpredicatable Advanced AI, Adoption Levels Low-High
People | Private and Public Sector | Automation | International | ||||||
---|---|---|---|---|---|---|---|---|---|
Higher Skilled | Lower Skilled | Large | Start-ups | Other SMEs | Public | Cognitive tasks | Physical world tasks | UK | Rest of world |
High | Low | Medium | High | Low | Low | Medium | Low | Medium | Medium low |
Unpredictable Advanced AI - wider context in 2030
Social: After a turbulent early 2020s, the middle of the decade saw a slightly calmer period with fewer global shocks, leading to modest improvements in societal stability. Use of AI continued to have mixed societal impacts, with some people benefiting from AI tools that helped to complete everyday tasks, and others falling victim to relatively small-scale AI misuse. As the end of the decade neared, major disruptions from use and misuse of highly capable new AI tools upended this relatively tranquil period. By 2030, people are divided over whether these tools can be harnessed for the benefit of society.
Economic: Small but discernible productivity improvements stemming from a combination of relative global stability and business use of mainstream AI tools during the mid-2020s meant that economic growth was starting to recover, with most people benefitting in some way. As new skills inequalities arose later in the decade, this started to translate into increased economic inequality. Wider disruptions from cyber-attacks and other conflict start to cause economic damage.
Technological: Powerful new AI tools are used to unlock science and technology discoveries in the late 2020s, including vaccines for a range of infectious diseases and novel materials for energy storage. This suggests we could be on the verge of a period of rapid technological innovation, provided wider disruptive impacts of AI don’t derail this.
Environmental: New AI tools that use less compute have the potential to significantly reduce the environmental footprint of the technology. Environmental improvements could also emerge from big science breakthroughs. However, the advanced new AI tools released in the late 2020s will need to be fully assured and turned into reliable products before they can be deployed into these applications and the potential benefits can be fully realised.
Unpredictable Advanced AI - Key policy considerations
Opportunities
- Innovators can use these new tools to solve grand challenges e.g., in medicine and environmental sustainability.
- The most capable AI tools are accessible to all (although they are not reliably safe).
- Open source tools being at the Frontier helps to open up the market to new entrants, which could be a benefit for innovation and an economic opportunity for the UK.
Implications for policy in the near term
- Frontier AI systems are currently the preserve of large technology companies, including the most capable open source models. But in scenarios where highly capable open source AI with lower compute requirements emerge, effective regulations or laws might need to cover any potential developer or user of these systems, not just large technology companies.
- Given highly capable open source models may be released, policymakers will need to consider systems that help to track, control, or shut them down post-release. This will be particularly important in the context of severe malicious use, such as large-scale cyber-attacks. Mechanisms that disincentivise such misuse will also need to be considered.
- If the most capable models are open source, where does competitive advantage come from? Does value flow even more to those with the most comprehensive, or best structured, data?
- Developments in Frontier open source AI have the potential to accelerate innovation in both the AI systems themselves and their applications, such as scientific discoveries. Any regulations or laws that limit these activities need to consider likely trade-offs with promoting innovation.
- A future where open source AI systems are at the Frontier could make it possible for any actor to use advanced AI in a military context. Policymakers will need to consider what can be done to prevent this or mitigate the impacts in terms of harm caused or escalating global conflict.
- In all scenarios, there will be benefits from upskilling the population in awareness and use of AI, to help mitigate risks like disinformation, and ensure everyone is able to enjoy the benefits of AI. This scenario highlights the fact that more widespread availability of advanced open source models may increase the importance of AI development skills that allow people and businesses to use, adapt and benefit from these tools.
- In all scenarios, higher levels of global cooperation would help to prevent or mitigate negative outcomes. Fostering this cooperation is therefore a key ‘low regret’ action for policymakers. This scenario highlights the challenge of open source AI systems, which can be freely shared across borders. Managing their negative impacts therefore needs a global solution.
5.5 AI Disrupts the Workforce
Data from this graphic shown below:
Scenario | Capability | Access | Safety | Use | Global Cooperation |
---|---|---|---|---|---|
AI Disrupts the Workforce | 4 | 1 | 5 | 3 | 1 |
At the frontier, relatively narrow but capable AI systems are starting to provide effective automation in many domains. By 2030, the most extreme impacts are confined to a subset of sectors, but this still triggers a public backlash, starting with those whose work is disrupted, and spilling over into a fierce public debate about the future of education and work. AI systems are deemed technically safe by many users, with confidence they won’t demonstrate unexpected behaviour, but they are nevertheless causing adverse impacts like increased unemployment and poverty.
See ‘Quick guide to the scenario narratives’ at the start of Chapter 5 to learn how each section was created.
Scenario details in 2030
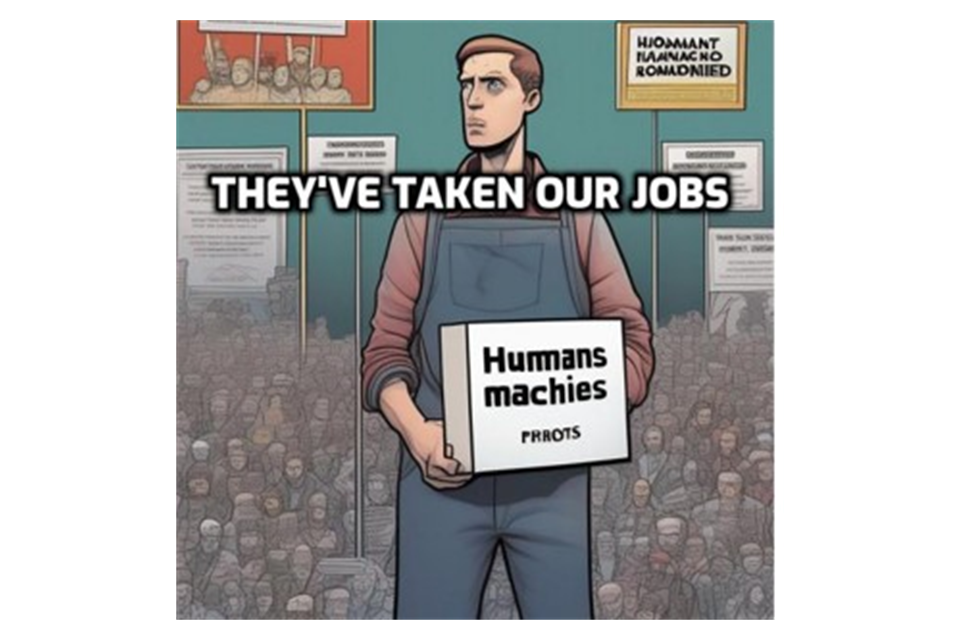
An AI generated image depicting a protest against AI displacing people’s jobs.
Figure 25 – Generated with AI Comic Factory. We prompted the tool to depict a person protesting AI taking jobs.
Capability. Following improvements in the performance of foundation models in the early 2020s, the middle of the decade saw fierce competition between tech firms centred around domain-specific fine-tuning of base models to be highly capable at specific tasks. A key breakthrough has been the ability of AI to interact with the physical world via robotic systems, as well as autonomous vehicles, leading to relatively widespread disruptions to labour markets. As they are rapidly deployed into the real world, these autonomous systems gather vast amounts of data, which is channelled back into fine-tuning their capabilities.
Key question: What kinds of major disruptions could emerge from widespread use of narrow AI systems?
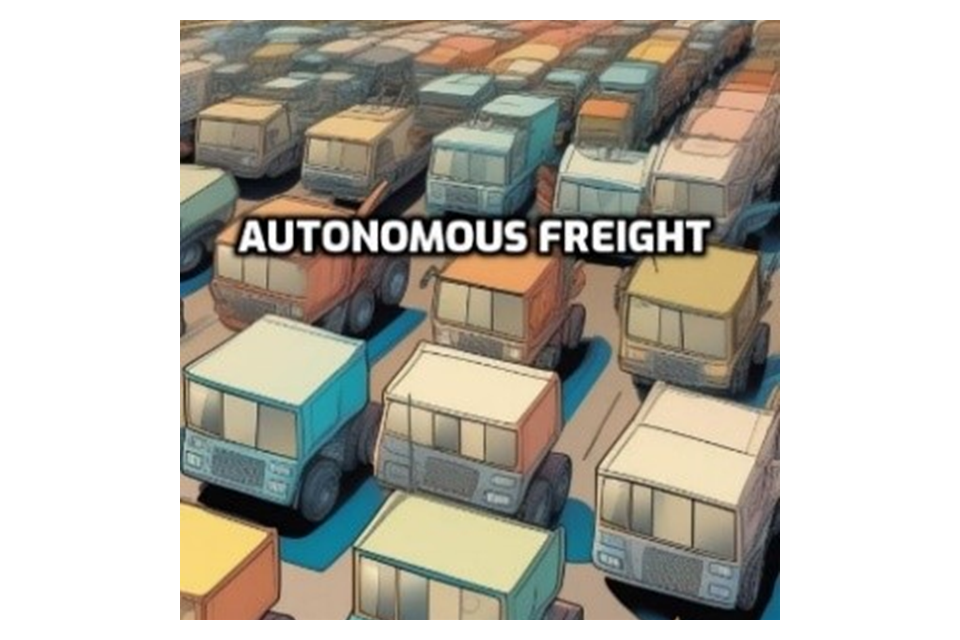
An AI generated image showing futuristic commercial vehicles tightly packed on a road.
Figure 26 – Generated with AI Comic Factory. We prompted the tool to depict a fleet of autonomous vehicles.
Ownership, constraints, and access. AI has continued to be dominated by technology giants with developments driven by scaling of compute and data. Concerns over the exhaustion of high-quality data have led labs to prioritise acquiring or synthesising new datasets tailored for specific tasks, leading to improvements in a range of narrow capabilities.
Furthermore, delays in planning and construction meant the building of new data centres didn’t keep up with demand. This reinforced the focus on narrow AI systems, which have somewhat lower compute requirements. Smaller scale developers struggle to compete as they can’t afford the data or compute resources.
AI systems are intuitive and user-friendly, which aids rapid deployment, but they are only accessible through highly controlled software interfaces.
Key question: What kinds of AI models will get built if high quality text data runs out?
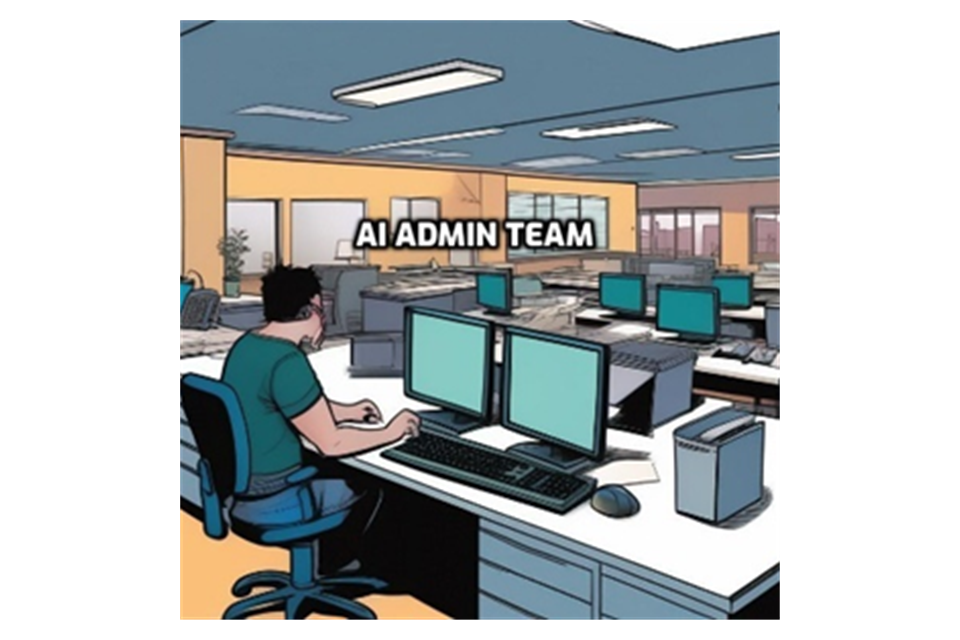
An AI generated image showing a lone office worker doing admin.
Figure 27 – Generated with AI Comic Factory. We prompted the tool to depict an office of workers doing admin.
Safety. The controlled nature of AI systems supports a widespread perception that they are ‘technically safe’ - i.e. they perform how most operators of these systems expect them to – and are adopted by many businesses. However, there are concerns regarding firms rapidly integrating these systems into decision making processes without implementing effective bias detection systems, which leads to adverse impacts for service users. These issues are acknowledged; however, the intense competition between technology firms exacerbates concerns as firms race to develop their technologies, potentially at the risk of causing harm.
Key question: How can we ensure competition between businesses doesn’t drive practices that result in AI-related harm?
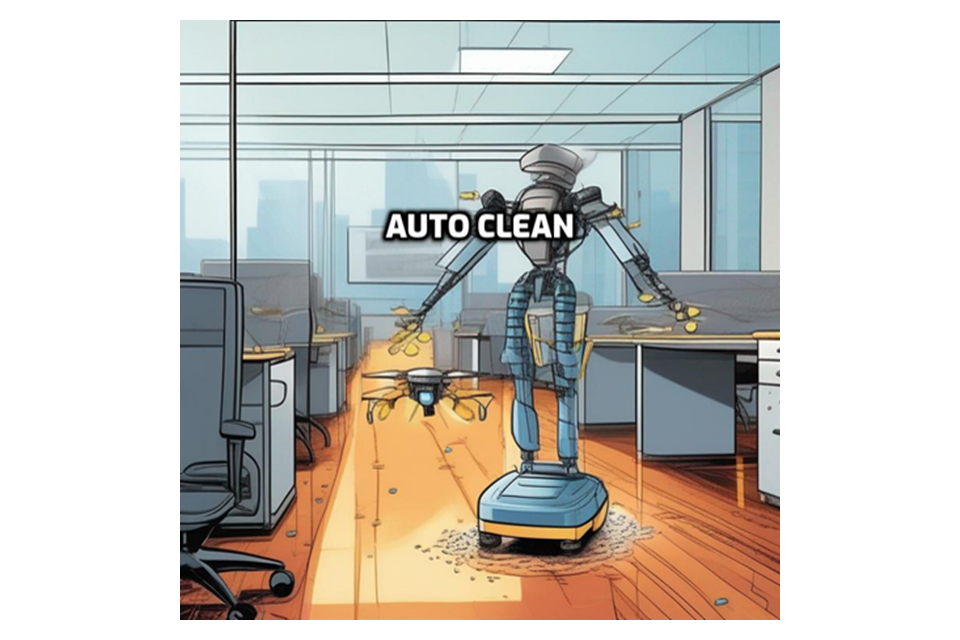
An AI generated image showing autonomous robots cleaning an office space.
Figure 28 – Generated with AI Comic Factory. We prompted the tool to depict robots cleaning an office space.
Level and distribution of use. By 2030, there is significant deployment of AI across the economy, driven by improvements in AI automation capability and the opportunity this offers to realise productivity gains and reduce labour costs. This is most highly concentrated in certain sectors – e.g. IT, accounting, transportation (taxis and freight) - and in the biggest companies who have the resources to deploy these new systems.
The management consultancy sector has rapidly pivoted to focus on assisting businesses to use these productivity enhancing systems. New entrants, who don’t have the problem of integrating AI systems into legacy infrastructure, are also leapfrogging incumbents.
Sectors that already have a degree of automation, like agriculture, see an AI enabled acceleration of this trend. More heavily regulated sectors, like construction, are less affected. But as regulations change in other countries, there are calls from these sectors for the UK to do the same. These systems require significantly less human oversight than before which leads to a reduction in jobs in affected sectors.
Whilst this may be transient, it results in a rampant public backlash, though the impact of industrial action is limited in heavily automated sectors. This transition favours workers with the skills to oversee and fine-tune models (a new class of ‘AI managers’), at the expense of other workers, resulting in greater inequality.
Some commentators are increasingly concerned that AI generated outputs are less creative and unique, a problem which could get worse as systems run out of human generated training data. Public disapproval of AI focusses on the economic and societal impacts – perceived to be driving unemployment and poverty - rather than concerns over the safety of systems.
Key question: What policies should be implemented to help structurally unemployed workers and upskill the workforce?
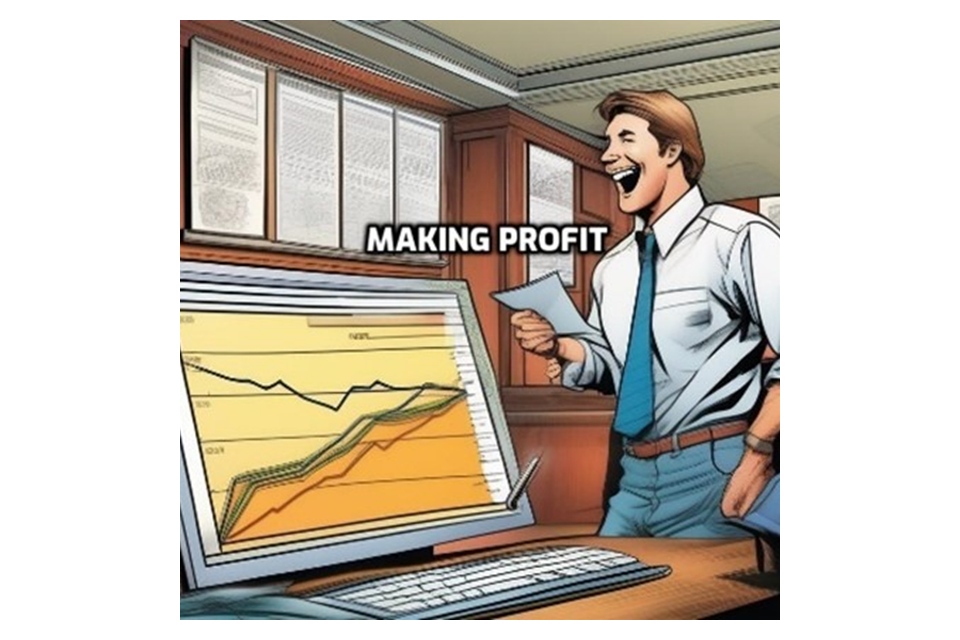
An AI generated image showing positive sales figures on a computer screen and a happy looking sales-person.
Figure 29 – Generated with AI Comic Factory. We prompted the tool to depict a business making profit with positive sales reports.
Geopolitical Context. There is fierce economic and technological competition between nation states, leading to countries racing to develop their AI capabilities before their adversaries. By 2030, efforts to onshore production of advanced semiconductors to Western countries have started to make some headway, which reduces some of the inter-reliance between continental blocks and increases the risk of conflict. This leads to limited global cooperation on a range of issues, including climate change and AI.
