Understanding UK AI R&D commercialisation and the role of standards: Executive Summary
Published 12 May 2022
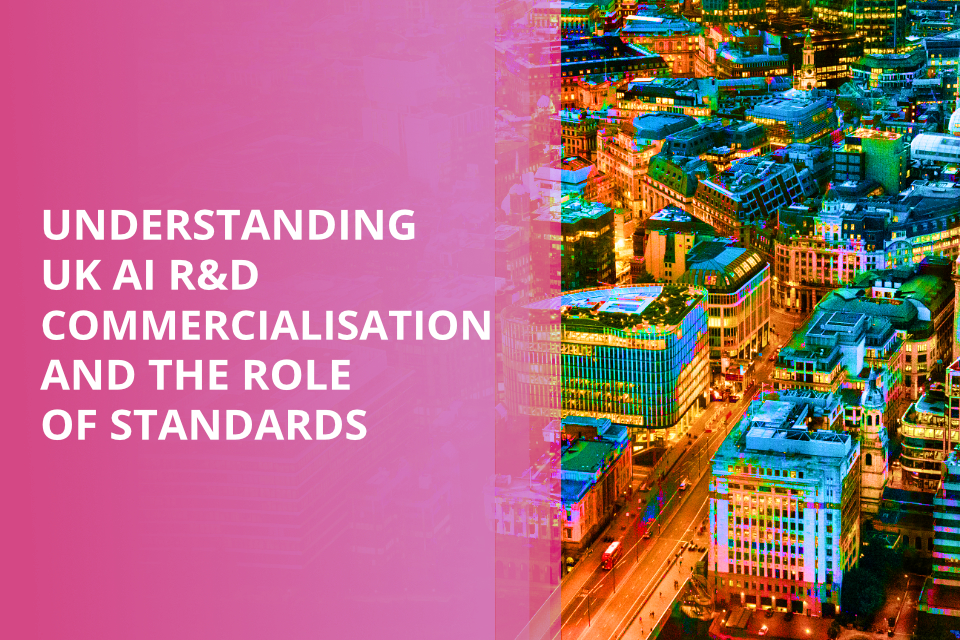
About this research
The Department for Digital, Culture, Media and Sport’s (DCMS) upcoming Digital Strategy and the National AI Strategy (published in September 2021) both acknowledge the transformational potential of AI technology to increase productivity and create long-term economic growth. Despite this promise, there are a number of barriers that potentially hamper the commercialisation of AI Research & Development (R&D). Understanding the ways in which AI R&D commercialisation can be supported is the core purpose of this research.
A key goal of this report is to help teams from across government, public funding bodies, universities’ technology transfer offices, industry and other associated organisations understand what steps can be taken to support and increase the commercialisation of AI R&D in the UK. We present several considerations for policymakers and other stakeholders to address barriers to the commercialisation of AI R&D that emerged through the research.
This report identifies the most prevalent ways, or ‘routes’, by which AI R&D is commercialised in the UK:
- University spinouts: businesses that grow out of a university research project, which attempt to transform research into a commercial product or service;
- Startups: businesses in the early stages of operations, exploring a new business model, product or service;
- Large firms that commercialise AI R&D: firms that commercialise AI R&D: ‘Big Tech firms’ [footnote 1], and also other large technology companies such as ARM, Graphcore, IBM, Netflix and Twitter; and,
- Direct hire and joint tenure arrangements: relationships between industry and academia that allow for a back and forth flow of AI talent between the two.
