Public attitudes to data and AI: Tracker survey (Wave 4) report
Published 16 December 2024
1. Foreword
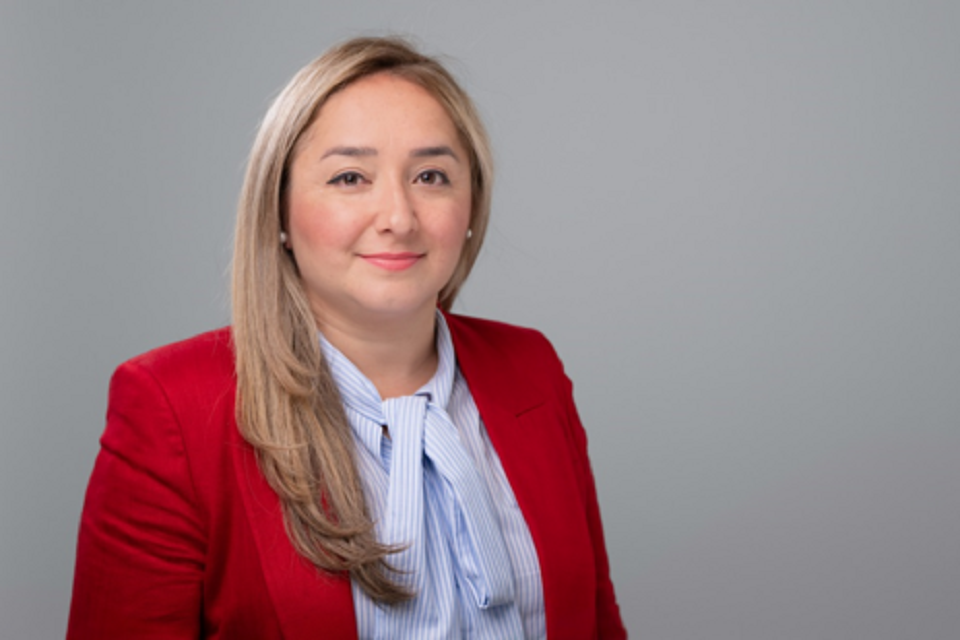
Minister for AI and Digital Government Feryal Clark
Data and AI hold unparalleled potential to drive transformative change for communities across the country. By leveraging these technologies, we can not only boost economic growth but also revolutionise public services — delivering life-saving advancements in healthcare and fostering world-class education in our classrooms. That’s why data-driven innovation lies at the heart of the Department for Science, Innovation and Technology’s (DSIT) ambitious vision to accelerate economic growth, elevate public services, and improve living standards for everyone.
The newly proposed Data Bill marks a pivotal step toward turning this vision into reality. Yet, realising the full economic and societal benefits of data and AI depends on one essential factor: public trust. We must ensure these technologies are designed and implemented in ways that resonate with public expectations while addressing their valid concerns.
To this end, DSIT is building a revitalised Digital Centre of Government, underpinned by cutting-edge research, to create trustworthy digital tools that reflect UK values and work for everyone. Our approach to digital identity, for example, is rooted in extensive public dialogue and collaboration with industry stakeholders to earn and sustain public confidence.
Further advancing this goal, the Responsible Technology Adoption Unit (RTA) conducts an annual tracker survey that provides invaluable insights into public attitudes toward data and AI. Now in its fourth year, this survey has mapped evolving public perceptions, aspirations, and concerns since 2021. The latest findings offer fresh perspectives on the use of data-sharing and AI in public services, ensuring that new initiatives—such as the National Data Library—align with public values and earn trust.
Public opinion on data and AI is complex and context-dependent. To ensure an inclusive understanding, this year’s survey captured the perspectives of over 5,000 people, with additional voices from Northern Ireland, Scotland, and Wales. This makes it the first survey of its kind in the world, offering a comprehensive view of public sentiment across the UK.
These insights will continue to shape the government’s approach to data and AI, centering public voices as we navigate the immense opportunities these technologies offer. We hope this latest survey, like its predecessors, will serve as a cornerstone for building trust and empowering society to harness the transformative potential of data and AI.
Minister for AI and Digital Government Feryal Clark
2. Executive summary
1. Despite growing public trust and improved optimism about data practices, concerns about data use persist.
Increasingly, the public agrees that data use is beneficial to society and recognises the useful role data can play in designing products and services that benefit them. However, while public attitudes regarding the value and transparency of data use are becoming more positive, concerns around accountability persist. Anxieties are primarily rooted in data security, unauthorised sales of data, surveillance, and a lack of control over data sharing. These concerns are particularly prevalent among older individuals. Notably, these issues mirror the themes participants recalled hearing about in news stories.
2. When it comes to data sharing, public preferences are driven by overall trust in the organisations involved.
Public preferences for data sharing are primarily influenced by the organisations that are involved, and participants place less importance on how the data is used or the safeguards that are in place. Notably, digitally disengaged people show lower levels of trust than the overall population across all data actors. However, across all groups, the NHS and academic researchers consistently rank high in trust, whereas social media companies and the Government generally receive lower levels of trust. Reflecting this, data sharing scenarios involving NHS services are favoured, while those involving big technology companies are less preferred.
3. Despite near universal awareness of AI, public perceptions are dominated by concerns.
Awareness of artificial intelligence (AI) is nearly universal among the online population. A majority of adults are now able to explain the term, at least to some extent. However, deeper understanding of AI varies significantly, with younger people, those of higher socioeconomic status, and London residents reporting higher levels of familiarity. Despite improved awareness, the public’s associations with AI remain dominated by negative concepts, reflecting fears and concerns, though younger individuals tend to have more positive perceptions.
4. The public increasingly uses AI chatbots for both personal and work-related matters, but there is a knowledge gap regarding how AI systems are trained.
AI chatbots are increasingly used by UK adults for both personal and work-related tasks. Six in ten members of the public report having used chatbots in the past three months, with over four in ten of these using them at least once a month. Use of AI for visual content creation is less common, with about a quarter of the public using it at least once a month for both professional and personal purposes. Despite their growing popularity, there is a knowledge gap about how AI systems are trained, with seven in ten members of the public reporting they know only a little or nothing about this topic.
5. UK adults have mixed perceptions on AI’s societal and personal impact, highlighting the dual potential of AI to provide benefits and generate worries.
UK adults have mixed perceptions about AI’s impact on society and themselves. Around four in ten expect a positive impact while three in ten anticipate a negative one, both for society and them personally. When considering specific areas, AI is expected to have a positive impact on climate change monitoring, and public services including healthcare and crime prevention. Notably, over half the public think that AI is already used, at least sometimes, to deliver public services. However, there are concerns about its negative role in the spread of misinformation and job displacement.
6. Concerns about data security and the impact of AI are more pronounced among digitally disengaged individuals compared with the broader online population.
The vast majority of individuals with very low digital familiarity report being concerned about data security and management. Furthermore, this wave of the survey sees declining trust in organisations to keep their data safe, especially social media and big technology companies. When it comes to data, key concerns include unauthorised sales of personal data, insufficient security measures, and limited control over their data. Regarding AI, those with low digital familiarity recognise the positive impact of AI on society but are less likely to expect to benefit from it personally, with many anticipating negative effects.
3. Introduction
The Responsible Technology Adoption Unit’s (RTA) Public Attitudes to Data and AI (PADAI) Tracker Survey monitors public attitudes towards data-driven technologies, including artificial intelligence (AI), over time. This report summarises findings from the fourth wave (Wave 4) of research and identifies how public attitudes have changed since the previous waves (Wave 1, Wave 2 and Wave 3). The research was conducted by Savanta on behalf of the RTA.
The research uses a mixed-mode data collection approach comprising online interviews (Computer Assisted Web Interviews - CAWI) and a smaller telephone survey (Computer Assisted Telephone Interviews - CATI) to ensure that those with very low digital engagement are represented in the data.
Key information on each survey wave, including survey mode, respondent profile, sample size and fieldwork dates can be found in Table 1. Full details of the methodology, including notes on interpreting the data in this report, are provided in the Methodology section.
Table 1: Overview of survey Waves 1, 2, 3, and 4
Wave 1 (Dec 2021/Jan 2022) | Wave 1 (Dec 2021/Jan 2022) | Wave 2 (Jun/Jul 2022) | Wave 2 (Jun/Jul 2022) | Wave 3 (Aug/Sept 2023) | Wave 3 (Aug/Sept 2023) | Wave 4 (Jul/Aug 2024) | Wave 4 (Jul/Aug 2024) | ||
CAWI (Online sample) | CATI (Telephone sample) | CAWI (Online sample) | CATI (Telephone sample) | CAWI (Online sample) | CATI (Telephone sample) | CAWI (Online sample) | CATI (Telephone sample) | ||
Respondents | Demographically representative sample of UK adults (18+) | ‘Digitally disengaged’ (those with very low digital engagement) | Demographically representative sample of UK adults (18+) | ‘Digitally disengaged’ (those with very low digital engagement) | Demographically representative sample of UK adults (18+) | ‘Digitally disengaged’ (those with very low digital engagement) | Demographically representative sample of UK adults (18+) with regional boosts for Scotland, Wales, Northern Ireland | ‘Digitally disengaged’ (those with very low digital engagement) | |
---|---|---|---|---|---|---|---|---|---|
Number of interviews | 4,250 | 200 | 4,320 | 200 | 4,225 | 209 | 4,947 - Inclusive of the following boosts: Wales = 50; Scotland = 511; Northern Ireland = 530 | ||
Fieldwork dates | 29 November to 20 December 2021 | 15 December to 14 January 2022 | 27 June to 18 July 2022 | 1 to 20 July 2022 | 11 to 23 August 2023 | 15 August to 7 September 2023 | 15 July to 16 August 2024 | 15 July to 12 August 2024 |
4. Public recall of data-related news stories
More than two in five UK adults recall seeing a news story about data in the past six months. Echoing findings from previous waves, news stories recalled were most likely to relate to data breaches or leaks, misuse of data, or privacy concerns, though there have been changes since Wave 3 in the salience of these themes. Regional differences highlight distinct localised concerns, such as data breaches in Northern Ireland and privacy laws in Scotland. When compared with previous waves, the sentiment of the recalled news stories is shifting from predominantly negative to more balanced, with the most significant changes observed among the older age group.
4.1 Introduction
In previous waves, we have observed that the public’s perceptions of data appeared aligned with the perceived prevalence of certain media narratives around data. For example, last year we saw a peak in the recall of stories related to data breaches in policing in Northern Ireland, which was mirrored in an increase in concerns about data breaches compared with previous years. This suggests that media stories on data may be an important contextual factor in shaping public opinion on data more broadly. As a result, this section explores public recall of data usage in the media to determine the present context, before looking at overall attitudes towards data.
4.2 Public recall of data usage media stories
Respondents were asked if they had seen, read, or heard anything about data usage in the last six months through news articles, TV, or radio. More than two in five (44%) UK adults report having noticed such news stories, a slight decrease from Wave 3 (48%) but higher than Waves 1 (37%) and 2 (40%), as shown in Figure 1. This suggests that while there is some variability, overall awareness of data usage stories in the media has generally increased over time. Regional differences in reported exposure to data-related news exist, with those in Northern Ireland (51%) being more likely than those in England (44%), Wales (44%) or Scotland (42%) to recall a data-related news story. The volume of responses in Northern Ireland, was likely driven by the Police Service Northern Ireland (PSNI) data breach which occurred in August 2023 but received significant reporting in the lead up to Wave 4.
Figure 1: Public recall of news stories about data in the last six months (Showing % who recall such news stories)
Q9. Have you read, seen, or heard anything about data being used in the last 6 months, for example in news articles, or on TV or radio? BASE: All online respondents: November/December 2021 (Wave 1), n=4,250, June/July 2022 (Wave 2), n=4,320, August 2023 (Wave 3), n=4,225, July/August 2024 (Wave 4), n=4,947
Consistent with previous waves, the digitally disengaged population is more likely to recall data-related news stories than the broader UK public (49% vs. 44%). However, unlike the online population, their recall has remained stable throughout the duration of research, suggesting that such content is consistently reaching those who are less digitally engaged.
4.3 Shifting sentiments in data-related news stories
Those who recalled a news story about data use were asked to report the sentiment of these stories. Over recent waves, there appeared to be growing proportions that perceived data-related news stories as negative, increasing from 53% in Wave 2 to 65% in Wave 3, as seen in Figure 2. In a notable shift, Wave 4 shows a reduction in recalled negative portrayal, with 56% of adults viewing data usage news as negative, a 9 percentage point decrease from Wave 3. This drop in recall of negative media stories is especially pronounced among older adults (35-54 and 55+). Meanwhile, instances of positive recall have risen to 19%, up from 15% and 12% in Waves 2 and 3 respectively. This recall of positive stories contrasts with the trend observed in Wave 3, which saw a surge in recall of negative data stories. However, the data stories recalled this wave remain more negative than the data stories recalled in Wave 1, where 37% said the story was mainly negative and 25% said it was mainly positive. This suggests that, while stories highlighting the positive role of data are gaining traction, whether due to higher volume or higher recall of negative stories, it is negative data stories that are most salient among the public.
Figure 2: The recalled presentation of data in news stories over time (Showing % selected each option)
Q11. Overall, do you think this story presented the way data was being used positively or negatively? BASE: All online respondents who have read, seen or heard a story about data being used recently: November/December 2021 (Wave 1) n=1,499, June/July 2022 (Wave 2) n=1,678, August 2023 (Wave 3) n=1,303, July/August 2024 (Wave 4), n=1,312
The change in recall of negative data-related stories since Wave 3 is even more pronounced among the digitally disengaged audience, where recall of such stories dropped from two in three (67%) in Wave 3 to just under half (49%) in Wave 4 and is now at similar levels to Wave 2 (50%). Positive recall in this population has been relatively consistent and has not seen a statistically significant change this wave (20% in Wave 2, 21% in Wave 3, and 32% in Wave 4). The reduction in negative sentiment and the stable levels of positive recall among those with very low digital engagement indicate a shift in their perception of data stories, or a change in nature of news stories related to the use of data.