Key questions: How can we move from global competition to global cooperation?
How did we get here? Key turning points in 2025
- Worldwide data centre capacity shortages and price spikes led to development of AI-systems shifting towards narrow capabilities and lower compute requirements.
- AI vendors started to see higher returns from domain-specific solutions that are easier to roll out, rather than general purpose solutions, which accelerates this shift in focus.
- High levels of inflation lasted longer than expected, and the cost of labour stayed high relative to the cost of deploying AI. Firms therefore used AI systems to replace labour.
AI Disrupts the Workforce - Frontier AI Adoption in 2030
The chart to the right shows high-level adoption assumptions for AI in 2030. AI systems have been widely adopted across the economy, automating a variety of physical and cognitive tasks. Public sector adoption of AI is more mixed - stronger trade unions limit job substitution, but AI helps refocus roles on tasks where human input is most important. People with high levels of skill in using AI tools do the work that would previously have been done by multiple people.
Data from this graphic shown below:
AI Disrupts the Workforce, Adoption Levels Low-High
People | Private and Public Sector | Automation | International | ||||||
---|---|---|---|---|---|---|---|---|---|
Higher Skilled | Lower Skilled | Large | Start-ups | Other SMEs | Public | Cognitive tasks | Physical world tasks | UK | Rest of world |
High | Low | High | High | Medium | Low | Medium high | Medium high | Medium high | Medium high |
AI Disrupts the Workforce - wider context in 2030
Social: By 2030, increased unemployment, wage inequalities and increased poverty have eroded social cohesion. There are increasing concerns over the use of AI for public surveillance as well as its potential to exacerbate existing inequalities, e.g. recruitment bias. This results in rampant backlash in the form of protests and industrial action, causing economic disruption. However, AI benefits society in other ways; for example, increased productivity results in cheaper goods and services. Jobs that require in-person skills, such as hospitality and entertainment, become particularly desirable, especially for young people, as do the skills required to build or fine-tune AI systems.
Economic: By 2030, productivity is starting to significantly increase as AI is adopted across a range of sectors. Despite increased incomes for developers of AI systems and businesses deploying these systems, there are net reductions in employment across society as well as significant wage declines and income inequalities. Many white-collar and blue-collar jobs are automated with the biggest impacts in sectors that rely on repetitive cognitive work, like accounting, but also to some extent in sectors that require physical tasks to be undertaken, like agriculture.
Technological: AI models possess advanced narrow capabilities and to a lesser extent, general capabilities. As such, previously voiced concerns about existential risk from AGI are heard less often than in the early 2020s. With the ability of AI to interact with the real world, there has been significant research and developments into robotic systems to capitalise on such AI advancements.
Environmental: Following a shortage of GPU chips in the early 2020s, chip supply has largely caught up with demand by 2030, and the environmental footprint of AI has significantly increased. There has been limited research into compute-efficient AI. However, as computational costs increase, firms begin to develop more compute-efficient solutions. Automated manufacturing and construction reduce the cost of green technology and infrastructure, partially offsetting the environmental harms of AI.
AI Disrupts the Workforce - Key policy considerations
Opportunities
- Productivity increases lead to lower prices for consumers.
- Many sectors and businesses see improved efficiency, with higher wages for the people who own and train AI systems.
- Economic growth leads to higher tax revenues and improved public finances.
Implications for policy in the near term
- Negative economic and societal impacts from AI use could occur even if there is successful implementation of technical AI safety systems and limited malicious use. Policymakers will therefore need to look beyond control of AI systems and their misuse, to consider wider interventions that can manage the systemic impacts of AI use.
- Whilst a scenario with highly general AI systems at the Frontier might see more significant impacts overall, a scenario with widespread use of highly effective narrower AI systems could still lead to major economic and societal upheavals. Policymakers will therefore need to consider narrow AI systems within scope when planning policy responses to such risks.
- Given the possibility that AI may not be like previous technological revolutions, and could lead to a permanent net reduction in jobs, policymakers will need to consider how they would respond to such an outcome. This could include considerations around workers’ rights, income inequality, re-training, taxation, and the overall balance of human vs machine labour.
- Even if more jobs are created by AI in the long run, many jobs could be significantly disrupted during the transition to an AI enabled economy. Policymakers will therefore also need to consider measures to mitigate the adverse, shorter-term impacts on many workers. This is likely to include upskilling the population in awareness and use of AI, which will help ensure people are able to enjoy the economic benefits, as well as mitigating other risks like disinformation.
- Automation has the potential to significantly increase productivity, with knock-on benefits for living standards. If policymakers mitigate economic and societal risks from automation by slowing down business adoption, they will likely need to consider the trade-off with productivity increases.
- In all scenarios, higher levels of global cooperation would help to prevent or mitigate negative outcomes. Fostering this cooperation is therefore a key ‘low regret’ action for policymakers. This scenario highlights the role that economic competition could play in making this cooperation more challenging, suggesting it will be important to establish cooperative arrangements in advance of significant economic impacts from AI use.
5.6 AI ‘Wild West’
Data from this graphic shown below:
Scenario | Capability | Access | Saftey | Use | Global Cooperation |
---|---|---|---|---|---|
AI ‘Wild West’ | 3 | 5 | 2 | 3 | 2 |
At the frontier, there is a diverse range of moderately capable AI systems being operated by different actors. Whilst vibrant new economic sectors are developing based on the use of AI, widespread safety concerns and malicious use reduce societal enthusiasm. Authorities are struggling with the volume and diversity of misuse. A focus on tackling the immediate impacts of this crisis has made it hard to reach a global consensus on how to manage the issues long-term.
See ‘Quick guide to the scenario narratives’ at the start of Chapter 5 to learn how each section was created.
Scenario detail in 2030
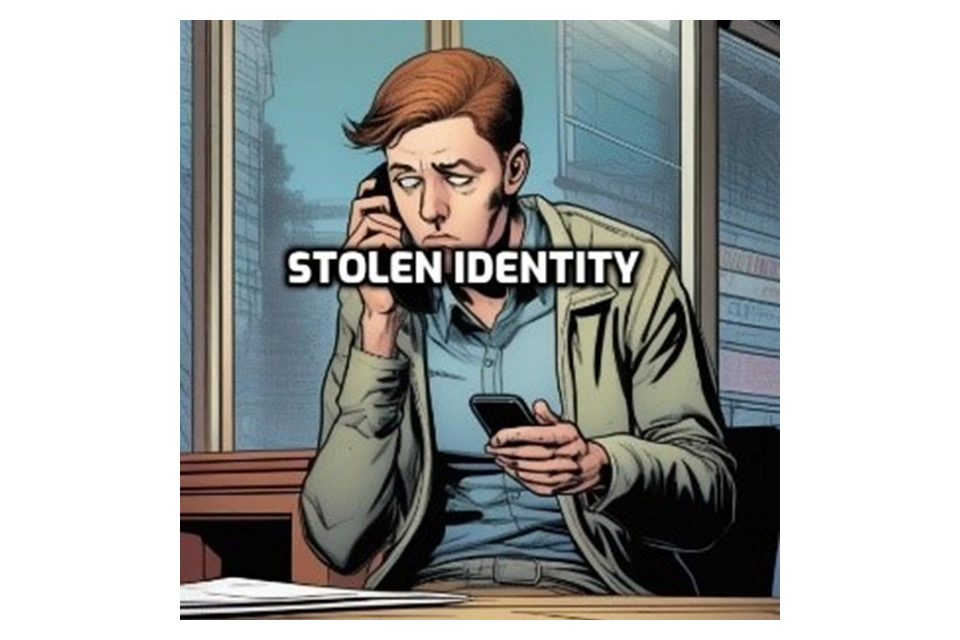
An AI-generated image showing an individual looking sad after having their identity stolen by AI.
Figure 30 – Generated with AI Comic Factory. We prompted the tool to depict a person on the phone looking worried.
Capability. Throughout the 2020s there have been moderate improvements in AI capability, particularly generative AI, such as creation of long-form, high-quality video content. It is now easy for users to create content that is almost impossible to distinguish from human-generated output. This includes capabilities that can be used for malicious purposes, such as very accurate and easy-to-use cloning of voices and other biometric data. Free LLMs can create large volumes of sophisticated text that cannot be identified as AI-generated, making the creation and spreading of disinformation easier.
Key question: How can we safeguard against models designed to go undetected?
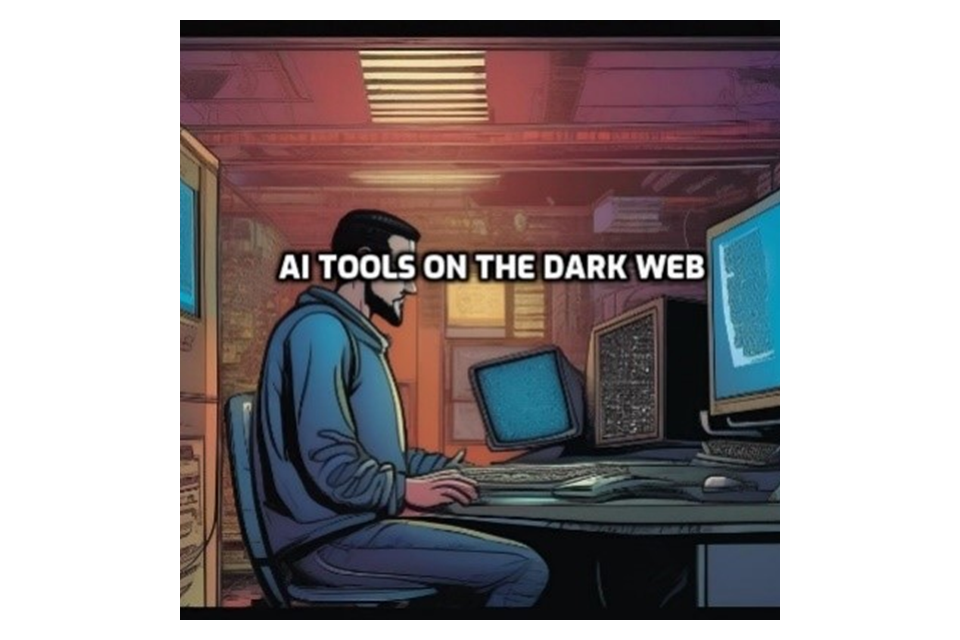
An AI generated image showing AI being used maliciously on the dark web.
Figure 31 – Generated with AI Comic Factory. We prompted the tool to depict a hacker on a computer in a dark room.
Ownership, constraints, and access. There is a diverse AI market in 2030 - big tech labs compete alongside start-ups and open source developers. Suppliers are also based in a wide range of countries. Big labs continue to buy up the most successful start-ups at a steady rate, but this ongoing ‘Cambrian explosion’ of new AI products and services mean that the market remains relatively diverse.
In addition, some of the most advanced models are released by authoritarian states. Use of these tools in the UK starts to grow due to their ability to perform specific tasks more accurately, although use in certain critical sectors remains low.
Disputes about whether these AI tools are being used for espionage by the states that produce them affect the wider geopolitical context.
Key question: How can governments effectively regulate such a diverse, global market and ensure standards are met in the UK?
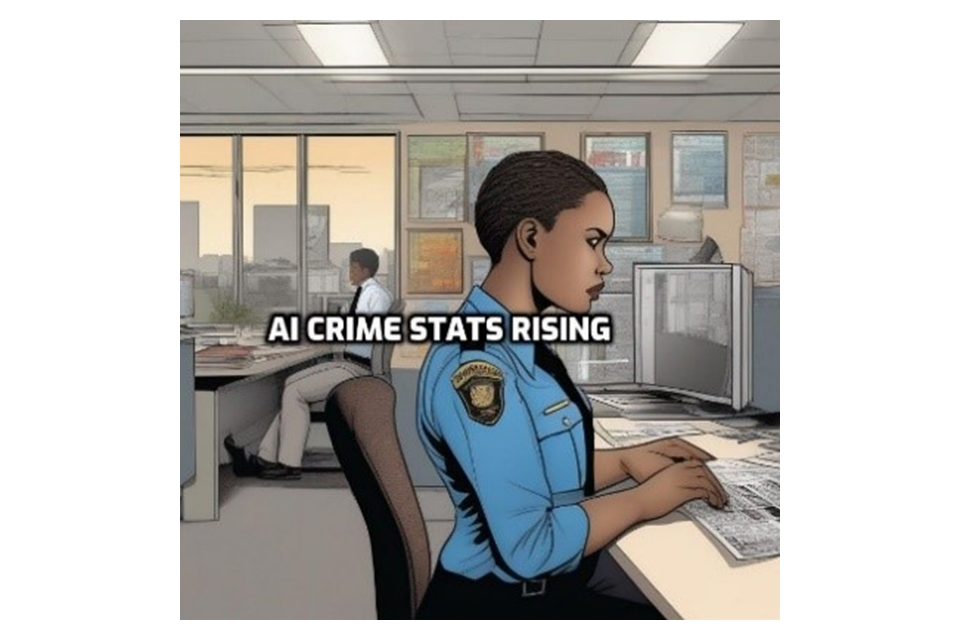
An AI generated image showing a concerned looking police office looking at increasing AI crime stats.
Figure 32 – Generated with AI Comic Factory. We prompted the tool to depict a police officer working at a computer, looking distressed.
Safety. There are many different types of AI system in use, which are distributed and hard to monitor. This causes widespread adverse impacts such as criminal groups using these AI systems to carry out scams and fraud. Throughout the 2020s, use of voice and facial cloning by malicious state and non-state actors for espionage, misinformation, and political interference increases, violating people’s privacy and human rights.
Despite relatively effective safety systems and frameworks being created by mainstream AI companies, the main safety challenges relate to controlling misuse.
Key question: Do we need AI-based enforcement to combat the high volume of misuse?
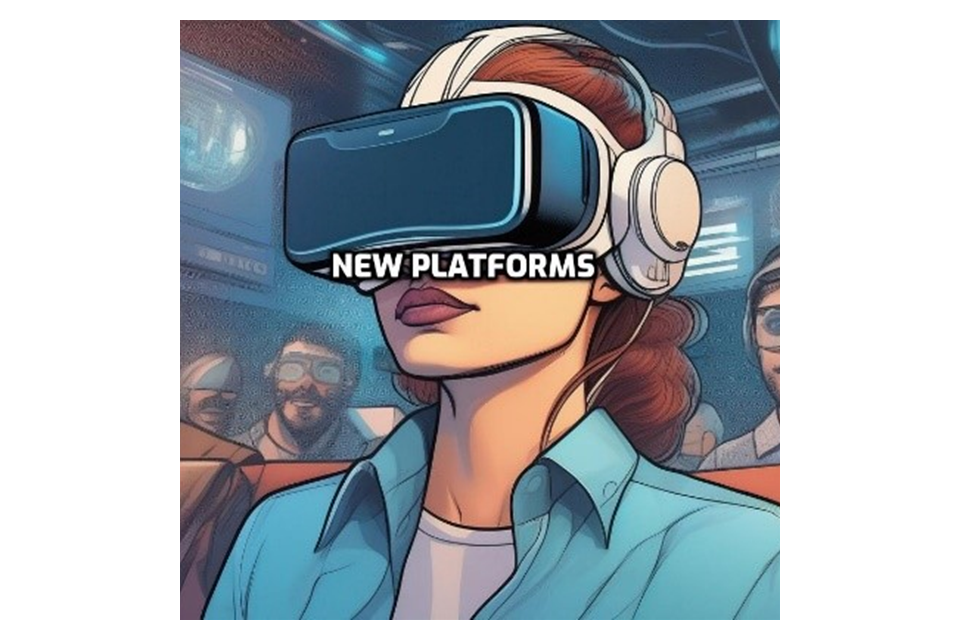
An AI generated image showing a person using a virtual reality device.
Figure 33 – Generated with AI Comic Factory. We prompted the tool to depict people wearing VR headsets.
Level and distribution of use. Levels of AI use are unevenly spread across society, with some people and businesses making intensive use of the diverse services on offer, and others being more wary. Part of this caution is driven by a big increase in misuse of AI, seeing many members of the public fall victim to organised crime.
Businesses also have trade secrets stolen by other nations on a large scale, causing economic damage. Intellectual property issues are also rife in the creative industries, with numerous blockbuster songs, films and novels created partly or fully using AI generated content.
Alongside these impacts, there are also wider job losses from automation in areas like computer programming. But this is offset to some extent by the creation of diverse new digital sectors and platforms based on AI systems, and the productivity benefits for those who are able to augment their skills with AI. However, concerns of an unemployment crisis are starting to grow due to the ongoing improvements in AI capability.
Key question: How can we stop individuals, businesses, and public sector organisations from being overwhelmed by cyber-attacks, fraud, and other challenges?
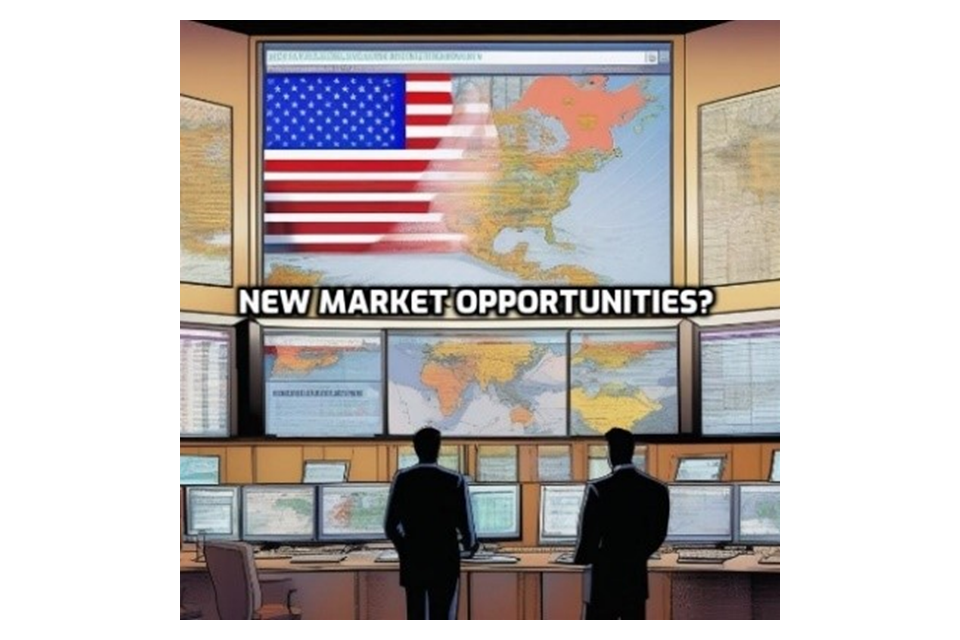
An AI generated image showing new market opportunities appearing on a global scale.