Taxonomy of commercialisation routes
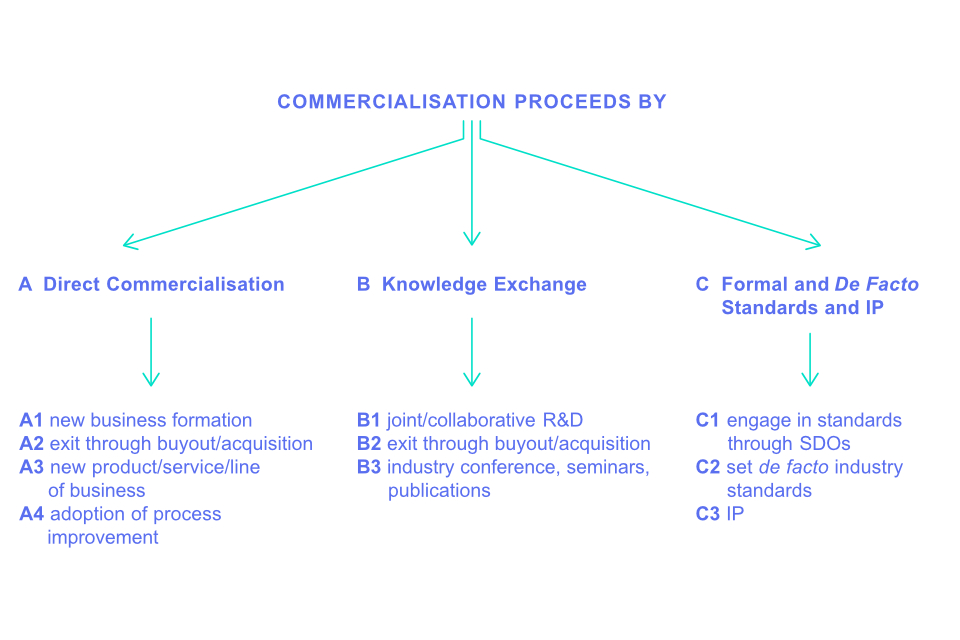
The report explores the main enablers, barriers and challenges for AI commercialisation through the specific routes above, and also generally across the commercialisation process as a whole. For each of these routes we investigated the key ‘enabling institutions’ (such as The Alan Turing Institute and universities’ Technology Transfer Offices), the role of public funding (Innovate UK, UKRI, etc.) and private funding (from ‘angel’ and venture capital investors).
Other issues that receive particular focus are:
- The role of Standards Development Organisations (SDOs). The development of technical standards for AI, though at a nascent stage, may potentially have an impact on the commercialisation of AI R&D in a similar way to the impact technical standards had on other digital and emerging technologies such as mobile.
- AI in healthcare and the life sciences. UK businesses have seen particular success with applied AI products in this area, in spite of the manifest challenges presented in such a ‘high stakes’ sector.
We derived insights through four strands of research:
- The development of a taxonomy of the ways in which AI R&D is commercialised, or ‘commercialisation routes’;
- Analysis of data sources giving insight into the activity of UK AI businesses;
- Subsequent comparison with AI commercialisation activity in eight other countries: the United States, Canada, China, France, Germany, Israel, Japan, and South Korea;
- Over 40 interviews that were conducted with representatives from ten categories of stakeholder.
Overview of the commercialisation process for AI R&D, including enabling institutions, key barriers and enablers, resources
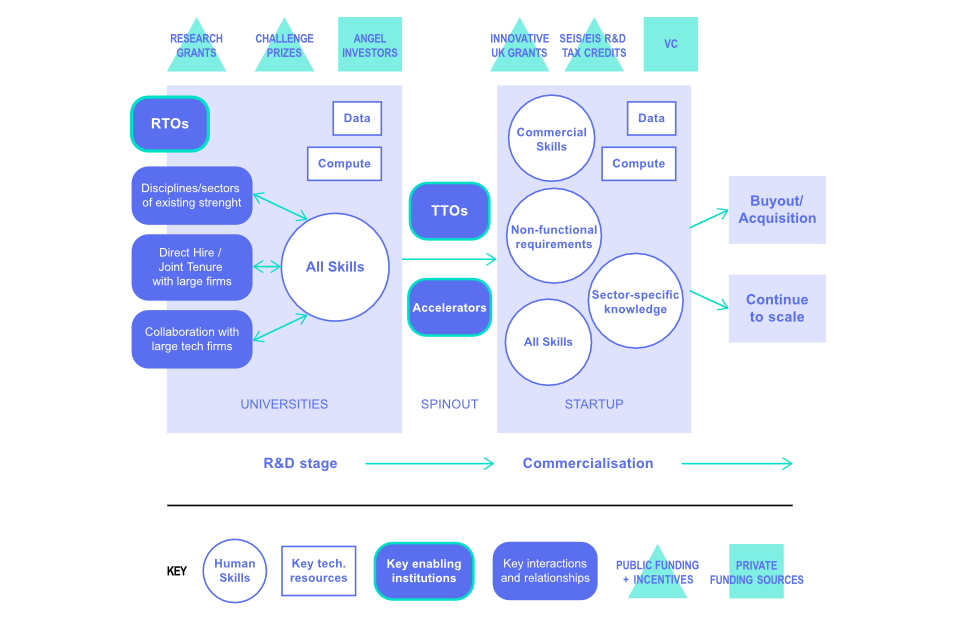
Key findings
We identified the following key themes:
The value of AI comes from its application to an existing problem. AI techniques are rarely products in themselves, but create value in the marketplace when applied to a problem in a business or industry sector.