4.4 Dominant themes in data-related news
Among adults who could recall such news stories, a broader range of topics emerged compared with the previous wave. Stories such as electoral data breaches and the CrowdStrike outage were mentioned by a minority this year, reflecting prominent news stories reported on shortly before data collection started. However, over half (52%) of the general public could not remember what the story they had seen, heard, or read was about; an increase since Wave 3 (36%).
The most frequently mentioned news stories relate to data breaches and misuse of data. These are consistent with Wave 3 but show some fluctuations, as seen in Figure 3. For example, data breaches or leaks are the most widely recalled topic in Wave 4 (17%), though mentions halved from Wave 3 (36%). This reduction is observed across all demographic groups and across the UK. Misuse of data by government, companies or individuals (12%) and privacy concerns about data (8%) are also regularly mentioned, with mentions of the latter doubling since Wave 3 (10% and 4%, respectively).
Frequently mentioned negative news stories include NHS leaks of patient data and the PSNI data breach. While the topics of frequently recalled data-related news stories are generally consistent across the UK, some nuances appear. For example, residents of Scotland frequently mention concerns around privacy, the use of data in advertising, and data protection related laws and rights. Those in Northern Ireland are most likely to recall stories about data breaches or leaks, particularly due to recent coverage around the PSNI data breach. Although the PSNI breach occurred in August 2023 (around the time of Wave 3 data collection), it received significant coverage in May 2024, shortly before data collection for Wave 4, when the Independent Commissioner’s Office (ICO) announced a £750,000 fine for PSNI. The NHS leak of patient data also occurred in June 2024, shortly before data collection started.
Figure 3: Recall of what the news story was about (Showing all themes mentioned by those who recall seeing a news story about data in the past six months)
Q10. In a couple of sentences, please could you briefly tell us what the story you saw about data was about? Base: All CAWI respondents who say they have seen a news story about data in the last 6 months: Aug 2023 (Wave 3) n=2014, July/August 2024 (Wave 4), n=2,204 (coded responses to an open text question).
The digitally disengaged population most often recalls news stories about the use of data in healthcare (17%), which is far more prevalent than among the online participants (5%). Data breaches and leaks are also frequently mentioned among those with very low digital engagement (16%), though recall has decreased from Wave 3 (28%). In the context of the recent UK General Election, electoral data and breaches by the Electoral Commission (8%) are more prominently mentioned by the digitally disengaged audience than among the online sample.
5. Trust in data actors and views on the use of data in society
The NHS is the most trusted organisation of those tested, with academic researchers also garnering high levels of trust among the public. Conversely, social media companies receive the lowest levels of public trust. Trust in Government is also low, though there has been a minor uptick in trust since Wave 3. While attitudes towards data use are growing more positive, concerns around accountability persist. The public’s key anxieties remain data security and the unauthorised sale of data, which resonate particularly strongly with older individuals, while the younger population worry more about the environmental impacts of data processes. Overall, despite a gradual increase in trust and optimism about data practices, significant concerns remain.
5.1 Trust in data actors
Respondents were presented with a list of data actors and asked to indicate if they generally trust or do not trust each to act in their best interest. The NHS remains the most trusted organisation among those tested, with 85% of UK adults trusting its ability to act in the public’s best interest; consistent with Wave 3 (85%) but a slight decrease from the 89% trust levels noted in Waves 1 and 2. Academic researchers follow closely as the second most trusted (76%), holding stable trust levels across all waves. Meanwhile, trust levels for pharmaceutical researchers (68%), banks and financial institutions (68%), and regulators (67%) surpass two thirds of UK adults.
In contrast, social media companies (33%) and the Government (38%) find themselves at the lower end of the trust spectrum. Trust in social media companies remains static in the past three waves after a decline from Wave 1. Trust in the Government has seen an upward movement this wave (38% Wave 4 vs 31% Wave 3).
Figure 4: Trust in data actors over time (showing % Sum: Trust).
NB: Some actors were not asked across all four survey waves, so the chart only shows data for some of the waves.
Q1. To what extent, if at all, do you generally trust the following organisations to act in your best interest? Base: All CAWI respondents: November/December 2021 (Wave 1), n = 4,250, June/July 2022 (Wave 2), n = 4,320, Aug 2023 (Wave 3) n=4,225, July/August 2024 (Wave 4), n=4,947
Across all data actors digitally disengaged adults show lower levels of trust than the overall population, although the extent of these differences varies. The NHS remains the most trusted organisation, being trusted by 80% of those who are digitally disengaged (compared with 85% among the online sample). Banks and financial institutions sit in second place with 63% trust among the digitally disengaged. Utility providers come third (52%), a notable contrast with their seventh-place trust ranking in the online sample, suggesting a difference in which of these actors are trusted between the two populations. The discrepancy in trust is particularly noticeable when comparing online and digitally disengaged groups’ views of academic researchers (76% online versus 42% digitally disengaged) and pharmaceutical researchers (68% online versus 38% digitally disengaged). Trust in social media platforms is remarkably low among the digitally disengaged at just 6%, compared with 33% from the online population.
5.2 Trust in data actions among actors
Respondents were asked to what extent they trust various actors to keep data safe, effectively use data to improve products or services, use data to benefit society, let individuals make decisions about how data is used, and be open and transparent about what they do with data. Detailed responses per actor are captured in Table 2.
Trust in data actions largely mirrors overall trust in the actors, with the NHS the most trusted for all actions tested. However, trust in the NHS to use data to improve products or services has dropped since last wave (78% Wave 3, 73% Wave 4), as for using data to benefit society (75% Wave 3, 72% Wave 4), and being open and transparent about what they do with data (72% Wave 3, 69% Wave 4). See a case study about public perception of healthcare in the context of data and AI for more information.
Case study: Public perception of healthcare, data, and AI in the UK
The NHS enjoys high public trust, yet there is a noticeable gap between general trust in the organisation and confidence in its data management practices. Most of the public (85%) are confident in the commitment of the NHS to act in their best interest (see Figure 4). However, confidence in the NHS’s handling of data has declined, with only 73% trusting them to use data effectively, down from 78% in Wave 3. This decline is most notable among those aged 35-54 and 55+, as well as within the higher socio-economic grades (ABC1).
Meanwhile, health emerges as a critical concern for UK adults, with 35% identifying it as one of the most pressing issues currently facing the country, an increase from 28% in Waves 1 and 2, and 32% in Wave 3. This concern is particularly pronounced among those aged 55 and over (43%) and is higher in Northern Ireland and Wales (both 42%) compared with England (34%).
Despite health being a critical issue for the UK public, there is optimism about the potential benefits of data and AI in healthcare, as discussed in Chapter 6. Health is seen as the most significant area where data can be used to make improvements that benefit the public in this country, with 21% of adults selecting it as the top opportunity. Optimism about AI in healthcare is significant, with 52% expecting AI to have a positive impact on this area. This belief is more common among those aged over 55 (56%) and people from Black ethnic backgrounds (64%). However, there is a call for careful management to prevent negative outcomes of AI in healthcare, with 29% ranking it among the top three areas that government must carefully manage. Addressing these concerns is essential to harness the full potential of data and AI in improving healthcare outcomes.
Banks and other financial institutions, academic researchers at universities, and researchers at pharmaceutical companies all engender relatively high levels of trust across all data actions. Trust levels for these statements for all actors have remained stable since Wave 3, with the only exception being a decrease in trust among researchers at pharmaceutical companies to let individuals make decisions about how data is used (54% in Wave 3, 49% in Wave 4).
On the opposite end of the trust spectrum lie social media companies, which have the lowest levels of trust among all statements. The Government and big technology companies also see relatively low levels of trust. For social media companies and big technology companies, trust for all statements has remained stable since Wave 3, while for the Government, there have been increases in trust for all statements since Wave 3.
Different actors are seen as more or less trustworthy based on the specific data action in question. For example, social media companies are least likely to be trusted to be open and transparent about what they do with data (30%) and using data to benefit society (30%). By contrast, other than keeping data safe (48%), the Government is most likely to be trusted to effectively use data to improve the products or services you receive (46%) and to use your data to benefit society (46%) (see case study below for more information).
Table 2: Trust in organisations, and in their actions with data (Showing % Sum: Trust)
Q14. To what extent, if at all, do you trust the [organisation] to…? BASE: Approximately half of all online respondents per organisation shown, July/August 2024 (Wave 4), n=4,947
Case study: Trust in the Government and its use of data
UK adults’ trust in the Government to act in their best interest has risen since the previous wave (31% Wave 3 vs. 38% Wave 4), although it still trails behind other organisations, as shown in Figure 4. This boost in confidence spans various demographic groups, including gender, socioeconomic status, and all devolved nations. Notably, trust has increased among those aged 18-34 showing a rise from 34% to 41% in Wave 4, and those aged 35-54 from 26% to 38%. However, the digitally disengaged population remains less trusting, with 22% expressing trust in the Government; (a 5-percentage point decrease from Wave 3, but not statistically significant).
This overall rise in government trust among the online population is mirrored in the heightened confidence in its data-handling practices. All five types of data actions tested have seen increases in trust since the previous wave. Nearly half of adults trust the government to keep their data safe (48%, +6 percentage points), its commitment to use data to enhance services (46%, +4 percentage points), use data to benefit society (46%, +5 percentage points), allow the public to make decisions about their data (41%, +4 percentage points), and its transparency about data use (41%, +4 percentage points). As with overall trust in the Government, this enhanced trust in its data-related actions is mainly seen among 18–34-year-olds and 35–54-year-olds. However, the digitally disengaged group lags in this upsurge of trust, with fewer adults trusting the government to keep their data safe compared with Wave 3 (25%, -6 percentage points).
Among the UK public, there is a prevailing belief that government departments exchange data about specific individuals. Nearly half (46%) assume data sharing occurs occasionally, while over a quarter (27%) believe this ‘always’ takes place. This sentiment is particularly strong in Northern Ireland, where 78% believe data is always or sometimes shared between government departments; a sentiment more pronounced than in England (73%), Wales (71%), and Scotland (68%). Notably, men (30%) and those in the C2DE socioeconomic group (29%) are more likely to assume that such data sharing is a constant occurrence. Regression analysis uncovered that older adults are more likely to believe cross-government data sharing is occurring compared with younger adults. Moreover, individuals who agree that they are made aware of how their data is going to be used by organisations were also more likely to think this data sharing was occurring, as were individuals who reported feeling distrustful of the government (Annex 1a).
Similar perspectives on trust in the Government and views on data sharing are observed in the digitally disengaged population, suggesting these stances feed into broader public trends. Approximately two-thirds (65%) believe that government departments share individual-specific data with each other, with a quarter (25%) convinced this always happens and 40% believing it happens occasionally.
5.3 Value of data use to society
The survey also explores the public’s views on the value of data use by presenting respondents with a series of statements and asking them to indicate the extent to which they agree or disagree with each. As shown in Figure 5, the public remain more likely to recognise the individual-level benefits of data than the societal-level benefits, with persisting concerns around equitable and responsible use of data.
Most adults (58%) agree that data is useful for creating products and services that benefit them, a steady increase since Wave 1. A large share (44%) also agree that collecting and analysing data is beneficial for society, holding steady from Wave 3 (44%) and increasing from 40% in the first two waves. Generally, those who agree that data is useful for creating products and services that benefit them also agree that collecting and analysing data is good for society, with those aged 18-34 (63% for creating products and services, 51% for collecting and analysing data) or 35-54 (62% for creating products and services, 47% for collecting and analysing data), those in the ABC1 socio-economic group (60% for creating products and services, 47% for collecting and analysing data), and graduates (63% for creating products and services, 50% for collecting and analysing data) being more likely to agree with both statements.
Over four in ten (43%) now agree that organisations disclose how data will be collected and used, increasing from 38% in Wave 2 to 43% in Wave 4 (NB: statement was not asked in Wave 3). Agreement that people have control over who uses their data and how is lower, at 35%, consistent with Wave 3 (35%) but a significant increase since Wave 2 (29%). Regression analysis shows that those who are younger, those in the C2DE socio-economic group, or those of Asian or Black heritage are more likely to feel they have control over who uses their data and how relative to older, those in the ABC1 socio-economic group, and White adults. Analysis also reveals that individuals living in Northern Ireland are less likely to feel this way relative to individuals living in London; this was the only significant effect of region in this model and could be related to increased coverage of the PSNI data breach at the time of fieldwork (Annex 1b).
Despite a large proportion of adults recognising the positive contributions of data to society, concerns about accountability for data misuse persist, with two in five (40%) agreeing that misuse of data by organisations is addressed, a decrease from 45% in Wave 3. Moreover, less than a third (31%) agree that data use benefits all social groups equally, slightly down from 33% in Wave 3 but an increase from 27% in Wave 2. Regression analysis found that younger adults, adults in the C2DE socio-economic group, and adults of Asian, Black, and Mixed ethnic heritage were more likely to agree that organisations are held accountable compared with older adults, those in the ABC1 socio-economic group, and White adults (Annex 1c).
Figure 5: Agreement with the following statements about data (showing % Sum: Agree).
NB: Some statements were not asked across all four survey waves, so the chart only shows data for some of the waves.
Q12. Please indicate how much you agree or disagree with each of the following statements Base: All respondents: November/December 2021 (Wave 1), n = 4,250, June/July 2022 (Wave 2), n = 4,320, August 2023 (Wave 3), n = 4,225, July/August 2024 (Wave 4), n=4,947
The digitally disengaged group are more likely to agree that collecting and analysing data is good for society (56%) compared with the online population (44%), suggesting that even those less engaged with the digital world recognise the broader societal benefits of data use. However, they are less likely to see data as useful for creating beneficial products and services (49% vs. 58% online population), indicating a gap between understanding data’s broader impact and seeing its usefulness for themselves.