Figure 34 – Generated with AI Comic Factory. We prompted the tool to show a booming world trade market.
Geopolitical Context. Many countries are tackling increased and more effective nefarious or hostile activity enabled by AI, and struggle to hold perpetrators to account when they act across borders. Authoritarian states have made breakthroughs in AI capability using data gleaned from widespread domestic deployment of systems for delivery of services and surveillance. Commercial AI systems launched by these states have begun to be used to enable espionage and geopolitical interference. This leads to an environment where global cooperation is challenging to achieve – even if a way forward is agreed by democratic nations, there still remain fundamental differences of approach with other global blocks.
Key question: How can global agreement be reached on AI governance if there are significant differences in the priorities and values that are baked into AI systems?
How did we get here? Key turning points in 2025
- Capable and easy-to-use plug-ins for open source LLMs start appearing on the dark web tailored for highly effective phishing and identity theft.
- A new generation of digital platforms is launched for social interaction and commerce, but providers take time to devise appropriate moderation approaches, making them even more fertile ground for AI misinformation and scams than the platforms of the early 2020s.
- AI-based services and products originating from authoritarian states begin to be marketed globally. Owing to their competitive pricing of these services as well as their advanced capability, some people and businesses in the UK find these useful for everyday tasks and their use grows, but many are more cautious.
AI ‘Wild West’ - Frontier AI Adoption in 2030
The chart to the right shows adoption assumptions for AI in 2030. Lower capability AI systems have been widely adopted across sectors, helping with everyday tasks. AI systems in this scenario are relatively user-friendly, which helps with uptake across skill levels. AI systems from authoritarian states are not adopted in the public sector or by large businesses. Authoritarian states with stricter controls generally use AI in a more widespread way.
Data from this graphic shown below.
AI ‘Wild West’, Adoption Levels Low-High
People | Private and Public Sector | Automation | International | ||||||
---|---|---|---|---|---|---|---|---|---|
Higher Skilled | Lower Skilled | Large | Start-ups | Other SMEs | Public | Cognitive tasks | Physical world tasks | UK | Rest of world |
High | Medium Low | Medium | High | Medium | Medium Low | High | Medium Low | Medium | Medium High |
AI ‘Wild West’ - wider context in 2030
Social: The public have mixed views over the increasing use of AI. Many highlight the public benefits that these systems enable as well as the improvements in technical safety procedures implemented by big tech companies. However, there has been difficulty in preventing a range of harms, including the use of AI for radicalisation, disinformation, and increasing disengagement with democratic processes. Schools are grappling with how to educate a generation of young people whose notions of ‘facts’ are confused by exposure to AI generated content.
Economic: From the early 2020s, generative AI tools deployed for administrative tasks across business sectors start to have a positive impact on productivity. AI assistants play a role in improving access to digital skills, although this primarily benefits younger people and there are concerns overreliance on these tools is leading to reduced creativity. Later in the decade, the digital economy becomes a further driver of economic growth, with new business sectors offering novel services and digital products. However, the increase in fraud, with significant financial losses for businesses and individuals, acts as a drag on the economy.
Technological: Development and use of AI systems have become entangled with roll-out of the next generation of internet technologies, based on new digital platforms that are more open and interoperable. There is an explosion of start-ups taking advantage of this new environment, which facilitates the spread of diverse AI tools but makes it easier for AI misuse to go unchecked.
Environmental: An increasing number of start-ups are focussing on AI for environmental applications and improving sustainability. AI environmental monitoring can detect important real-time changes in the environment, and the data from this is used to make decisions about managing these environmental changes. AI systems are also increasingly used to analyse and manage energy consumption.
AI ‘Wild West’ - Key policy considerations
Opportunities
- New businesses are developing popular new products and services with diverse AI systems.
- People are empowered to use AI in a way that suits them, as diverse systems meet a range of needs.
- Productivity improvements offer the potential for improved living standards if economic policy can facilitate this.
Implications for policy in the near term
- Whilst a few large US-based technology companies will likely dominate the Frontier of AI in the short term, that is not guaranteed longer-term. Policymakers will need to consider how regulations and laws would work in a world where development slows down and smaller businesses or non-US developers catch up.
- Criminal access to Frontier AI could increase the severity and volume of offences and online harms. Policymakers working in law enforcement and national security will need to consider development of systems to manage high volumes of misuse, possibly using AI themselves. Online content moderation systems and laws may need to be adapted in a similar way.
- In a scenario with widespread AI misuse, public services are likely to be a major target for attacks. Policymakers will need to ensure the public sector is equipped with the tools and technical expertise to remain resilient to such attacks.
- A diverse market for AI systems will likely bring benefits for users of these tools, as well as innovation in capability and applications. Any regulations that make it more difficult for new AI developers to compete against incumbents will need to consider this trade-off.
- In all scenarios, there will be benefits from upskilling the population in awareness and use of AI, to help mitigate risks like disinformation, and ensure everyone can enjoy the benefits of AI. This scenario highlights the importance of systems to help the public identify AI generated content when it becomes impossible to distinguish from human generated content.
- In all scenarios, higher levels of global cooperation would help to prevent or mitigate negative outcomes. Fostering this cooperation is therefore a key ‘low regret’ action for policymakers. This scenario highlights the challenge of regulating a diverse global market, operating with different approaches to governance at a local level.
5.7 Advanced AI on a Knife Edge
Data from this graphic shown below.
Scenario | Capability | Access | Saftey | Use | Global Cooperation |
---|---|---|---|---|---|
Advanced AI on a Knife’s Edge | 5 | 1 | 4 | 5 | 2 |
A big lab launches a service badged as AGI and, despite scepticism, evidence seems to support the claim. Many beneficial applications emerge for businesses and people, which starts to boost economic growth and prosperity. Despite this system clearing the agreed checks and guardrails, there are growing concerns that an AI this capable can’t be evaluated across all applications and might even be able to bypass safety systems.
See ‘Quick guide to the scenario narratives’ at the start of Chapter 5 to learn how each section was created.
Scenario detail in 2030
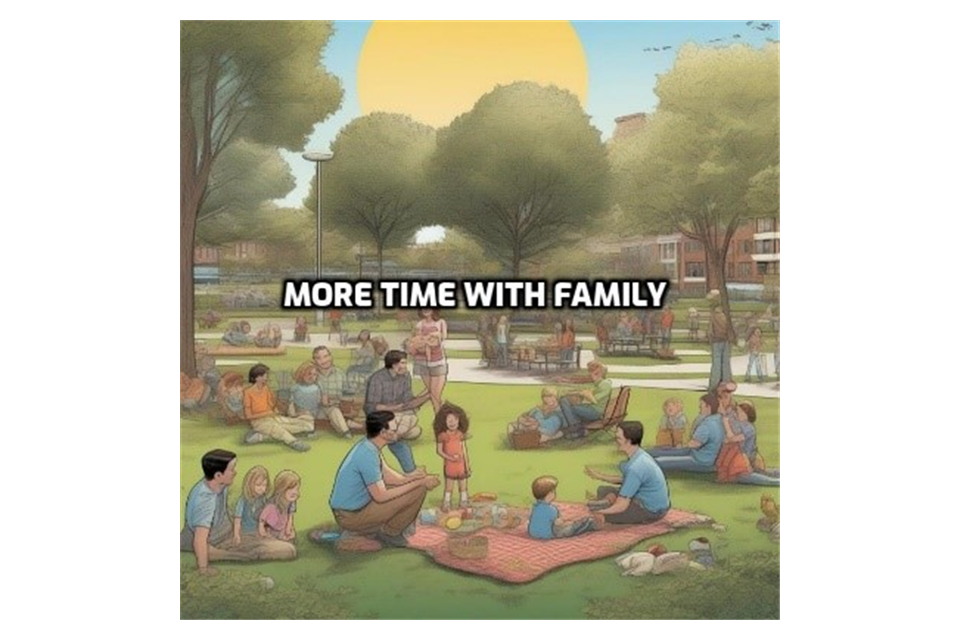
An AI generated image showing families gathered in a park.
Figure 35 – Generated with AI Comic Factory. We prompted the tool to show a happy scene of families in a park.
Capability. Through the mid-2020s, architectural breakthroughs and increases in available compute and training data supported ongoing rapid improvements in AI capability. As 2030 nears, a big tech firm claims to have developed an ‘AGI’.
This system exhibits long-term memory and reasoning skills, being able to work on the same task for several days or weeks, as well as the ability to complete complex tasks requiring multiple planning steps.
This system can operate autonomously, devising its own sub-goals and requiring little or no human oversight. Crucially, this system demonstrates seemingly boundless generality, completing almost any task without explicit training.
There is convincing evidence that the system might be capable of rapid self-improvement although this has so far been managed by existing safety mechanisms. The system possesses multimodality as well as an impressively accurate real-world model. It has already been connected to improved robotic systems, with carefully designed guardrails, to carry out physical tasks.
Key question: How can we evaluate the broad range of capabilities of such a highly generalised AI system?
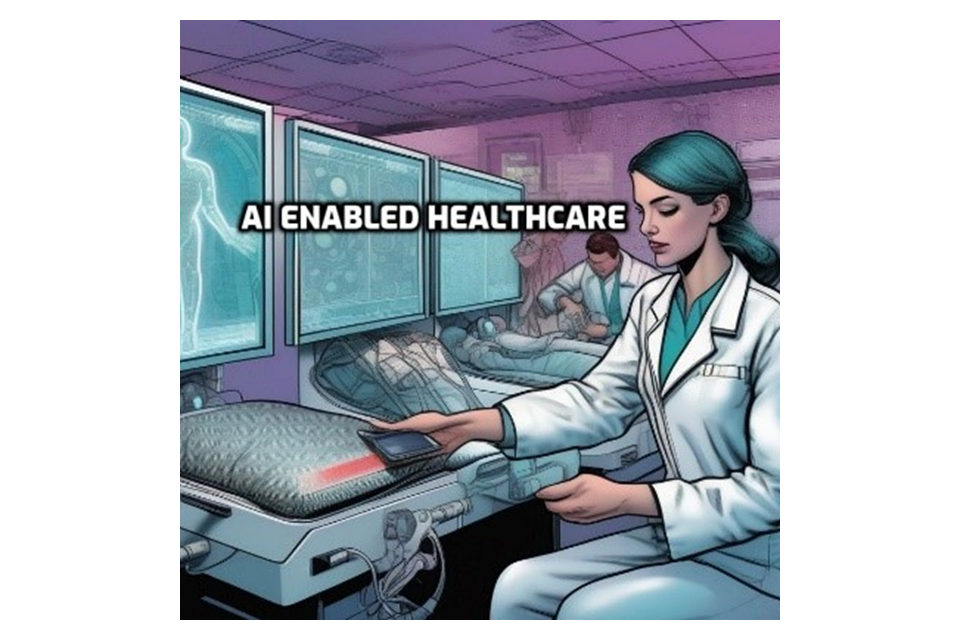
An AI generated image showing AI enhanced healthcare machines.
Figure 36 – Generated with AI Comic Factory. We prompted the tool to depict lab technicians using AI in healthcare.
Ownership, constraints, and access. The AGI-like system has been developed by a big tech company, requiring vast amounts of compute and training data. Other Frontier labs are expected to be close behind, with a big influx of investment to try and replicate the results.
Access to these advanced tools is restricted to paying users and businesses (although it is affordable to most) and the architecture and weights of the system have not been made public.
Cyber security is being invested in heavily, with many hackers trying to gain access, and concerns these systems could find their way into the hands of bad actors.
Smaller start-ups and the open source community continue to develop AI systems and tools, but nowhere near the level of capability of the Frontier labs.
Key question: A huge amount of power sits with private companies – how do governments respond?
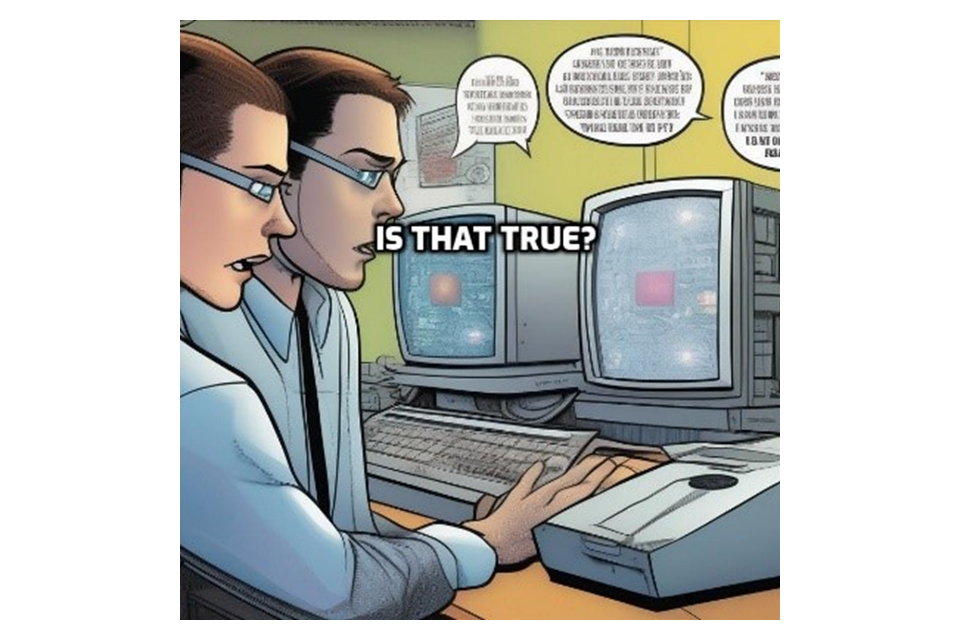
An AI generated image showing 2 IT technicians looking concerned at a computer due to AI misinformation.
Figure 37 – Generated with AI Comic Factory. We prompted the tool to depict a worker in an office looking worried.
Safety. Throughout the 2020s, Frontier labs and tech companies have worked to develop safety measures and guardrails in tandem with continued scaling of AI capability.
However, the development of a system badged as ‘AGI’ has raised concerns that existing measures may no longer be sufficient to evaluate its vast range of possible applications. Confidence in detection of AI deception is low and evaluators aren’t sure if results accurately reflect expected behaviour, or whether the AI is conforming to expectations because it recognises it is being evaluated.
There are also concerns that the system might be able to evade guardrails to carry out its own training runs or improve its own code. Some researchers think this could trigger uncontrolled development of a superintelligence, which could lead to catastrophic impacts if it had access to critical systems. Even if such a risk doesn’t come from losing control of the system, there could be serious consequences if it fell into the hands of bad actors.
Key question: How can we be confident in evaluation results if an AI system becomes aware it is being evaluated?
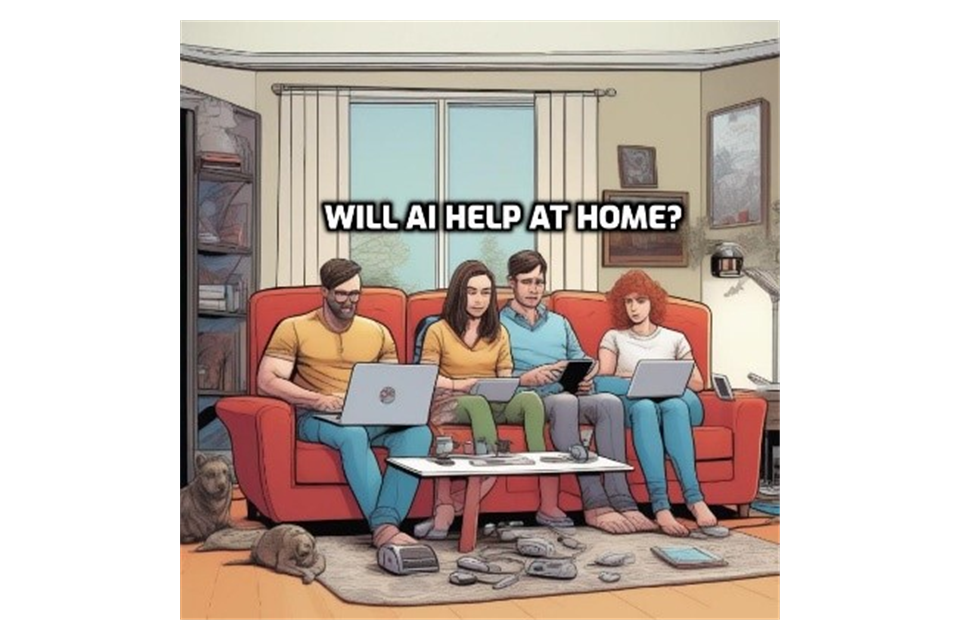
An AI generated image showing a family at home using AI smart-home technology.
Figure 38 – Generated with AI Comic Factory. We prompted the tool to depict a family in their living room using technology.
Level and distribution of use. The continued increase in AI capability throughout the 2020s has resulted in widespread adoption of AI tools by businesses, facilitating productivity increases across a range of sectors.
Although this has caused notable disruption to labour markets, the integration of these tools has been reasonably well managed. Some employers have used tools to augment working practises rather than fully displace workers, using the resultant gains to implement shorter working weeks.
Most people are happy to integrate these systems into their daily lives in the form of advanced personal assistants.
And given AI is also playing a role in solving big health challenges, many feel positive about its impacts on society.
However, with the recent development of an ‘AGI’ system, bigger disruptions to labour markets are on the horizon, and there are an increasing number of expert commentators who raise serious concerns about the existential risks that could be around the corner. Will this concern cause a U-turn in levels of AI use?
Key question: Once we become dependent on such an advanced AI system, how can its use be paused to resolve safety concerns?
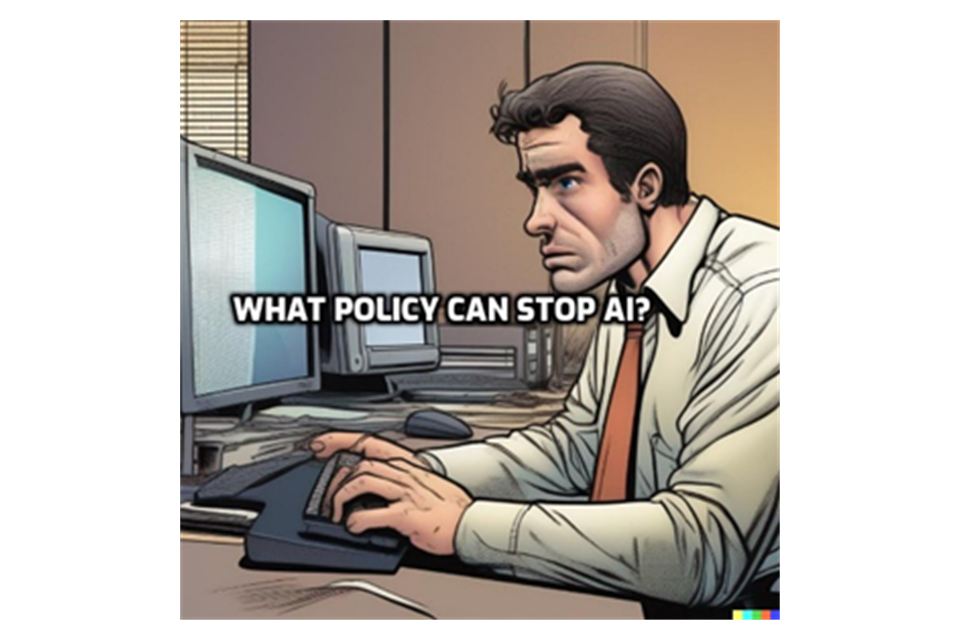
An AI generated image showing a policy worker attempting to regulate AI.