- Businesses seeking to implement an AI solution to a real-world problem require access to sufficient compute resources (computing capability afforded by hardware, supercomputers, data centres, etc.).
- Commercialisation of AI R&D depends on the availability of sector-specific data. The UK Biobank and NHS genomics dataset are good examples of such datasets, and also the digitalisation of existing data which can make AI commercialisation possible.
AI businesses require a broad set of commercial and sector-specific skills in addition to technical AI skills.
- To successfully commercialise AI R&D, businesses require multi-skilled teams that combine technical expertise with other capabilities, such as commercial business experience, knowledge of applicable regulations and law, and experience of addressing customer needs.
- Academics or ‘research-founders’, being specialists in the technicalities and in designing AI, tend to be ill-equipped to navigate these additional commercial or sector-specific challenges.
The UK has successfully commercialised AI in challenging ‘high-stakes sectors.
- In high stakes sectors such as health, security, financial services, transport and nuclear energy, poor product execution can cause immeasurable harm. AI systems need to go through an extended period of training and testing before they pass competency and safety assessments before being deployed. Accordingly, financial success and profit take some time to materialise, which presents a challenge for potential investors.
- Despite these challenges, the UK has notable commercial successes in applying AI in sectors of existing strength (e.g. healthcare, pharmaceuticals, financial technology or ‘fintech’).
Universities’ equity share in their AI spinouts presents an important commercial barrier. Universities are the locus of AI R&D in the UK, and spinouts present the most direct route by which that R&D can be commercialised. However, the value presented by university R&D may not be fully realised owing to large equity shares being retained by the parent university.
- In the spinout process, research-founders face challenges negotiating intellectual property (IP) and equity shares with Technology Transfer Offices (TTOs). Large equity shares retained by a university can discourage researchers from pursuing spinouts by reducing the personal financial incentive to do so, and also the attractiveness of the business to private investors.
- In the US, universities take a far smaller share of spinout equity, an arrangement which US-based venture capital investors (VC) are more used to. The large equity share retained by UK universities presents a barrier to future investment in UK spinouts by VC.
