Cyber security risks to artificial intelligence
Published 15 May 2024
Executive summary
The Department for Science, Innovation and Technology commissioned Grant Thornton UK LLP and Manchester Metropolitan University to develop an assessment of the cyber security risks to Artificial Intelligence (AI). The assessment aimed to identify and map vulnerabilities across the AI lifecycle and assess the exploitation and impact of each vulnerability, delineating software vulnerabilities and those specific to AI to help contextualise the findings.
The assessment comprised two literature reviews, evaluating two distinct but complementary research streams: academic literature, and government and industry reports. The findings were also integrated with feedback from cross-sector client and expert interviews. Cross-validation was applied across research publications and a cut-off point of 10th February 2024 was set for publications to ensure that the report was based on the latest analysis considering the ever-changing technological landscape.
The literature reviews identified a series of vulnerabilities, including specific ones to AI across each phase of the AI lifecycle, namely design, development, deployment, and maintenance. The vulnerabilities have been comprehensively mapped across each phase of the AI lifecycle, with an assessment of their exploitation and impact (see Section 4). To offer additional perspective on the potential risks, a set of 23 case studies were identified, both real-world and theoretical proof of concepts, involving cyber-attacks linked to AI vulnerabilities (see Section 5).
Insights gained from interviews with 5 clients, from the Insurance, Banking, and Media domains demonstrated the market readiness and the practical implications of AI vulnerabilities. The findings categorised organisations into two distinct groups: those unaware of AI’s use and consequent cyber security risks within their operations, and those recognising these risks yet lacking internal expertise for risk assessment and management.
The current literature on the cyber security risks to AI, does not contain a single comprehensive evaluation of AI-specific cyber security risks across each stage of the AI lifecycle. This risk assessment has therefore aimed to fill this gap, whilst building on the important contributions made by industry and other governments. This has been delivered by thoroughly evaluating the potential exploitation, impact, and AI-specific cyber security risks associated with each lifecycle phase. Additionally, as noted above, the report mapped cyber-attacks that have originated from vulnerabilities in AI systems.
The report also highlighted that the rapid adoption of AI continues to introduce complex cyber security risks that traditional practices may not sufficiently address. A holistic approach to address the cyber risks across the entire AI lifecycle is essential. By mitigating vulnerabilities at every stage of the AI lifecycle, organisations can bolster robust security measures and fortify resilience against evolving cyber threats.
1. Introduction
Artificial intelligence (AI) has recently witnessed a rapid acceleration in its development and integration across various sectors, profoundly impacting industries, economies, and societies worldwide. This remarkable progress is primarily attributed to significant breakthroughs in machine learning, deep learning, and the massive advancement of computational capabilities.
While AI technologies have advanced and enhanced efficiency and productivity, they remain susceptible to an ever-growing number of security threats and vulnerabilities. The rapid advancement of AI therefore necessitates the need for a robust understanding of the evolving risks that are specifically associated with AI.
The Department for Science, Innovation, and Technology commissioned Grant Thornton UK LLP and Manchester Metropolitan University to develop a comprehensive assessment of the cyber security risks that are specifically associated with AI. This included identifying specific vulnerabilities at each stage of the AI lifecycle, with a delineation between software and those specific to AI and assessing the exploitation and impact of each vulnerability.
Aim and objectives of the assessment
The assessment aimed to develop an understanding of the cyber security risks to AI by identifying specific vulnerabilities at each stage of the AI lifecycle and assessing the exploitation and impact of each vulnerability. To realise its aim, the study pursued the following objectives:
-
Identification and Delineation of AI-Specific Vulnerabilities: This report sought to clearly outline vulnerabilities specific to AI, and those applicable to traditional software. This distinction is to inform the development of robust security protocols and frameworks for AI.
-
Assessment of Exploitation and Impact: The report sought to examine how malicious actors can leverage the vulnerabilities to compromise AI systems, steal data, disrupt services, or conduct other unauthorised activities. This involves exploring potential attack vectors that could exploit the vulnerability.
-
Identification of Cyber Attack Case Studies: The report sought to identify case studies of cyber-attacks against AI models and systems, encompassing both real-world occurrences and theoretical proof of concepts.
By addressing these objectives, the report aims to provide actionable insights for safeguarding AI models and systems against cyber threats, improving the integrity, confidentiality, and availability of critical data and operations for AI systems.
Report structure
The report is structured as follows. Section 2 provides an overview of the methodology employed to conduct the risk assessment, while Section 3 explains the AI lifecycle and provides an overview of the current state of research in cyber security of AI models and systems. Section 4 presents the findings of the risk assessment, identifying the vulnerabilities, and potential exploits and impacts across the four phases of the AI lifecycle. The findings provide evidence reflecting each of these phases and the associated cyber security risks. Following that, a list of case studies is presented in Section 5, demonstrating the impact on AI systems, highlighting various attack characteristics, personas, ML paradigms, and use cases. Finally, Section 6 provides insights from interviews with clients across diverse sectors, gauging their market readiness and exploring their navigation through the cyber security realm, particularly concerning AI vulnerabilities.
2. Methodology
We adopted a holistic approach to the risk assessment and undertook a comprehensive evaluation by integrating literature review and client interviews. We conducted two literature reviews, evaluating two distinct, yet complementary research streams. Literature stream one (LS1) focused on government and industry reports while literature stream two (LS2) focused on high impact, peer reviewed academic publications. This meant that each stream had a clear and focused scope, while maintaining a more comprehensive understanding of the various dimensions of cyber security risks associated with AI. Government and industrial reports helped in validating findings from academic literature and demonstrating how concepts and empirical studies relate to real-world settings. Conversely, academic literature provided conceptual models that helped in contextualising the findings presented in government and industrial reports.
The Preferred Reporting Items for Systematic Reviews and Meta-Analyses (PRISMA) method (Page et al., 2021) was used to define the inclusion of literature. The PRISMA method, as a systematic approach, was employed to conduct comprehensive reviews across various databases for relevant studies, screening to determine eligibility based on predetermined criteria, extracting key data from selected studies, and synthesising the findings to draw conclusions. This structured process supports transparency and reliability in the review, allowing for informed decision-making and presentation of findings. By using the PRISMA method, we aimed to minimise bias and enable coverage of the literature landscape across both LS1 and LS2. Furthermore, the PRISMA method facilitates the synthesis of findings from multiple studies, allowing us to integrate the two research streams of LS1 and LS2 into a single coherent and relevant study enriching the overall depth and scope of the findings and drawing meaningful conclusions.
Consistent terminology was incorporated as search terms across both LS1 and LS2, including ìArtificial Intelligenceî, ìAIî, ìRiskî, ìCyberî, and ìSecurityî. Accordingly, we identified an initial batch of records from the following resources: 1) government policy, parliamentary committees, and official documents, 2) national cyber security guidance, 3) international reports, corporate documents, information notes, and opinion papers, 4) industry reports, white papers, and articles, all for LS1, and academic publication databases for LS2. We assessed records for eligibility by evaluating the scope, detail, and rigour of the papers, and whether the work had been superseded by newer iterations. We then manually evaluated each articleís relevance to this specific study for inclusion in the final synthesis of information gathered. This comprehensive search helped identify the key related works to inform the study. However, the sources included were restricted to English-language, with materials in all other languages excluded from consideration. Also, no limit was applied based on the year of publication. Limiting the resources by recent years may result in overlooking influential contributions that have shaped the field of AI. A comprehensive approach that encompasses both older and recent literature was applied to ensure a more thorough understanding of the research landscape. Details of each stage of the PRISMA method employed in this study is provided in the diagram below.
The selected literature was summarised, analysed, and synthesised, with the insights and findings integrated into the risk assessment. The findings were mapped in tables that include the cyber security vulnerabilities throughout the AI lifecycle, and their exploitation and impact. A list of case studies was also collated, presenting detailed accounts of security incidents, and enriching the understanding of how security risks manifest in different contexts.
Informed by the academic literature review, we conducted interviews with two experts in both AI technologies and cyber security. Integrated analysis between the literature research and the feedback from the 5 client interviews allowed us to gain a comprehensive understanding on the landscape of cyber risk to AI, evaluating the current developments in industry and the potential impact of academic research to industrial applications.
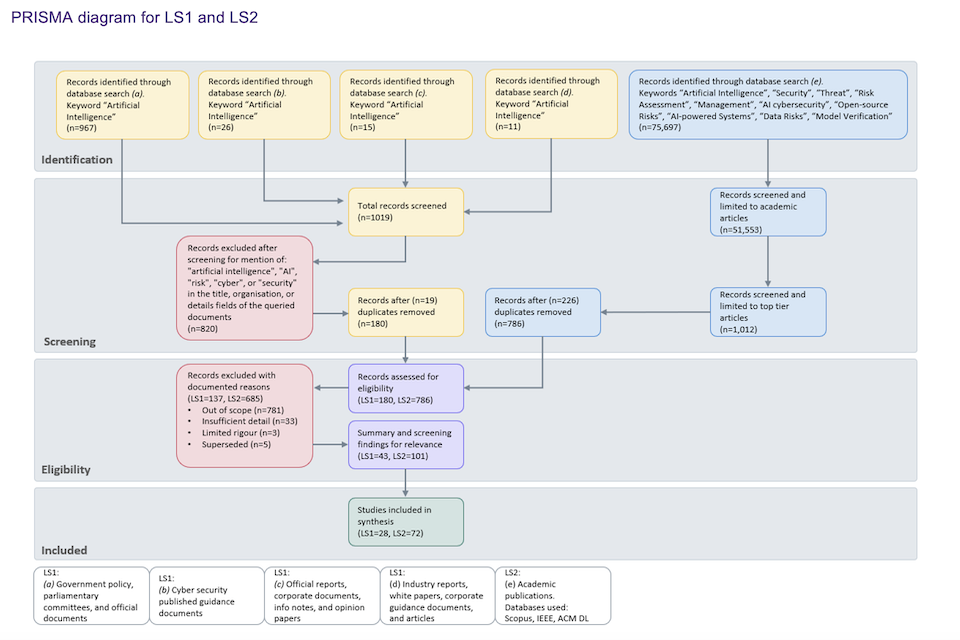
Figure 1 - PRISMA diagram for LS1 and LS2.