Figure 39 – Generated with AI Comic Factory. We prompted the tool to depict a worried government worker on a computer.
Geopolitical context. Throughout the 2020s, leading technology companies and governments across the world make clear their intentions to collaborate on the development of safe and reliable AI systems. Some progress is made, especially around the development of safety measures.
However, there is a challenging lack of agreement around how to ensure safety from the new generation of AI systems, particularly given how embedded AI use has become across society. The concentration of power that sits with the big tech companies is also seen by some as a barrier to effective global regulation.
In addition, there is increasing concern over how to prevent bad actors accessing and misusing the AGI-like system. The disagreement and the complexity of the issues mean progress on cooperation is slow.
Key question: How can concerns over potential catastrophic impacts be translated into global action on control of advanced AI systems?
How did we get here? Key turning points in 2025
- A major architectural breakthrough allays concerns that scaling laws are reaching their limit and sets Frontier labs on the path to developing increasingly advanced AI.
- Companies who augment workers with AI, rather than make people redundant, are found to perform better, but as AI capability rapidly increases, it’s unclear how long this will last.
- Competition between Frontier labs leads to rapid development of more capable systems.
- A highly capable AI system is the driving force behind a major medical breakthrough, promising widespread benefits. Public perception swings in favour of continued development of more advanced AI systems and increased integration into society.
Frontier AI Adoption in 2030
The chart to the right shows high-level adoption assumptions for Frontier AI in 2030. The availability of highly capable, easy-to-use systems that are embedded into software and other products means that overall adoption is very high in this scenario. Adoption is slightly lower for lower skilled people, SMEs and the public sector. The UK is not world-leading in the development and adoption of advanced AI systems but remains competitive internationally and has benefitted from the majority of opportunities that advanced AI has so far presented.
Data from this graphic shown below.
Advanced AI on a Knife Edge, Adoption Levels Low-High
People | Private and Public Sector | Automation | International | ||||||
---|---|---|---|---|---|---|---|---|---|
Higher Skilled | Lower Skilled | Large | Start-ups | Other SMEs | Public | Cognitive tasks | Physical world tasks | UK | Rest of world |
High | Medium High | High | Medium Low | Medium Low | Medium Low | High | Medium | Medium High | High |
Advanced AI on a Knife Edge - wider context in 2030
Social: By 2030, continued rapid progress in AI capability has so far resulted in increased prosperity, with many people having improved wellbeing supported by a better work-life balance. Although some have been disrupted by rapid change, there is a generally positive public perception of the technology. However, the newly developed “AGI” system has positioned the technology on a knife-edge: does it pose a genuine catastrophic risk or does it promise revolutionary opportunities for society? In either case, major upheavals and disruptions to society are on the horizon. Even if risks can be managed, there will be winners and losers, and rapid changes that many find unsettling.
Economic: The adoption by businesses of increasingly advanced AI systems has resulted in significant productivity increases for businesses that have adopted the technology. Sectors where tasks and processes can be conveniently automated have benefited the most from AI, resulting in economic growth and prosperity. That said, businesses have recognised the value of human-AI interaction to successfully complete tasks, and this is supported by AI tools being easy for most workers to use. This results in limited net job losses. Countries where Frontier labs are based have gained a strategic economic advantage from significant investment drives.
Technological: Throughout the 2020s, advances in AI have been both facilitated by developments in other technologies (e.g. robotics, neurotechnology) as well as driving technological progress in other fields. The development of a highly capable and generalised AI system with physical world capability has the potential for a vast range of revolutionary technological advances.
Environmental: The end of the 2020s saw the development of the most powerful and compute-intensive AI models to date. The huge energy requirements of these systems pose an increasing challenge to rolling out sufficient renewable energy infrastructure to power the green economy of the 2030s, or worse, result in burning of more fossil fuels in cases where servers sit in countries with more carbon-intensive electricity grids. Breakthroughs in science and technology facilitated by the AGI system could potentially have huge environmental benefits, e.g. speeding up delivery of fusion energy and carbon capture technologies.
Advanced AI on a Knife Edge - Key policy considerations
Opportunities
- The use of advanced AI across the economy increases growth with the potential to significantly improve living standards.
- Advanced AI systems can improve services for the public and drive innovation in health and other beneficial areas.
- People may be able to spend less time working and more time doing the things they enjoy.
Implications for policy in the near term
- As Frontier AI systems become more generally capable, it will become challenging to evaluate their safety across all possible applications. Effective AI evaluation of a given model will therefore either need an approach that doesn’t rely on testing all possible applications, or continues for a sufficiently long period after a model has been deployed.
- As AI systems get more capable and integrated into the economy and our lives, it may get more difficult to slow down, patch or roll back releases of new AI models if they do things that raise concerns post-deployment. Policymakers will therefore need to ensure AI developers design rapid, effective recall and containment mechanisms well in advanced of such a situation arising.
- A huge amount of power will sit with whoever controls a highly general, advanced AI system. Policymakers will need to consider how to effectively regulate any businesses who wield this power, and what measures are needed to ensure these systems don’t fall into the wrong hands.
- This scenario has the most significant potential benefits, but it also has the most potential to end with a catastrophic outcome. Making risk-reward calculations is likely to be most challenging in this world. It is important to note that some experts we spoke to felt such outcomes were implausible by 2030, but others did think this scenario was sufficiently plausible to be worth planning for.
- In all scenarios, there will be benefits from upskilling the population in awareness and use of AI, to help mitigate risks like disinformation, and ensure most people are able to enjoy the benefits of AI. This scenario highlights the potential for skills we see as important today being lost if we start to rely on highly advanced AI. What risks could this reliance expose us to if the AI system has to be shut off?
- In all scenarios, higher levels of global cooperation would help to prevent or mitigate negative outcomes. Fostering this cooperation is therefore a key ‘low regret’ action for policymakers. This scenario highlights the risk that ownership of advanced AI systems could see an increase in the market power of big tech companies, making effective global governance even more challenging than it is today.
5.8 AI Disappoints
Data from this graphic shown below.
Scenario | Capability | Access | Saftey | Use | Global Cooperation |
---|---|---|---|---|---|
AI Disappoints | 1 | 1 | 4 | 2 | 3 |
AI capabilities have improved somewhat, but the Frontier is only just moving beyond advanced generative AI and incremental roll out of narrow tools to solve specific problems (e.g. in healthcare). Many businesses have also struggled with barriers to effective AI use. Investors are disappointed and looking for the next big development. There has been progress in safety, but some are still able to misuse AI. There is mixed uptake, with some benefiting, and others falling victim to malicious use, but most feel indifferent towards AI. See ‘Quick guide to the scenario narratives’ at the start of Chapter 5 to learn how each section was created.
Scenario detail in 2030
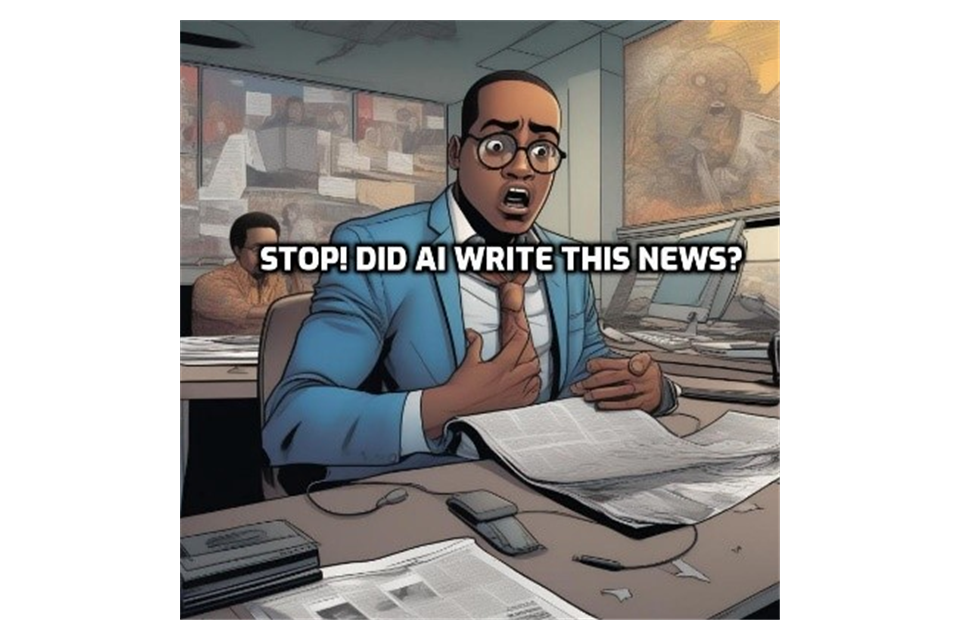
An AI generated image showing a news reported looking shocked at AI content in the newsroom.
Figure 40 – Generated with AI Comic Factory. We prompted the tool to depict a newsroom filled with shocked journalists.
Capability. During the 2020s, AI developers achieved some innovation beyond current capabilities, but not has much as expected. Cognitive based tasks like text production are improving but in cases where factual accuracy is important there are still risks that systems will make mistakes. AI systems also struggle to complete multi-step tasks like complex reasoning or high-quality video generation, and they usually require close human oversight.
By 2030, semi-autonomous AI agents are in use but only by those who have overcome practical implementation barriers.
Key question: How much more development is needed before we can be confident in the performance of AI systems in applications where accuracy is important?
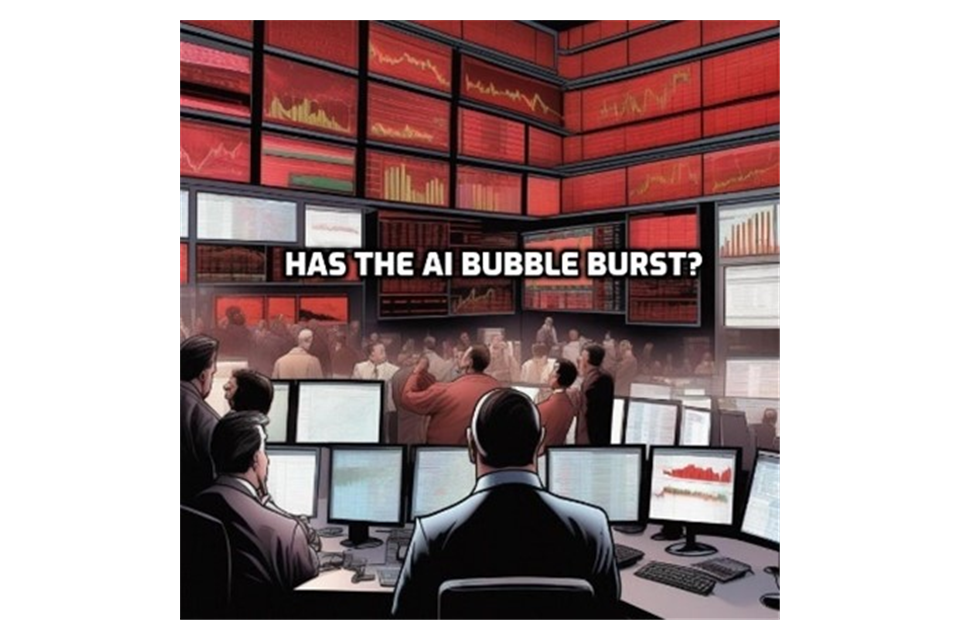
An AI generated image showing a crisis room with red screens and people reacting to AI caused breakdown.
Figure 41 – Generated with AI Comic Factory. We prompted the tool to depict a trade floor undergoing a market crash.
Ownership, constraints, and access. Big labs still lead the industry, but progress is slowed by stubborn problems around system accuracy and output quality.
Problem solving in these areas is taking longer than expected. Challenges stemming from a shortage of high-quality data contribute to this delay. These setbacks allow space in the market for open source developers to catch up.
The overarching slow pace of development drives away deep-tech investors who shift their focus and resources to fusion energy and quantum computing, where breakthroughs are starting to be made.
Key question: Might a slowing of AI development cause a drop in investment, which could reinforce the slowing of development?
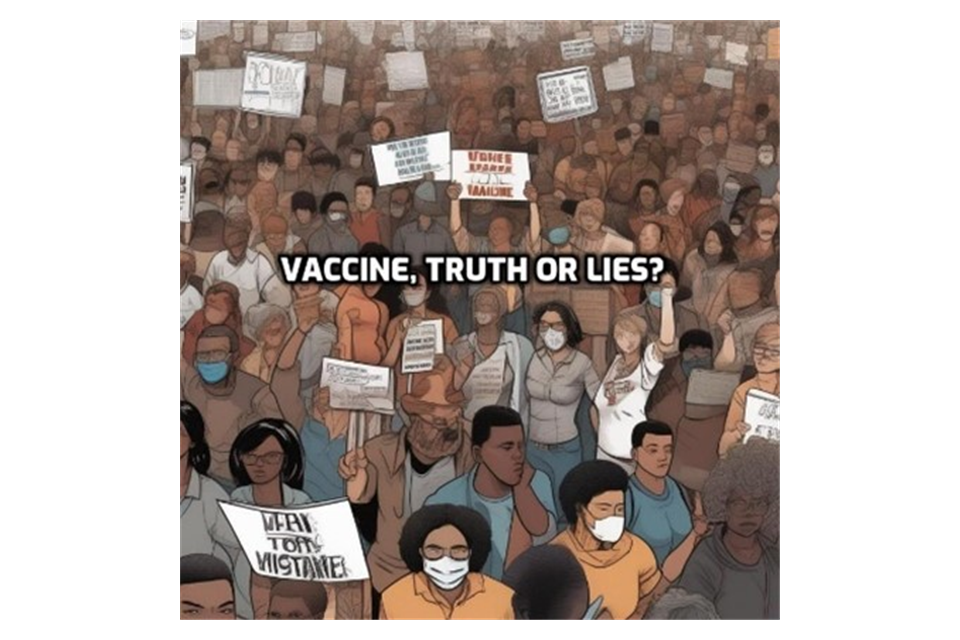
An AI generated image showing a large crowd of people protesting a vaccine developed by AI.
Figure 42 – Generated with AI Comic Factory. We prompted the tool to depict a protest against a new vaccine.
Safety. By the late 2020s there has been some progress in the technical safety of AI systems, the slow pace of capability development providing space for AI safety researchers to make a number of breakthroughs.
However, there are notable cases of malicious AI misuse that target vulnerable groups in society. To some degree, harm is also caused by the inaccuracy of AI systems – some may be subject to unsound decisions that don’t reflect reality.
Key question: How can those affected by unsound decisions report them and ensure AI systems are changed in response?
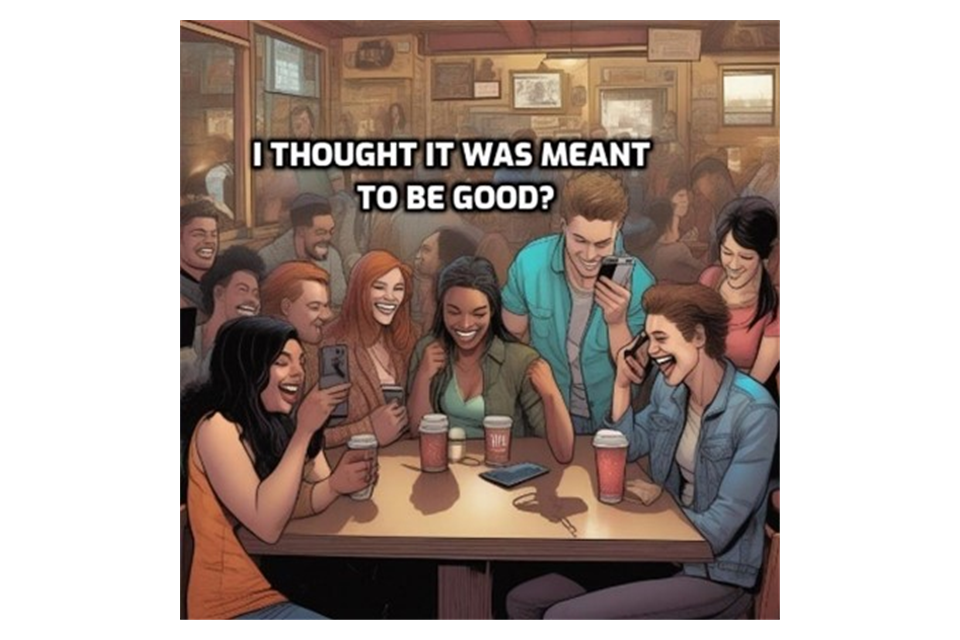
An AI generated image showing people reacting happily while talking and looking at their phones.
Figure 43 – Generated with AI Comic Factory. We prompted the tool to depict people laughing at the failed promise of AI.
Level and distribution of use. Lower-than-expected capability of AI systems has led to disillusionment, a slow-down in investment and limited use by the business community.
Many companies’ existing data structures were not set up to get the most out of AI systems, and they did not have the skills to adequately resolve these issues.
The usefulness and impact of AI was overestimated, with parts of the population experiencing few AI-driven lifestyle changes, and this is only reinforced by inequitable access to training. Those with the right skills enjoy the benefits of AI but many struggle to learn how to effectively use the temperamental AI tools on offer.
Ongoing cases of biased AI decision making are quickly reported by the media, adding to lukewarm perceptions of the benefits of AI.
By the late 2020s, there are examples of advanced AI systems in operation, but enthusiasm is tainted by peoples’ experiences earlier in the decade. Some companies marketing AI-based products avoid disclosing their use of AI, in case it impacts sales.
Key question: Will practical barriers, like legacy IT systems, be a stubborn barrier to increasing levels of AI use?