Prevalence of channel A1, New business formation through university funding (selected countries)
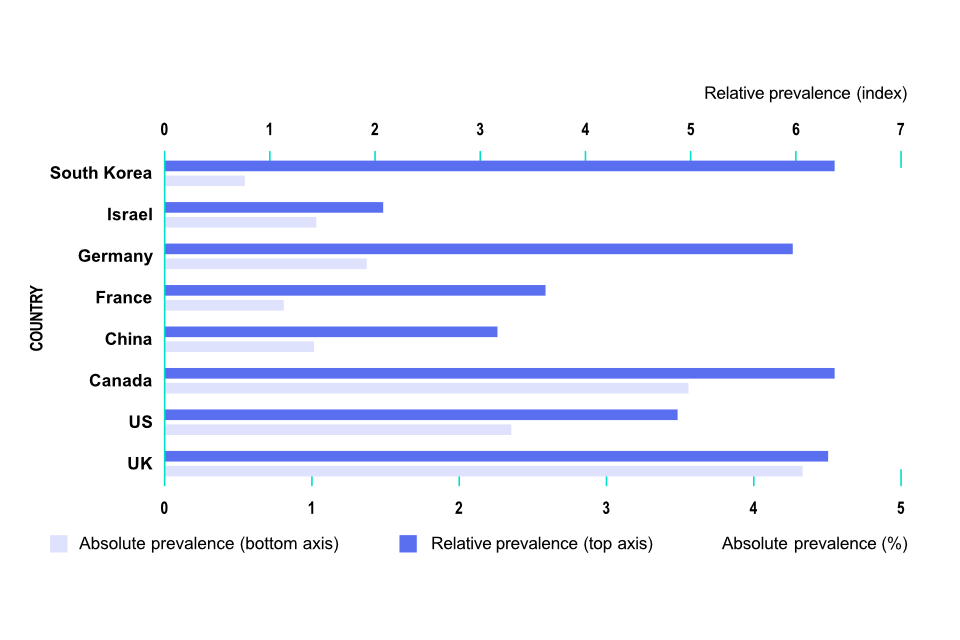
There is a significant flow of AI talent from universities to large technology firms. Interviews confirm that UK AI talent leaving academia for employment in large technology firms is a reality, and this is skewing the landscape of AI commercialisation.
- Graduates and researchers are highly incentivised to take up positions at large technology companies. This occurs in part because of earning potential.
- Other influencing factors are that large technology companies provide access to both the compute resources necessary to develop AI at scale, and the large datasets that are a fundamental component of AI development.
- Researchers, who might have founded a spinout, may instead choose to work for a large technology company. Contracts with large technology companies may place restrictions on the activities that the most talented AI researchers can pursue.
- High competition for AI talent makes it difficult for smaller businesses to recruit and retain AI talent.
Private and public funding are associated with differing outcomes for businesses. Private investment is largely focussed on areas of R&D that can quickly progress to commercialisation, whereas R&D supported by public investment can take a more long-term approach to commercialisation.
- Plausible explanations for this include the relative scarcity of private investors that are willing to fund R&D at a low level of tech readiness, and that public bodies have more ‘patient’ funding available given the positive externalities associated with many of these merit goods.
- Criteria for public funding awards may not be well aligned with commercial considerations and market needs.
Prevalence of channel A3a, Development of new products/services using public funding (selected countries)