5.4 Perceived risks of data use in society
Respondents were also presented with a list of possible risks related to the use of data in society and asked to select those which they perceive as being risks. As noted in previous waves, the most prevalent concerns were that data is not held securely or is hacked or stolen (57%), possibly linked to prominent news coverage around data breaches. Another prevalent concern regards the data being commodified by organisations for profit (54%). Around a third of the UK public express concerns over data being used for surveillance purposes (33%) and a lack of choice over when their data is shared (32%). The primary concerns about data use have remained consistent since Wave 3, highlighting their continuing significance.
The older population (55+) tends to be the most concerned about these risks, except for the environmental consequences of data processing and storage, which is an issue that particularly resonates with younger adults (18-34). These findings are consistent with previous waves of the survey.
The digitally disengaged audience express high levels of concern over each of the listed potential risks. Around nine in ten consider data being sold onto other companies to profit from (93%), data being hacked or stolen (92%), important decisions being made without human input (91%), people not having enough choice about when their data is shared (89%) and some people in society being left behind (88%) as risks linked to the use of data in society.
6. Preferences for data sharing
When given options for how and why public and private organisations could share people’s personal data, the preferences of the public are primarily shaped by the actors involved in the data sharing. People are less influenced by the specific purpose of the data sharing or by the presence of governance safeguards. Scenarios in which data was shared or received by NHS services are viewed most favourably, while people are less inclined to select scenarios involving big technology companies in either the sharing or recipient role. Whether data is anonymised or identifiable has minimal overall impact on preferences, except in the context of data shared by schools, where it noticeably affects choices.
6.1 Introduction
There is a growing consensus, both among public bodies and outside commentators, that increased data sharing between different organisations in the public and private sectors could be of great value to the UK public. Commentators often point to actions taken in the pandemic as proof, for example the Clinically Extremely Vulnerable People Service. In this project the NHS shared data on those most vulnerable to coronavirus with local governments and supermarkets to ensure they could obtain food and medicines while also remaining at home and minimising their risk of infection. Since then, there have been multiple other such data-sharing schemes attempted, such as the Ministry of Justice’s BOLD programme. However, in all this work there has also been an awareness of how important it is that any greater sharing of public data is done with public consent. This is perhaps best articulated by the first recommendation of the Office for Statistics Regulation’s 2023 report Data Sharing and Linkage for the Public Good:
The government needs to be aware of the public’s views on data sharing and linkage, and to understand existing or emerging concerns. Public surveys such as the ‘Public attitudes to data and AI: Tracker survey’ by the Centre for Data Ethics and Innovation (CDEI) provide valuable insight[footnote 1]. They should be maintained and enhanced, for example to include data linking.
The following chapter takes up this recommendation, by producing a large-sample quantitative study of preferences on data sharing in the UK. By using a conjoint experiment, we can assess the influence of different features of two hypothetical data-sharing scenarios, to uncover the effects of these features on peoples’ comfort with the sharing of personal data.
6.2 Choice-based experiment (Conjoint)
We incorporated two conjoint experiments into the online survey to study people’s preferences for how organisations might collect and share their personal data. What follows is a brief description of the design of the conjoint experiment and its outputs, to help clarify the results that follow. The results of the experiment show which of these features influenced people’s preferences. A full list of all features can be seen in Annex 2. For a full description of the method, please see the Methodology section. An example of how pairs of scenarios were presented to respondents is illustrated in Figure 6.
Figure 6: Example of a possible scenario pairing, as presented to respondents in the anonymised (Model A) conjoint experiment
These features of the data sharing scenarios are grouped in the analysis into the following four overarching categories, which we call ‘attributes’:
- Attribute 1: Actor 1. The organisation collecting and sharing the data. An example feature would be ‘NHS services’.
- Attribute 2: Actor 2. The organisation receiving the data shared by Actor 1. The conjoint used the same list of organisations for both Actor 1 and Actor 2, with a restriction in place to ensure that the same organisation did not appear in both scenarios.
- Attribute 3: Use case. The purpose of the data sharing. An example feature would be ‘measure the quality of their work’.
- Attribute 4: Governance mechanism. A step taken to reassure people that their data will remain secure even when shared between organisations. An example feature would be ‘members of the public will be able to decide whether certain uses of the shared data are allowed or not’.
By analysing which scenarios were chosen by respondents across all pairings, we can assess the following:
-
The relative importance of each attribute as a driver of the general public’s preferences regarding data sharing.
-
Whether individual features of a data sharing scenario make respondents more or less likely to prefer it.
We conducted two conjoint experiments (Model A and Model B), each shown to half of the online survey sample. These experiments are identical, aside from one feature. In Conjoint Model A, respondents were told that the personal data being shared was anonymised (e.g. people using the data cannot match the information to an individual person), whereas in Conjoint Model B respondents were told the data being shared was identifiable (e.g. people using the data can match the information to an individual person). By comparing the results of these two experiments, we can assess whether and how much peoples’ preferences were affected by this different context.
Please note that due to the nature of the conjoint experiment, people were not given the option of simply refusing to share their data. They were asked to pick either the best option of the two scenarios shown, or the ‘least bad’ option. As such, results should be interpreted in the context where refusing to share data wasn’t a choice.
6.3 Attribute analysis
Respondents’ preferences in data sharing scenarios are largely influenced by the organisations involved, with the organisation sharing data (Actor 1) and the organisation receiving the data (Actor 2) driving over 70% (73% Model A / 72% Model B) of adults’ preferences in both conjoint experiments.
Individually, Actor 2 is slightly more influential than Actor 1, driving 39% of choices in Model A and Model B, compared with Actor 1’s driving 34% in Model A and 33% in Model B. Both Actors are much more influential than use cases (driving 12% of decisions, both Models), and governance mechanisms (15%, both Models). In summary, individual decisions are slightly more influenced by which organisation was receiving the data, than by which organisation is sharing the data.
The consistency of these findings across the two conjoint experiments suggests that comfort with data sharing is less about whether data is anonymous or identifiable, and more about who is collecting or using the data.
Table 3: Overview of results for the four attributes of each data-sharing scenario, by Model
Model A | Model B | ||
– | – | – | |
Actor 1 | 34% | 33% | |
---|---|---|---|
Actor 2 | 39% | 39% | |
Use case | 12% | 12% | |
Governance mechanisms | 15% | 15% |
CONJOINTQ. Which of these data-sharing scenarios do you most prefer, assuming that all the data shared is [Model A] anonymised (e.g. people using the data cannot match the information to an individual person) [Model B] identifiable (e.g. people using the data can match the information to an individual person). (BASE: All online respondents July-Aug ’24 (Wave 4) who saw each Model, Model A n=2468, Model B n=2479
6.4 Impact of Actors on preferences for data sharing
The same list of organisations was used for both the data-sharing Actor 1 and data-receiving Actor 2 attributes. Organisations have a similar relative impact on preferences, regardless of whether they are sharing or receiving data, with schools as the only exception. This section discusses the results by actor, grouped according to whether they had a positive, negative or mixed impact on respondents’ preferences. It is important to note that any impact identified by the conjoint experiment is a relative score, which means that any positive or negative impacts an actor has on preferences, should be interpreted as a positive/negative impact compared with the impacts of the other tested actors.
Starting with actors with a positive impact, NHS services, whether sharing or receiving data, has the greatest positive impact on respondent preferences among all the actors tested. This is the case across all demographic groups in both Models, though its impact is stronger among White adults compared with those of an ethnic minority background.
This finding aligns with results from other questions in the survey looking at trust levels in the NHS regarding data tasks (see Healthcare case study in Chapter 2). While the share of UK adults that trust the NHS to perform various data actions varies (e.g. 73% trust the NHS to effectively use data, while 66% trust the NHS to let them make decisions about how their data is used), the level of trust in the NHS to perform each data action outranks all other tested organisations. Given these results from elsewhere in the survey, the preference seen for NHS services in the conjoint possibly reflects an overarching trust among adults in the organisation to act in their best interest.
Local Authorities as an actor also has a universally positive impact on preferences, albeit milder compared with NHS services.
On the contrary, big technology companies as both data sharers and receivers have the strongest negative impact of all actors on data sharing preferences. This negative impact is larger when technology companies are receiving data, suggesting that sharing of public data with these companies is likely to receive pushback.
This finding aligns with other results beyond the conjoint, which reveal a relative distrust in big technology companies – for instance, social media companies are consistently the least trusted actor to perform all tested data actions. However, the term ‘big technology companies’ used in the conjoint differs from distinctions made in other survey questions between ‘big technology companies’ and ‘social media companies’. It is therefore possible that respondents in the conjoint may have been thinking of either type of organisation when choosing their preferred scenario.
Job centres as an actor has a smaller negative effect on preferences for data sharing compared with big technology companies, but its impact is more negative compared with other types of public organisation tested in the conjoint. Preferences differ depending on whether job centres receive data that is identifiable or anonymised, with people feeling less comfortable with job centres receiving identifiable data.
The involvement of private healthcare providers also has a negative impact on preferences: it has a small negative impact on people’s preferences when providers are in the data sharing role (Actor 1), and a relatively moderate negative impact when they are in the data receiving role (Actor 2). This suggests that, while the public is not particularly positive toward private healthcare providers, they are more concerned about them receiving data than sharing it. This echoes a finding from elsewhere in the survey (see Chapter 2) that half of adults (54%) see the risk of data being sold for profit as one of the three greatest risks for data use in society. It is when private healthcare is in the position of receiving data that it could profit from, that people are most wary about its involvement.
The police have a small negative impact on people’s preferences for data sharing in both roles. This may be a result of the perceived risk that data shared with police in this way might be used for surveillance, as seen in the survey; a third (33%) selected this as one of the three greatest risks for data use in society.
The inclusion of schools as an actor in the experiment provides us with two findings. First is that people are opposed to schools sharing identifiable data, most likely due to sensitivities around sharing children’s data. The second finding is that, if the data shared are anonymous, people’s negativity around schools sharing data reduces.
In the identifiable data Model B, schools occupying the data sharing role had a greater negative impact on preferences than data sharing by private healthcare providers, the police and job centres. Only identifiable data sharing by big technology companies has a more negative impact on preferences than identifiable data sharing by schools. Schools receiving identifiable data also has a slight negative impact on adults’ preferences. In sum, it’s clear that UK adults are opposed to the idea of schools sharing identifiable data.
In contrast, in the Model A experiment, anonymised data sharing by schools has a much less negative impact than in the identifiable experiment. Anonymised data sharing by police and job centres has a larger negative impact on preferences than sharing by schools. In addition, schools receiving anonymised data has a slight positive impact on peoples’ preferences, as opposed to the slight negative impact seen when schools share identifiable data. So, making data anonymous may allay peoples’ fears about data sharing by schools in particular.
Figures 7a and 7b: Impact of which organisation shares (7a) and receives (7b) personal data, on respondent preferences, by Model
6.5 Impact of use cases on preferences for data sharing
Use cases as an attribute have the lowest overall impact on people’s preferences, which may be because the tested use cases were only specified at a high level. This was necessary to ensure the data sharing scenario made sense regardless of which actors were sharing or receiving the data. However, more specific use cases might have garnered a different response. That said, different use cases do have different types of impact, even if they are slight. For example, either helping the data receiver to ‘track progress towards meeting a major objective’, or ‘measure the quality of their work’, has a slight negative impact on people’s preferences. Conversely, improving the experience of those who interact with the data receiver has a slight positive impact. Use cases that help the data receiver learn more about the UK show a very slight positive impact in anonymised data scenarios and a neutral effect when the data is identifiable.
6.6 Impact of governance mechanisms on preferences for data sharing
Governance mechanisms, like use cases, only have a slight impact on people’s data-sharing preferences. However, their impact does vary between the two conjoint models. The mechanisms of full transparency about who can access shared data has a slightly positive impact on preferences with anonymised data but has no impact with identifiable data. The use of trusted research environments and time-limited data access have a slight negative impact in Model A (anonymised) and are neutral in Model B (identifiable).
The other tested mechanisms include full transparency about all data usage, which has a neutral impact in both Models, and public control over certain data use permissions, which slightly improves preferences in both Models. These two outcomes suggest that people respond better to mechanisms on clarifying or adjusting the people with authority over the data and how it is used, rather than mechanisms that either impose mechanical restrictions (e.g. TREs, time limits) or that clarify how the data will be used.
7. Familiarity with AI and views of its impact
Self-reported awareness of artificial intelligence (AI) is nearly universal among the online population, with most adults now saying they can explain the term. Despite increased awareness, the level of familiarity varies notably across ages, ethnic groups, and regions. 18-34-year-olds and those in ABC1 socio-economic groups generally have a better grasp of AI, suggesting an age gap in how widely it’s understood. Public anxiety around AI remains high, as seen in negative words like ‘scary’ and ‘worried’ dominating top-of-mind associations. However, associations also show changing conceptions of AI in the public consciousness, with a shift from futuristic visions of ‘robots’ to understanding now based more around specific uses of this technology such as large language models and chatbots, which are becoming increasingly prominent in day-to-day lives.
7.1 Reported awareness of AI
Nearly every adult in the UK now self-reports being aware of AI, with 96% saying they are aware of the term; an increase from 89% in Wave 2 and 95% in Wave 3. Notably, there has been a rise in deeper familiarity with this form of technology, with 71% now able to at least partially explain AI, as opposed to 57% and 66% in Waves 2 and 3 respectively.
While reported familiarity with the term ‘artificial intelligence’ is consistent across demographic groups, the ability to explain the term varies. 18-34-year-olds are more likely (79%) to report being able to explain the term, at least partially, than those aged 35-54 (72%) or 55+ (63%). Graduates (79%) are also more likely than non-graduates (65%) to say they are able to explain what AI is, at least partially. Additionally, 77% of those in ABC1 socio-economic grades feel more able to explain AI, compared with 63% in C2DE socio-economic grades, indicating a potential knowledge gap.