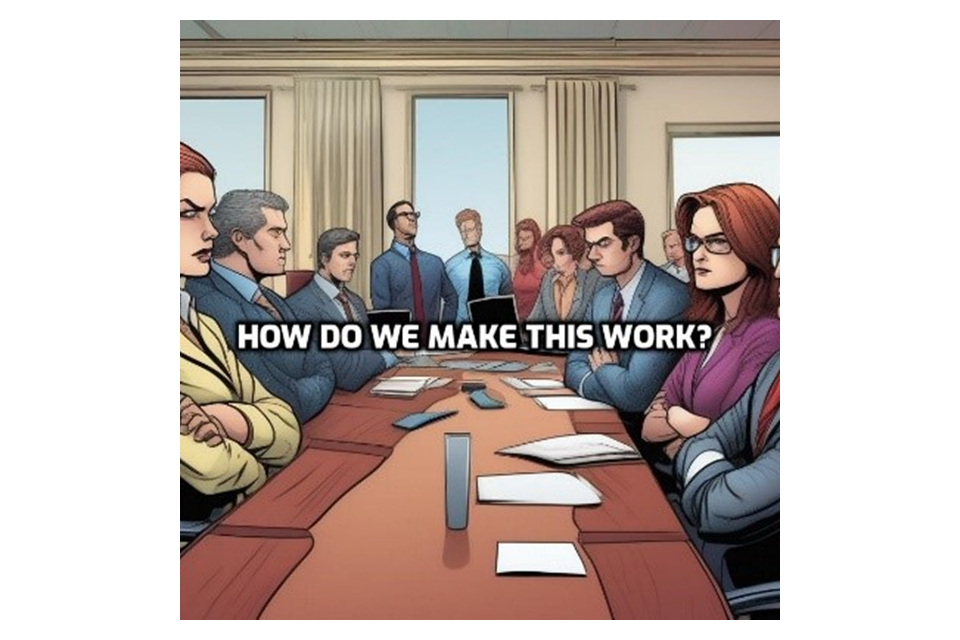
An AI generated image showing a conference room full of people discussing the solution to AI failing.
Figure 44 – Generated with AI Comic Factory. We prompted the tool to depict business leaders of mixed genders at a board meeting.
Geopolitical context. Towards the late 2020s there is a growing willingness to collaborate internationally, especially as the effects of climate change worsen, and some adverse impacts of AI become significant enough to take action on. One factor that affects the UK’s position in this international cooperation is a skills shortage, both in AI and other technical skills. This could lead to an urgent need for the UK to attract skilled migrants and could potentially impact on international relations.
Key question: Will collaboration on other global challenges help to accelerate action on governance of AI?
How did we get here? Key turning points in 2025
- An ‘AI bust’ causes a brief financial crisis from rapid disinvestment when a number of high-profile AI systems are delayed in their rollout due to ongoing accuracy problems. Many start-ups go bust and there is market consolidation towards big tech firms.
- AI companies are widely derided in the media for over-promising on system capability, contributing to disinvestment and low levels of use.
AI Disappoints - Frontier AI Adoption in 2030
The chart to the right shows high-level adoption assumptions for Frontier AI in 2030. Levels of AI use are relatively low, with the highest levels of use amongst higher skilled people, and in bigger or more tech-savvy businesses. However, even these uses are low compared to other scenarios.
Data from this graphic shown below.
AI Disappoints, Adoption Levels Low-High
People | Private and Public Sector | Automation | International | ||||||
---|---|---|---|---|---|---|---|---|---|
Higher Skilled | Lower Skilled | Large | Start-ups | Other SMEs | Public | Cognitive tasks | Physical world tasks | UK | Rest of world |
Medium | Low | Medium | Medium | Low | Low | Medium | Low | Low | Low |
AI Disappoints - wider context in 2030
Social: In the early 2020s society was excited over the basic capabilities of Frontier generative AI systems. But the lack of accuracy and complex functionality of the systems leads to the public quickly losing interest. By the late 2020s the lack of advancements in AI leads to a disillusioned public, who are more concerned with the adverse impacts of climate change. Several high-profile misuse cases provoke mistrust of AI and a public call for improved regulation and safety. By 2030, AI systems with improved performance are becoming more embedded in software and services again, but this is done without much fanfare due to negative public perceptions of AI. There is also more limited scrutiny of this less overt use of AI in online services, with implications for the user, including worsening online addictions and polarisation.
Economic: AI systems have over-promised and under-delivered across business sectors, with only marginal improvements in productivity. The AI systems and models that are in use are mostly simple machine learning algorithms and tend not to be branded as ‘AI’. Most jobs remain uninfluenced by AI, as systems are unreliable and incapable of preforming complex tasks. As businesses and governments begin to accept that AI is not the silver bullet for ongoing productivity stagnation, more begins to be invested in human capital and other technologies. AI breakthroughs could be on the horizon in the 2030s, but the disappointments of the 2020s will not be forgotten easily.
Technological: About the same time that AI disappointments start to become apparent, there are developments in other emerging technologies, including quantum computing and fusion energy. Whilst these aren’t game-changing breakthroughs, they are big enough to divert the attention of investors, who start to direct finite investment pots away from AI systems into these more promising technologies, which could be as important for solving big global challenges as AI was once thought to be. Many AI researchers despair at this rapid U-turn as they see many of the performance problems of AI systems as fixable if only the investment was there.
Environmental: The impact of AI system use on the environment is marginal – compute demands continue to increase but at a relatively slow rate, and efficiency improvements offset this somewhat. However, the failure to deliver reliable AI systems that can be effectively used to protect the environment and improve sustainability is also a disappointment.
AI Disappoints - Key policy considerations
Opportunities
- The scale and severity of AI misuse is relatively low in this scenario compared to others.
- The slower pace of development may make it easier for organisations to deploy AI in a careful, considered way that minimises negative side effects, as long as they can secure the right AI skills.
- Many jobs remain unaffected by AI, causing relatively limited job displacement.
Implications for policy in the near term
- Policy makers are looking to Frontier AI as a potential solution to long-term stagnating productivity, which is adversely impacting living standards and the public finances. If AI doesn’t develop as anticipated or proves difficult for many organisations to use in practice, policy makers seeking to boost productivity may need to focus on helping to remove barriers to adopting the technology, including skills.
- Even if AI isn’t grabbing the headlines, policymakers will need to ensure existing AI safety issues, like disinformation, are not neglected. Policymakers may also need to be prepared that businesses could choose to not brand AI-based systems as ‘AI’, due to negative public perceptions, which could make them more difficult to regulate.
- There is a possibility of several disappointing years for AI development, but more significant and beneficial developments beyond 2030. In a scenario with rapid market disinvestment from AI, policymakers should consider whether there could be long-term, strategic benefits from maintaining UK AI skills and infrastructure during this slow-down.
- In all scenarios, there will be benefits from upskilling the population in awareness and use of AI, to help mitigate risks like disinformation, and ensure most people are able to enjoy the benefits of AI. This scenario highlights the importance of data and software engineering skills that will be needed to practically integrate AI tools into organisational IT systems, and that a lack of these skills could be a significant barrier to AI adoption.
- In all scenarios, higher levels of global cooperation would help to prevent or mitigate negative outcomes. Fostering this cooperation is therefore a key ‘low regret’ action for policymakers. This scenario highlights that a slowdown in development of AI could make it easier to reach a global consensus on how to regulate the technology, but there is also a risk this could cause policy makers to deprioritise the issue.
6. Public Engagement
We undertook a public engagement exercise, drawing on deliberative methods, with the aim of understanding how the public feels about the possible future developments in AI outlined in our scenarios.
6.1 Key findings from the engagement
Members of the public we spoke to felt that safety is paramount. There was a consensus among participants that safety was a higher priority than other factors. They found risks easier to imagine and tangible, whilst key opportunities for the future were harder to grasp and value.
Members of the public we spoke to felt that government and regulators are responsible for ensuring the safe use and development of AI, as well as safeguarding jobs. They also felt that organisations using AI were responsible for following regulation and behaving ethically, in order to build trust with the public.
6.2 Engagement introduction
As part of this project, we wanted to explore public sentiment and attitudes towards AI and the range of future outcomes in our scenarios. In support of this, we ran a public engagement, in partnership with the Centre for Data Ethics and Innovation (CDEI) and Thinks, a global insight and strategy consultancy.
Deliberative public engagement is a well-established means of providing qualitative understanding of public feeling on a particular topic1. In previous Foresight projects we have used public engagement, to improve our understanding of societal perceptions and test public reactions to scenarios . By combining narrative futures with deliberative public engagement, we can gauge public opinion on events that have not yet happened, and the factors that may drive change in the future.
To meet the project deadlines this engagement was conducted on a smaller scale and more rapidly than would ideally be the case. This means this research has some limitations in terms of the breadth and depth of the opinions gathered from the public. Experts we spoke to agreed that large-scale, deliberative public engagement will be needed to capture a wider range of perspectives across a greater range of AI-related issues to inform the development of AI safety systems and regulations. A further larger study could also build on our public engagement, informed by the issues we found the public were most concerned with.
Research objectives
The research objectives were to understand:
- how the public feels about these future scenarios – including the risks and opportunities of each;
- perceived plausibility of each of the future scenarios;
- which elements depicted in the scenarios the public feels particularly strongly about, whether positively or negatively; and
- what the public thinks will need to change, or stay the same, to reach a positive future scenario.
Public workshops approach
Thinks convened a total of 32 participants across 3 locations with a sample diverse in demographics and opinions on AI. Each participant took part in 2 workshops, allowing for learning, reflection, and deeper insight than a focus group approach. Participants were not ‘informed citizens’ as they would have been in a full dialogue or deliberative process, but by extending discussions across 2 workshops and drawing on deliberative methods Thinks were able to explore their views in depth and get beyond knee-jerk reactions.
Workshop one took place face-to-face and began with an exploration of participants’ baseline understanding of AI. The workshop included an initial prioritisation phase where participants were asked to consider the relative importance of safety, capability, use, and constraints when thinking about the future of AI in the UK. They also reflected on how these factors interact with one another, and how these interactions affect their prioritisation. To help them talk about these issues the following factors were provided to participants:
Safety - How likely a system is to make mistakes or be mis-used. A safer AI system is more technically reliable, well understood and appropriately used.
Capability - How many different types of tasks a system can successfully achieve. A more capable AI system is more accurate and efficient, requires less human oversight and can complete more varied tasks.
Use - How many different types of people and organisations are using AI. Higher use of AI systems means more widespread use and more skills and training.
Constraints - Things that could slow down the development of AI. More constraints on AI means stricter regulation, stronger laws and more limited access to the data and powerful computers needed for AI systems.
The ‘Constraints’ factor is a version of the ‘Ownership, access, and constraints’ critical uncertainty that shaped the scenarios, but adapted to include regulations or laws as an additional potential constraint. The rationale for this is that we wanted to give participants an opportunity to discuss regulations and laws in this part of the exercise, which would not have been possible if we had used the original critical uncertainties.
Participants then explored the five scenarios, using summaries and stimuli provided by facilitators. At the start of the session, participants were told that the scenarios have been designed to focus on the risks that could come about in the absence of any potential new AI regulations (as described above). Participants were also reminded throughout the session that none of the scenarios are particularly positive because of this design choice, not because more positive futures aren’t plausible. With this context, they were then prompted to consider the opportunities, risks, and plausibility of each. To conclude, participants revisited their views on the importance of safety, capability, use and constraints when preparing for a future with AI, informed by their understanding of the potential outcomes.
Participants started workshop two, online, by reflecting on key findings and the overarching risks and opportunities identified in their previous discussions. This provided greater confidence in the risks and opportunities identified in workshop one as participants reviewed researcher analysis. Thinks then led participants through a process of clarifying and prioritising the key opportunities and risks; exploring the barriers to achieving opportunities and motivators that would lead to risks; and the actions that should be taken, by specific actors, to mitigate risks and enable opportunities.
All workshops were recorded and a trained notetaker entered notes from each breakout discussion into a structured grid for thematic analysis.
6.3 Key findings
Prioritisation
Key message for policymakers – When developing AI policies and strategies, policy makers should be aware that members of the public we spoke to prioritise safety above other considerations. Participants felt that AI should have the capability to be useful, but never at the expense of safety, with safety measures being introduced ahead of capability developments. Policy makers should consider how best to achieve this balance and to communicate these mitigations to the public in an accessible way.
What the participants said - When considering the factors of safety, capability, use, and constraints, in relation to the development and impact of AI up to 2030, there was a consensus that safety was the highest priority for the UK. The need for high safety informed how the other factors were defined and prioritised. For example, constraints were seen as a proxy, or enabler, of safety, while use and capability were important but not at the expense of safety.
“It has to be safety. You have to protect people and businesses. Safety first. We may lose some use because they are directly linked. What we’d gain is that people would trust (AI).”
Discussions found there was a need for balance between these factors as higher capability was assumed to lead to more useful applications of AI for society and could also lead to safer applications and use. However, there was a concern that the more useful AI became the more accessible it would become to bad actors, leading to less control, and therefore less safety. In which case breadth of use was felt to be less important than the existence of appropriate constraints and that capability should never come at the expense of safety.
Participants also wanted to avoid a scenario in which AI had a lower capability and was therefore unable to achieve meaningful benefits. But they felt more comfortable with less capability in favour of safety.
Due to discussions being framed in terms of what society could, or should, look like in 2030 there was a temporal aspect to these conversations. Participants wanted the focus of AI to start around safety and then for work to move slowly and safely towards developing higher capability and more widespread use.
Risks
Key message for policymakers – Members of the public we spoke to were concerned about a wide range of risks posed by AI. Participants were most concerned with risks related to crime and economic or societal disruptions, and that these would have unequal impacts across society. As policy makers develop mitigations for these risks, they should consider how best to communicate these mitigations to build public confidence.
What the participants said - Participants found risks easier to imagine and articulate than opportunities, as they felt more tangible. This is likely to be influenced by the scenarios being designed to highlight risks and challenges, but participants also expressed concerns more than interest or excitement before seeing the scenarios. The key risks they identified for the future were mass unemployment, unfair distribution of financial gains and wider benefits, an increase in the amount and sophistication of crime, loss of social connections, autonomy, and skills, and AI as a threat to safety.
“If people lose their jobs to AI what will they do? You’re already seeing call centres shut down. You can’t just put them on a ship and send them off.”
There was a feeling that AI would not affect groups in society equally. Job losses were seen as an inevitable outcome of AI development, although there were mixed reactions over which jobs can and should be replaced by AI. This mass job loss was expected to further economic inequality and be driven by differences in AI-related skills and knowledge. Participants expected that this inequality would be particularly linked to generational differences with older generations expected to find it harder to adapt both to new skills, digitisation of services, and new forms of communication.
There was also a concern that people may become reliant on AI leading to a lower quality of life through increased ‘laziness’, as well as poorer social skills and connections. There was also a concern that poorly developed general AI applications could cause harm and that, if AI were to fail, people will have lost the skills needed to carry out certain tasks.
Opportunities
“I think medical would be the biggest positive effect you can get from AI; manuals of data to be processed, vaccines, curing a disease - if you can have something that can do that in even half the time it’s worth it.”
Key message for policymakers – Members of the public we spoke to felt that AI could enable significant scientific discoveries and improve their quality of life, but some were sceptical about these opportunities becoming reality. As evidence grows on the positive impacts of AI, and the policies that enable these, policy makers should consider how best to communicate this to the public. This could include demonstrating high-profile positive use cases, e.g., in public services.
What the participants said - Participants found key opportunities for the future harder to grasp or value than risks. Again, this is likely related to the fact that the scenarios are more focused on risks and challenges than they are on opportunities. Despite this, participants did feel that the opportunities for AI were that it could increase productivity, quality of life, economic growth, job opportunities (particularly for the younger generation), innovation, and would help the UK have a global influence and keep up with international scientific developments.
Several barriers and concerns were identified as risking the ability to achieve these opportunities. These concerns included thinking that only existing big corporations would benefit fully from AI due to it being easier for them to implement, that older generations would struggle to retrain, and that current behavioural habits, such as poor exercise regimes and striving for profit over other objectives, would prevent AI improving quality of life even if it improved individual and business efficiency.
Views on Scenarios
More detailed views on the risks and opportunities of AI were gained by examining how participants viewed the scenarios detailed in the previous section. However, as previously mentioned, given the design of the scenarios, the risks were often more prominent than opportunities for participants.
Scenario 1 – Unpredictable Advanced AI
Participants saw the main opportunity in this scenario being ‘big advancements’ made through faster data analysis, such as improving medicine (referring to the stimulus provided). This scenario prompted participants to express their lack of trust in the way AI is developed and tested, and how data is used in this process. Discussion examined the potential for AI to make mistakes or for misuse by bad actors. These concerns overshadowed the potential benefits.
“You can’t stop people from abusing this technology. More people could die through this way through terrorism than be saved via medicine. The fact everyone can use it is too much. You need something a lot safer and maybe not something everyone can access.”
To a lesser extent, participants were concerned about loss of human connection leading to a ‘depressed society’ and that society will become vulnerable to whatever technology we become dependent on.
Participants felt that high constraints would be necessary in this scenario to address their largely safety-based concerns. Participants also felt that this scenario was largely plausible with some feeling that it was ‘already starting to happen’ and that living in this scenario would be frightening and chaotic.
Scenario 2 – AI Disrupts the Workforce
“It makes my job easier because it takes out human error. I can confidently make changes to systems and it makes my life simpler. Five years earlier I would not have known about these automating systems, but I’ve upskilled myself and it’s made my job more enjoyable and easier to do.”
Participants identified a number of opportunities in this scenario, including streamlining of tasks, and generally making tasks easier. They recognised the potential for economic growth, but this was largely overshadowed by confusion around how an economy could grow if “a nation of unemployed people” didn’t have money to spend. Overall, there was a perception that this scenario would risk increasing inequality through job losses and that it would be the business owners and developers of AI who would benefit more than the general public.
This scenario exemplified participants’ concerns about job losses, which were seen as inevitable and likely to lead to increased welfare spending, poorer mental health, and increased public unrest. Older generations were seen to be particularly vulnerable in this scenario as well as those without clear pathways to retrain. Participants supported interventions to manage the consequences of job losses, such as regulating companies and helping people to re-train, funded through reinvested profits from AI use.
Participants saw this scenario, and its subsequent backlash, as inevitable. Although, a small number of participants felt that this scenario was more about managing ‘teething problems’ that come from embedding a new technology in society.
Scenario 3 – AI ‘Wild West’
Participants recognised the potential opportunities as wide-ranging, given a scenario with very diverse AI applications. They discussed a range of ideas, from doing menial tasks like monitoring food supplies and ordering shopping ultimately leading to more free time (per the stimuli) through to smart watches detecting a heart attack and calling emergency services.
“With the way AI is going, is everyone going to have access? There’s going to be cheaper versions or better versions, and it’s going to come down to what you can afford.”
Participants were most concerned about the sophistication of fraud and potential for misuse on a large scale. They felt that this would be hard to police, and this would leave everyone in society vulnerable to bad actors using AI systems. They were also concerned about reliance on AI applications leading to poorer mental health due to decreased social connection, and a reduction in human skills and productivity. Additionally, there was a concern about the use of data and the reliability of AI applications.