Study limitations
A limitation in our approach lies in the possibility of missing relevant sources beyond those encompassed by our defined literature streams, LS1 and LS2. While these streams sought to capture a wide array of publications from various stakeholders, including government bodies, industry, and academic institutions, the disparate nature of the literature means that there may exist insights and perspectives from alternative sources not included in our search criteria. Additionally, our reliance on keyword extraction and manual evaluation for eligibility screening may have inadvertently overlooked relevant literature. To mitigate the limitations above, we employed a cross validation approach where LS1 search, screening, and selected sources were validated by LS2 researchers, and vice versa. This included examining the relevance, credibility, and contribution to the risk assessment. Through collaborative discussion, the researchers identified gaps or overlaps in the literature coverage between the two streams and determined areas where additional literature needed to be sourced and redundant information that needed elimination.
Another limitation stems from the early stage of integration between AI and cyber security in industry, resulting in a scarcity of suitable case studies available for review. The limited availability of real-world cases may have constrained our ability to draw concrete conclusions or generalise findings beyond theoretical frameworks. This scarcity may have also hindered the depth of analysis regarding practical implementations, resulting in a potential gap in understanding the practical implications of AI cyber security measures. To mitigate this limitation, we conducted a set of interviews with Grant Thornton clients across diverse sectors, to integrate real-world experiences and concerns, ensuring that the risk assessment incorporates practical challenges encountered by organisations.
Finally, while interviews with experts provided valuable additional insights, the selection of interviewees and the scope of questions may have introduced sampling bias and limited the breadth of perspectives captured. Moreover, although the interviewees possessed a general understanding of machine learning applications, some lacked a detailed grasp of the AI’s lifecycle that is necessary to assess vulnerabilities and the risk landscape effectively.
3. Background
The AI lifecycle
In this report we discuss AI across various phases, known as the AI lifecycle, shown in figure 2 below. The AI lifecycle comprises four main phases: Design, Development, Deployment, and Maintenance, with each playing a crucial role in creating and sustaining an effective AI system.
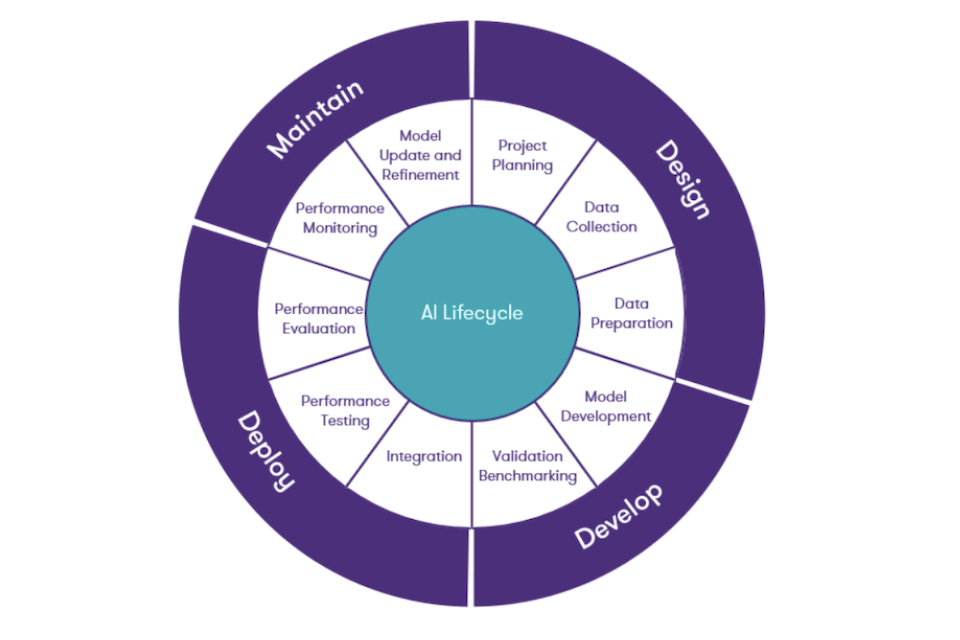
Figure 2 - The AI Lifecycle
In the Design phase, the focus is on understanding the problem domain, gathering, and preparing relevant data, selecting appropriate algorithms, and designing a prototype to validate key concepts. The primary motivation is establishing the planning and rationale for AI integration, conceptualising the AI system, and outlining its goals and functionalities (Choudhury and Asan, 2020). This phase lays the groundwork for the subsequent development of the AI system.
The Development phase involves data pre-processing, implementing the AI system through algorithm selection, model training, evaluation, and optimisation. Data is prepared and fed into the chosen algorithms to train the AI models, which are then evaluated and optimised to achieve the desired performance metrics. Iterative experimentation is common in this phase to fine-tune the model and improve its effectiveness (Nguyen et al., 2021).
Once developed, the system progresses to the Deployment phase, and is integrated into its operational environment. Here, the trained model is assessed for scalability and performance, and monitored for any issues or drift in performance. Strategy and framework for continuous monitoring and feedback collection are employed to ensure the AI system operates effectively in real-world conditions and to address any issues that may arise (Hu et al., 2021).
The Maintenance phase involves ongoing activities such as performance monitoring, model retraining, bug fixing, updates, and refinements of the AI system. AI models may degrade over time, requiring periodic retraining with updated data to maintain performance. Addressing bugs, implementing updates, and ensuring the security and compliance of the system are critical to its long-term success. By employing a structured approach through each phase of the lifecycle, organisations can develop and maintain AI systems that deliver value and impact while mitigating risks and ensuring accountability (Lehne et al., 2019).
Operations and evaluation are pivotal in enabling the sustained viability and efficacy of AI systems (European Union Agency for Cybersecurity., 2020; Silva and Alahakoon, 2022). Operations encompass the routine supervision and control of the AI system, which consists of data ingestion, processing, and decision-making (Silva and Alahakoon, 2022). Ongoing assessment throughout the AI lifecycle enables the identification of potential hazards, deficiencies, and emergent obstacles, thereby promoting preventative measures to preserve the integrity and functionality of the system (Joint Task Force Transformation Initiative, 2012; European Union Agency for Cybersecurity., 2023d).
The AI cyber security landscape
The term “AI cyber security landscape” refers to the extensive range of considerations, strategies, technologies, and regulatory efforts aimed at securing artificial intelligence systems against cyber risks. This encompasses the identification, evaluation, and mitigation of potential vulnerabilities within AI systems, as well as the development of protective measures to guard these systems against cyber-attacks. Assessing the cyber risks in the evolving landscape of AI is challenging due to the increasing complexity and interconnectedness of AI and cyber security. Academic researchers, government departments, and leading AI organisations are actively investigating effective approaches to tackle these challenges. This section provides a summary of the existing studies that have been used to frame our risk assessment. We provide a thematic overview of the limited studies in this area and differentiate them based on the scope of the studies. We also include a summary of those Government agencies, departments and bodies that are responsible for evaluating and addressing cyber risks to AI to further contextualise this work.
To establish a foundational understanding of the breadth and depth of research conducted in this domain, a thorough examination of the volume of studies was performed. We identified 72 risk evaluations or assessments related to AI, along with 28 publications related to government departments, agencies, functions, or industry organisations looking at the cyber security risks to AI. In terms of the scope, 12 studies specifically discussed project planning risks, 22 discussed data collection and preparation, 19 examined model development, 10 were concerned with validation and benchmarking, and 7 investigated risks to distribution and maintenance. Furthermore, 18 publications provided a high-level overview of the AI cyber security landscape, and 22 studies examined possible risks comprehensively, underscoring the significance of employing extensive risk assessment methodologies.
A notable gap prevalent in the studies above lies in the lack of attention to the distinct phases comprising the whole AI life cycle when mapping cyber security risks. As such, 29 of the total number of studies focused on areas that were considered to be within a specific phase of the AI lifecycle, informed by the scoping work conducted, whereas the remaining 71 assessed risks across multiple phases or adopted a more comprehensive approach. Furthermore, while studies documenting individual cyber-attacks on AI systems are available, a comprehensive mapping of these attacks and their implications to AI systems was not prominently featured. This indicates a notable gap in the current literature, as such mappings are crucial for understanding how vulnerabilities may translate into actual attacks in practical settings.
Following the comprehensive review of current publications, it becomes evident that proactive measures are being undertaken globally. Government institutions worldwide, such as the EU General Secretariat of the Council (2022), the US AI Safety Institute (USAISI) (2023b), China’s Artificial Intelligence Industry Alliance (Luong and Arnold, 2021), Japan’s AI Safety Institute (METI, 2024), and the Republic of Korea’s Ministry of Science and ICT Strategy (MSIT, 2024) are deeply engaged in understanding and managing AI innovation risks. Notably, the establishment of entities like the UK Government’s (2023) Central AI Risk Function (CAIRF), the Frontier AI Taskforce, and AI Safety Institutes, alongside concerted efforts by the UK National Cyber Security Centre and the US Cybersecurity and Infrastructure Security Agency (2023), signifies a collective move towards enhanced risk management in AI innovation.
These initiatives, along with the Cyber Security Strategy (2023a) outlined by the European Union Agency for Cyber Security (ENISA), and the Artificial Intelligence Risk Management Framework (NIST, 2023a) by the National Institute of Standards and Technology (NIST), play a pivotal role. They not only acknowledge the need for structured risk identification and prioritisation but also hint at a broader understanding that encompasses both conventional and AI-specific considerations. Such comprehensive strategies are crucial for addressing the gaps identified in the initial review, particularly the need for detailed mappings of theoretical and real-life cyber-attacks across the AI lifecycle. By integrating these governmental efforts into the landscape of AI cyber security risk assessment, there is a defined pathway towards mitigating the vulnerabilities identified and enhancing the trustworthiness of AI systems globally.