The proportion of UK adults who report they can explain what AI is in detail has grown this wave, reaching the highest levels since tracking began (see Figure 8). Young people lead here too, with 29% of those aged 18-34 reporting being capable of detailed explanations compared with those in older age brackets (15% of those aged 35-54 and 7% of those aged 55+). There are also regional differences in reported knowledge of AI. In London, one quarter (25%) report being able to describe AI in detail; a higher share than every other UK region and devolved nation, and likely attributable to the high concentration of the technology industry in the capital.
Figure 8: Awareness of AI over time (Showing % selected each option)
Q21. Have you ever heard of the term Artificial Intelligence (AI)? BASE: All online respondents: June/July 2022 (Wave 2) n=4320, August 2023 (Wave 3), n=4225, July/August 2024 (Wave 4), n=2,204
The digitally disengaged population has shown a marked increase in self-reported awareness of AI across the survey waves, growing from 65% in Wave 2 to 85% by Wave 4. This increase is primarily driven by shallow levels of awareness, with a noticeable uptick in those who have heard of AI but cannot explain it (44% in Wave 3, 48% in Wave 4) as well as those who can offer a partial explanation (28% in Wave 3, 34% in Wave 4). This contrasts with the online population, where the increased familiarity with the term is driven by an increase in the proportion who say they could explain the term in detail.
7.2 Words associated with AI
Public anxiety around AI and its associated impacts was brought to light when respondents were asked to provide one word to represent how they feel about AI (see Figure 9). Negative associations dominate, reflecting concern felt by large segments of the UK public. In fact, three of the four most mentioned words are explicitly negative, with the fourth being neutral. The most frequently mentioned word was ‘scary’ (n=444), followed by ‘worried’ (n=289), ‘unsure’ (n=159), and ‘concerned’ (n=151). Only one of the top twelve words, ‘good’ (n=116), was unequivocally positive.
In line with broader survey trends showing that younger people have more positive perceptions of AI, younger demographics have more positive word associations compared with older groups. Among those aged 55+, the ten most mentioned words have negative connotations. While attitudes of 35–55-year-olds also lean negative, words like ‘good’, ‘excited’, and ‘future’ are among their top ten. Adults aged 18-34 are more likely to use explicitly positive terms like ‘good’, ‘smart’, ‘intelligence’, and ‘future’, indicating that concerns and optimism about AI vary across age groups.
Figure 9: Word cloud of public sentiment towards AI by UK adults, Wave 4 (visualising the top 50 most often mentioned words)
Q22. Please type in one word that best represents how you feel about ‘Artificial Intelligence’. Base: All online respondents to say they have heard of AI in July/August 2024 (Wave 4) and to leave a valid response, n=3225
While the words that the public associate with AI have not changed much since Wave 3, looking back to Wave 2, there are notable linguistic differences. The incidence of the words ‘scary’ (Wave 2 n=292, Wave 4 n=444) and ‘worried’ (Wave 2 n=171, Wave 4 n=289) have increased, indicating that while familiarity with the term ‘artificial intelligence’ has increased, this may not have translated into greater comfort with its increasing prevalence in society. In addition, associations with the word ‘robot’ decreased from Wave 2 (n=228) to Wave 3 (n=151) and again to Wave 4 (n=103). This possibly indicates that as awareness of AI has increased and there has been greater uptake of AI systems like chatbots, the public’s understanding of AI has moved beyond sensationalist conceptions of the term towards a more realistic understanding.
8. Use of AI chatbots and knowledge of how AI is being trained
The majority of the public have used an AI chatbot for personal or work-related purposes within the last three months, with 44% using an AI chatbot to do either of these activities at least once a month. For personal use, four in ten adults report at least monthly chatbot usage. Work-related usage is lower, with nearly three in ten using it at least monthly. The use of AI chatbots by UK adults for both personal and work-related matters has seen a notable increase this wave. Use of AI tools for visual content creation is less prevalent, with roughly a quarter of the public utilising such software at least once a month for both professional and personal purposes. Yet, despite their growing popularity, there is a marked disparity in the understanding of how AI systems, including chatbots, are trained. The connection between AI development and data usage is not well grasped among UK adults, with seven in ten reporting they know only a little or nothing about this subject. In contrast, expectations for AI usage in public service delivery are high, with more than half of the public believing that AI is at least sometimes used, although some remain unsure about its role in this context.
8.1 Growing use of AI chatbots
To understand public adoption of AI, respondents were given a brief description of large language models (referred to as ‘chatbots’ in the survey) and then asked how often they had used them in the past three months for personal or work-related purposes. The majority (60%) of the public report having used an AI chatbot for either personal or work-related activities within the last three months. Over four in ten (44%) say they have used chatbots for either purpose at least once a month and nearly a quarter (23%) have done so at least once a week.
For personal usage, four in ten (40%) adults report using chatbots at least once a month, an increase from 34% in Wave 3. Chatbot usage for work saw lower levels of uptake, with nearly three in ten (28%) reporting using them at least once a month, an increase from 24% in Wave 3 (see Figure 10). 18–34-year-olds, along with men, Londoners, ABC1 respondents, and graduates, are particularly more likely to have had at least monthly interactions with chatbots. Furthermore, individuals in England (29%) and Northern Ireland (29%) are more likely to have used chatbots for work-related reasons at least once a month than their counterparts in Wales (21%) and Scotland (20%). For personal usage, only England sees higher levels of at least monthly usage (41%) compared with Scotland (33%) and Wales (34%).
Figure 10: Use of AI chatbots over time (Showing % who say they used them at least once a month)
Q36. Thinking about the last three months, how often, if at all, have you used chatbots for personal use, in your day-to-day life, and for work or in your job. Base: All respondents: August 2023 (Wave 3), n = 4,225, July/August 2024 (Wave 4), n=4,947
8.2 Use of AI for visual content creation
While less common than the use of chatbots, the use of AI to create and edit visual content does have some presence among the UK public, as shown in Figure 11. Over a quarter (27%) of adults report having used AI tools to create visual content outside their workplace at least once per month, and 23% report doing so for work. Roughly 1 in 10 UK adults (11%) use content-creation AI weekly outside of work, and an equal proportion (11%) use it at least once a week for professional tasks. However, the majority of the public do not use AI for these purposes, with more than half of UK adults reporting that they have not used AI for image, video, or audio creation either outside of work (57%) or professionally (65%) in the last three months.
Men, 18–34-year-olds, university graduates, residents of London and those with high levels of digital engagement are more likely to have used such AI tools at least monthly. AI usage for content creation is higher in Northern Ireland and England outside of work (both 28%) and sits at 26% and 24% respectively for work-related tasks, compared with the lower use rates seen in Scotland (22% outside of the workplace and 19% at work) and Wales (23% outside of the workplace and 19% at work), as shown in Figure 11.
Figure 11: Use of AI to create or edit images, video, or audio by Nation (Showing % who say they used each at least once a month)
Q41. Thinking about the last three months, how often, if at all, have you used AI to create or edit images, video or audio outside of work, and for work or in your job? Base: All respondents: July/August 2024 (Wave 4), n=4,947
8.3 Knowledge about data used to train AI
Despite the increasing use of AI technologies, there is a notable lack of knowledge about how data is used to train AI models, with 71% of adults having minimal or no knowledge about this topic. Just 6% of adults say they know a great deal about how data is used to train AI systems, while 19% claim to know a fair amount, as shown in Figure 12. Altogether, this suggests a quarter of UK adults have some understanding of how AI is trained with the use of data, however it is important to note that this knowledge is self-reported and therefore could be subject to biases. In contrast, a third of adults (34%) report having limited knowledge, and 37% have none on this subject.
Figure 12: Knowledge of how data is used to train AI (Showing % selected each option)
Q42. Before today, how much, if anything, did you know about how data is used to train AI systems? Base: All respondents: July/August 2024 (Wave 4), n=4,947
Among those who report knowing at least a fair amount about how data is used to train AI, men, 18–34-year-olds, and graduates are more prevalent. Regional disparities are evident too, with the proportions that know at least a fair amount higher in Northern Ireland (28%) and England (26%) than in Scotland (19%) and Wales (21%). This reflects the usage patterns of AI chatbots and AI tools for visual content creation, suggesting a possible link between engagement with AI technologies and understanding its underlying functionalities, characteristic of early adopters of this type of technology.
Regression analysis shows that females, older people, individuals living in Scotland or Yorkshire & Humberside, non-graduates, people from C2DE socio-economic groups, those who report feeling unable to explain AI, and those using chatbots less than monthly are all relatively less likely to know how data is used to train AI. Analysis also demonstrates that individuals of a Black or Mixed ethnic background and people who agree that they are made aware of how their data is going to be used by organisations are relatively more likely to self-report understanding how data is used to train AI (Annex 1d).
9. Balancing opportunities and risks of AI
The UK public has mixed perceptions about AI’s impact on society and themselves. While many view AI positively on a societal level, fewer are optimistic about its impact on them personally. The public acknowledges that AI has the potential to bring both benefits and challenges. Whilst it is recognised for its potential to have a positive impact on sectors like healthcare and crime prevention, there are also concerns about its potential to cause job displacement and spread misinformation. The varying levels of optimism and pessimism across different demographics suggest the public associate a diverse range of hopes and fears with the future of AI.
9.1 Impact of AI on society versus themselves
Overall, UK adults have mixed perceptions of AI’s impact on society and on themselves personally, as shown in Figure 13 [footnote 2]. When considering AI’s societal impact, 43% of adults believe it will be positive, slightly outpacing the 33% who expect the impact to be negative. This suggests a tendency towards optimism about AI’s potential benefits for society as a whole. When it comes to AI’s impact on them personally, a slightly smaller proportion (39%) anticipate a positive effect, while 29% foresee a negative impact. This indicates that while many people see the broader societal benefits of AI, they are slightly less certain about its benefits for them individually. In addition, around 20% hold a neutral view on AI’s societal impact, and 23% remain neutral about its personal impact, suggesting a large share of the public is still undecided or ambivalent about AI. Notably, the share of those who say they “don’t know” about AI’s personal impact is twice as high as those who say they “don’t know” about AI’s societal implications (8% versus 4%), potentially indicating a lack of awareness of AI’s implications at an individual level.
Figure 13: Opinions on the impact AI will have on them personally and on society (Showing % selected each option)
Q23c. On a scale from 0-10 where 0 = very negative impact and 10 = very positive impact, based on your current knowledge and understanding, what impact do you think Artificial Intelligence (AI) will have overall on society and you personally? Base: All respondents: July/August 2024 (Wave 4), n=4,947
Perceptions of AI’s impact vary across demographic groups, with males, 18–34-year-olds, graduates, those of an ethnic minority background, and those with high digital engagement being generally more optimistic about AI’s impact on both society and themselves. This is evident in the results of the regression analysis which shows that females, older adults, non-graduates, those in the C2DE socio-economic groups, and those less able to explain AI are all relatively less likely to predict a positive impact of AI on society (Annex 1e) or personally (Annex 1f).
In comparison, optimism about personal benefits from AI is higher in Northern Ireland and England (both 40%) than in Scotland and Wales (both 33%). That said, regression shows that individuals living in Northern Ireland, Scotland, Wales, and several regions within England are less likely to feel optimistic about the impact of AI on society (Annex 1e) and on them personally (Annex 1f) relative to individuals living in London; this is possibly due to the high concentration of the technology industry in the capital.
Digitally disengaged adults are more pessimistic about AI overall than the online population. Half (51%) expect AI to have a negative impact on them personally, compared with 17% who feel positive. However, their views are more balanced when it comes to AI’s impact on society, with 43% believing it will have a positive impact and 34% foreseeing a negative one.
9.2 AI’s potential benefits and risks
UK adults were asked to rate the impact they think AI will have on various situations on a scale from positive to negative. Results reveal varying degrees of recognition for AI’s impact across different sectors. More details on how AI risks are depicted on social media, and how selected topics of risks are reported in online conversations, are captured in the Social Listening boxes throughout this chapter.
9.3 Social Listening: Conversations about the risks of AI
The risks of AI were often presented in a sensational style in the social media posts of news media accounts, with many drawing on the comments of a high-profile technology figure who warned of AI’s potential threat to humanity. Drawing on comments by industry experts, some posts highlighted the potential for a “loss of control” of AI technology while others presented AI as an existential threat to the world.
AI technology is as dangerous as nuclear weapons – we should be worrying about it!
Tech figure has repeatedly warned about the potential risks of AI, warning of its potential for civilisation destruction.
In contrast, social media posts more broadly covered a range of risks posed by AI, including increased surveillance, misinformation, and fake media, and the threat to businesses of AI-powered cyber-attacks. There was also a focus on the risk that the UK might not have the infrastructure in place to realise “the huge potential of AI”. Posts cited “weaknesses” in technology and data infrastructures but also other barriers to technology adoption, such as investment and trust in the technology.
Report shows that AI-powered cyber threats have impacted three quarters of UK businesses.
AI could grow UK productivity by over £100 billion, transforming efficiency and saving businesses billions of hours every year. But there are challenges to adoption including trust issues and not enough investment.
Expected potential gains from AI – for people, society and the economy - are threatened by substantial weaknesses in the UK’s technology infrastructure. We want to create diverse, fair data-centric AI. Find out more in our white paper #data #AI
AI for climate change monitoring emerges as the most recognised benefit, with 54% of adults acknowledging its potential to have a positive impact in this space (this was a new option introduced this wave). More details are provided in the Case study and Social listening boxes featured below.
9.4 Social Listening: AI and climate change
Echoing the survey findings about the public’s optimism towards using AI to monitor climate change, some social media posts cited the potential of AI to track the climate and to help “combat climate change”. Claims of how AI will help to tackle climate change were often vague. The more concrete examples cited included the use of AI to enhance climate forecasting, and the use of AI to identify ways of reducing energy consumption or emissions.
Breakthrough in climate and weather forecasting with the help of AI.
Industry head says AI will push energy transition and tackle climate change.
Alongside more optimistic posts about the benefit of AI in tackling climate change, was a focus on the AI industry’s energy consumption, specifically “energy hungry data centres”, which some noted were “driving emissions up.”