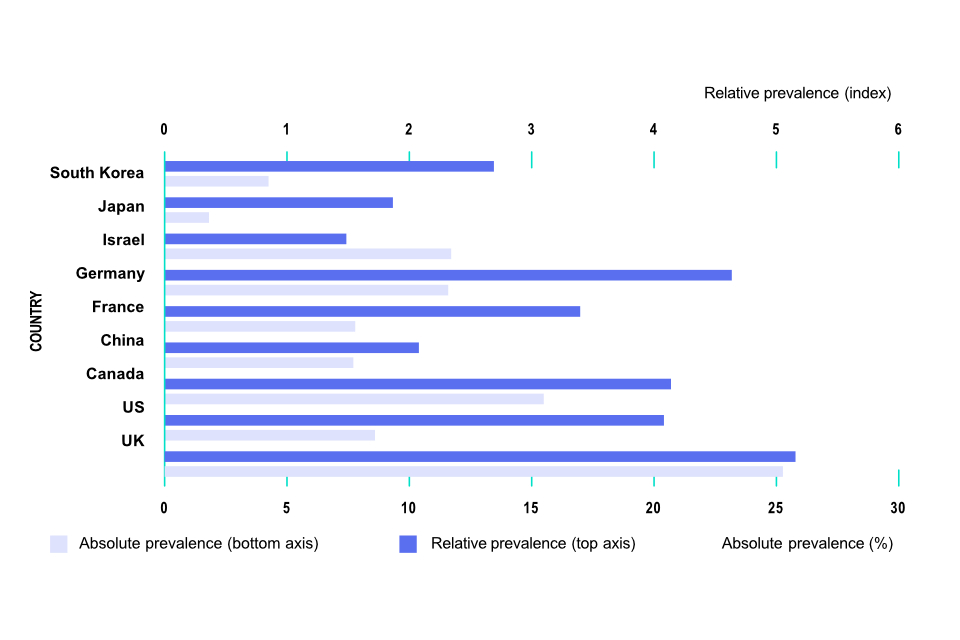
Prevalence of channel A3b, Development of new products/services using private funding (selected countries)
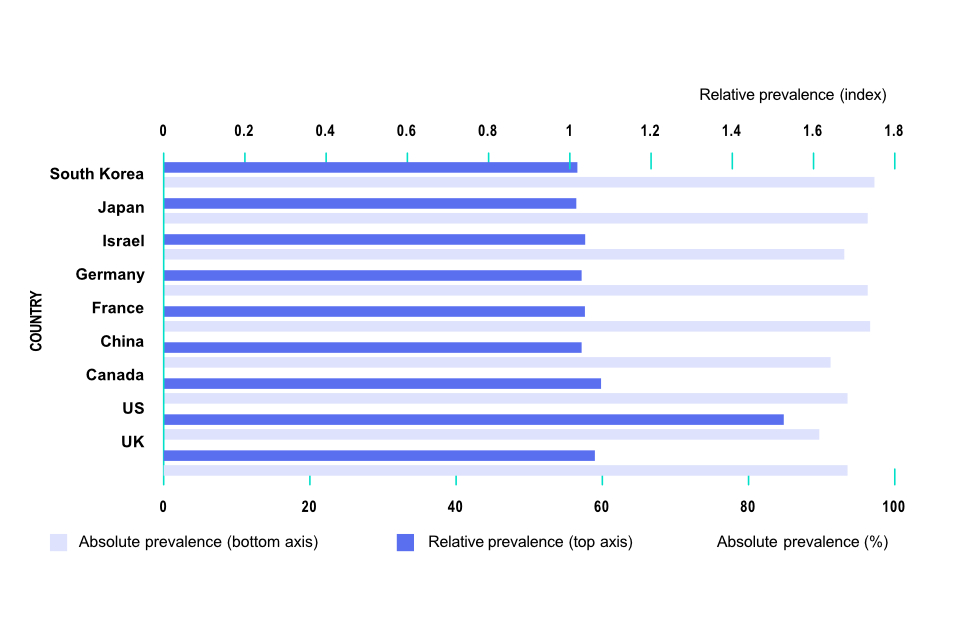
Securing intellectual property protection for AI R&D is difficult. Software patents are difficult to secure both in the UK and elsewhere, which makes realising the value in novel AI highly challenging.
- In the US, business process patents allow more of an AI’s value to be captured through IP. Several interviewees expressed the view that the IP regime in the UK isn’t well-suited to this. Further study of possible changes to IP law around software and business processes would be needed before specific recommendations could be made.
Without trust, attempts to commercialise AI will be ineffective. Some AI systems are not easily explainable. Fostering public and industry confidence that AI systems work as intended and do not cause unintended harm is highly important.
- The work of Standards Developing Organisations (SDOs) and the creation of technical standards for AI may play a central role in establishing trust amongst customers, users and between businesses in areas such as privacy, security, fairness and the removal of algorithmic bias.
- Technical standards may eventually support interoperability between the products and systems of different businesses, easing uptake of new products and thereby increasing their commercial value.
- However, interviewees noted that the potential impact of SDOs and technical standards on AI commercialisation is not yet well established; such technical standards are currently in the early stages of development.
- At present, our interviews indicated that engagement with SDOs is largely done by large technology companies.
-
Throughout this report, ‘Big Tech firms’ refers to Google (Alphabet), Amazon, Facebook (now Meta), Apple, and Microsoft. ↩