Leading AI organisations including Anthropic, AWS, Cohere, Darktrace, Google DeepMind, Meta, Microsoft, NVIDIA, OpenAI, OWASP, Palo Alto, and Rapid7 are also addressing challenges and risks related to advanced AI models. While specific references (Apruzzese et al., 2023; Anthropic, 2023a, 2023b, 2023c; AWS, 2024; Brundage et al., 2018; Cohere, 2023; Google, 2023; Horvitz, 2022; Marshall et al., 2024; NVIDIA, 2024; OpenAI, 2023, 2024; OWASP, 2024; Shevlane et al., 2023) highlight several contributions from a selection of these entities, it’s important to recognise the broader community’s efforts. Many organisations, not limited to those listed, contribute significantly to the research, development, and implementation of AI safety strategies and methodologies to mitigate emerging cyber risks associated with AI deployment. As such, the collective knowledge, recommendations, and actions of this diverse group of stakeholders are also critical in navigating the complex landscape of AI safety and cybersecurity.
Current literature, however, does not cover a comprehensive evaluation of AI-specific cyber security risks across each stage of the AI lifecycle. Ignoring these phases undermines the efficacy of risk mitigation strategies, leaving organisations vulnerable to unforeseen threats that may emerge at any point along the AI life cycle. As such, there exists a pressing need for a more encompassing perspective, recognising and addressing Cyber Security risks across all stages of the AI life cycle. This risk assessment aims to fill this gap by thoroughly evaluating the potential exploitation, impact, and AI-specific cybersecurity risks associated with each lifecycle phase. The selected literature was summarised, analysed, and synthesised, with the insights and findings integrated into the risk assessment. A list of case studies was also collated, enriching the understanding of how security risks manifest in different contexts. Informed by the literature review, we further conducted interviews with experts in both AI technologies and cyber security, which allowed us to gain and present a comprehensive understanding on the landscape of cyber risk to AI.
4. Findings of the risk assessment
Our analysis is structured to navigate the intricate landscape of risks, emphasising distinctions between general software vulnerabilities and those specifically unique to AI systems. When selecting the vulnerabilities for inclusion, it is essential to distinguish their roots and association with either AI, software, or both. Our rational is that if a vulnerability emerges only due to the existence of AI, particularly machine learning, it is identified exclusively as an AI vulnerability. Conversely, if the vulnerability derives from fundamental software infrastructure issues and persists regardless of the presence of AI, it is attributed to software. However, if the vulnerability persists when either AI or software are involved, it is considered to be associated with both. This analytical approach assists in delineating the specific impact of vulnerabilities on different components of our categorisation method across the AI lifecycle.
We refrained from ordering or ranking the identified vulnerabilities in the risk assessment due to the subjectivity and potential bias inherent in such a ranking, as well as the absence of existing literature that inform this approach. Furthermore, each vulnerability is evaluated independently without considering possible existing relationships between them, or their exploitation and impact. While it is acknowledged that certain cyber vulnerabilities associated with AI may permeate various phases of the AI lifecycle, our risk assessment deliberately refrains from incorporating this aspect due to potential subjectivity concerns. Our focus remains on providing a structured assessment rather than assigning hierarchical significance.
Design phase
The design phase of AI systems represents a critical stage where the foundation for system development is laid, encompassing various intricate processes such as data gathering, preparation, planning, and model design.
Vulnerability | Exploitation |
Lack of Robust Security Architecture (AI/Software) - A resilient security architecture is not adequately designed, lacking access controls, secure design principles, and proper network configurations (BÈcue, PraÁa and Gama, 2021; European Union Agency for Cybersecurity., 2023). | Lack of security architecture may allow unauthorised access or malicious code injection. Injected poisoned data during training compromises decision-making and biases outputs (BÈcue, PraÁa and Gama, 2021). |
Inadequate Threat Modelling (AI) - Insufficient identification of potential threats, vulnerabilities, and attack vectors in the AI system, leading to overlooking vulnerabilities and inadequate system design (Bradley, 2020; European Union Agency for Cybersecurity., 2020; Zhang et al., 2022). | Adversaries exploit unanticipated threats and attack surfaces, injecting poisoned data during data preparation or collection, impacting the model design phase (Bradley, 2020; Hu et al., 2021; Zhang et al., 2022). |
Insufficient Data Privacy Safeguards (AI) -Lack of measures to safeguard sensitive data during model development, testing, and deployment, risking privacy breaches (Chiang and Gairola, 2018; European Union Agency for Cybersecurity., 2023). | Insufficient data privacy may lead to unauthorised access, injecting poisoned data, and compromising the confidentiality of user data during various phases of AI (Chiang and Gairola, 2018). |
Insecure Authentication and Authorisation (Software) - Weak or improperly implemented authentication and authorisation mechanisms, including risks like weak password policies and lack of multi-factor authentication (European Union Agency for Cybersecurity., 2020, 2023; Mirsky et al., 2023). | Attackers can exploit vulnerabilities to gain unauthorised access, steal sensitive data, or impersonate legitimate users, leading to data breaches and unauthorised system changes (European Union Agency for Cybersecurity., 2020, 2023; Mirsky et al., 2023). |
Inadequate Input validation and sanitisation (Software) - Attackers manipulate SQL queries through input fields due to inadequate validation, leading to unauthorised access and modification of database information (Hu et al., 2021). | Exploitation can result in unauthorised access to or modification of database information, leading to data theft, loss, or corruption. In severe cases, attackers may gain administrative rights to the database system information (Hu et al., 2021). |
Inadequate output encoding (Software) - Including untrusted data in a web page without proper output encoding or escaping allows attackers to execute malicio scripts, endangering user data and compromising interactions (Carlo et al. 2023). | Exploitation can lead to session hijacking, website defacement, redirection to malicious sites, or phishing, directly impacting users and compromising their data and interaction with the application (Carlo et al. 2023). |
Insecure Data Storage and Transmission (Software) - Improper encryption or handling of data during storage or transmission, risking interception and unauthorised access, leading to data theft and breaches. | Unsecured data can be intercepted during transmission or accessed through breached storage systems, resulting in data theft and breaches. |
Insufficient Error Handling and Logging (Software) - Poor error handling and inadequate logging mechanisms may reveal system details to attackers and hinder incident detection and response (European Union Agency for Cybersecurity., 2023). | Exploiting inadequate error handling can provide insights into the system’s architecture, facilitating further attacks. Ineffective logging hinders the detection and response to security incidents. (European Union Agency for Cybersecurity., 2023). |
Inadequate Security Assessment in AI Model Selection (AI) - Failure to consider security implications when selecting AI models, leading to the adoption of models with inherent vulnerabilities (Boulemtafes, Derhab and Challal, 2020). | Attackers may exploit vulnerabilities in selected AI models, such as susceptibility to adversarial attacks or poor generalisation, leading to wrong outputs, system compromises, or manipulation of model behaviour, potentially resulting in financial losses, reputational damage, or privacy violations (Boulemtafes, Derhab and Challal, 2020). |
Data Collection and Preparation (AI/Software) - Model performance and training depend on the quality data preparation, including feature selection, extraction, integration, and cleaning. This impacts system performance and the ability to handle high-dimensional data (Haakman et al., 2021; Silva and Alahakoon, 2022). | Weak access controls can be exploited by attackers to gain unauthorised access, potentially modifying AI models, leaking sensitive data, or disrupting the development process (Mirsky et al., 2023). |
Use of unreliable sources to label data (AI/Software) - Label modification vulnerability allows adversaries to alter or manipulate data labels, compromising the integrity and reliability of machine learning models (Majeed and Hwang, 2023). | Label modification: This sub-threat is specific to Supervised Learning, resulting in an attack in which the attacker corrupts the labels of training data (Majeed and Hwang, 2023). |
Bias Injection into Machine Learning Models (AI) - This vulnerability refers to the deliberate introduction of biases into machine learning models or the datasets used to train them, resulting in skewed or unfair outcomes in decision-making processes. These biases can stem from various sources, including biased training data or intentional manipulation of the model’s design (Ferrer et al., 2021; Vassilev 2024). | Attackers exploit this vulnerability by injecting biased data into the training process or manipulating the model’s architecture to the side of certain outcomes. This injection of bias can lead to discriminatory or inaccurate predictions, perpetuating societal biases or causing unjust treatment of individuals (Ferrer et al., 2021; Vassilev 2024).** |
Development phase
The development phase of the AI lifecycle is where AI models are created and refined for specific tasks. This phase involves selecting appropriate algorithms, and training and evaluating the model’s performance. Iterative processes for model tuning and optimisation are conducted to enhance accuracy and robustness.