Tech giant admits that their carbon emissions are bigger than ever thanks to the energy demands of AI.
Many of the posts referring to AI and climate change and energy consumption appear to be driven by comments made by a high-profile AI industry figure, who defended the industry’s energy consumption and offered up AI as a solution to climate change.
Industry head argues that AI’s energy consumption should not be a big concern, as AI will aid improvements in efficiency and support the transition to sustainable energy.
Case Study: Public perceptions of climate change, AI, and data
While the public is optimistic about AI’s potential to help monitor and combat climate change, this view is not matched by awareness of AI’s negative environmental impacts. Additionally, climate change is not seen as a critical issue by the majority of UK adults, indicating a gap in understanding the broader implications of AI and a lower prioritisation of climate change.
There is notable optimism regarding AI’s role in monitoring climate change, with 54% of UK adults believing AI will have a positive impact (see Figure 14). This sentiment is particularly pronounced among ABC1 socio-economic groups, those from Black ethnic backgrounds, London residents, university graduates, and those with high digital engagement. Even among the digitally disengaged, 55% agree on AI’s positive role in monitoring climate change, although 30% of this population believe AI will have a negative impact, compared to 14% of the online population who think the same.
Despite optimism regarding AI’s role, only 17% of the public consider climate change and the environment as the most important issues facing the country. More frequently, the cost of living (56%), health (35%), the economy (33%), and immigration (31%) are seen as pressing issues. Climate change ranks joint fifth, on par with crime and housing, and has seen a decline in perceived importance since previous survey waves. Concern about the climate is somewhat higher among groups that are optimistic about the use of AI in monitoring climate change, namely those from higher socio-economic grades (ABC1) and university graduates (both at 19%), as well as among adults aged over 55 (20%).
Additionally, the share of the public that believe the greatest risk for data use in society is the negative impact that it will have on the environment is much lower (12%) compared with other risks. Risks such as data not being held securely (57%) and data being sold onto organisations for profit (54%) see almost quintuple the levels of concern. Levels of concern for how data will impact the environment are more prominent amongst 18–34-year-olds (16%) and 35-54-year-olds (13%) than those aged 55 or over (7%). We also see higher levels of concern amongst Londoners (17%) compared with all other English regions and Nations, apart for the North-East of England.
In addition to this, 4% of adults see climate change and the environment as top areas for improvements through data use, with health (21%) and the cost of living (14%) perceived as more important areas to focus on. A slightly higher share of those over 55, men, and those in ABC1 socio-economic grades (5% each) recognise the value of using data to address climate change, but these proportions remain modest compared to other issues.
Appreciation for AI’s benefits also extends to areas such as healthcare (52%; a decrease from 57% in Wave 2, but maintained since Wave 3 at 51%), crime prevention (49%; not comparable with Wave 3), education (41%; an increase from 39% in Wave 3), and the quality of public services (40%; a new option this Wave). See Figure 14 and refer to the Case study about public perceptions of healthcare in Chapter 2 for more detail.
9.5 Social Listening: Use of AI in healthcare
Reflecting the optimism about AI applications in healthcare that is notable in the survey findings, analysis of social media posts revealed how AI was often presented as a positive disruptor, “revolutionising” and “transforming” healthcare. Social media posts cited AI in a range of healthcare applications, including the early detection of Parkinson’s Disease, and predicting the risk of heart attack. There was also a frequent focus on the potential for AI to be used to detect cancer, with some citing hospital trials of “cutting edge” AI to detect prostate cancer, as well as GPs’ use of AI technology in England to “boost” cancer detection.
Using breakthrough AI in Heart MRI analysis has potential to revolutionise NHS care.
UK hospitals trial the use of cutting-edge AI in detecting prostate cancer.
Posts by news media accounts cited the application of AI technologies in various healthcare contexts, with some noting their potential to “ease pressure on the NHS”. However, alongside this was coverage of several NHS data breaches, for example, in London and in Scotland. This provides some context to the survey findings which revealed optimism towards the use of data and AI in healthcare, alongside a decline in the public’s confidence in the NHS’s handling of data.
Alarming extent of London Hospital cyberhack uncovered as names, medical conditions and test results of over 100,000 patients included in online data dump.
Hackers publish patients’ confidential NHS data after cyberattack.
Hacking group claims to possess three terabytes of NHS Scotland data.
Turning to look at levels of concern, two in five (40%) adults foresee a negative impact of AI on job opportunities for themselves and their families, a sentiment more common among individuals who do not use chatbots for work (48%) and residents of Northern Ireland (46%). Further, 44% of the public predict that AI will have a negative impact on the trustworthiness of the news and information published online. These concerns are particularly prevalent among those with no (50%) or little (48%) knowledge about how data is used to train AI systems.
Regression analysis shows that females, older people, those living in Yorkshire & Humberside, and non-graduates are less likely to view AI’s impact on job opportunities as positive relative to males, younger people, those living in London, and graduates (Annex 1g). This is similar in the case of the trustworthiness of online news and information, with the addition of Scotland, Wales, and the South-West (as well as Yorkshire & Humberside) as areas where individuals are significantly less likely to view AI’s impact as positive, relative to Londoners (Annex 1h).
The growing prevalence of deepfakes and other AI-produced video content that create convincing impersonations, particularly amplified during the 2024 election campaigns and circulated on social media, could be fuelling this increased concern. Refer to the Social Listening box below for more details on the online conversations about the impact of AI on the job market.
9.6 Social Listening: Impact of AI on job opportunities
In line with the survey’s findings revealing the public’s concern about the role of AI in job displacement, news media social media posts were often driven by the publication of research reports. These were frequently drawn on to speculate on the headline number of job losses that will be caused by AI, as well as the kinds of jobs that are “most at risk”. This was contrasted with fewer posts that were more reassuring in tone, with some suggesting AI would allow companies to “recruit more staff.”
8 million jobs In Britain could be replaced with AI – here are the roles that are most at risk.
Bosses are concerned too! Chief execs worry that AI could take their jobs.
More than a third of companies say that AI will let them recruit more staff.
Similarly, when analysing social media posts more broadly, there was clear concern that UK jobs will be “replaced with AI,” yet there were also counter narratives proposing that AI could “enhance” jobs, boost productivity, and could lead to job creation. These counter-narrative posts often cited research to support their claims, whereas posts about job losses often appeared to be more anecdotal.
No part of these plans will embolden businesses to take on more staff and lots will be looking to replace with AI where possible.
According to research around two thirds of jobs in Britan could be “enhanced” by AI.
Figure 14: Opinion on the impact of AI on the following situations (Showing % selected each option)
Q25b. To what extent do you think the use of Artificial Intelligence will have a positive or negative impact for the following types of situations? Base: All respondents: July/August 2024 (Wave 4), n=4,947
9.7 Perceptions of use of AI in delivery of public services
When it comes to AI being used in the delivery of public services such as in schools, hospitals, or courts, over half (53%) of the public think AI is used at least sometimes, with 6% saying it is used always. About a quarter (24%) think such use of AI is hardly ever or never the case, and less than a quarter aren’t sure (23%). This suggests that there is still some uncertainty and lack of awareness about AI’s role in public services.
Notably, 18–34-year-olds are more likely to have an opinion on the use of AI in this context, with only 12% saying they “don’t know,” while a third (34%) of those aged 55+ are unsure. Similarly, digital engagement plays a role: those with higher digital engagement are more likely to say AI is used always or sometimes (58%) and less likely to say they “don’t know” (18%) compared to those with low levels of digital engagement who say they “don’t know” (38%).
9.8 Areas for AI regulation
When prompted, the public highlights the need for careful management of AI to prevent negative outcomes in three primary areas: healthcare (29%), the military (26%), and banks and finance (25%). At least a quarter of the sample selected each of these areas as their top three options, which is similar to Wave 3, indicating stable views over time (see Figure 15).
However, it is important to note that people’s views on the need for regulating AI use in selected areas, namely healthcare, the police, and social care, have decreased since Wave 2. This may suggest that public confidence in AI governance within selected public services is gradually increasing. In contrast, the public calls for more regulation of AI in other critical sectors, where public trust may be still lacking, such as banking and finance, self-driving cars, and welfare benefits.
Figure 15: Top seven areas governments need to carefully manage to ensure AI does not lead to a negative outcome for users over time (Showing % ranked in top three selections, showing top seven most often selected areas in Wave 4)
Q32. Top three box: Which of the following areas do you think is important that governments carefully manage to make sure the use of AI does not lead to negative outcomes for users? Base: All respondents: June/July 2022 (Wave 2), n = 4,320, August 2023 (Wave 3), n = 4,225, July/August 2024 (Wave 4), n=4,947
10. Insights from the digitally disengaged population
Alongside the nationally representative online sample, research was also conducted using telephone (CATI) interviewing among members of the UK population who have very low levels of digital engagement. This digitally disengaged population exhibits lower trust in organisations compared with the online population, however trust in the NHS remains high, in line with previous survey waves and mirrors the views of the online population. Concerns about data security and management are prevalent among those digitally disengaged, with declining trust in most organisations’ ability to keep data safe, particularly social media and big technology companies. While the digitally disengaged acknowledge AI’s potential societal benefits, they are less optimistic about its personal impacts, with a significant portion expecting it to have negative effects. Key concerns about data include unauthorised data sales, insufficient security measures, and the public’s limited agency over their own data.
10.1 Trust in organisations overall and to handle their data
The digitally disengaged population show lower levels of trust across all organisations than the online population but to varying degrees. As with the wider public, the NHS is the most trusted organisation, being trusted by four in five (80%) of those digitally disengaged in Wave 4. Utilities providers are the third most trusted organisation (52%), a notable difference from the online population where they rank seventh, indicating a difference in the hierarchy of trust in actors between the two groups. Large differences in levels of trust exist between those digitally disengaged and the online population, particularly for academic researchers (76% online vs. 42% digitally disengaged) and pharmaceutical researchers (68% online vs. 38% digitally disengaged). Trust in social media is especially low among the digitally disengaged at 6%.
Just like the online sample, digitally disengaged respondents were asked about their trust in various organisations to use their data to benefit society, keep it safe, and allow them to make decisions about its use. Their trust levels in data actions generally align with overall trust in the organisations. The NHS is most trusted to use data to benefit society (71%), keep data safe (65%), and let respondents make decisions about data use (60%). Trust in the NHS to use data to benefit society and let individuals make decisions about how data is used have remained stable over time. Other organisations with relatively high trust in data management include banks and financial institutions, pharmaceutical researchers, and utilities providers, echoing the overall trust shown in these organisations. However, trust in all organisations for all statements tested are lower among the digitally disengaged audience than among the online population.
Social media companies engender very low levels of trust in using data to benefit society (11%), keep data about you safe (9%) and let you make decisions about how your data is used (9%), with big technology companies also faring poorly (22%, 22%, and 19% respectively). Trust in the government is low and considerably lower than among the online population for using your data to benefit society (33% digitally disengaged vs 46% online), keep data about you safe (25% digitally disengaged vs 48% online), and let you make decisions about how your data is used (29% digitally disengaged vs 41% online).
Figure 16: Trust in actors to manage data in the following ways (Showing % who do trust)
Q14. Do you trust or distrust … to…? BASE: July/August 2024 (Wave 4), social media n=79, The Government n=76, Academic researchers at universities n=77, Social media companies n=89, Big technology companies n=76, Utilities providers n=83, Regulators n=69, Researchers at pharmaceutical companies n=69, HR and recruitment services n=75, Banks and other financial institutions n=97
Most organisations tested have seen declining levels of trust in keeping data safe since Wave 2. The notable exception to this is HR and recruitment firms which have seen their trust to keep data about you safe almost double (46%) since Wave 3 (26%).
10.2 Impact of AI on society and on themselves
Views are mixed regarding the societal impact of AI, with slightly more expecting it to be positive (43%) than negative (34%), and a notable proportion of this audience expecting the impact to be neutral (20%). Optimism about AI’s societal impact is lower than the peak observed in Wave 2 (64%).[footnote 3]
When it comes to the personal impact of AI, negative sentiment prevails: 17% think that the impact will be positive, while half (51%) expect it to be negative. The strength of negativity among a large segment of the digitally disengaged population is evident; one in five (20%) selected ‘0’ on a 0-10 scale, indicating they think AI will have a very negative impact on them personally.
Figure 17: Opinion on the impact AI will have on society (Showing % selected each option)
Q23c_1; Q23c_2. On a scale from 0-10 where 0 = very negative impact and 10 = very positive impact, based on your current knowledge and understanding, what impact do you think Artificial Intelligence (AI) will have overall on society? On a scale from 0-10 where 0 = very negative impact and 10 = very positive impact, based on your current knowledge and understanding, what impact do you think Artificial Intelligence (AI) will have overall on you personally? All telephone respondents aware of AI, BASE: July/August 2024 (Wave 4), n=77
10.3 Risks associated with data
Concerns about data risks are prevalent among this population, with around nine in ten expressing concern that data will be sold for profit (93%), data will not be held securely (92%), important decisions will be made by computers without human input (91%), individuals will not have enough choice about when their data is shared (89%), and some people in society will not be able to access services (88%). Lower down the hierarchy of perceived risks is the negative impact of data processing on the environment, but this is still identified as a risk by 70% of digitally disengaged adults.
The digitally disengaged are more likely to identify each statement as a risk than the online population, often to a very large extent. However, the top two concerns are the same for both audiences, with data being sold for profit (93% digitally disengaged vs 57% online) and data not being held securely (92% digitally disengaged vs 54% online) being the most prevalent perceived risks, indicating these concerns are consistent across the UK population.
11. Methodology
The Responsible Technology Adoption Unit (RTA)’s Public Attitudes to Data and AI Tracker Survey monitors public attitudes towards data and AI over time. This report summarises the fourth Wave (Wave 4) of research and makes comparisons with the first, second, and third Wave (Wave 1, Wave 2, and Wave 3).