Overall, the risks in this scenario were seen to outweigh the potential benefits. The participants felt this scenario was somewhat plausible if government doesn’t regulate AI development well. It also evoked a sense of powerlessness amongst participants as to how companies and governments develop and regulate AI and whether it would leave the public vulnerable to misuse.
Scenario 4 – Advanced AI on a Knife Edge
“There’s a risk that it’d get smarter than you and can bypass security. That’s not a risk worth taking.”
Participants saw the opportunities of this scenario as significant, although difficult to conceptualise This challenge may have evoked the most explicit expression of feeling scared of the unknown. Risks such as losing human autonomy and human inferiority to AI were evoked by this scenario. Cyber-attacks, fraud, data misuse, and privacy concerns were also evoked by this scenario. While not a focus of their discussions, participants felt this scenario could also lead to job losses, and expressed concerns about the minority outlined in the scenario as being without access which could lead to growing inequality and crime. Participants again felt that potential opportunities such as advanced AI assistants and safety in driverless cars were outweighed by risks.
Participants had mixed views on how plausible this scenario is, though some felt it to be quite plausible given the perceived pace of development. Constraints on AI were described as positive and necessary in this scenario to ensure that AI creators navigate risks safely.
Scenario 5 – AI Disappoints
“The others were more gloomy. This is more ‘society is still here, and jobs are still here’.”
This scenario was preferred by participants as it didn’t evoke feelings of fear and avoided key risks outlined in previous scenarios. The main risk identified was wasting resources in the process of developing and purchasing AI, however some felt they were comfortable with accepting wasted resources to avoid risk and felt this scenario would be ideal as it had high safety and high constraints.
Despite the concerns, some participants felt that constraints and safety could be relaxed somewhat in this scenario to help unlock the potential of AI. Caveating that, this should be in balance with, and not at the expense of, safety and constraints to mitigate the risks previously discussed. Others expressed a preference for taking development slowly leading with safety and constraints but working towards better capability and usability.
Participants were conflicted on the plausibility of this scenario with some feeling it was realistic based on past ‘hypes’ which hadn’t delivered. Others felt differently, expecting that AI would be driven forward by society pushing for ‘the next big thing’.
Summary of views on scenarios
Table 4 below provides a summary of participants’ views on the risks and opportunities presented by each scenario.
Scenario | Summary | Risks | Opportunities |
---|---|---|---|
Unpredictable Advanced AI | Highly capable but unpredictable open source models are released. Serious negative impacts arise from a mix of misuse and accidents. There is significant potential for positive benefits if harms can be mitigated. | Potential for serious accidents using AI or for misuse by bad actors. | ‘Big advancements’ made through faster data analysis. |
AI Disrupts the Workforce | Capable narrow AI systems controlled by tech firms are deployed across business sectors. Automation starts to disrupt the workforce. Businesses reap the rewards, but there is a strong public backlash. | Increasing inequality through job losses, and inequality in distribution of benefits obtained from AI. | Streamlining of tasks, and making tasks easier, possible economic growth. |
AI ‘Wild West’ | A wide range of moderately capable systems are owned and run by different actors, including authoritarian states. There is a rise in tools tailored for malicious use. The volume of different AI systems is a problem for authorities. | Reliance on AI applications would lead to societal vulnerabilities, poorer mental health, reduction in human skills, AI misuse. | Varied impacts across sectors from domestic life to healthcare services. |
Advanced AI on a Knife Edge | Systems with high general capability are rapidly becoming embedded in the economy and peoples’ lives. One system may have become so capable that it can bypass safety systems that have been put in place. | Losing human autonomy, human inferiority to AI, cyber-attacks, fraud, data misuse and privacy concerns. | Advanced AI tools and increased safety in certain situations such as in driverless vehicles. |
AI Disappoints | AI capabilities have improved somewhat, but big labs are only just moving beyond advanced gen AI. Investors are disappointed and looking for the next big development. There is a mixed uptake across society. | Wasting resources in the process of developing and purchasing AI. | Avoiding key risks from other scenarios. |
Table 4: Summary of public participants views on the scenarios
Responsibilities
During the workshops, participants also identified responsibilities for four different actors in society. They felt that government and regulators were responsible for safeguarding jobs and ensuring the safe use and development of AI through regulation and monitoring organisations, particularly profit-driven businesses.
“People developing AI have a responsibility, and that should be instilled through government/regulators. Businesses wouldn’t do it on their own, but governments would enforce it. Governments should force businesses to think in terms of the consequences of their actions.”
They felt that organisations using AI are responsible for following regulation, behaving ethically and being transparent with the public to build trust. Participants supported government taking an active role to prevent mass unemployment by preventing companies from making employees redundant and ensuring those who have jobs replaced by AI are retrained and supported to find another role, using taxation to fund reinvestment into society.
They also felt that government is responsible for ensuring people are upskilled and educated about AI and that individuals have a responsibility to actively learn about AI themselves. To support this learning, participants felt that schools and universities have a responsibility to ensure the curriculum is future-proof.
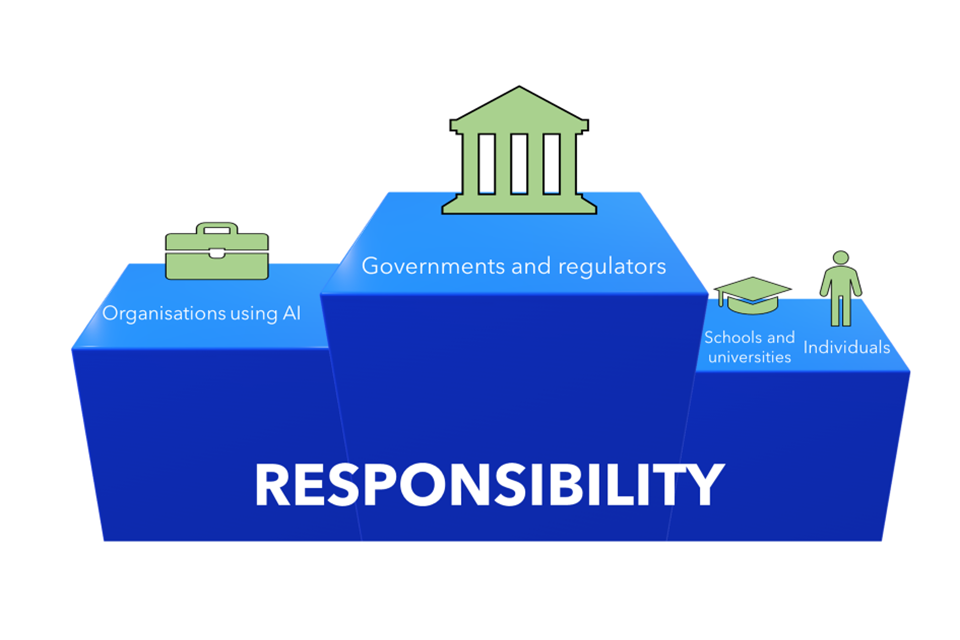
An image showing degrees of responsibility for AI regulation.
7. Conclusions
The research and scenarios presented in this report are intended to help policy makers explore the interacting uncertainties around the future development of AI in a manageable and consistent way to enable policy and strategy alignment across different government departments. The scenarios have been designed to help users explore the potential implications of different AI futures, ultimately helping them to develop policies and strategies that are resilient to risks, and to seize opportunities for the economy, society, and public services. This final section considers how policy makers can use the outputs of the project in their policy development. Other organisations, such as businesses and local authorities, may also find these approaches helpful.
7.1 Key findings
The evidence gathering and scenario analysis that underpins this report has highlighted a series of key findings, which policymakers could consider before using the scenarios themselves to support policy development. These are:
-
Future Frontier AI systems could have widespread positive impacts for society, including improvements in productivity and living standards, better public services, and scientific breakthroughs in health or energy. However, most experts we spoke to agreed that policy makers will need to act to fully realise these benefits, whilst mitigating the potential risks.
-
The risks posed by future Frontier AI will include the risks we see today, but with potential for larger impact and scale. These include enhancing mass mis- and disinformation; enabling cyber-attacks or fraud; reducing barriers to access harmful information; and harmful or biased decisions.
-
New risks could also emerge. What Frontier AI will be capable of by 2030 is highly uncertain but will shape the risks it poses. Risks will also depend on wider uncertainties in access to AI systems, ownership, safety measures, use by people and businesses, and geopolitics.
-
As Frontier AI systems become more generally capable, it will become difficult to evaluate their performance and safety across all possible applications. Approaches to evaluation that address this dynamic will be needed.
-
Use of highly capable narrow AI systems could also present policy makers with challenges, for example if used in widespread automation. This will need to be considered, alongside more general AI systems, when developing risk management policies.
-
Over the next few years, a few large technology companies are likely to dominate Frontier AI. Longer term there is more potential for a shift away from this dynamic. However, in scenarios where highly advanced AI systems remain controlled by a few companies, they will hold a huge amount of power.
-
Highly capable open source AI systems could also emerge. In scenarios where that happens, effective regulations or laws may need to cover any potential developer or user of these systems, not just the original creators.
-
Negative impacts from future Frontier AI could be driven by a range of factors. These include rapid changes in AI capability, malicious use, ineffective safety systems, and wider societal consequences of well intentioned use. Policy makers will therefore need to consider interventions that can prevent or mitigate impacts associated with all these factors.
7.2 Overview of how to use the scenarios
In addition to the findings above, the following practical methods, adapted from the GO-Science Futures Toolkit, can be used to help develop and test strategy and policy.
These scenarios have deliberately been written “policy off” - they don’t include any new policy or approach by the UK government in shaping the future of AI. They all include challenges and problems for policymakers to solve. That’s intended to allow policy makers to test their ideas in each scenario. How well does a given policy perform in each world? Is it still effective? Does it help mitigate harms? Is it conditional on something else happening in this future?
Of course, none of the scenarios will individually or perfectly describe the ‘real’ future we experience. This will likely feature elements of all these worlds. As we made clear in Chapter 4 an alternative, more positive, future is possible. Realising that future is likely to need action by governments. Indeed, it is implausible to imagine the UK government does nothing between now and 2030 to steer towards it. We shouldn’t overstate the influence one government, any government, has over developments. But nor is it accurate to assume that nothing any government does will make a difference. Policies enacted now could help navigate toward a more favourable future than any presented in these scenarios. We recommend three different types of exercise to get the most value out of this work, and identify pathways to a better future:
-
Governments using this work to identify the future they do want for AI. Then working back from there to develop a plan to navigate towards it. We call this backcasting.
-
Exploring how different policy responses perform in each scenario, and how they might need to be adapted to achieve their objectives in different contexts. We call this stress-testing.
-
Testing plans and assumptions against unanticipated shocks to ensure they are sufficiently resilient to a range of possible outcomes.
Backcasting (navigating to a preferred future)
The scenarios presented here are all, for different reasons, unfavourable. So what does a more favourable future look like? There are some obvious things that emerge from the scenarios. These include:
- Stronger international collaboration – a consistent theme of the scenarios is it being much harder to manage challenges if the mitigations governments put in place are not global. It follows that building trust and a common approach to AI is likely to be part of navigating to a more positive future.
- Improving technical safety – in all worlds, a better understanding of the inner workings of Frontier AI models, and a definitive answer to whether or not they have certain abilities, is likely to help manage Frontier risks. Similarly, technical measures to prevent misuse that are harder to circumvent than today’s would help in all worlds.
- Addressing bias – uses of AI are always going to be held back by any bias or unfairness, whether perceived or actually held within models. Measures to identify, minimise and mitigate bias in Frontier AI models are likely to be part of any favourable future.
- AI skills and awareness to support the safe use of AI – all scenarios look more favourable if organisations and companies in the UK are well placed to use the latest advances in AI safely and effectively.
However, for many of the dimensions of our scenarios, what constitutes the most favourable future is contested. For example:
- How openly accessible - are the benefits of open source access in terms of innovation, a wider community to spot issues, and less market concentration worth the risks that come from anyone being able to access and adapt an AI model?
- Pace of change – faster development should mean greater benefits, sooner, in terms of productivity and breakthroughs. But there are plenty of voices in favour of slowing down, to give regulatory mechanisms a chance to keep up.
- Who benefits – a consistent theme of the scenarios is the risk to those (individuals or sectors) who are left behind. How shared the benefits of future AI are between citizens and their creators, how to share them and the role of government in this are all likely to be hotly debated out to 2030.
For these, a more detailed policy conversation that examines the trade-offs will be necessary. Once that has been done, an approach that could help strategy-makers is backcasting. This is a method used to identify the steps that need to be taken to reach a specific preferred future outcome. It could be used by AI policymakers to identify a preferred AI future, connect it to the present and identify what needs to be done, or what would need to happen, to realise it. This could either be done with a single vision of a preferred future scenario, or a set of preferred outcomes in the context of a range of scenarios:
- Preferred scenario: This approach is most appropriate for developing a big-picture strategy that has the potential to shape most or all scenario outcomes. Assuming none of the five scenarios presented in this report is a preferred future end state, this would require policy makers to come up with an entirely new preferred scenario for AI in 2030 (e.g. by using the ‘vision of a positive AI future’ in Chapter 5, or elements of the five scenarios, for inspiration). This should be written down as a short 2030 scenario narrative.
- Preferred outcomes in the context of a range of scenarios: This approach recognises that many factors in the scenarios are global in nature (like AI capability, or market structure) and can only be partly influenced by UK policymakers. It is most appropriate for a developing strategy or set of policies that could shape some, but not all, scenario outcomes. This would require policymakers to identify a set of outcomes that could be achieved in the context of any of the five scenarios by changing the direction of parts of the scenarios that the UK can influence. This should be written down as amended versions of the five 2030 scenario narratives, including the preferred outcomes.
Backcasting: Step-by-step
Once you have decided on your preferred future, the approach is then as follows:
-
Used to:
- identify what needs to change between the present and a given future.
- break out of thinking constrained by how today feels.
- Output: a shared view of a preferred future and the steps required to deliver it.
- Outcome: it could be a plan to achieve a positive future with prioritised steps, or a set of warning signs on the way to a future we’d like to avoid.
-
Approach: workshop discussion that builds on the scenarios
Step 1: Decide on your preferred vision(s) of the future (see above).
Step 2: Identify the steps needed to achieve your preferred future.
Step 3: To do this, build a timeline that moves backwards from the preferred future to the present and identify what needs to happen at each point in the timeline to achieve the desired outcomes (Table 5 provides an example template). Include potential policies and interventions and the activities needed to implement them.
Step 4: Map these policies, interventions and activities onto the timeline and link ones that are related together or are dependent on one another for their implementation. The result should be a series of linked steps, similar to a ‘roadmap’ leading you from the present to your preferred 2030 future.
Step 3: Identify how to deliver the steps that are in your control.
Step 4: Identify how you can influence the steps outside your control.
Step 5: If testing your preferred future in the context of more than one scenario, also identify any policies or interventions that may apply across different scenarios.
Question | Now | Next year | 2025 | 2027 | 2030 |
---|---|---|---|---|---|
What needs to happen at each point in the timeline? Include activity leading to full implementation. | Preferred outcome for in 2030 |
Which steps are within your control? Identify how to deliver these steps. | Are there any policies or interventions that help with other scenarios? | Which steps are outside of your control? Identify how to influence these steps. | |||
---|---|---|---|---|---|
Table 5: Template for Backcasting
Tip: starting from the future and working backwards (rather than starting from today and working towards a specific future) helps get over the human bias for assuming today is permanent.
Policy stress-testing (informing policy options)
Stress-testing is a method for testing policy, strategy, or project objectives against a set of scenarios to see how well they stand up to a range of external conditions. Policy teams can use this to test their ideas to manage Frontier AI – for example a new approach to regulation, research proposals or new organisational structures. Do these ideas work in all versions of the future? If so, they can be considered resilient. Or are they only a good idea in one scenario? If so, how confident are we in making that world happen? What would need to change if the future looked different?
Policy stress-testing: Step-by-step
-
Used to:
-
explore how different scenarios might affect strategic objectives.
-
identify which objectives are robust across the full range of scenarios and which will need to be modified if conditions change in the future.
-
-
Output: Feedback on how a new or existing policy, strategy or project might be affected in different scenarios and how it might need to be modified to ensure resilience across a range of future conditions.
-
Outcome: A more resilient policy, strategy, or project.
-
Approach: Work through the grid below (Table 6), discussing how your policy fares in the longer-term, under the different conditions of each scenario.
-
Take a step back to look across the full grid: What is imperative for us to do in the near-term? What do we need to adapt or track to ensure the effectiveness of this policy across a range of possible futures?
Policy proposal (That we do xxx to achieve YYY) | Scenario 1 | Scenario 2 | Scenario 3 | Scenario 4 | Scenario 5 |
---|---|---|---|---|---|
What aspects of this scenario make delivering this policy easier or more difficult? (Think in terms of enablers and barriers) | |||||
In 2030, is this policy intervention considered a success or a failure? Why? (What might a success narrative look like?) | |||||
Who benefits from this intervention in this scenario? Who doesn’t? Who or what is adversely affected? | |||||
What would we need to start, stop, change, or continue doing now/in the near term for this policy to achieve its objective in this scenario? |
Table 6: Example policy stress-testing exercise
Considering shocks (preparing for the unexpected)
Our scenarios cannot have included all possible AI-relevant events out to 2030. They’ll help colleagues think about a wider range of possible futures. But even using rigorous assumption or uncertainty analysis and scenario development, we are still susceptible to wildcards and shocks. Our organisational and personal biases often prevent us from considering lower likelihood, yet high impact phenomena that can destabilise our context, or accelerate our trajectory towards a given scenario. These events or changes are considered ‘shocks’ to us and to our systems –and manifest in three ways (Table 7).
Considering shocks: Step-by-step
- Used to: Avoid organisational and personal biases against considering lower likelihood, high impact outcomes.
- Output: A clearer idea of future events that might derail a strategy or policy and contingency plans should the event occur.
- Outcome: A more resilient policy, strategy, or project.
- Approach: The ‘Wildcards’ set out in Chapter 3 can be used for this exercise, or new ones could be devised, informed by other evidence in this report or elsewhere. Ask:
-
If this were to happen between now and 2030, would it make each scenario more or less likely? How would it change the conditions within each scenario?
-
What is our capacity to respond to a shock in any given scenario? What would we need to start, stop, adapt, or continue doing to improve our resilience?