Vulnerability | Exploitation |
Insecure AI Code Recommendations (AI) - Vulnerabilities in open-source code, particularly in tools like GitHub Copilot, arise from limitations in programming models. These models may inadvertently learn insecure coding patterns, leading to recommendations of code with security vulnerabilities (Hu et al., 2021; Carlo et al., 2023; UK National Cyber Security Centre and US Cybersecurity and Infrastructure Security Agency 2023). | Exploitation involves using insecure code suggestions to create or maintain software with hidden vulnerabilities. This can lead to unauthorised access, data breaches, and the propagation of insecure coding practices (Mirsky et al., 2023). |
Code Vulnerabilities (AI/Software) - Code vulnerabilities in the source code of the AI system are susceptible to exploitation, compromising system integrity, confidentiality, or availability (Chiang and Gairola, 2018; Mirsky et al., 2023). | Attackers may exploit coding errors, buffer overflows, or insecure dependencies to execute arbitrary code, manipulate AI models, or gain unauthorised access to system resources (Carlo et al., 2023). |
Unsecured Data Handling in AI Systems (AI/Software) - Inadequately protecting data during storage and transmission between different components of the AI system (Silva and Alahakoon, 2022). | Attackers may intercept or manipulate data during storage or transmission, leading to unauthorised access or tampering with sensitive information (Nguyen et al., 2021). |
Weak Access Controls (AI/Software) - Inadequately restricting access to AI development environments, models, or data, allowing unauthorised users to perform actions beyond their permissions (Chiang and Gairola, 2018). | Exploitation involves attackers gaining unauthorised access, potentially modifying AI models, leaking sensitive data, or disrupting the development process (Mirsky et al., 2023). |
Inadequate Input validation and sanitisation (AI) - This vulnerability allows injecting instructions/commands into an AI model, causing it to deviate from its intended behaviour. It encompasses injecting commands that lead the model to perform unintended tasks, potentially compromising system integrity (Hu et al., 2021; Vassilev 2024). | The AI model’s behaviour could be inadvertently modified by an adversary who injected commands into it, causing it to execute unauthorised tasks or generate incorrect responses. The potential consequences are manifold; they may consist of unauthorised access, data breaches, or subversion of the system’s output, contingent upon the particular use case and context (Hu et al., 2021; Mirsky et al., 2023; Vassilev, 2024). |
Susceptibility to Input Perturbation (AI) - Input perturbation enables altering valid inputs to AI models, resulting in incorrect outputs, commonly known as evasion or adversarial examples. This poses risks primarily to decision-making systems, impacting the reliability of their outputs (Hu et al., 2021). | Malicious actors can perturb valid inputs to AI models, causing them to produce incorrect outputs consistently, leading to incorrect decisions in critical applications, such as autonomous vehicles or healthcare diagnosis, resulting in safety hazards, financial losses, or compromised security (Hu et al., 2021). |
Insecure AI Supply Chain (AI/Software) - Failure to secure AI-related components acquired from external suppliers, leading to the potential compromise of the AI system’s security and integrity (Hu et al., 2021). | Malicious actors may exploit vulnerabilities in insecurely sourced AI components to introduce backdoors, malware, or other malicious code into the system, potentially leading to data breaches, system compromises, or unauthorised access (Hu et al., 2021). |
Inadequate Asset Protection (AI/Software) - Lack of proper identification, tracking, and protection of AI-related assets, including models, data, software, and documentation, leaving them vulnerable to unauthorised access, manipulation, or theft (European Union Agency for Cybersecurity., 2020; Rodrigues, 2020). | Attackers may exploit weaknesses in asset management processes to gain unauthorised access to sensitive AI-related assets, such as models or datasets, leading to data breaches, intellectual property theft, or compromise of system integrity (Rodrigues, 2020). |
Deployment phase
The deployment phase of the AI lifecycle marks the transition of developed AI models from development environments to real-world applications. In this phase, the focus shifts towards ensuring that the AI solution operates effectively and efficiently in operational settings. Model deployment, infrastructure setup, and monitoring mechanisms are implemented to support the ongoing operation of AI systems.
Vulnerability | Exploitation |
Insecure API Endpoints (AI/Software) - Vulnerabilities in interfaces that allow communication between different components of the AI system, inadequately securing endpoints that expose functionality to external entities (Boulemtafes, Derhab and Challal, 2020; Carlo et al., 2023). | Exploitation involves attackers taking advantage of inadequately protected API to gain unauthorised entry, introduce malicious inputs, or disrupt the regular operation of the AI system. Impact includes unauthorised data access, denial of service, or manipulation of AI model inputs (Carlo et al., 2023). |
Infrastructure (AI/Software) - Infrastructure considerations in the deployment phase involve the configuration and setup of physical and virtual components necessary to support the operational integration of the AI system within its designated environment (Silva and Alahakoon, 2022). | Inadequate attention to infrastructure security exposes vulnerabilities, allowing unauthorised access leading to data breaches, operational downtime, or service disruptions. Adversarial attacks on infrastructure security may be exploited during deployment for unauthorised access leading to service disruptions (Rodrigues, 2020; Carlo et al., 2023). |
Lack of Encryption During Data Transmission (Software) - The lack of encryption during data transmission refers to the failure to secure data as it travels between different components or entities within the AI system (Boulemtafes, Derhab and Challal, 2020). | Adversarial attacks may take advantage of the absence of encryption during data transfer and manipulate sensitive information. Eavesdropping on unencrypted data during transmission enables malicious actors to illegally obtain or manipulate confidential information, unauthorised access to private data and a breach of data confidentiality (Mirsky et al., 2023). |
Configuration Vulnerabilities in Cloud Services (AI/Software) - Misconfiguration of cloud services like improperly setting up or configuring cloud-based components, such as storage, computing resources, or databases (Boulemtafes, Derhab and Challal, 2020). | Adversarial attacks exploit misconfigured cloud settings to gain unauthorised access to AI services, potentially leading to data breaches or service disruptions (Boulemtafes, Derhab and Challal, 2020; Carlo et al. 2023). |
Model Stealing (AI) - Model stealing allows attackers to extract the architecture or weights of a trained AI model, create functionally equivalent copies. Additionally, certain software vulnerabilities, such as insecure storage or weak access controls, can inadvertently facilitate model stealing (Chang et al., 2020). | Attackers can extract the architecture or the trained AI models, creating functionally equivalent copies for unauthorised use or intellectual property theft. This could lead to financial losses, unauthorised access to proprietary technology, or exploitation of competitive advantages (Chang et al., 2020). |
Prompt Extraction (AI/Software) - Prompt extraction enables attackers to extract or reconstruct the system prompt provided to an AI model, potentially revealing confidential information, and compromising system security (Hu et al., 2021). | It is possible for adversaries to extract or reconstruct system prompts that are delivered to AI models, which may compromise the security of the system and possibly reveal sensitive information including unauthorised access , breaches of data security, or violations of privacy (Hu et al., 2021). |
Model Output Accessibility (AI) - Failure to protect AI models and data from direct and indirect access, increasing the risk of unauthorised model reconstruction, data theft, or tampering, compromising model integrity and trustworthiness (Bouacida and Mohapatra, 2021; Hu et al., 2021; Vassilev 2024). | Attackers may attempt to access models directly or query models through applications to reconstruct model functionality, steal sensitive data, or tamper with models, undermining their reliability and trustworthiness, potentially leading to data breaches, loss of intellectual property, or compromised system performance (Bouacida and Mohapatra, 2021; Hu et al., 2021; Vassilev 2024). |
Inadequate Evaluation and Testing (AI/Software) - Releasing AI models, applications, or systems without thorough security evaluation, testing, or clear communication of limitations, exposing users to potential security risks or failure modes (Rodrigues, 2020; Carlo et al., 2023). | Attackers may exploit vulnerabilities in inadequately evaluated or tested AI systems to compromise user data, disrupt system operations, or exploit security weaknesses, potentially leading to data breaches, financial losses, or reputational damage, undermining user trust and confidence in the AI system (Rodrigues, 2020; Carlo et al., 2023). |
Maintenance phase
In the maintenance phase of the AI lifecycle, the focus shifts to sustaining the performance and relevance of deployed AI solutions over time. This involves ongoing monitoring of model performance, data quality, and system integrity to ensure continued effectiveness in real-world applications. Maintenance tasks include updating models with new data to maintain relevance and accuracy, addressing any drift or degradation in performance, and adapting to evolving user needs or environmental changes. Regular evaluations and audits are conducted to assess the AI solution’s performance against predefined metrics and to identify areas for improvement or optimisation (2020; Bouacida and Mohapatra, 2021; 2023).
Vulnerability | Exploitation |
Delayed Security Patches (AI/Software) - Delayed security patches refer to the postponement of applying updates or fixes to known vulnerabilities in the software and components used in the AI (Carlo et al., 2023). | Attackers can exploit unpatched vulnerabilities, compromising system integrity, executing arbitrary code, manipulating AI models, or gaining unauthorised access to sensitive information. Adversarial attacks may target known vulnerabilities in outdated AI components, attempting unauthorised access, model manipulation, or data theft (Carlo et al., 2023). |
Model Decay and Concept Drift (AI) - Model decay and concept drift refer to the deterioration of AI model effectiveness over time due to changing input distributions or shifts in the underlying data (Zhang et al., 2022; European Union Agency for Cybersecurity. 2020). | Exploiting decreasing model performance allows adversaries to manipulate predictions, leading to biased outputs, incorrect predictions, or degraded performance. Adversaries may intentionally influence input data distributions to manipulate AI predictions, resulting in incorrect or biased outcomes (European Union Agency for Cybersecurity., 2020; Zhang et al., 2022). |
Insider Threats (AI) - Insider threats involve malicious activities by internal personnel who have access to the AI system, models, or sensitive (Mirsky et al., 2023). | Insiders exploit access privileges to engage in unauthorised activities, compromising the confidentiality, integrity, and availability of AI models and sensitive data. Adversarial attacks manifest as deliberate actions by employees with privileged access, including data theft or sabotage of AI models and data (Mirsky et al., 2023) |
Insufficient Logging (AI/Software) - Logging in the maintenance phase involves systematically recording and monitoring system activities, errors, and performance metrics to facilitate the ongoing evaluation and optimisation of the AI system (Zhang et al., 2022; European Union Agency for Cybersecurity. 2020). | Insufficient logging procedures may slow problem detection, impede resolution, and enable malicious individuals to exploit undetected weaknesses, resulting in unauthorised access or manipulation of the AI system. Adversarial attacks might focus on insufficient logging, aiming to take advantage of limited insight into system operations and possibly leaving vulnerabilities undetected (Bradley, 2020). |
5. List of case studies
Attacks on AI systems are making a noticeable change from controlled settings to practical production systems (Hu et al., 2021). This change highlights the increasing need for comprehending such attacks within real-world situations (Hu set al., 2021; Carlo et al., 2023; European Union Agency for Cybersecurity., 2023d). Case studies provide real-life examples where risks have materialised or have been mitigated, offering practical insights to the risk assessment.