The research uses a mixed-mode data collection approach comprising online interviews (Computer Assisted Web Interviews - CAWI) and a smaller telephone survey (Computer Assisted Telephone Interviews - CATI) to ensure that those low or no digital skills are represented in the data.
The Wave 1 online survey (CAWI) ran among UK the general adult population (18+) adults from 29 November 2021 to 20 December 2021 with a total of 4250 interviews collected in that time frame. A further 200 telephone interviews (CATI) were conducted between 15 December 2021 and 14 January 2022.
The Wave 2 online survey (CAWI) ran among UK the general adult population (18+) adults from 27 June 2022 to 18 July 2022 with a total of 4320 interviews collected in that time frame. A further 200 telephone interviews (CATI) were conducted between 1 and 20 July 2022.
The Wave 3 online survey (CAWI) ran among a demographically representative sample of 4225 UK adults (18+). This survey ran from 11 to 23 August 2023. A further 209 UK adults were interviewed via telephone (CATI) between 15 August and 7 September 2023.
For Wave 4, Savanta completed a total of 4947 online Interviews (CAWI) across a demographically representative sample of UK adults (18+); the sample included boosts for each of the three Devolved Nations. This survey ran from 25 July to 16 August 2024. A further 200 UK adults were interviewed via telephone (CATI) between 15 July and 9 August 2024.
Please note that there was a six-month interval between Wave 1 and Wave 2, but a 12-month interval between Wave 2 and Wave 3, and between Wave 3 and Wave 4. Therefore, this report mainly concentrates on the differences between the data from Wave 3 and Wave 4. However, as some Wave 1 and Wave 2 statements were introduced for Wave 4, we do draw comparisons in some cases.
We welcome any further feedback or questions on our approach at public-attitudes@dsit.gov.uk.
12. Sampling and weighting
12.1 Representative Online (CAWI) Sample
Quotas have been applied to the online sample to ensure that it is representative of the UK adult population, based on age, gender, socio-economic grade (CAWI sample only), ethnicity, and region. In addition, interlocked quotas on age and ethnicity were used during fieldwork to monitor the spread of age across these two categories and ensure a balanced final sample. The online sample was provided by Cint. All the contact data provided is EU General Data Protection Regulation (GDPR) compliant.
The online sample was weighted based on official statistics concerning age, gender, ethnicity, region, and socio-economic grade in the UK to correct any imbalances between the survey sample and the population to ensure it is nationally representative. Random Iterative Method (RIM) weighting was used to ensure that the final weighted sample matches the actual population profile.
Where possible, the most up to date ONS UK population estimates have been used for the fieldwork quotas and weighting scheme to ensure a nationally representative sample. 2022 mid-year population estimates were used for age, gender, and region, and the 2021 Census data for socio-economic groups (SEG). For ethnicity, we combined information from the 2021 Census data available for England, Wales, and Northern Ireland and the 2022 Census for Scotland.
The online sample weighting used in Wave 4 was updated to reflect the most up to date population estimates and therefore differs slightly from the weighting scheme used in Wave 1, Wave 2, and Wave 3. Very low digital familiarity (CATI) Sample
For Wave 4, 200 respondents with very low digital familiarity were contacted and interviewed via telephone survey (CATI). The named sample list of respondents’ contact details was provided by TeamSearch. All the contact data provided is GDPR compliant.
This telephone sample captures the views of those who have low to no digital skills and are, therefore, likely to be excluded from online surveys (CAWI). They are likely to be affected by digital issues in different ways to other groups. As the answers respondents give to questions may be impacted by how the question was delivered (e.g. by whether they saw it on a screen, or had it read to them over the phone), any comparisons drawn between the CATI and CAWI samples should be treated with caution.
To select those with very low digital familiarity, we asked the below screening question in the Wave 4 telephone survey questionnaire. Respondents needed to agree to the statements or say they do not do the activities for three out of the five statements asked to qualify for the telephone interview.
Statements
-
I don’t tend to use email
-
I don’t feel comfortable doing tasks such as online banking
-
I feel more comfortable shopping in person than online
-
I find using online devices such as smartphones difficult
-
I usually get help from family and friends when it comes to using the internet
The sample of those with very low digital familiarity was set fieldwork quotas and weighted to be representative of the digitally excluded population captured in FCA Financial Lives 2022 survey. The FCA Financial Lives 2022 survey was chosen as the basis to weight data rather than other similar datasets, including ONS Data and Lloyds Digital Skills 2022 report, as the FCA Financial Lives survey includes ethnicity breakdowns in data tables. We are aware that this group is slightly skewed towards ethnic minority (excluding White minority) adults, hence, the inclusion of ethnicity breakdown was of great importance.
The quotas used were set on broader bands within key categories of gender, age, ethnicity, employment, UK nations, and regions of England. Random Iterative Method (RIM) weighting was used for this study, such that the final weighted sample matches the actual population profile. Those respondents who prefer not to answer questions on age and ethnicity are weighted to 1.0, and those who do not identify as male or female are also weighted to 1.0.
This weighting of the very low digital familiarity (CATI) sample was used for the first time in Wave 2, Wave 1 data has not been weighted. Data from Wave 1 should, therefore, not be compared with other Waves.
For Wave 4, age bands were slightly adjusted in the weighting scheme. While the weighting scheme used in Wave 2 and 3 grouped respondents into 18-64 and 65+, this weighting scheme would have resulted in an inefficient weighting for Wave 4, due to a higher number of respondents aged 75, (NB: this is expected of the digitally disengaged population to skew into a higher age bracket).
To resolve this, we explored a new weighting scheme that adjusted the age groups to 18-74 and 75+, which improved the weighting efficiency significantly and provided balanced weight factors. Our findings indicate that while the figures differ slightly between the two schemes, the significant differences remain consistent. This adjustment ensures that our research methodology remains robust and reflective of the UK adult population.
12.2 Demographic Profile of the Online (CAWI) sample & very low digital engagement (CATI) sample
The demographic profile of the online and very low digital engagement (CATI) samples, before and after the weights have been applied, are provided in the table below.
Online (CAWI) sample
Unweighted | Unweighted | Weighted | Weighted | ||
— | Sample Size | Total Sample (%) | Sample Size | Total Sample (%) | |
Gender | |||||
---|---|---|---|---|---|
Female | 2628 | 53% | 2549 | 52% | |
Male | 2299 | 47% | 2378 | 48% | |
Identify in another way | 15 | <1% | 15 | <1% | |
Prefer not to say | 5 | <1% | 5 | <1% | |
Age | |||||
NET: 18-34 | 1382 | 28% | 1364 | 28% | |
NET: 35-54 | 1664 | 34% | 1622 | 33% | |
NET: 55+ | 1901 | 38% | 1961 | 40% | |
Socio-economic classification | |||||
ABC1 | 2809 | 57% | 2746 | 56% | |
C2DE | 2138 | 43% | 2201 | 45% | |
Region | |||||
Northern Ireland | 530 | 11% | 137 | 3% | |
Scotland | 511 | 10% | 409 | 8% | |
North-West | 468 | 10% | 547 | 11% | |
North-East | 201 | 4% | 199 | 4% | |
Yorkshire & Humberside | 338 | 7% | 404 | 8% | |
Wales | 501 | 10% | 231 | 5% | |
West Midlands | 379 | 8% | 435 | 9% | |
East Midlands | 332 | 7% | 361 | 7% | |
South-West | 321 | 7% | 427 | 9% | |
South-East | 564 | 11% | 681 | 14% | |
Eastern | 311 | 6% | 460 | 9% | |
London | 491 | 10% | 656 | 13% | |
NET England | 3405 | 69% | 4170 | 84% | |
Ethnicity | |||||
NET: White | 4215 | 85% | 4206 | 85% | |
NET: Mixed | 99 | 2% | 81 | 2% | |
NET: Asian | 356 | 7% | 371 | 8% | |
NET: Black | 189 | 4% | 156 | 3% | |
NET: Other | 44 | 1% | 88 | 2% | |
NET: Ethnic minority (excl. White minority) | 688 | 14% | 697 | 14% |
Telephone (CATI) sample
Unweighted | Unweighted | Weighted | Weighted | ||
— | Sample Size | Total Sample (%) | Sample Size | Total Sample (%) | |
Gender | |||||
---|---|---|---|---|---|
Female | 113 | 57% | 98 | 49% | |
Male | 84 | 42% | 99 | 50% | |
Age | |||||
NET: 18-74 | 92 | 48% | 103 | 54% | |
NET: 75+ | 100 | 52% | 89 | 46% | |
Region | |||||
NET England | 163 | 82% | 60 | 80% | |
NET: Scotland, Wales, Northern Ireland | 37 | 19% | 40 | 20% | |
Ethnicity | |||||
NET: White | 180 | 90% | 175 | 87% | |
NET: Ethnic minority (excl. White minority) | 20 | 10% | 25 | 13% |
12.3 Wave on Wave comparability
The same questions were asked in Waves 1, 2, 3, and 4 of the tracker survey to enable comparison between the four time points. For Wave 4, we have replaced some questions from previous Waves and added or removed some answer options. In future Waves we will rotate different items and questions into the survey at different intervals as annual data points are not required for all. Additionally, the question wording has been updated in some instances which are clearly marked in data tables with variable names marked ‘b’.
The following notable edits were applied to CAWI and CATI questionnaires in Wave 4:
CAWI
-
Q12, Answer options: “When organisations collect my data, I am made aware of how they are going to use it” was added.
-
Q39, Answer options: “AI will collect and use personal data without consent” and “The energy demands of using AI will have a negative impact on the environment” were added.
-
Q25b, Answer options: “Supporting the police with the prevention and detection of crime ”, “Monitoring climate change (e.g. by analysing carbon emissions, or monitoring deforestation)”, “The trustworthiness of news and information published online” were added.
-
Q32, Answer options: “AI used in the tax system (e.g. ensuring everyone pays the right tax)” was added.
-
Q33b, Answer options: “The tax system” were added.
-
Q23c was updated from Q23b last year to include AI’s personal impact as well as societal.
-
New questions Q23c, Q40, Q41, Q42, Q43 were added.
CATI
-
New questions Q17d was added.
-
Q23c was updated from Q23b last year to include AI’s personal impact as well as societal.
-
Q25b, Answer options: “Monitoring climate change (e.g. by analysing carbon emissions, or monitoring deforestation)”, “The trustworthiness of news and information published online”, “The quality of public services (e.g. schools, hospitals, courts)” were added.
13. Analysis
The data from the CAWI survey has been analysed using a combination of descriptive, conjoint and regression analysis, and social listening. The CATI data has been analysed using descriptive analysis only, due to its relatively smaller sample size (the conjoint module was not included in the CATI survey).
13.1 Statistical significance and interpretation
When interpreting the figures in this report, please note that only statistically significant differences (at a 95% confidence level) are reported and that the effect of weighting is considered when significance tests are conducted. Significant differences are highlighted in the analytical report and are relative to other directly relevant subgroups (e.g. those identifying as male vs those identifying as female).
13.2 Digital engagement score
A proxy score for digital engagement has been used to break up respondents into three groups, using a score based on self-reported levels of confidence in using technology, and frequency of use of four digital services. The scores have been assigned as follows:
-
Q4b: Respondents are given a score of 3 for each digital service used ‘a lot’, score of 1.5 for used ‘occasionally’, 0 for ‘don’t do at all’, with a maximum of 12 points on this question.
-
Q5: Respondents are given points for every answer; ‘very confident’ = 12 points, ‘somewhat confident’ = 8, ‘not confident’ = 4, ‘not at all confident’ = 0, any other response = 0.
-
The total maximum score one can have from Q4b and Q5 combined is 24.
The distribution of scores was then analysed using Jenks method to identify logical divisions between the groups:
-
Low digital engagement (previously low digital familiarity): 0-12.5 (428 respondents)
-
Medium digital engagement (previously medium digital familiarity): 13-19 (1630 respondents)
-
High digital engagement (previously high digital familiarity): 19.5-24 (2167 respondents)
The CATI survey data has been treated as an extension to this means of dividing the data, providing a ‘digitally disengaged’ (previously ‘very low digital familiarity’) group.
N.B.: In previous Waves, ‘digital engagement’ was labelled as ‘digital familiarity’. This was updated in Wave 4 to reflect active participation in digital activities, rather than familiarity, as people may be familiar but choose not to engage.
13.3 Conjoint Analysis
Conjoint analysis is a survey-based research approach for measuring the value that individuals place on different features and considerations for decision making. It works by asking respondents to directly compare different combinations of features to determine how they value each one.
A conjoint experiment was created to test preferences for four attributes of a scenario where personal data was shared between two organisations. Those attributes were:
-
The organisation sharing the data (Actor 1)
-
The organisation receiving the data (Actor 2)
-
The purpose of the data sharing (Use case)
-
A step taken to reassure people that their data will remain secure even when shared between organisations. (Governance mechanism)
In addition, respondents were split equally between two different conjoint experiments. These experiments were identical apart from a single line in the question text. In the Model A conjoint, respondents were asked which data-sharing scenario they preferred ‘assuming that all the data shared is anonymised (e.g. people using the data cannot match the information to an individual person). In the Model B conjoint, respondents were asked which data-sharing scenario they preferred ‘assuming that all the data shared is identifiable (e.g. using the data can match the information to an individual person).’
Each Model tested pairs of data sharing scenarios (where the items within attributes varied) asking respondents to select which scenario they preferred. Respondents were shown six pairs of scenarios; each scenario being allocated based on achieving as even a distribution of combinations as possible across the sample. Each pair could contain some identical characteristics, but the pairs as a whole could not be identical. In addition, a restriction was put in place that ensured Actor 1 and Actor 2 were different organisations. Even with this methodology not all combinations can feasibly be shown though, this problem is addressed in the analysis phase of the work.