Type 1 shock ‘Policies we expect to work, but what if they do not?’ | Type II shock ‘Things we expect to continue, but what if they do not?’ | Type III shock ‘Things we don’t expect to happen, but what if they do?’ |
---|---|---|
E.g. We expect policies around AI systems clearing checks and guardrails agreed by government and leading labs to succeed, but what if they don’t because “general intelligence” can’t be evaluated across all applications? | E.g. We expect big tech companies to continue to be at or close to the Frontier – even if someone gets a temporary edge on them, they’ll catch up quickly, but what if they don’t? | E.g. We don’t expect there to be global harmony around industry safety standards being universally supported, but what if they are? |
Table 7: Examples of the three main shocks
8. Acknowledgements
GO-Science would like to thank everyone who contributed to the work of this project and generously provided their advice and guidance.
This project was led by Jack Snape, Martin Glasspool and Tom Wells. The GO-Science team included Rory Saitch, Mike Askew, Millie Evans, Chris Bullett, Forhad Tanvir, and Samuel Arnold.
We are grateful to the individuals and organisations listed below for their time, effort, and invaluable contributions.
-
GO-Science staff from a variety of teams who gave support throughout different stages of the project, including: Emily Connolly, Jordan Kehoe, Carrie Heitmeyer, Emily White, David Busse, Philippa Green, Nina Grassmann, Nathan Sharpe, and Ted Hayden.
-
Government Departments that contributed to the working group, steering group and scenario workshops: Office for Artificial Intelligence, Department for Science Innovation and Technology, Office for National Statistics, Home Office, Cabinet Office, HM Treasury, Foreign, Commonwealth and Development Office, Joint Intelligence Organisation, Department for Education, Department for Health and Social Care, Innovate UK, Food Standards Agency, National Crime Agency, Government Communications Headquarters, Competition and Markets Authority, Defence Science and Technology Laboratory, National Cyber Security Centre (Assessments), HM Revenue and Customs, Ministry of Defence, and Directorate for Public Governance.
-
External experts who took part in our scenarios workshops and/or who provided advice and guidance at various stages of the project. The views expressed in this report do not necessarily reflect the views of these individual experts, or their organisations: Dr Shahar Avin (Senior Research Associate, Centre for the Study of Existential Risk, University of Cambridge), Dr Haydn Belfield (Research Fellow and Academic Project Manager, University of Cambridge, Leverhulme Centre for the Future of Intelligence and Centre for the Study of Existential Risk), Dr Matt Botvinick (Senior Director of Research, Google DeepMind), Dr Samuel R. Bowman (Associate Professor, New York University and Member of Technical Staff, Anthropic, PBC), Dave Braines (CTO Emerging Technology, IBM Research Europe, UK), Professor Alastair Denniston (University of Birmingham), Dexter Docherty (Foresight Analyst, OECD), Professor Michael Fisher (Royal Academy of Engineering Chair in Emerging Technologies, University of Manchester), Professor Mark Girolami, FREng FRSE (Chief Scientist, The Alan Turing Institute), Dr Demis Hassabis (CEO, Google DeepMind), Professor Sabine Hauert (Professor of Swarm Engineering, University of Bristol), Lewis Ho (Researcher, Google DeepMind), Ardi Janjeva (Research Associate, Centre for Emerging Technology and Security, The Alan Turing Institute), Professor Nick Jennings CB FREng FRS (Vice-Chancellor and President, Loughborough University), Professor Anton Korinek (Professor of Economics, University of Virginia and Darden School of Business; Economics of AI Lead, Centre for the Governance of AI), Dr Shane Legg CBE (Chief AGI Scientist, Google DeepMind), Professor Maria Liakata (Professor in Natural Language Processing, Queen Mary University of London, UKRI/EPSRC Turing AI Fellow), Dr Xiaoxuan Liu (Senior Clinician Scientist in AI and Digital Health, University Hospitals Birmingham NHS Foundation Trust & University of Birmingham), Dr Nicklas Berild Lundblad (Global Policy Director, DeepMind), Dr Trystan B Macdonald (Clinical Research Fellow, Ophthalmology Department, University Hospitals Birmingham NHSFT, Institute of Inflammation and Aging, College of Medical and Dental Sciences, University of Birmingham, Birmingham, UK), Professor Derek McAuley FREng (University of Nottingham), Professor John A McDermid OBE FREng (Director, Assuring Autonomy International Programme, Lloyd’s Register Foundation Chair of Safety, Institute for Safe Autonomy, University of York), Jessica Montgomery (Director, AI@Cam, University of Cambridge), Professor Marion Oswald MBE (Professor of Law, Northumbria University; Senior Research Associate, The Alan Turing Institute), Dr Robert Elliott Smith (Director of AI and Data Science, Digital Catapult), Dr Charlotte Stix (External Policy Advisor, Apollo Research), Professor Shannon Vallor (Baillie Gifford Chair in the Ethics of Data and Artificial Intelligence, University of Edinburgh; co-Director, Bridging Responsible AI Divides), Don Wallace (Policy Development & Strategy, Google DeepMind), Professor Michael Wooldridge (Director of Foundational AI, Alan Turing Institute, and Professor in the Department of Computer Science at the University of Oxford).
-
Thinks and CDEI for their support and the delivery of the public engagement and the 32 members of the public that took the time to contribute to this project.
We appreciate the immense pool of expertise we were able to access during this project and hope that the final report will be useful to these contributors and an even wider group.
9. Glossary
Term | Description |
---|---|
Actors | Individuals and organisations – government, businesses, citizens, for example – that are active in the policy or strategy area. |
Agency | Ability to autonomously perform multiple sequential steps to try and complete a high-level task or goal. |
Application Programming Interface | Application refers to any software with a distinct function. Interface can be thought of as a contract of service between 2 applications. This contract defines how the 2 communicate with each other using requests and responses. |
Artificial General Intelligence Also: General AI, Strong AI, Broad AI | Artificial general intelligence (AGI) describes a machine-driven capability to achieve human-level or higher performance across most cognitive tasks. |
Artificial Intelligence | Machine-driven capability to achieve a goal by performing cognitive tasks. |
Autonomy | The ability to operate without direct human oversight. |
Axes of Uncertainty | The critical uncertainties for the policy or strategy area in the future that are used to frame scenarios. The axes should capture the most important uncertainties but also be independent of each other. |
Backcasting | A way of connecting a given future to the present and overcoming “present bias” in the way that we plan. It is a tool for determining the steps that need to be taken to deliver a preferred future. |
Capability | The ability to achieve a goal. The extent of a system’s ability to achieve a range of goals. |
Cognitive Tasks | A range of tasks involving a combination of information processing, memory, information recall, planning, reasoning, organisation, problem solving, learning, and goal-orientated decision-making. |
Compute | Computational processing power, including CPUs, GPUs, and other hardware, used to run AI models and algorithms. |
Critical Uncertainty | Factors that are important to the future of AI development but highly uncertain. |
‘Day in the life of’ | A ‘day in the life of’ narrative (sometimes shortened to DILO) is a used to illustrate how the conditions in a given scenario might shape the life of an individual stakeholder or a range of different stakeholders. DILOs can be used alongside scenario narratives to add detail and interest or they can form the central narrative itself. |
Disinformation | Deliberately false information spread with the intent to deceive or mislead. |
Foundation Models | Machine learning models trained on very large amounts of data that can be adapted to a wide range of tasks. |
Frontier AI | AI models that can perform a wide variety of tasks and match or exceed the capabilities present in today’s most advanced models. This is the government’s chosen definition. |
Futures | An approach or way of thinking about the possible, probable, and preferable futures and the underlying structures that could give rise to particular future characteristics, events, and behaviour. |
Generative AI | AI systems that can create new content. |
Impact Mapping | A graphic strategy planning method to decide which features to build into a product. |
LLMs | Machine learning models trained on large datasets that can recognise, understand, and generate text and other content. |
Machine Learning | An approach to developing AI where models learn patterns from data and how to perform tasks without being explicitly programmed. |
Misinformation | Incorrect or misleading information spread without harmful intent. |
Morphological Analysis | The process of examining possible resolutions to unquantifiable, complex problems involving many factors. |
Narrow Artificial Intelligence | AI systems able to perform a single or limited range of tasks. |
PESTLE | A generic term for the drivers shaping the future policy environment. PESTLE is an acronym which stands for Political, Economic, Societal, Technological, Legislative and Environmental drivers. There are a number of common variants which describe the same drivers or a subset of them – PEST, STEP, STEEP, STEEPL – and some (PESTLEV, for example, where the V stand for Values) which introduce additional drivers. |
Policy Stress-Testing | A method for testing strategic objectives against a set of scenarios to see how well they stand up against a range of external conditions. Sometimes called ‘Wind-tunnelling’. |
Reinforcement learning | A subset of machine learning that allows an AI-driven system (sometimes referred to as an agent) to learn through trial and error using feedback from its actions. |
Scenario | Compelling stories about a range of different possible futures. They describe future worlds so that people can understand what it feels like to live there and what this will mean for them. |
Stakeholder | Any group or individual who has an interest in or an influence on the policy or strategy area. |
10. References
DSIT, (2023)Future risks of frontier AI Department for Science, Innovation and Technology.
DSIT (2023) AI Safety Summit: introduction Department for Science, Innovation & Technology.
Open AI (2022) Introducing ChatGPT Open AI
Buchholz, K., (2023) Threads Shoots Past One Million User Mark at Lightning Speed
Duarte, F., (2023) Number of ChatGPT Users (2023) Exploding Topics
Hsiao, S., (2023) What’s ahead for Bard: More global, more visual, more integrated Google
Meta (2023) Introducing New AI Experiences Across Our Family of Apps and Devices Meta
Open AI (2023) GPT-4V(ision) system card Open AI
Senior, A.W., Evans, R., Jumper, J. et al. (2020) Improved protein structure prediction using potentials from deep learning Nature.
Koh, D. M., Papanikolaou, N., Bick, U. et al (2022) Artificial intelligence and machine learning in cancer imaging Communications Medicine.
Hunter, B., Chen, M., Ratnakumar, P., Alemu, E., Logan, A., Linton-Reid, K. et al (2022) A radiomics-based decision support tool improves lung cancer diagnosis in combination with the Herder score in large lung nodules EBioMedicine.
Bender, E. et al (2021) On the Dangers of Stochastic Parrots: Can Language Models Be Too Big? Conference on Fairness, Accountability, and Transparency (FAccT ’21), March 3–10, 2021, Virtual Event, Canada.
HMG (2023) Safety and Security Risks of Generative Artificial Intelligence to 2025.
Janjeva, A. et al (2023) Strengthening Resilience to AI Risk: A guide for UK policymakers Centre for Emerging Technology and Security, The Alan Turing Institute.
Tobin, J. (2023) Artificial intelligence: Development, risks and regulation House of Lords Library.
Shabsigh, G. & Boukherouaa, E. B. (2023) Generative Artificial Intelligence in Finance: Risk Considerations International Monetary Fund.
Hoffmann, M. & Frase, H. (2023) Adding Structure to AI Harm: An Introduction to CSET’s AI Harm Framework Center for Security and Emerging Technology.
OWASP (2023) OWASP Top 10 for LLM Applications The Open Web Application Security Project (OWASP)
Littman, M. L. et al (2021) Gathering Strength, Gathering Storms: The One Hundred Year Study on Artificial Intelligence (AI100) 2021 Study Panel Report Stanford University.
OECD (2023) Advancing accountability in AI: Governing and managing risks throughout the lifecycle for trustworthy AI OECD.
Future of Life Institute (2023) Pause Giant AI Experiments: An Open Letter Future of Life Institute
Centre for AI Safety. Statement on AI Risk Centre for AI Safety. [Accessed October 2023].
Zakaria, F. (2023) On GPS: AI ‘godfather’ warns of threat to humanity CNN.
Mitchell, M. (2023) Do half of AI researchers believe that there’s a 10% chance AI will kill us all?
SMC (2023) Expert reaction to a statement on the existential threat of AI published on the Centre for AI Safety website Science Media Centre.
GO-Science (2018) Futures toolkit for policymakers and analysts Government Office for Science.
Ridley, T (2011) General Morphological Analysis (GMA) Link Springer.com
Kaplan, J., McCandlish, S., Henighan, T., Brown, T., Chess, B., Child, R., Gray, S., Radford, A., Wu, J., Amodei, D., (2020) Scaling Laws for Neural Language Models arXiv:2001.08361
Seger, E., Dreksler, N., Moulange, R., Dardaman, E., Schuett, J., Wei, K., Winter, C., Arnold, M., Ó hÉigeartaigh, S., Korinek, A. and Anderljung, M., (2023) Open-Sourcing Highly Capable Foundation Models: An Evaluation of Risks, Benefits, and Alternative Methods for Pursuing Open-Source Objectives. SSRN
Spitale, G., Biller-Andorno, N., Germani, F., (2023) AI model GPT-3 (dis) informs us better than humans Science Advances
Dell’Acqua, F., McFowland, E., Mollick, E., Lifshitz-Assaf, H., Kellogg, K., Rajendran, S., Krayer, L., Candelon, F., Lakhani, K., (2023) Navigating the Jagged Technological Frontier: Field Experimental Evidence of he Effects of AI on Knowledge Worker Productivity and Quality. Harvard Business School Technology and Operations Management Unit
Maslej, N., Fattorini, L., Brynjolfsson, E., Etchemendy, J., Ligett, K., Lyons, T., Manyika, J., Ngo, H., Niebles, J. C., Parli, V., Shoham, Y., Wald, R., Clark, J., and Perrault, R., (2023) The AI Index 2023 Annual Report.
Valmeekam, K., Sreedharan, S., Marquez, M., Olmo, A., and Kambhampati, S., (2023) On the planning abilities of LLMs (a critical investigation with a proposed benchmark)
Kojima, T., Gu, S. S., Reid, M., Matsuo, Y., and Iwasawa, Y., (2022) LLMs are zero-shot reasoners.
Giattino, C., Mathieu, E., Samborska, V., Broden, J., and Roser, M., (2022) Artificial Intelligence.
Shlegeris, B., Roger, F., Chan, L., & McLean, E. (2022) Language models are better than humans at next-token prediction arXiv preprint arXiv:2212.11281.
Ganguli, D., Hernandez, D., Lovitt, L., Askell, A., Bai, Y., Chen, A., Conerly, T., Dassarma, N., Drain, D., Elhage, N., and El Showk, S., (2022) Predictability and Surprise in Large Generative Models.
Epoch, (2023), Parameters in notable artificial intelligence systems Our World in Data.
Wei, J., Tay, Y., Bommasani, R., Raffel, C., Zoph, B., Bourgeaud, S., Yogatama, D., Bodma, M., Zhou, D., Metzler, E., Chi, E., Hashimoto, T., Vinyals, O., Liang, P., Dean, J., Fedus, W., (2022) Emergent Abilities of LLMs. Cornell University
Lu, S. et al (2023) Are Emergent Abilities in LLMs just In-Context Learning?
Berglund, L. et al (2023) The Reversal Curse: LLMs trained on “A is B” fail to learn “B is A” arXiv.
Bubeck, S., Chandrasekaran, V., Eldan, R., Gehrke, J., Horvitz, E., Kamar, E., Lee, P., Lee, Y.T., Li, Y., Lundberg, S., Nori, H., Palangi, H., Ribeiro, M. T., and Zhang, Y., (2023) Sparks of Artificial General Intelligence: Early experiments with GPT-4.
Knight, W., (2023) Some Glimpse AGI in ChatGPT. Others Call It a Mirage Wired.
Jumper, J., Evans, R., Pritzel, A. et al. (2021) Highly accurate protein structure prediction with AlphaFold Nature
Kirillov, A. et al (2023) Segment Anything arXiv.
Awais, M. et al (2023) Foundational Models Defining a New Era in Vision: A Survey and Outlook arXiv.
InfoQ (2023) Meta open-sources computer vision foundation model DINOv2 InfoQ.
Lanchantin, J. et al (2023) A Data Source for Reasoning Embodied Agents Hugging Face.
Ajay, A. et al (2023) Compositional Foundation Models for Hierarchical Planning Hugging Face.
Fu, D. et al (2023) From Deep to Long Learning?
Liu, N. et al (2023) Lost in the Middle: How Language Models Use Long Contexts arXiv.
Pinecone (2023) Less is More: Why use Instead of Larger Context Windows Pinecone.
Herique Martine, P. et al (2022) ∞-former: Infinite Memory Transformer arXiv.
Zhong, W. et al (2023) MemoryBank: Enhancing LLMs with Long-Term Memory arXiv.
Yuan, Y. et al (2023) Retrieval-Augmented Text-to-Audio Generation arXiv.
Chebotar, Y., and Yu, T., (2023) RT-2: New model translates vision and language into action.
Jayesh, (2023) How Autonomous Agents Work: The Concept and Its LangChain Implementation.
Briggs, J., Ingham, F., (2023) LangChain: Introduction and Getting Started. PineCone
AutoGPTAUTOGPT AutoGPT
Yang, H., Yue, S. and He, Y., (2023) Auto-GPT for Online Decision Making: Benchmarks and Additional Opinions.
Lablab (2023) What is AutoGPT and how can I benefit from it?
Xiao, H (2023) Auto-GPT Unmasked: The Hype and Hard Truths of Its Production Pitfalls Jina.
The Alan Turing Institute, (2023) Multi Agent Systems The Alan Turing Institute
Wang, L., Ma, C., Feng, X., Zhang, Z., Yang, H., Zhang, J., Chen, Z., Tang, J., Chen, X., Lin, Y., Zhao, W., Wei, Z., Wen, J., (2023) A Survey on LLM based Autonomous Agents arXiv:2308.11432
Zhou, W., Jiang, Y., Li, L., Wu, J., Wang, T., Qiu, S., Zhang, J., Chen, J., Wu, R., Wang, S., Zhu, S., Chen, J., Zhang, W., Zhang, N., Chen, H., Cui, P., Sachan, M., (2023) Agents: An Open-source Framework for Autonomous Language Agents. arXiv:2309.07870
The RoboCat team, (2023) RoboCat: A self-improving robotic agent
Bousmalis, K., Vezzani, G., Rao, D., Devin, C., Lee, A.X., Bauza, M., Davchev, T., Zhou, Y., Gupta, A., Raju, A., et al., (2023) RoboCat: A Self-Improving Foundation Agent for Robotic Manipulation.
LessWrong [Accessed 16 August 2023]. Recursive Self-Improvement.
Creighton, J., (2019) The Unavoidable Problem of Self-Improvement in AI: An Interview with Ramana Kumar, Part 1.
Li, Y., Choi, D., Chung, J., Kushman, N., Schrittwieser, J., Leblond, R., Eccles, T., Keeling, J., Gimeno, F., Dal Lago, A. and Hubert, T., (2022) Competition-level code generation with alphacode.
Vaswani, A., Shazeer, N., Parmar, N., Uszkoreit, J., Jones, L., Gomez, A.N., Kaiser, Ł. and Polosukhin, I., (2017) Attention is all you need
DSIT, (2023) Future risks of frontier AI Department for Science, Innovation and Technology.