The following case studies demonstrate the effects on operational AI systems, showcasing different attack features, personas, machine learning methodologies, and impacted use cases. The attacks use many tactics, such as evasion, poisoning, model replication, and exploiting conventional software vulnerabilities. They include various malicious actors, from regular users to skilled red teams, who focus on attacking machine learning models in environments such as cloud-hosted, on-premises, and edge installations. These case studies examine security-sensitive and non-security-sensitive applications, offering detailed insights into the vulnerabilities AI systems encounter in real-world scenarios.
Name & Source | Description |
ChatGPT Plugin Privacy Leak \n Incident Date: May 2023. \n Actor: Embrace The Red. \n Target: OpenAI ChatGPT. \n (Gupta et al., 2023) | A vulnerability in ChatGPT known as “indirect prompt injection” allows an attacker to take control of a chat session and steal the conversation’s history by using ChatGPT plugins to feed malicious websites. Users may be susceptible to Personal Identifiable Information (PII) leaks from the retrieved chat session because of this attack. |
Indirect Prompt Injection Threats: Bing Chat Data Pirate \n Incident Date: 2023 \n Actor: Kai Greshake, Saarland University. \n Target: Microsoft Bing Chat \n (Greshake et al., 2023) | A user may grant Bing Chat permission to browse and access webpages that are presently open during a chat conversation using Microsoft’s new Bing Chat LLM Chatbot. Researchers showed how an attacker might insert a malicious script into a user’s browser to stealthily transform Bing Chat into a social engineering tool that searches for and steals personal data. This attack may be conducted without the user having to ask questions about the website or do anything other than engage with Bing Chat while the page is open in the browser. |
PoisonGPT \n Incident Date: July 2023 \n Actor: Mithril Security Researchers \n Target: HuggingFace Users. \n (Huang et al., 2023) | An open-source, pre-trained large language model (LLM) manipulated by researchers at Mithril Security to produce a bogus reality. To highlight the LLM supply chain’s weakness, they were able to successfully upload the poisoned model back to HuggingFace, the biggest model hub that is available to the public. Users could have downloaded the contaminated model, obtaining, and disseminating contaminated information and data, potentially leading to a number of negative outcomes. |
Arbitrary Code Execution with Google Colab. \n Incident Date: July 2022 \n Actor: Tony Piazza \n Target: Google Colab. \n (Valizadeh and Berger, 2023) | Google Colab is a virtual machine based Jupyter Notebook service. Jupyter Notebooks, which include typical Unix command-line features and executable Python code snippets, are often used for ML and data science study and experimentation. This code execution feature not only lets users alter and visualise data, but it also lets them download and manipulate files from the internet, work with files in virtual machines, and more. \n Additionally, users may use URLs to share Jupyter Notebooks with other users. Users who use notebooks that include malicious code run the risk of unintentionally running the malware, which might be concealed or obfuscatedófor example, in a downloaded script. \n A user is prompted to provide the notebook access to their Google Drive when they open a shared Jupyter Notebook in Colab. While there may be good reasons to provide someone access to Google Drive, such as letting them replace files with their own, there may also be bad ones, including data exfiltration or opening a server to the victim’s Google Drive. The ramifications of arbitrary code execution and Colab’s Google Drive connection are brought to light by this experiment. |
Bypassing ID.me Identity Verification \n Incident Date: October 2020 \n Reporter: ID.me internal investigation \n Actor: One individual \n Target: California Employment \n Development Department \n (Laborde et al., 2020) | Using ID.me’s automatic identification verification system, a person submitted at least 180 fraudulent unemployment claims in the state of California between October 2020 and December 2021. After dozens of false claims were accepted, the person was paid at least $3.4 million. \n The man used the stolen personal information and pictures of himself donning wigs to create several false identities and bogus driver’s licences. He then registered for ID.me accounts and completed their identity verification procedure. The procedure compares a selfie to an ID picture to authenticate personal information and confirm the user is who they say they are. By using the same wig in his supplied selfie, the person was able to confirm identities that had been stolen. \n After that, the person used the ID.me validated identities to submit false unemployment claims with the California Employment Development Department (EDD). The faked licences were approved by the system because to shortcomings in ID.me’s identity verification procedure at the time. After being accepted, the person had payments sent to many places he could access and used cash machines to withdraw the funds. The person was able to take out unemployment benefits totalling at least $3.4 million. Eventually, EDD and ID.me discovered the fraudulent activities and alerted federal authorities to it. Regarding this and another fraud case, the person was found guilty of wire fraud and aggravated identity theft in May 2023 and was given a sentence of 6 years and 9 months in prison. |
Achieving Code Execution in MathGPT via Prompt Injection. \n Incident Date: 28 January 2023 \n Actor: Ludwig-Ferdinand Stumpp \n Target: MathGPT (https://mathgpt.streamlit.app/) \n (Ding, Cen and Wei, 2023) | GPT-3 is a large language model (LLM) that is used by the publicly accessible Streamlit application MathGPT to respond to user-generated arithmetic problems. \n When it comes to directly executing accurate maths, LLMs like the GPT-3 perform poorly, according to recent research and experiments. When requested to develop executable code that answers the given query, however, they may provide more precise results. The user’s natural language inquiry in the MathGPT application is translated into Python code using GPT-3 and then executed. The user is shown the result of the calculation together with the code that was run. \n Prompt injection attacks, in which malicious user inputs force the models to behave unexpectedly, might affect certain LLMs. The actor in this incident investigated many prompt-override paths, generating code that allowed the actor to execute a denial-of-service attack and get access to the environment variables of the application host system and the application’s GPT-3 API key. Consequently, the actor may have crashed the programme or used up all the API query budget. \n The MathGPT and Streamlit teams were informed of the attack pathways and their outcomes, and they promptly took action to address the vulnerabilities by filtering on certain prompts and changing the API key. |
Compromised PyTorch-nightly Dependency Chain \n Incident Date: 25 December 2022 \n Reporter: PyTorch \n Actor: Unknown \n Target: PyTorch \n (Ladisa et al., 2023) | A malicious malware submitted to the Python Package Index (PyPI) code repository from December 25 to December 30, 2022, compromised Linux packages for PyTorch’s pre-release version, known as Pytorch-nightly. The malicious package was installed by pip, the PyPI package manager, instead of the genuine one since it had the same name as a PyTorch dependent. \n Due to a supply chain assault dubbed “dependency confusion,” confidential data on Linux computers running impacted pip versions of PyTorch-nightly was made public. PyTorch made the announcement about the problem and the first efforts taken to mitigate it on December 30, 2022. These included renaming and removing the torchtriton dependencies. |
Confusing Antimalware Neural Networks. \n Incident Date: 23 June 2021 \n Actor: Kaspersky ML Research Team \n Target: Kaspersky’s Antimalware ML Model \n (Djenna et al., 2023) | ML malware detectors are increasingly being used in cloud computing and storage systems. In these situations, users’ systems are used to build the model’s characteristics, which are then sent to the servers of cyber security companies. This gray-box situation was investigated by the Kaspersky ML research team, who demonstrated that feature information is sufficient for an adversarial assault against ML models. \n Without having white-box access to one of Kaspersky’s antimalware ML models, they effectively avoided detection for the majority of the maliciously altered malware files. |
Backdoor Attack on Deep Learning Models in Mobile Apps \n Incident Date: 18 January 2021 \n Actor: Yuanchun Li, Jiayi Hua, Haoyu Wang, Chunyang Chen, Yunxin Liu \n Target: ML-based Android Apps \n (Li et al., 2021) | Deep learning models are becoming essential parts of mobile apps. Microsoft Research researchers have shown that a large number of deep learning models used in mobile applications are susceptible to “neural payload injection” backdoor attacks. They gathered real-world mobile deep learning applications from Google Play and conducted an empirical investigation on them. They found 54 apps that may be attacked, including well-known security and safety apps that are essential for facial identification, parental control, currency recognition, and financial services. |
Face Identification System Evasion via Physical Countermeasures \n Incident Date: 2020 \n Actor: MITRE AI Red Team \n Target: Commercial Face Identification Service \n (Zheng et al., 2023) | The AI Red Team from MITRE executed a physical-domain evasion attack against a commercial face identification service to cause a deliberate misclassification. Traditional ATT&CK enterprise techniques, including executing code via an API and locating valid accounts, were interspersed with adversarial ML-specific assaults in this operation. |
Microsoft Edge AI Evasion \n Incident Date: February 2020 \n Actor: Azure Red Team \n Target: New Microsoft AI Product \n (Sivaram et al., 2022) | A red team exercise was conducted by the Azure Red Team on a novelty from Microsoft that is specifically engineered to execute AI workloads at the periphery. The objective of this exercise was to induce misclassifications in the ML model by perpetually manipulating a target image using an automated system. |
Microsoft Azure Service Disruption \n Incident Date: 2020 \n Actor: Microsoft AI Red Team \n Target: Internal Microsoft Azure Service \n (Torkura et al., 2020) | A red team exercise was conducted by the Microsoft AI Red Team on an internal Azure service with the explicit purpose of causing service disruption. Traditional ATT&CK enterprise techniques, including data exfiltration and valid account discovery, were interspersed with adversarial ML-specific steps, including offline and online evasion examples, in this operation. |
Tay Poisoning \n Incident Date: 23 March 2016 Reporter: Microsoft \n Actor: 4chan Users \n Target: Microsoft’s Tay AI Chatbot \n (Neff and Nagy, 2016) | Microsoft developed the Twitter chatbot Tay with the intention of amusing and involving users. In contrast to preceding chatbots, which relied on pre-programmed scripts for responses, Tay was capable of being directly influenced by the conversations it engaged in due to its machine learning capabilities. \n A concerted assault incentivised malevolent users to disseminate abusive and profane language via Twitter towards Tay, resulting in Tay producing content that was just as inflammatory towards other users. \n Within twenty-four hours of the bot’s introduction, Microsoft discontinued Tay and issued a public apology detailing the lessons it had learned from its failure. |