A visual example of the Model A conjoint experiment setting (comparing two data sharing scenarios out of six pairs in total) in the online survey is as follows:
The data captured was analysed in Sawtooth using a combination of logistic regression and a hierarchical Bayesian (HB) algorithm. An individual respondent’s data was regressed to create utility scores; these scores can be the appeal of an attribute within the data sharing scenarios. These utility scores were then used to determine the likelihood of a respondent selecting an attribute or combination of attributes (the propositions displayed in the exercise).
The HB algorithm analysed an individual respondent’s utilities for the data sharing scenarios they were shown, compared them to the sample average and then estimated their likely choices for scenarios not shown based on their variation from the sample average.
The utility scores were transformed to show the likelihood that an AI usage scenario was selected, with a probability of 50% being the base probability, 100% being chosen every time, and 0% being never selected.
For further reading on HB analysis please refer to the Sawtooth website.
13.4 Regression analysis
Regression analysis is used to test associations between different characteristics and responses, for example, to test for associations between demographic characteristics and attitudes toward data. This technique can identify the size and strength of these relationships, while holding all other variables in the model equal, but not cause and effect.
Logistic regression is used to test for associations between a single ‘dependent’ variable and multiple ‘independent’ variables. Logistic regression is used as many of the ‘dependent’ variables in this report are survey questions based on Likert scales and not continuous data. Therefore, the data is transformed into a binary variable with two categories, for example ‘agreeing’ with a statement inclusive of ‘strongly agree’ and ‘somewhat agree’, and ‘neutral or not agreeing’ with the statement inclusive of all other responses.
Logistic regression provides us with an ‘odds ratio’ (OR). This tells us the odds of someone with a particular characteristic or attitude reporting, for example, that they agree with a statement, compared with someone with another characteristic or attitude, after taking other possible influences into account. For example, regression analysis ran for this tracker survey shows that those with Black ethnic background are more likely (OR = 2.46, meaning 2.46 times as likely) to think that organisations are held accountable when they misuse data, compared with White respondents (Annex 1c, Q12).
A goodness of fit measure, Akaike Information Criterion (AIC), is reported with all models. This can be used to compare models with the same dependent variable and understand which one is the best fit for the data where a smaller AIC indicates a better fit. AIC penalises models for including more variables, therefore, variables that are not found to be statistically significant are removed from the final models iteratively.
We tested selected hypotheses using interactive effects on the type of data mentioned in the question and the individual’s demographic characteristics. Interaction effects occur in complex study areas when an independent variable interacts with another independent variable and its relationship with a dependent variable changes as a result. This effect on the dependent variable is non-additive, i.e., the joint effect of two variables interacting is significantly greater or significantly less than the sum of the parts. It is important to understand whether this effect occurs because it tells us how two or more independent variables work together to impact the dependent variable and ensure our interpretation of data is correct. The presence or absence of interactions among independent variables can be revealed with an interaction plot and interaction term effect can be included in an analytic model in order to quantify its significance. When we have statistically significant interaction effects, we have to interpret the main effects considering the interactions.
13.5 Social media analysis methodology (“social listening”)
To complement Wave 4 of this survey, qualitative social media analysis was conducted to explore the ways in which people are talking about data and AI on the social media platform, X (formerly Twitter). Two datasets of posts were collected from X using the social media analytics platform, Sprout Social. To collect the data, two social media ‘listening topics’ were created: one to collect posts about data and AI from a subset of the most followed news media accounts on X (referred to as the ‘news media’ topic), and one to collect posts about AI from across X (referred to as the ‘broad’ topic).
The listening topics were designed using Sprout Social’s user interface, using keywords to ensure that the posts collected mentioned AI and/or data and that they were likely to originate from the United Kingdom. The news media listening topic collected posts about data and AI posted between 15th January and 16th August 2024. The broad listening topic collected posts about AI from across X between 15th June and 16th August 2024.
This resulted in two datasets of 3,539 posts (news media) and 9,121 posts (broad topic).
The full news media dataset and a random sample of one third of the broad topic dataset (3,040 posts) was analysed qualitatively, key themes were identified and using analysis software Nvivo, the posts were allocated to the themes. There were twenty-eight themes identified in the broad topic dataset and twenty-one themes identified in the news media dataset. Once the data had been allocated to themes, the most relevant themes in relation to the survey findings were summarised and included in the report.
Further information on the sources, software, techniques, and processes used to collect and analyse the X data can be found in the annex.
14. Annex 1: Regression models
14.1 Annex 1a (Q40): How often do you think government departments share data with each other about specific individuals?
Characteristic | Odds ratio | 95% Confidence level | p-value | |
— | — | — | — | |
Age (decade) | 1.10 | 1.05, 1.15 | <0.001 | |
---|---|---|---|---|
When organisations collect my data, I am made aware of how they are going to use it | ||||
Neutral / disagree | – | – | ||
Agree | 1.23 | 1.03, 1.47 | 0.024 | |
Trust in government | ||||
Trust | – | – | ||
Do not trust | 1.20 | 1.00, 1.43 | 0.047 | |
AIC | 3,492 |
14.2 Annex 1b (Q12): “I have control over who uses my data and how”
Characteristic | Odds ratio | 95% Confidence level | p-value | |
— | — | — | — | |
Age (decade) | 0.89 | 0.86, 0.92 | <0.001 | |
---|---|---|---|---|
Regions | ||||
London | – | – | ||
East Midlands | 0.97 | 0.72, 1.30 | 0.8 | |
Eastern | 0.90 | 0.66, 1.23 | 0.5 | |
North-East | 1.00 | 0.71, 1.41 | >0.9 | |
North-West | 0.83 | 0.63, 1.09 | 0.2 | |
Northern Ireland | 0.76 | 0.58, 1.00 | 0.047 | |
Scotland | 1.02 | 0.78, 1.33 | 0.9 | |
South-East | 0.88 | 0.67, 1.14 | 0.3 | |
South-West | 1.12 | 0.82, 1.51 | 0.5 | |
Wales | 0.90 | 0.69, 1.18 | 0.5 | |
West Midlands | 1.16 | 0.88, 1.54 | 0.3 | |
Yorkshire & Humberside | 0.87 | 0.65, 1.17 | 0.4 | |
Socioeconomic grade | ||||
ABC1 | – | – | ||
C2DE | 1.14 | 1.01, 1.28 | 0.038 | |
Ethnicity | ||||
White | – | – | ||
Asian | 1.38 | 1.09, 1.74 | 0.007 | |
Black | 1.85 | 1.36, 2.52 | <0.001 | |
Mixed | 1.44 | 0.96, 2.16 | 0.079 | |
Other | 0.79 | 0.39, 1.51 | 0.5 | |
Prefer not to say | 0.91 | 0.47, 1.72 | 0.8 | |
AIC | 6,257 |
14.3 Annex 1c (Q12): “When organisations misuse data, they are held accountable”
Characteristic | Odds ratio | 95% Confidence level | p-value | |
— | — | — | — | |
Age (decade) | 0.92 | 0.89, 0.95 | <0.001 | |
---|---|---|---|---|
Socioeconomic grade | ||||
ABC1 | – | – | ||
C2DE | 1.22 | 1.09, 1.38 | <0.001 | |
Ethnicity | ||||
White | – | – | ||
Asian | 1.68 | 1.33, 2.11 | <0.001 | |
Black | 2.46 | 1.79, 3.39 | <0.001 | |
Mixed | 1.82 | 1.21, 2.75 | 0.004 | |
Other | 1.52 | 0.82, 2.82 | 0.2 | |
Prefer not to say | 0.82 | 0.43, 1.53 | 0.5 | |
AIC | 6,331 |
14.4 Annex 1d (Q42): How much did you know about how data is used to train AI systems?
Characteristic | Odds ratio | 95% Confidence level | p-value | |
— | — | — | — | |
Gender | ||||
---|---|---|---|---|
Male | – | – | ||
Female | 0.54 | 0.46, 0.63 | <0.001 | |
I identify in another way | 1.30 | 0.39, 4.17 | 0.7 | |
Prefer not to say | 3.50 | 0.30, 80.4 | 0.3 | |
Age (decade) | 0.70 | 0.67, 0.74 | <0.001 | |
Regions | ||||
London | – | – | ||
East Midlands | 0.90 | 0.63, 1.29 | 0.6 | |
Eastern | 0.70 | 0.47, 1.05 | 0.091 | |
North-East | 0.88 | 0.57, 1.35 | 0.6 | |
North-West | 0.84 | 0.61, 1.18 | 0.3 | |
Northern Ireland | 0.87 | 0.63, 1.19 | 0.4 | |
Scotland | 0.62 | 0.44, 0.87 | 0.006 | |
South-East | 0.74 | 0.54, 1.02 | 0.069 | |
South-West | 0.84 | 0.57, 1.24 | 0.4 | |
Wales | 0.74 | 0.53, 1.03 | 0.077 | |
West Midlands | 0.92 | 0.65, 1.30 | 0.6 | |
Yorkshire & Humberside | 0.69 | 0.47, 1.00 | 0.050 | |
Education level | ||||
Graduate | – | – | ||
Non-graduate | 0.64 | 0.55, 0.75 | <0.001 | |
Socioeconomic grade | ||||
ABC1 | – | – | ||
C2DE | 0.80 | 0.68, 0.94 | 0.008 | |
Ethnicity | ||||
White | – | – | ||
Asian | 1.30 | 0.99, 1.70 | 0.061 | |
Black | 1.59 | 1.12, 2.28 | 0.010 | |
Mixed | 1.68 | 1.05, 2.69 | 0.030 | |
Other | 1.15 | 0.52, 2.51 | 0.7 | |
Prefer not to say | 1.76 | 0.80, 3.87 | 0.2 | |
Self-reported ability to explain AI | ||||
Can explain AI | – | – | ||
Cannot explain AI | 0.49 | 0.40, 0.60 | <0.001 | |
When organisations collect my data, I am made aware of how they are going to use it | ||||
Neutral / disagree | – | – | ||
Agree | 1.58 | 1.36, 1.85 | <0.001 | |
Personal use of chatbots | ||||
At least monthly | – | – | ||
Less than monthly | 0.29 | 0.25, 0.34 | <0.001 | |
AIC | 4,172 |
14.5 Annex 1e (Q23c): On a scale of 0-10, what impact do you think AI will have on…?
Impact on society
Characteristic | Odds ratio | 95% Confidence level | p-value | |
— | — | — | — | |
Gender | ||||
---|---|---|---|---|
Male | – | – | ||
Female | 0.60 | 0.53, 0.69 | <0.001 | |
I identify in another way | 0.32 | 0.09, 1.02 | 0.057 | |
Prefer not to say | 1.34 | 0.12, 29.5 | 0.8 | |
Age (decade) | 0.90 | 0.87, 0.94 | <0.001 | |
Regions | ||||
London | – | – | ||
East Midlands | 0.80 | 0.56, 1.13 | 0.2 | |
Eastern | 0.67 | 0.47, 0.95 | 0.027 | |
North-East | 1.05 | 0.69, 1.60 | 0.8 | |
North-West | 0.68 | 0.50, 0.93 | 0.015 | |
Northern Ireland | 0.56 | 0.41, 0.75 | <0.001 | |
Scotland | 0.52 | 0.38, 0.71 | <0.001 | |
South-East | 0.71 | 0.52, 0.96 | 0.026 | |
South-West | 0.64 | 0.45, 0.91 | 0.013 | |
Wales | 0.62 | 0.46, 0.85 | 0.003 | |
West Midlands | 0.85 | 0.61, 1.19 | 0.4 | |
Yorkshire & Humberside | 0.62 | 0.44, 0.88 | 0.007 | |
Education level | ||||
Graduate | – | – | ||
Non-graduate | 0.72 | 0.62, 0.83 | <0.001 | |
Socioeconomic grade | ||||
ABC1 | – | – | ||
C2DE | 0.82 | 0.71, 0.95 | 0.008 | |
Ethnicity | ||||
White | – | – | ||
Asian | 1.52 | 1.14, 2.02 | 0.005 | |
Black | 1.87 | 1.28, 2.79 | 0.002 | |
Mixed | 1.02 | 0.65, 1.64 | >0.9 | |
Other | 1.41 | 0.69, 3.09 | 0.4 | |
Prefer not to say | 1.12 | 0.50, 2.63 | 0.8 | |
Self-reported ability to explain AI | ||||
Can explain AI | – | – | ||
Cannot explain AI | 0.58 | 0.49, 0.67 | <0.001 | |
AIC | 4,899 |
14.6 Annex 1f (Q23c): On a scale of 0-10, what impact do you think AI will have on…?
Impact on you personally
Characteristic | Odds ratio | 95% Confidence level | p-value | |
— | — | — | — | |
Gender | ||||
---|---|---|---|---|
Male | – | – | ||
Female | 0.69 | 0.60, 0.80 | <0.001 | |
I identify in another way | 0.44 | 0.13, 1.44 | 0.2 | |
Prefer not to say | 1.17 | 0.11, 25.9 | >0.9 | |
Age (decade) | 0.84 | 0.80, 0.88 | <0.001 | |
Regions | ||||
London | – | – | ||
East Midlands | 0.66 | 0.46, 0.96 | 0.030 | |
Eastern | 0.56 | 0.38, 0.82 | 0.003 | |
North-East | 1.01 | 0.65, 1.57 | >0.9 | |
North-West | 0.76 | 0.54, 1.06 | 0.11 | |
Northern Ireland | 0.59 | 0.42, 0.81 | 0.001 | |
Scotland | 0.51 | 0.37, 0.72 | <0.001 | |
South-East | 0.74 | 0.53, 1.02 | 0.063 | |
South-West | 0.65 | 0.44, 0.97 | 0.033 | |
Wales | 0.59 | 0.42, 0.82 | 0.002 | |
West Midlands | 0.82 | 0.57, 1.16 | 0.3 | |
Yorkshire & Humberside | 0.61 | 0.42, 0.88 | 0.009 | |
Education level | ||||
Graduate | – | – | ||
Non-graduate | 0.65 | 0.56, 0.75 | <0.001 | |
Ethnicity | ||||
White | – | – | ||
Asian | 1.89 | 1.38, 2.61 | <0.001 | |
Black | 1.80 | 1.20, 2.78 | 0.006 | |
Mixed | 1.26 | 0.77, 2.15 | 0.4 | |
Other | 1.15 | 0.55, 2.54 | 0.7 | |
Prefer not to say | 0.73 | 0.32, 1.70 | 0.4 | |
Self-reported ability to explain AI | ||||
Can explain AI | – | – | ||
Cannot explain AI | 0.50 | 0.42, 0.58 | <0.001 | |
AIC | 4,278 |
14.7 Annex 1g (Q25b): To what extent do you think the use of AI will have a positive or negative impact on the following types of situations?