Krizhevsky, A., Sutskever, I., and Hinton, G. E. (2012) Imagenet classification with deep convolutional neural networks
Harvie, L., (2023) The Intersection of Machine Learning and Embedded Systems: A Comprehensive Overview Medium
Savage, N., (2019) How AI and neuroscience drive each other forwards Nature
Hassabis, D., Kumaran, D., Summerfield, C., & Botvinick, M. (2017) Neuroscience-Inspired Artificial Intelligence Neuron.
The Economist (2023)The race of the AI labs heats up The Economist.
CBInsights (2021) The Race for AI: Which Tech Giants Are Snapping Up Artificial Intelligence Startups CBInsights
Perspective Economics (2023) Artificial Intelligence Sector Study Perspective Economics
CMA (2023) AI Foundation Models: Initial report Competition and Markets Authority.
ONS, (2023) Understanding AI uptake and sentiment among people and businesses in the UK: June 2023 Office for National Statistics.
Hu, K., (2023) ChatGPT sets record for fastest-growing user base Reuters.
Carr, D., (2023) ChatGPT Starts to Bounce Back in US as School Year Resumes Similarweb
Tong, A., (2023) Exclusive: ChatGPT traffic slips again for third month in a row Reuters
Patel, D., and Ahmad, A. (2023) Google “We Have No Moat, And Neither Does OpenAI” SemiAnalysis.
Meta (2023) Meta and Microsoft Introduce the Next Generation of Llama
Schmid, P., Sanseviero, O., Cuenca, P., von Werra, L. and Launay, J. (2023) Spread Your Wings: Falcon 180B is here Hugging Face.
R, M. (2023) Thinking about the security of AI systems National Cyber Security Centre.
International Data Corporation (2023) Worldwide Spending on AI-Centric Systems Forecast to Reach $154 Billion in 2023 International Data Corporation
Tricot, R. (2021) Venture capital investments in artificial intelligence: Analysing trends in VC in AI companies from 2012 through 2020 OECD Digital Economy Papers.
DSIT, (2023) National semiconductor strategy Department for Science, Innovation and Technology
TSI Semiconductor Rapid Technology Assessment, TSI Semiconductor Science Power Index.
Waters, R., (2023) Falling costs of AI may leave its power in hand of a small group The Financial Times
Edwards, B., (2023) Nvidia’s new monster CPU+GPU chip may power the next gen of AI chatbots arsTechnica
Sparkes, M., (2023) Chip shortages are producing winners and losers in the AI gold rush NewScientist
The Economist (2023) Taiwan’s dominance of the chip industry makes it more important The Economist.
OpenAI (2018) AI and Compute OpenAI.
Epoch, (2023), Computation used to train notable artificial intelligence Our World in Data.
Will Knight (2023) OpenAI’s CEO Says the Age of Giant AI Models Is Already Over.
Karl Rupp, (2022), Moore’s law: The number of transistors per microprocessor Our World in Data.
Bloom, N., Charles I. J., Van Reenen, J., Webb, M., (2022) Are Ideas Getting Harder to Find?
Bessen, J., Impink, S., Reichensperger, L., Seamans, R., (2022) The role of data for AI startup growth Research Policy, Volume 51, Issue 5, 104513
Villalobos, P., Sevilla, J., Heim, L., Besiroglu, T., Hobbhahn, M., and Ho, A., (2022) Will We Run out of Data? An Analysis of the Limits of Scaling Datasets in Machine Learning arXiv.
Heikkilä, M., (2022) How AI-generated text is poisoning the internet
Shumailov, I., Shumaylov, Z., Zhao, Y., Gal, Y., Papernot, N., Anderson, R., (2023) The Curse of Recursion: Training on Generated Data Makes Models Forget arXiv:2305.17493v2
Franzen, C., (2023) The AI feedback loop: Researchers warn of ‘model collapse’ as AI trains on AI-generated content VentureBeat
Murgia, M., (2023) Why computer-made data is being used to train AI models Financial Times
Walsh, D., (2023) The legal issues presented by generative AI MIT Management
Herd, S., (2023) Capabilites and alignment of LLM cognitive architectures LESSWRONG.
Seth Lazar and Alondra Nelson (2023) AI Safety on whose terms? Science
Weidinger, L., Rauh, M., Marchal, N., Manzini, A., Hendricks, L.A., Mateos-Garcia, J., Bergman, S., Kay, J., Griffin, C., Bariach, B. and Gabriel, I. (2023) Sociotechnical Safety Evaluation of Generative AI Systems arXiv:2310.11986
OpenAI (2023) OpenAI’s Approach to Frontier Risk OpenAI.
OpenAI (2023) Our approach to AI safety OpenAI.
Center for Countering Digital Hate. (2023) Misinformation on Bard, Google’s new AI chat CCDH.
OpenAI (2023) GPT-4 System Card OpenAI.
Hadfield-Menell, D., Dragan, A., Abbeel, P., and Russell, S. (2017) The Off-Switch Game arXiv:1611.08219v3
Orseau, L. & Armstrong, S. (2016) Safely Interruptible Agents Google DeepMind.
Oswald, M., (2018) Algorithm-assisted decision-making in the public sector: framing the issues using administrative law rules governing discretionary power.
Bai, Y., Kadavath, S., Kundu, S., Askell, A., Kernion, J., Jones, A., Chen, A., Goldi, A., Mirhoseini, A., McKinnon, C., Chen, C., Olsson, C., Olah, C., Hernandez, D., Drain, D., Ganguli, D., Li, D., Tran-Johnson, E., Perez, E., Kerr, J., Mueller, J., Ladish, J., Landau, J., Ndousse. K., Lukosuite K., Lovitt, L., Sellitto, M., Elhage, N., Schiefer, N., Mercado, N., DasSarma, N., Lasenby, R., Larson, R., Ringer, S., Johnston, S., Kravec, S., El Showk, S., Fort, S., Lanham, T., Telleen-Lawson, T., Conerly, T., Henigham, T., Hume, T., Bowman, S., Hatfield-Dodds, Z., Mann, B., Amodei, D., Jospeh, N., McCandish, S., Brown, T., Kaplan, J., (2022) Constitutional AI: Harmlessness from AI Feedback arXiv:2212.08073
Azeem Azhar and Nathan Warren (2023) Partisan machines; fusion feats; decoding AI; heavy cars Exponential view.
Jeffrey Dastin (2018) Amazon scraps secret AI recruiting tool that showed bias against women Reuters
Lowe, R. and Leike, J. (2022) Aligning language models to follow instructions.
Salahuddin, Z., Woodruff, H., Chatterjee, A., Lambin, P., (2022) https://www.sciencedirect.com/science/article/pii/S0010482521009057Transparency of deep neural networks for medical image analysis: A review of interpretability methods Computers in Biology and Medicine, Volume 140, 105111
Nwadiugwu, M.C., (2020) Neural networks, artificial intelligence and the computational brain
Zhang, B.T., (2015) Bio-Inspired Human-Level Machine Learning Seoul National University.
Zhao, H., Chen, H., Yang, F., Liu, N., Deng, H., Cai, H., Wang S., Yin, D., Du, M., (2023) Explainability for Large Language Models: A Survey arXiv:2309.01029v2
Vilone, G., (2021) Notions of explainability and evaluation approaches for explainable artificial intelligence Information Fusion, Volume 76, pages 86-106
Bureau of Arms Control, Verification and Compliance (2023) Political Declaration on Responsible Military Use of Artificial Intelligence and Autonomy US Department of State.
MOD (2022) Defence Artificial Intelligence Strategy Ministry of Defence.
Blanchard, A., Hall, J., (2023) Terrorism and Autonomous Weapon Systems: Future Threat or Science Fiction CETaS Expert Analysis
Conboy, C. (2021) Opposition to killer robots remains strong — poll Stop Killer Robots.
Noor, O. (2022) 70 states deliver joint statement on autonomous weapons systems at UN General Assembly Stop Killer Robots.
Brooks, R., Artificial intelligence and its impact on everyday life University of York
Mordor intelligence (2023) AI in social media market size & share analysis - growth trends & forecasts
Elliott, A., (2019) The culture of AI: Everyday life and the digital revolution Routledge.
Edelman, (2019) 2019 EDELMAN AI SURVEY Edelman.
ONS, (2023) Public awareness, opinions and expectations about artificial intelligence: July to October 2023 Office for National Statistics
Hancock, J.T., Naaman, M. and Levy, K., (2020) AI-mediated communication: Definition, research agenda, and ethical considerations
Varnum, M., (2023) Large Language Models May Radically Change Psychology Psychology today
Park, J., O’Brien, J., Cai, C., Morris, M., Liang, P., Bernstein, M., (2023) Generative Agents: Interactive Simulacra of Human Behavior arXiv:2304.03442.
Deshpande, A., (2023) Anthropomorphization of AI: Opportunities and Risks Montreal AI Ethics Institute.
Ofcom, (2022) A review of Ofcom’s research on digital exclusion among adults in the UK. Ofcom
British Academy (2022) Understanding digital poverty in the UK
DSIT, DCMS, (2021) Quantifying the UK Data Skills Gap
Slane, R., (2022) Demand for AI Skills Triples in the UK Labour Market Lightcast
DCMS, OAI, The Rt Hon Chris Philp MP (2023) £23 million to boost skills and diversity in AI jobs [https://www.gov.uk/government/organisations/department-for-digital-culture-media-sport br /> Department for Digital, Culture, Media & Sport</a>, [https://www.gov.uk/government/organisations/office-for-artificial-intelligence ffice for Artificial Intelligence and [https://www.gov.uk/government/people/chris-philp-mp he Rt Hon Chris Philp MP</a>
World Economic Forum, (2023) Future of Jobs Report World Economic Forum.
Jurowetzki, R., Hain, D., Mateos-Garcia, J., Stathoupoulos, K., (2021) The Privatization of AI Research(-ers) : Causes and Potential Consequences – From university-industry interaction to public research brain-drain? arXiv:2102.01648
Harriss, L., Fearns, J., Lally, C., (2023) Data science skills in the UK workforce UK Parliament
IBM (2022) IBM Global AI Adoption Index 2022 Watson
Sweney, M., (2023) BT to axe up to 55,000 jobs by 2030 as it pushes into AI The Guardian
Ford, B., (2023) IBM to Pause Hiring for Jobs That AI Could Do Bloomberg
OECD, (2023) Employment Outlook 2023
Kochhar, R., (2023) Which U.S. Workers Are More Exposed to AI on Their Jobs? Pew Research Center
Piizzinelli, C., Panton, A., Mendes Tavares, M., Cazzaniga, M., Li, L., (2023) Labour Market Exposure to AI: Cross-country Differences and Distributional Implications International Monetary Fund
Stewart, I., De, D., Cole, A., (2015) Technology and people: The great job-creating machine Deloitte
Hötte, K., Somers, M., Theoforakopoulos, A., (2022) Technology and jobs: A systematic literature review
Jain, S., Ilzetzki, E., (2023) The impact of artificial intelligence on growth and employment.
DSIT, BEIS, (2021) The potential impact of AI on UK employment and the demand for skills Department for Science, Innovation and Technology and the Department for Business, Energy and Industrial Strategy
Hawksworth, J., Berriman, R., Goel, S., (2018) Will robots really steal our jobs? PricewaterhouseCoopers
Tabrizi, B., Pahlavan, B., (2023) Companies That Replace People with AI Will Get Left Behind Harvard Business Review
ONS, (2023) Business insights and impact on the UK economy Office for National Statistics
Spiroe, H., (2020) Developing a deeper understanding of public attitudes towards data-driven technologies Centre for Data Ethics and Innovation
CO, CDDO, OAI, (2021) Ethics, Transparency and Accountability Framework for Automated Decision - Making Cabinet Office, Central Digital & Data Office, Office for Artificial Intelligence
Stilgoe, J., Owen, R. and Macnaghten, P., (2013) Developing a framework for responsible innovation
Zaremba, W., Dhar, A., Ahmad, L., Eloundou T., Santurkar, S., Argawal, S., Leung, J., (2023) Democratic inputs to AI OpenAI
Modhvadia, R., (2023) How do people feel about AI?
Burke, D., (2004) GM food and crops: what went wrong in the UK? Embo Reports: 5(5) : 432 - 436
Dupont, J., Wride, S., Ali, V., (2023) What does the public think about AI? Public First
The New York Times, (2023) The people onscreen are fake. The disinformation is real.
Alba, D., (2023) How a fake AI photo of a Pentagon blast went viral and briefly spooked stocks Los Angeles Times
Honigberg, B., (2022) The Existential Threat of AI-Enhanced Disinformation Operations Just Security
NCSC, (2023) NCSC Annual Review 2023 National Cyber Security Centre.
Gutnik, D., Evseev, P., Miroshnikov, K., Shneider, M., (2023) Using Alphafold Predictions in Viral Research
Curr Issues Mol Biol, Curr Issues Mol Biol.
Sohn, R., (2022) AI Drug Discovery Systems Might Be Repurposed to Make Chemical Weapons, Researchers Warn Scientific American
GCHQ, (2021) Pioneering a New National Security GCHQ
UN. Secretary General, (2022) Countering disinformation for the promotion and protection of human rights and fundamental freedoms : report of the Secretary-Genera United Nations
West, D., (2023) How AI will transform the 2024 elections
UNESCO (2021) Ethics of Artificial Intelligence UNESCO.
GPAI, (2023) The Global Partnership on Artificial Intelligence GPAI.
G7 Hiroshima Summit (2023) G7 Hiroshima Leaders’ Communiqué G7.
Tambiana Madiega (2023) Briefing: Artificial intelligence act European Parliament.
DSIT, (2023) Policy Paper, Chair’s Summary of the AI Safety Summit 2023, Bletchley Park. Department for Science, Innovation and Technology.
UNFCCC (2015) Paris Agreement UNFCCC.
BBC (2021) US rejoins Paris accord: Biden’s first act sets tone for ambitious approach.
UNFCCC (2022) List of participants. Part one. Parties and observer States UNFCCC.
NATO Parliamentary Assembly [Accessed 16 August 2023]. Finland and Sweden Accession NATO.
NATO (2023) Relations with partners in the Indo-Pacific region NATO.
CrisisWatch, (2023) CrisisWatch International Crisis Group.
Wood, N., (2022) Autonomous weapons systems and force short of war
Johnson, J., (2019) Artificial intelligence & future warfare: implications for international security
POST., (2022) Automation in Military Operations POSTNOTE, UK Parliament.
Varas, A., Varadarajan, R., Goodrich, J., Yinug, F., (2021) Strengthening the global semiconductor supply chain in an uncertain era Semiconductor Industry Assosication
Mark, J., Roberts, D., (2023) United Satates - China semiconductor standoff: A supply chain under stress Atlantic Council
DSIT, Chloe Smith MP, (2023) New £1billion strategy for UK’s semiconductor sector Department for Science, Innovation and Technology, and Chloe Smith MP
The White House, (2023) One Year after the CHIPS and Science Act, Biden-Harris Administration Marks Historic Progress in Bringing Semiconductor Supply Chains Home, Supporting Innovation, and Protecting National Security The White House
PwC and BEIS, (2021) The potential Impact of Artificial Intelligence on UK Employment and the Demand for Skills Department for Business, Energy and Industrial Strategy
The Pissarides Review, (2023) What drives UK firms to adopt AI and robotics, and what are the consequences for jobs? Institute for the future of Work
DSIT, (2023) Future risks of frontier AI Department for Science, Innovation and Technology.
Erber, G., Fritsche, U., Harms, P., (2017) The Global Productivity Slowdown: Diagnosis, Causes and Remedies Intereconomics, Volume 52. Number 1. pp.25-50
Remes, J., Manyika, J., Bughin, J., Woetzel, J., Mischke, J., Krishnan, M., (2018) Solving the headache of productivity Mckinsey Global Institute
Noy, S., Zhang W., (2023) Experimental Evidence on the Productivity Effects of Generative Artificial Intelligence MIT Economics
Kalliamvakou, E., (2022) Research: quantifying GitHub Copilot’s impact on developer productivity and happiness
Brynjolfsson, E., Li, D., Raymond, L., (2023) Generative AI At Work. National Bureau of Economic Research
Chui, M., Roberts, R., Yee, L., Hazan, E., Singla, A., Smaje, K., Sukharevsky, A., Zemmel, R., (2023) The economic potential of generative AI: The next productivity frontier McKinsey Digital
The Alan Turing Institute (2022) Session 3, Public Policy Stage - Machine learning in public services The Alan Turing Institute
CDDO, OAI, DSIT, DCMS, BEIS, (2020) A guide to using artificial intelligence in the public sector Central Digital and Data Office, Office for Artificial Intelligence, Department for Science, Innovation and Technology, Department for Digital, Culture, Media & Sport, Department for Business, Energy and Industrial Strategy
Tyler, C., Akerlof, K., Allegra, A., Arnold, Z., Canino, H., Doorenbal, M., Goldstein, J., Pedersen, D., Sutherland, W., (2023) AI tools as science policy advisers? The potential and the pitfalls Nature
Callaway, E., (2022) What’s next for AlphaFold and the AI protein-folding revolution Nature
Arnold, C., (2023) Inside the nascent industry of AI-designed drugs Nature Medicine
Agnesina, A., Rajvanshi, P., Yang, T., Pradipta, G., Jiao, A., Keller, B., Khailany, B., Ren, H., (2023) AutoDMP: Automate DREAMPlace-based Macro Placement Association for Computing Machinery
The Economist, (2023) How Scientists are using artificial intelligence The Economist
Degot, C., Duranton, S., Frédeau, M., Hutchinson, R., (2021) Reduce Carbon and Costs with the Power of AI Boston Consulting Group
Liu, Y., Esan, O., Pan, Z., An, L., (2021) Machine learning for advanced energy materials Energy and AI, Volume 3, 100049
Weinstein, D., (2023) Unlocking the AI-powered opportunity in the UK
Anderson, J., Kalra, N., Stanley, K., Sorensen, P., Samaras, C., Olutwatola, T., (2016) Autonomous Vehicle Technology RAND Corporation
Whittlestone, J., Clark, J., (2021) Why and How Governments Should Monitor AI Development arXiv:2108.12427
Ho, L., Barnhart, J., Trager, R., Bengio, Y., Brundage, M., Carnegie, A., Chowdhury, R., Dafoe, A., Hadfield, G., Levi, M., Snidal, D., (2023) International Institutions for Advanced AI arXiv:2307.04699
GO-Science expert engagement.
Bilcke, J., (2023) Deploying the AI Comic Factory using the Inference API Hugging Face.
GO-Science (2023) Net Zero Society: scenarios and pathways Government Office for Science.