ProofPoint Evasion \n Incident Date: 9 September 2019 \n Actor: Researchers at Silent Break Security \n Target: ProofPoint Email Protection System \n (Sivaram et al., 2022) | The code repository known as Proof Pudding details the method employed by ML researchers to circumvent ProofPoint’s email protection system (CVE-2019-20634). Specifically, they used the insights gained from a copycat email protection ML model to bypass the live system. More precisely, the understandings enabled scientists to construct malevolent electronic messages that obtained favourable ratings, evading detection by the system. ProofPoint assigns a numerical score to each word in an email, which is determined by a combination of several variables. If the email’s overall score is deemed insufficient, an error will be generated, designating it as SPAM. |
GPT-2 Model Replication \n Incident Date: 22 August 2019 \n Actor: Researchers at Brown University \n Target: OpenAI GPT-2 \n (Li, Zhang and He, 2022) | OpenAI developed the GPT-2 language model, which can produce text samples of exceptional quality. OpenAI adopted a tiered release schedule in response to concerns that GPT-2 could be exploited for malicious purposes, including impersonating others, generating misleading news articles, false social media content, or spam. Initially, they distributed a scaled-down, comparatively weaker iteration of GPT-2 accompanied by a technical elucidation of the methodology, while withholding the complete trained model. \n Brown University researchers effectively replicated the model using OpenAI-released information and open-source ML artefacts prior to the complete model’s release. This illustrates how an adversary equipped with adequate computational resources and technical expertise could have imitated GPT-2 and employed it for malevolent intentions prior to the AI Security community’s detection. |
ClearviewAI Misconfiguration \n Incident Date: April 2020 \n Actor: Researchers at spiderSilk \n Target: Clearview AI facial recognition tool \n (Anisetti et al., 2020) | A facial recognition application developed by Clearview AI searches publicly accessible images for matches. This instrument has been used by law enforcement agencies and other entities for investigative objectives. \n Despite being protected by a password; the source code repository of Clearview AI was compromised in a way that enabled any user to create an account. By exploiting this vulnerability, an unauthorised individual obtained entry to a repository of private code housing Clearview AI production credentials, keys to cloud storage containers containing 70,000 video samples, duplicates of its applications, and Slack tokens. A malicious actor who gains access to the training data can induce an arbitrary misclassification in the deployed model. |
Attack on Machine Translation Service - Google Translate, Bing Translator, and Systran Translate \n Incident Date: 30 April 2020 \n Actor: Berkeley Artificial Intelligence Research \n Target: Google Translate, Bing Translator, Systran Translate. \n (Wallace, Stern and Song, 2021) | Machine translation services (e.g., Systran Translate, Google Translate, and Bing Translator) offer user interfaces and APIs that are accessible to the public. These public endpoints were used by a research group at UC Berkeley to generate a replicated model whose translation quality was close to that of production-ready models. In addition to illustrating the functional theft of intellectual property from a black-box system, they effectively transferred adversarial examples to the actual production services using the replicated model. Adversarial inputs effectively induce targeted word shifts, generate profane outputs, and result in deleted sentences on the websites of Systran Translate and Google Translate. |
Camera Hijack Attack on Facial Recognition System \n Incident Date: 2020 \n Reporter: Ant Group AISEC Team \n Actor: Two individuals \n Target: Shanghai government tax office’s facial recognition service \n (Vennam et al., 2021) | By circumventing the conventional model of live facial recognition authentication, this form of camera hijack attack grants adversaries access to privileged systems and allows for the impersonation of victims. \n This assault was used by two individuals in China to infiltrate the tax system of the local government. They established a fictitious subsidiary corporation and issued tax system invoices to fictitious clients. The scheme was initiated by the individuals in 2018 and resulted in the fraudulent acquisition of $77 million. |
Bypassing Cylance’s AI Malware Detection \n Incident Date: 7 September 2019 \n Actor: Skylight Cyber \n Target: CylancePROTECT, Cylance Smart Antivirus \n (Lucas et al., 2021) | To circumvent the detection of Cylance’s AI Malware detector when appended to a malicious file, a universal bypass string was developed by Skylight researchers. |
VirusTotal Poisoning \n Incident Date: 2020 \n Reporter: McAfee Advanced Threat Research \n Actor: Unknown \n Target: VirusTotal \n (Ranade et al., 2021) | Extraordinary reports of a specific ransomware family increased, according to McAfee Advanced Threat Research. A rapid influx of ransomware samples from that specific family was disclosed via a well-known virus-sharing platform, according to the findings of the case investigation. Subsequent inquiry unveiled that the samples were equivalent in terms of string similarity and code similarity, with the degree of similarity ranging from 98 to 74%. Remarkably, the duration required to construct each sample was identical. Scientists discovered, upon further investigation, that the original file had been altered to contain aberrant variants using the metamorphic code manipulating tool “metame.” Although not consistently executable, the variants remain within the ransomware family until they are identified. |
Botnet Domain Generation Algorithm (DGA) Detection Evasion \n Incident Date: 2020 \n Actor: Palo Alto Networks AI Research Team \n Target: Palo Alto Networks ML-based DGA detection module \n (Upadhyay, 2020) | Using a generic domain name mutation technique, the Security AI research team at Palo Alto Networks was able to circumvent a Convolutional Neural Network-based botnet Domain Generation Algorithm (DGA) detector. It is a technique for generic domain mutation that can circumvent the majority of ML-based DGA detection modules. The generic mutation technique circumvents the majority of ML-based DGA detection modules DGA and can be used to evaluate the robustness and efficacy of all DGA detection methods developed by industry security firms prior to their deployment in production. |
Evasion of Deep Learning Detector for Malware C&C Traffic \n Incident Date: 2020 \n Actor: Palo Alto Networks AI Research Team \n Target: Palo Alto Networks malware detection system \n (Novo and Morla, 2020) | A deep learning model was evaluated by the Security AI research team at Palo Alto Networks for the purpose of detecting malware command and control (C&C) traffic in HTTP traffic. Drawing inspiration from the publicly accessible article by Le et al., they constructed a model that exhibited comparable performance to the production model and was trained on an analogous dataset. Following this, adversarial samples were generated, the model was queried, and the adversarial sample was modified accordingly until the model was evaded. |
6. Client interview insights
The study also integrated insights from interviews with Grant Thornton clients across diverse sectors, including private healthcare, insurance, fund management, law firms, hotel chains, global banks, national newspapers and leading academic researchers from both UK and USA. These organisations, employing between 1,000 to over 20,000 individuals, operate in the UK market.
Interviews were conducted to assess clients’ readiness and expertise in developing, managing and deploying AI products across the AI lifecycle, focusing on topics such as risk management, data integrity, incident response, model security, regulatory compliance, and threat intelligence. These discussions were intended to ground the assessment in practical experience, ensuring it accurately represented the current challenges these organisations are considering.
The table following this section lists clients and academics interviewed, denoting only 5 client organisations and 2 academics participated due to availability or internal policies. Organisations are anonymised with a unique prefix used in subsequent references. Out of 17 organisations invited to interview for the study, only 7 were interviewed either due to time constraints and/or organisational policies leading to withdrawal. Among these, only one organisation has currently deployed an AI solution within its organisational IT framework.
Organisation (prefix) | Interviewed | Position |
Healthcare and insurance (HI1) | Y | Group Security Director |
Cyber security specialist (CS1) | Y | N/A |
Global Top 50 bank (BK1) | Y | Global CISO |
National newspaper (NN1) | Y | Editor responsible for AI |
Pension investment fund manager (PI1) | Y | Global CISO |
Cyber security expert UK academic (AC1) | Y | Professor of Computer Science |
Cyber security expert USA government (AC2) | Y | AI Cyber security expert |
Application of AI / ML
The interviewees were queried on the current use of AI or ML within their organisation. Except for one banking client (BK1), all confirmed a lack of AI or ML applications in their workflows, excluding standard AI tools such as Microsoft’s Copilot or ChatGPT 3.5 within Microsoft Azure enterprise subscription services. One client specializing in cybersecurity (CS1) is deliberately developed policies to postpone the adoption and incorporation of cutting-edge AI solutions until the cyber security industry develops more robust cybersecurity solutions for AI systems with proven track record.
Data integrity and protection
Acknowledging the importance of data quality and integrity for AI model functionality, the clients were inquired about their data control measures specific to AI. It emerged that specialized controls for AI data integrity are not yet in place. The exception is BK1, who presumes that existing Data Loss Prevention systems would flag significant data breaches, although this has not been practically tested. BK1 has begun to create a risk classification system addressing AI-specific cybersecurity risks like data privacy and poisoning.
AI model security
The clients, including BK1, have not yet focused on the security of AI or ML models. BK1, however, has indicated plans to enforce minimum standards in their commercial agreements to enable legal recourse in the case of a security breach.
Risk assessment and management
Senior leadership acknowledged the looming importance of cyber security risks to AI and is seeking solutions from startups and corporate entities. HI1 has initiated a risk assessment and management process for AI systems in 2023, utilizing expertise from cybersecurity architects and the Group CISO. Theyíre seeking guidance from enterprise entities like Microsoft and MITRE AI, as well as recent papers from the UK’s NCSC. HI1 and PI1 acknowledge the need to advance their cybersecurity measures for AI and are engaging with specialized startups for solutions. However, they are aware of their limitations in assessing these services’ efficacy. To address this, they are collaborating with academic experts to evaluate the requirements and effectiveness of proposed solutions. BK1, meanwhile, has created an extensive risk taxonomy to address specific AI risks, including the ‘black box’ effect, model inversion, data privacy, poisoning, and the overall AI lifecycle. However, most organisations are focused on meeting existing regulations and security standards, with AI implementation not yet a priority.
Incident response
None of the clients have not yet developed incident response plans specifically for cybersecurity incidents affecting AI systems.