Job opportunities for people like you and your family
Characteristic | Odds ratio | 95% Confidence level | p-value | |
— | — | — | — | |
Gender | ||||
---|---|---|---|---|
Male | – | – | ||
Female | 0.71 | 0.62, 0.82 | <0.001 | |
I identify in another way | 0.16 | 0.01, 0.81 | 0.076 | |
Prefer not to say | 1.08 | 0.05, 11.9 | >0.9 | |
Age (decade) | 0.81 | 0.77, 0.84 | <0.001 | |
Regions | ||||
London | – | – | ||
East Midlands | 0.84 | 0.60, 1.17 | 0.3 | |
Eastern | 0.71 | 0.49, 1.02 | 0.064 | |
North-East | 1.01 | 0.69, 1.48 | >0.9 | |
North-West | 0.96 | 0.71, 1.29 | 0.8 | |
Northern Ireland | 0.76 | 0.56, 1.02 | 0.067 | |
Scotland | 0.78 | 0.58, 1.05 | 0.10 | |
South-East | 0.76 | 0.56, 1.02 | 0.066 | |
South-West | 0.73 | 0.51, 1.04 | 0.085 | |
Wales | 0.87 | 0.64, 1.18 | 0.4 | |
West Midlands | 1.09 | 0.80, 1.47 | 0.6 | |
Yorkshire & Humberside | 0.68 | 0.48, 0.95 | 0.027 | |
Education level | ||||
Graduate | – | – | ||
Non-graduate | 0.81 | 0.70, 0.93 | 0.003 | |
Ethnicity | ||||
White | – | – | ||
Asian | 1.31 | 1.01, 1.68 | 0.037 | |
Black | 1.19 | 0.85, 1.64 | 0.03 | |
Mixed | 1.40 | 0.90, 2.15 | 0.13 | |
Other | 1.60 | 0.82, 3.05 | 0.2 | |
Prefer not to say | 1.14 | 0.52, 2.34 | 0.7 | |
AIC | 5,026 |
14.8 Annex 1h (Q25b): To what extent do you think the use of AI will have a positive or negative impact on the following types of situations?
The trustworthiness of news and information published online
Characteristic | Odds ratio | 95% Confidence level | p-value | |
— | — | — | — | |
Gender | ||||
---|---|---|---|---|
Male | – | – | ||
Female | 0.68 | 0.59, 0.79 | <0.001 | |
I identify in another way | 0.50 | 0.11,1.62 | 0.3 | |
Prefer not to say | 1.02 | 0.05, 11.5 | >0.9 | |
Age (decade) | 0.77 | 0.74, 0.81 | <0.001 | |
Regions | ||||
London | – | – | ||
East Midlands | 0.81 | 0.58, 1.14 | 0.2 | |
Eastern | 0.78 | 0.54, 1.12 | 0.2 | |
North-East | 1.11 | 0.75, 1.63 | 0.6 | |
North-West | 0.98 | 0.73, 1.33 | >0.9 | |
Northern Ireland | 0.74 | 0.55, 1.00 | 0.053 | |
Scotland | 0.73 | 0.53, 0.99 | 0.042 | |
South-East | 0.79 | 0.59, 1.06 | 0.12 | |
South-West | 0.66 | 0.45, 0.95 | 0.028 | |
Wales | 0.63 | 0.46, 0.87 | 0.005 | |
West Midlands | 0.89 | 0.65, 1.21 | 0.5 | |
Yorkshire & Humberside | 0.70 | 0.49, 0.98 | 0.038 | |
Education level | ||||
Graduate | – | – | ||
Non-graduate | 0.76 | 0.65, 0.87 | <0.001 | |
Ethnicity | ||||
White | – | – | ||
Asian | 1.28 | 0.99, 1.65 | 0.056 | |
Black | 1.92 | 1.39, 2.65 | <0.001 | |
Mixed | 1.59 | 1.02, 2.45 | 0.040 | |
Other | 1.02 | 0.48, 2.04 | >0.9 | |
Prefer not to say | 1.09 | 0.48, 2.28 | 0.8 | |
AIC | 4,857 |
15. Annex 2: Conjoint Table
Conjoint Model A (anonymised) | Conjoint Model B (identifiable) | ||
— | — | — | |
Significance Level: 95% | a | b | |
Total | 2458 | 2489 | |
Actor 1: Data will be collected by… | |||
---|---|---|---|
NHS services | 70.4 | 69.1 | |
b | |||
Schools | 48.9 | 42.9 | |
b | |||
Job centres | 40.9 | 43.9 | |
a | |||
The police | 46.0 | 47.6 | |
a | |||
Local authorities | 57.3 | 58.6 | |
a | |||
Big technology companies | 39.4 | 37.4 | |
b | |||
Private healthcare providers | 46.1 | 49.5 | |
a | |||
Actor 2: … in order to help … | |||
NHS services | 75.5 | 75.6 | |
Schools | 52.3 | 48.7 | |
b | |||
Job centres | 43.3 | 39.6 | |
b | |||
The police | 47.3 | 47.1 | |
Local authorities | 57.9 | 60.5 | |
a | |||
Big technology companies | 29.3 | 30.6 | |
a | |||
Private healthcare providers | 42.7 | 45.9 | |
a | |||
Use case: … do the following… | |||
Track their progress towards meeting a major objective | 48.3 | 49.8 | |
a | |||
Measure the quality of their work | 47.7 | 47.5 | |
Improve the experiences of people who interact with them | 52.4 | 52.1 | |
Learn more about people living in the UK | 51.7 | 50.6 | |
b | |||
Governance mechanisms: …and steps will be taken to ensure that… | |||
There will be full transparency about who has access to the shared data, why they have it, and who granted that access | 53.1 | 49.7 | |
b | |||
People will only be able to access the shared data via a Trusted Research Environment (TRE). This means people can only work with the shared data online and will be prevented from downloading data to their computers | 48.5 | 50.0 | |
a | |||
People will only have access to the shared data for the time it takes to complete their project | 47.5 | 49.3 | |
a | |||
There will be full transparency about all past, current and future uses of the shared data | 49.6 | 49.5 | |
Members of the public will be able to decide whether certain uses of the shared data are allowed or not | 51.4 | 51.4 | |
Overview | |||
Actor 1 | 34% | 33% | |
Actor 2 | 39% | 39% | |
Use case | 12% | 12% | |
Governance mechanisms | 15% | 15% | |
16. Annex 3: Social media analysis (“social listening”)
16.1 Data sources
DSIT used the social media analytics platform Sprout Social to collect data from X (formerly Twitter). The data was collected prior to and during the period that the CATI and CAWI Public Attitudes to Data and AI survey was in field (15th July – 16th August 2024). This was with the aim of exploring the ways in which data and AI was being talked about on social media. The news media X data was collected from the period between 15th January and 16th August 2024; this was in line with the survey question which asked respondents “have you read, seen, or heard anything about data being used in the last 6 months, for example in news articles, or on TV or radio?” The broader X data was collected from the period between 15th June and 16th August 2024. This included the month prior to the Public Attitudes to Data and AI survey going into field, to ensure the query captured recent content about AI that the public may recall while completing the survey.
As explained in more depth in the next section, the data collected for both datasets was “backfilled” by the Sprout Social platform and was therefore not captured in real time. Only public content that was still available at the time of data collection (for example, posts that had not been deleted from X) was collected. Furthermore, as DSIT do not have full details of how the backfill was conducted, we cannot be certain that the datasets are comprehensive. Nevertheless, they provide an indication of how data and AI are being talked about on social media, specifically X.
16.2 Data specification and query design
Relevant X data was collected using the ‘listening topic’ function on Sprout Social. This function provides a user interface through which to implement a user defined Boolean search query. Each query used combinations of keywords alongside the Boolean operator “AND,” to define relevant posts. It was also possible to exclude words or phrases. The full lists of keywords used in each listening topic query can be provided on request, by emailing public-attitudes@dsit.gov.uk.
16.3 News media query
A search engine was used to source a list of the most followed newspaper X accounts. The top fifty-one active accounts from the list were included in the search query, as well as the X accounts of public service broadcasters (BBC, ITV, ITV Wales, STV, UTV, S4C, Channel 4, and Channel 5), selecting their specific news accounts where possible. A table listing the fifty-nine news media X accounts included in the query can be provided on request, by emailing public-attitudes@dsit.gov.uk.
The listening topic query searched for posts “from” these specific news media accounts that contained any of the following keywords and/or hashtags:
AI / #AI
Artificial Intelligence / #artificialintelligence
Data / #data
Dataset(s) / #dataset(s)
bigdata
Sprout Social backfilled the data to capture posts from up to six months prior to Wave four of the Public Attitudes to Data and AI survey going into field, up until the fieldwork completion date. This was to ensure the social media data aligned with the survey question which asked respondents, “have you read, seen, or heard anything about data being used in the last 6 months, for example in news articles, or on TV or radio?” This allows for comparison between social media posts from news media and public recall of news stories.
16.4 Broader query
The broader listening topic query was designed to collect posts from across X that mentioned the following AI keywords or hashtags:
AI / #AI
Artificial Intelligence / #artificialintelligence
Alongside the above keywords and hashtags, the query used the Boolean operator “AND” function to ensure that the posts collected included one or more of a list of further keywords. These keywords mapped onto the risks and benefits section of the survey, including around jobs, healthcare, education, and other keywords aligned with the broader themes of risks and benefits. Using keywords to narrow down which posts were captured inevitably means there will be conversations on other kinds of benefits and risks of AI taking place on X that were not included in the dataset. However, the aim of the social media analysis was to complement the survey findings around the key topics included in the benefits and risks section of the survey.
The query aimed to ensure, as much as possible, that posts originated from or were relevant to the UK. Although Sprout Social allows users to filter for the country the message or the user posting the message originates from, this relies on the availability of location data for all relevant posts. Rather than counting on this method, the Boolean operator “AND” was used again, this time to ensure the posts collected mentioned the UK or UK nations, regions, and/or cities. Drawing on data from the Centre for Cities, the query included the 15 largest cities in the UK by population, as well as Cardiff, Swansea, and Londonderry to ensure representation from all UK nations.
The query was limited to posts written in English. After launching the listening topic, it was observed that re-posts were creating a lot of noise and repetition in the dataset. In response, the query was edited to exclude re-posts/shares and then backfilled by Sprout Social so that it captured posts from the desired period.
16.5 Data collection and sampling
Using the social media analytics platform Sprout Social, the listening topic search queries produced two datasets. The news media dataset comprised 3,539 posts and the broader dataset comprised 9,121 posts. A random sample of one third of the broader dataset was generated using the random number generator in Excel. Once each post had been assigned a random number in Excel, the posts were ordered by lowest to highest number and the first 3,040 posts (one third of the dataset) were exported for analysis. This resulted in two similar sized datasets that could be qualitatively analysed within the time available.
16.6 Analysis
The full news media dataset and the random sample of the broader dataset were imported into the qualitative analysis software Nvivo. Key themes were identified, with one researcher allocating the posts to themes. Twenty-one themes around data and AI were identified from the news media dataset, with some posts being included in more than one theme. The news media themes included, “Data breach,” “Health” and “Jobs and employment.”
More themes were identified in the broader dataset, reflecting the diversity of topics that the posts relating to AI mentioned. In total, there were twenty-eight themes identified in the broader dataset, with some posts included in more than one theme. There was a lot of cross over between the themes within the news media dataset and the broader dataset, including around the themes of health, jobs and employment, climate, politics, education, misinformation, and privacy. However, there were also some distinct themes identified in the broader dataset including, “bias”, “facial recognition”, and AI and “music”. These themes often contained fewer posts but denote the breadth of discussion about AI taking place on X. A full list of the codes for each dataset, and their descriptions, can be provided on request, by emailing public-attitudes@dsit.gov.uk.
After the posts were allocated to themes, the researcher focused in on the themes that were most relevant to the wave four survey findings. The final themes that were selected for inclusion in the report were around jobs, climate, healthcare, and risks of AI, themes present in both datasets. By reading and re-reading the posts, the researcher summarised the content of each theme and identified key findings within them that pertained to how AI and data were being discussed on X. This was with the aim of adding context to the quantitative survey findings.
When writing up the summaries, posts were included to provide context to the reader and to demonstrate key findings. However, posts were not quoted directly and were instead paraphrased. This was primarily an ethical decision, to protect the anonymity of individual users, and also ensured that the reporting complied with the usage terms of X.
-
The prior name of the Responsible Technology Adoption Unit (RTA) ↩
-
The parameters of ‘positive’, ‘negative’, and ‘neutral’ were slightly adjusted this Wave. Respondents were asked to rate their perception of AI’s impact using a 10-point scale where 10 is a very positive impact and 0 is a very negative impact. In Wave 4, positive is inclusive of those choosing 6-10, negative 0-4, and neutral 5 (vs. previous Waves where positive was inclusive of 8-10, negative 0-3, and neutral 4-7. ↩
-
The parameters of ‘positive’, ‘negative’, and ‘neutral’ were slightly adjusted this Wave. Respondents were asked to rate their perception of AI’s impact using a 10-point scale where 10 is a very positive impact and 0 is a very negative impact. In Wave 4, positive is inclusive of those choosing 6-10, negative 0-4, and neutral 5 (vs. previous Waves where positive was inclusive of 8-10, negative 0-3, and neutral 4-7. ↩