Regulatory compliance and standard
The consensus among various stakeholders is a lack of awareness of any specific cybersecurity regulations tailored to AI. There is an acknowledgment of the extensive existing cybersecurity regulations, which, despite their breadth, do not address AI’s unique challenges. BK1 and PI1 are monitoring for the release of targeted AI cybersecurity regulations and standards, which they anticipate will prompt actionable changes within the industry.
Appendix 1 - References
Anisetti, M. et al. (2020) ëSecurity threat landscapeí, White Paper Security Threats [Preprint].
Abbas, R. et al. (2023) Artificial Intelligence (AI) in Cybersecurity: A Socio-Technical Research Roadmap. The Alan Turing Institute. Available at: https://www.turing.ac.uk/sites/default/files/2023-11/ai_in_cybersecurity.pdf (Accessed: 3 January 2024).
Anthropic (2023a) Anthropicís Responsible Scaling Policy, Version 1.0.
Anthropic (2023b) Frontier Model Security. Available at: https://www.anthropic.com/news/frontier-model-security#entry:146893@1:url (Accessed: 7 February 2024).
Anthropic (2023c) Frontier Threats Red Teaming for AI Safety. Available at: https://www.anthropic.com/news/frontier-threats-red-teaming-for-ai-safety#entry:146918@1:url (Accessed: 7 February 2024).
Apruzzese, G. et al. (2023) ëìReal Attackers Donít Compute Gradientsî: Bridging the Gap Between Adversarial ML Research and Practiceí, in 2023 IEEE Conference on Secure and Trustworthy Machine Learning (SaTML). 2023 IEEE Conference on Secure and Trustworthy Machine Learning (SaTML), Raleigh, NC, USA: IEEE, pp. 339ñ364. Available at: https://doi.org/10.1109/SaTML54575.2023.00031.
AWS (2024) Cloud Adoption Framework for Artificial Intelligence, Machine Learning, and Generative AI - Whitepaper.
BÈcue, A., PraÁa, I. and Gama, J. (2021) ëArtificial intelligence, cyber-threats and Industry 4.0: challenges and opportunitiesí, Artificial Intelligence Review, 54(5), pp. 3849ñ3886. Available at: https://doi.org/10.1007/s10462-020-09942-2.
Bouacida, N. and Mohapatra, P. (2021) ëVulnerabilities in Federated Learningí, IEEE Access, 9, pp. 63229ñ63249. Available at: https://doi.org/10.1109/ACCESS.2021.3075203.
Boulemtafes, A., Derhab, A. and Challal, Y. (2020) ëA review of privacy-preserving techniques for deep learningí, Neurocomputing, 384, pp. 21ñ45. Available at: https://doi.org/10.1016/j.neucom.2019.11.041.
Bradley, P. (2020) ëRisk management standards and the active management of malicious intent in artificial superintelligenceí, AI & SOCIETY, 35(2), pp. 319ñ328. Available at: https://doi.org/10.1007/s00146-019-00890-2.
Brammer, Z. (2023) How Does Access Impact Risk. Institute for Security and Technology.
Brundage, M. et al. (2018) ëThe Malicious Use of Artificial Intelligence: Forecasting, Prevention, and Mitigationí. arXiv. Available at: https://doi.org/10.48550/arXiv.1802.07228.
Carlo, A. et al. (2023) ëThe importance of cybersecurity frameworks to regulate emergent AI technologies for space applicationsí, Journal of Space Safety Engineering, 10(4), pp. 474ñ482. Available at: https://doi.org/10.1016/j.jsse.2023.08.002.
Chang, C.-L. et al. (2020) ëEvaluating Robustness of AI Models against Adversarial Attacksí, in Proceedings of the 1st ACM Workshop on Security and Privacy on Artificial Intelligence. New York, NY, USA: Association for Computing Machinery (SPAI í20), pp. 47ñ54. Available at: https://doi.org/10.1145/3385003.3410920.
Chen, S., Pande, A. and Mohapatra, P. (2014) ëSensor-assisted facial recognition: an enhanced biometric authentication system for smartphonesí, in Proceedings of the 12th annual international conference on Mobile systems, applications, and services. New York, NY, USA: Association for Computing Machinery (MobiSys í14), pp. 109ñ122. Available at: https://doi.org/10.1145/2594368.2594373.
Chiang, F. and Gairola, D. (2018) ëInfoClean: Protecting Sensitive Information in Data Cleaningí, Journal of Data and Information Quality, 9(4), p. 22:1-22:26. Available at: https://doi.org/10.1145/3190577.
Choudhury, A. and Asan, O. (2020) ëRole of Artificial Intelligence in Patient Safety Outcomes: Systematic Literature Reviewí, JMIR Medical Informatics, 8(7), p. e18599. Available at: https://doi.org/10.2196/18599.
Cohere (2023) The State of AI Security, Context by Cohere. Available at: https://txt.cohere.com/the-state-of-ai-security (Accessed: 28 March 2024).
Cuppens, F., Cuppens-Boulahia, N. and Garcia-Alfaro, J. (2019) ëMisconfiguration Management of Network Security Componentsí. arXiv. Available at: https://doi.org/10.48550/arXiv.1912.07283.
Ding, J., Cen, Y. and Wei, X. (2023) ëUsing Large Language Model to Solve and Explain Physics Word Problems Approaching Human Levelí. arXiv. Available at: https://doi.org/10.48550/arXiv.2309.08182.
Djenna, A. et al. (2023) ëArtificial Intelligence-Based Malware Detection, Analysis, and Mitigationí, Symmetry, 15(3), p. 677. Available at: https://doi.org/10.3390/sym15030677.
ETSI (2022) Securing Artificial Intelligence (SAI); AI Threat Ontology. Available at: https://www.etsi.org/deliver/etsi_gr/SAI/001_099/001/01.01.01_60/gr_SAI001v010101p.pdf (Accessed: 3 January 2024).
EU General Secretariat of the Council (2022) Proposal for a Regulation of the European Parliament and of the Council laying down harmonised rules on artificial intelligence (Artificial Intelligence Act) and amending certain Union legislative acts. Interinstitutional File. Hart Publishing.
European Union Agency for Cybersecurity. (2020) AI cybersecurity challenges: threat landscape for artificial intelligence. LU: Publications Office. Available at: https://data.europa.eu/doi/10.2824/238222 (Accessed: 29 February 2024).
European Union Agency for Cybersecurity. (2021a) Securing machine learning algorithms. LU: Publications Office. Available at: https://data.europa.eu/doi/10.2824/874249 (Accessed: 9 February 2024).
European Union Agency for Cybersecurity. (2021b) Securing machine learning algorithms. LU: Publications Office. Available at: https://data.europa.eu/doi/10.2824/874249 (Accessed: 7 February 2024).
European Union Agency for Cybersecurity. (2023a) Cybersecurity and privacy in AI: forecasting demand on electricity grids. LU: Publications Office. Available at: https://data.europa.eu/doi/10.2824/92851 (Accessed: 29 February 2024).
European Union Agency for Cybersecurity. (2023b) Cybersecurity of AI and Standardisation. LU: Publications Office. Available at: https://data.europa.eu/doi/10.2824/277479 (Accessed: 16 December 2023).
European Union Agency for Cybersecurity. (2023c) Standardisation in support of the cybersecurity of AI. LU: Publications Office. Available at: https://data.europa.eu/doi/10.2824/277479 (Accessed: 29 February 2024).
Ferrer, X. et al. (2021) ëBias and Discrimination in AI: A Cross-Disciplinary Perspectiveí, IEEE Technology and Society Magazine, 40(2), pp. 72ñ80. Available at: https://doi.org/10.1109/MTS.2021.3056293.
Google (2023) Introducing Googleís Secure AI Framework, Google. Available at: https://blog.google/technology/safety-security/introducing-googles-secure-ai-framework/ (Accessed: 7 February 2024).
Greshake, K. et al. (2023) ëNot What Youíve Signed Up For: Compromising Real-World LLM-Integrated Applications with Indirect Prompt Injectioní, in Proceedings of the 16th ACM Workshop on Artificial Intelligence and Security. New York, NY, USA: Association for Computing Machinery (AISec í23), pp. 79ñ90. Available at: https://doi.org/10.1145/3605764.3623985.
Gupta, M. et al. (2023) ëFrom chatgpt to threatgpt: Impact of generative ai in cybersecurity and privacyí, IEEE Access [Preprint]. Available at: https://doi.org/10.1109/ACCESS.2023.3300381.
Haakman, M. et al. (2021) ëAI lifecycle models need to be revised: An exploratory study in Fintechí, Empirical Software Engineering, 26, pp. 1ñ29. Available at: https://doi.org/10.1007/s10664-021-09993-1.
Horvitz, E. (2022) Artificial Intelligence and Cybersecurity: Rising Challenges and Promising Directions. Statement. U.S. Senate Armed Services Subcommittee on Cybersecurity: Microsoft.
House of Commons (2023) The governance of artificial intelligence: interim report: Government response to the Committeeís Ninth report. Policy Paper HC 248. UK Government.
House, T.W. (2023) Executive Order on the Safe, Secure, and Trustworthy Development and Use of Artificial Intelligence, The White House. Available at: https://www.whitehouse.gov/briefing-room/presidential-actions/2023/10/30/executive-order-on-the-safe-secure-and-trustworthy-development-and-use-of-artificial-intelligence/ (Accessed: 29 January 2024).
Hu, Y. et al. (2021) ëArtificial Intelligence Security: Threats and Countermeasuresí, ACM Computing Surveys, 55(1), p. 20:1-20:36. Available at: https://doi.org/10.1145/3487890.
Huang, C. et al. (2023) ëAn Overview of Artificial Intelligence Ethicsí, IEEE Transactions on Artificial Intelligence, 4(4), pp. 799ñ819. Available at: https://doi.org/10.1109/TAI.2022.3194503.
Joint Task Force Transformation Initiative (2012) Guide for conducting risk assessments. 0 edn. NIST SP 800-30r1. Gaithersburg, MD: National Institute of Standards and Technology, p. NIST SP 800-30r1. Available at: https://doi.org/10.6028/NIST.SP.800-30r1.
Khan, A.A. et al. (2023) ëAI Ethics: An Empirical Study on the Views of Practitioners and Lawmakersí, IEEE Transactions on Computational Social Systems, 10(6), pp. 2971ñ2984. Available at: https://doi.org/10.1109/TCSS.2023.3251729.
Laborde, R. et al. (2020) ëA User-Centric Identity Management Framework based on the W3C Verifiable Credentials and the FIDO Universal Authentication Frameworkí, in 2020 IEEE 17th Annual Consumer Communications & Networking Conference (CCNC). 2020 IEEE 17th Annual Consumer Communications & Networking Conference (CCNC), pp. 1ñ8. Available at: https://doi.org/10.1109/CCNC46108.2020.9045440.
Ladisa, P. et al. (2023) ëJourney to the Center of Software Supply Chain Attacksí, IEEE Security & Privacy, 21(6), pp. 34ñ49. Available at: https://doi.org/10.1109/MSEC.2023.3302066.
Le, H. et al. (2018) ëURLNet: Learning a URL Representation with Deep Learning for Malicious URL Detectioní. arXiv. Available at: https://doi.org/10.48550/arXiv.1802.03162.
Lehne, M. et al. (2019) ëWhy digital medicine depends on interoperabilityí, npj Digital Medicine, 2(1), pp. 1ñ5. Available at: https://doi.org/10.1038/s41746-019-0158-1.
Li, C., Zhang, M. and He, Y. (2022) ëThe Stability-Efficiency Dilemma: Investigating Sequence Length Warmup for Training GPT Modelsí, Advances in Neural Information Processing Systems, 35, pp. 26736ñ26750.
Li, Y. et al. (2021) ëDeepPayload: Black-box Backdoor Attack on Deep Learning Models through Neural Payload Injectioní, in 2021 IEEE/ACM 43rd International Conference on Software Engineering (ICSE). 2021 IEEE/ACM 43rd International Conference on Software Engineering (ICSE), pp. 263ñ274. Available at: https://doi.org/10.1109/ICSE43902.2021.00035.
Lucas, K. et al. (2021) ëMalware Makeover: Breaking ML-based Static Analysis by Modifying Executable Bytesí, in Proceedings of the 2021 ACM Asia Conference on Computer and Communications Security. New York, NY, USA: Association for Computing Machinery (ASIA CCS í21), pp. 744ñ758. Available at: https://doi.org/10.1145/3433210.3453086.
Luong, N. and Arnold, Z. (2021) ëChinaís Artificial Intelligence Industry Allianceí, Center for Security and Emerging Technology. Available at: https://cset.georgetown.edu/publication/chinas-artificial-intelligence-industry-alliance/ (Accessed: 3 March 2024).
Majeed, A. and Hwang, S.O. (2023) ëWhen AI Meets Information Privacy: The Adversarial Role of AI in Data Sharing Scenarioí, IEEE Access, 11, pp. 76177ñ76195. Available at: https://doi.org/10.1109/ACCESS.2023.3297646.
Marshall, A. et al. (2024) Securing the Future of AI and ML at Microsoft. Available at: https://learn.microsoft.com/en-us/security/engineering/securing-artificial-intelligence-machine-learning (Accessed: 7 February 2024).
METI (2024) Launch of AI Safety Institute, Ministry of Economy, Trade and Industry. Available at: https://www.meti.go.jp/english/press/2024/0214_001.html (Accessed: 3 March 2024).
Mirsky, Y. et al. (2023) ëThe Threat of Offensive AI to Organizationsí, Computers and Security, 124(C). Available at: https://doi.org/10.1016/j.cose.2022.103006.
Mˆkander, J. et al. (2023) ëAuditing large language models: a three-layered approachí, AI and Ethics [Preprint]. Available at: https://doi.org/10.1007/s43681-023-00289-2.
MSIT (2024) MSIT announce strategy to realize trustworthy artificial intelligence. Available at: https://www.msit.go.kr/eng/bbs/view.do?sCode=eng&mId=4&mPid=2&pageIndex=&bbsSeqNo=42&nttSeqNo=509&searchOpt=ALL&searchTxt (Accessed: 3 March 2024).
Narayanan, B.N. and Davuluru, V.S.P. (2020) ëEnsemble Malware Classification System Using Deep Neural Networksí, Electronics, 9(5), p. 721. Available at: https://doi.org/10.3390/electronics9050721.
Neff, G. and Nagy, P. (2016) ëTalking to Bots: Symbiotic Agency and the Case of Tayí, International Journal of Communication, 10, pp. 4915ñ4931.
Nguyen, D.C. et al. (2021) ëFederated Learning for Internet of Things: A Comprehensive Surveyí, IEEE Communications Surveys & Tutorials, 23(3), pp. 1622ñ1658. Available at: https://doi.org/10.1109/COMST.2021.3075439.
Nieuwenhuis, L.J.M., Ehrenhard, M.L. and Prause, L. (2018) ëThe shift to Cloud Computing: The impact of disruptive technology on the enterprise software business ecosystemí, Technological Forecasting and Social Change, 129, pp. 308ñ313. Available at: https://doi.org/10.1016/j.techfore.2017.09.037.
NIST (2023a) Artificial Intelligence Risk Management Framework (AI RMF 1.0). NIST AI 100-1. Gaithersburg, MD: National Institute of Standards and Technology (U.S.), p. NIST AI 100-1. Available at: https://doi.org/10.6028/NIST.AI.100-1.
NIST (2023b) U.S. Artificial Intelligence Safety Institute, NIST. Available at: https://www.nist.gov/artificial-intelligence/artificial-intelligence-safety-institute (Accessed: 3 March 2024).
Novo, C. and Morla, R. (2020) ëFlow-based Detection and Proxy-based Evasion of Encrypted Malware C2 Trafficí, in Proceedings of the 13th ACM Workshop on Artificial Intelligence and Security. New York, NY, USA: Association for Computing Machinery (AISecí20), pp. 83ñ91. Available at: https://doi.org/10.1145/3411508.3421379.
NVIDIA (2024) NVIDIA AI Cybersecurity, NVIDIA. Available at: https://www.nvidia.com/en-gb/industries/cybersecurity/ (Accessed: 7 February 2024).
OpenAI (2023) OpenAIís Approach to Frontier Risk. Available at: https://openai.com/global-affairs/our-approach-to-frontier-risk (Accessed: 7 February 2024).
OpenAI (2024) OpenAI Platform. Available at: https://platform.openai.com (Accessed: 7 February 2024).
OWASP (2024) AI Security and Privacy Guide. Available at: https://owasp.org/www-project-ai-security-and-privacy-guide/ (Accessed: 9 February 2024).
Page, M.J. et al. (2021) ëPRISMA 2020 explanation and elaboration: updated guidance and exemplars for reporting systematic reviewsí, BMJ, 372, p. n160. Available at: https://doi.org/10.1136/bmj.n160.
Ranade, P. et al. (2021) ëGenerating Fake Cyber Threat Intelligence Using Transformer-Based Modelsí, in 2021 International Joint Conference on Neural Networks (IJCNN). 2021 International Joint Conference on Neural Networks (IJCNN), pp. 1ñ9. Available at: https://doi.org/10.1109/IJCNN52387.2021.9534192.
Rodrigues, R. (2020) ëLegal and human rights issues of AI: Gaps, challenges and vulnerabilitiesí, Journal of Responsible Technology, 4, p. 100005. Available at: https://doi.org/10.1016/j.jrt.2020.100005.
Shevlane, T. et al. (2023) ëModel evaluation for extreme risksí. arXiv. Available at: https://doi.org/10.48550/arXiv.2305.15324.
Silva, D.D. and Alahakoon, D. (2022) ëAn artificial intelligence life cycle: From conception to productioní, Patterns, 3(6). Available at: https://doi.org/10.1016/j.patter.2022.100489.
Sivaram, J. et al. (2022) ëAdversarial Machine Learning: The Rise in AI-Enabled Crimeí. Rochester, NY. Available at: https://doi.org/10.2139/ssrn.4155496.
Torkura, K.A. et al. (2020) ëCloudStrike: Chaos Engineering for Security and Resiliency in Cloud Infrastructureí, IEEE Access, 8, pp. 123044ñ123060. Available at: https://doi.org/10.1109/ACCESS.2020.3007338.
Upadhyay, S. (2020) ëDomain generation algorithm (DGA) detectioní.
UK Department for Science, Innovation and Technology (2023a) AI regulation: a pro-innovation approach. Command Paper 815. UK Government. Available at: https://www.gov.uk/government/publications/ai-regulation-a-pro-innovation-approach (Accessed: 18 December 2023).
UK Department for Science, Innovation and Technology (2023b) AI Safety Institute: overview. Policy Paper. UK Government. Available at: https://www.gov.uk/government/publications/ai-safety-institute-overview (Accessed: 18 December 2023).
UK National Cyber Security Centre and US Cybersecurity and Infrastructure Security Agency (2023) Guidelines for secure AI system development. Guideline. UK Government.
Usynin, D. et al. (2021) ëAdversarial interference and its mitigations in privacy-preserving collaborative machine learningí, Nature Machine Intelligence, 3(9), pp. 749ñ758. Available at: https://doi.org/10.1038/s42256-021-00390-3.
Valizadeh, M. and Berger, M. (2023) ëSearch-Based Regular Expression Inference on a GPUí, Proceedings of the ACM on Programming Languages, 7(PLDI), p. 160:1317-160:1339. Available at: https://doi.org/10.1145/3591274.
Vassilev, A. (2024) Adversarial Machine Learning: A Taxonomy and Terminology of Attacks and Mitigations. NIST AI NIST AI 100-2e2023. Gaithersburg, MD: National Institute of Standards and Technology, p. NIST AI NIST AI 100-2e2023. Available at: https://doi.org/10.6028/NIST.AI.100-2e2023.