AI Barometer Part 4 - Transport and logistics
Published 17 December 2021
1. Key messages
Capital-heavy nature of sector affects scale of opportunity and challenge: The applications of data-driven technology across this sector are some of the broadest and most advanced across all sectors, and their scale means that marginal improvements can result in considerable gains in safety, energy efficiency, and in economic terms. However, the scale of these systems and the capital already invested in them means that technological improvements are typically slow and incremental.
The biggest opportunities are at system-level: Many of the most significant benefits of data-driven technology will only be felt in this sector when implemented on a system wide basis, requiring significant investment and time. For example, the use of automated traffic management systems would require sizeable changes to existing technology and operating procedures. Similarly, realising some of the efficiencies of autonomous vehicles, such as optimised driving approaches, may depend on a sufficient proportion of all road vehicles being autonomous.
Benefits will need behaviour change as well as tech progress: While data-driven technology has the potential to significantly reduce energy usage, this relationship is not clear cut. Improvements in mobility can result in rebound effects that lead to increased demand for mobility services - potentially cancelling out the environmental benefits such technologies can offer. Technology may not result in improved environmental outcomes unless it is accompanied by behaviour change - for example, passengers switching to more energy efficient forms of transport such as mass transit.
Safety-critical context poses challenges: The most significant risks associated with technologies in this sector centre around their safety, particularly in hybrid environments involving both human and machine-driven behaviour. Risks range from a lack of explainability of decision-making in robots and autonomous vehicles, to cyberattacks on critical physical infrastructure.
UK well-placed to lead on governance: Our panel expressed optimism about the state of the UK’s data ethics ecosystem and its capacity to take a different approach in competing on responsible governance and standard setting for applications in this sector.
Spotlight: Autonomous Vehicle Governance
Laws around vehicles have evolved to accommodate increasingly automated vehicles. All vehicles must meet a minimum set of regulatory, technical, and safety standards to gain ‘Type Approval’ from the UK’s Vehicles Certification Agency and so be permitted on public roads. In 2018 the Automated and Electric Vehicles Act passed into law, imposing statutory liability on vehicle owners and motor insurers for any damage caused by an automated vehicle when being operated in driverless mode.
The Centre for Connected and Autonomous Vehicles (CCAV) has tasked the Law Commission of England and Wales and the Scottish Law Commission with undertaking a more complete legal review looking to develop a new regulatory framework for automated vehicles. The Law Commissions have published three consultation papers on the topic and it is expected that a new Act will be put in front of parliament in early 2022. In the most recent consultation the Law Commissions have proposed two regulatory bodies: the first a pre-deployment regulator, tasked with ensuring the safety of new automated vehicles; and, the second, a safety and use regulator, responsible for tasks like auditing algorithms and analysing near misses.
Alongside this work, CCAV is working with the Centre for Data Ethics and Innovation (CDEI) to develop proposals focused on the ethical development and use of AVs in the future considering issues such as the data protection in the context of automated vehicles.
2. State of Play
The UK’s recent departure from the EU and reconfiguration of its trade policy, along with the surge in remotely-delivered services over the course of the pandemic, has underlined the importance of an effective transport and logistics infrastructure.
While self-driving vehicle technology is one of the most prominent applications of AI systems both in this sector and beyond, transport and logistics contexts provide a broad range of use cases for data-driven technologies that span well beyond in-vehicle applications. Other examples include transport system management, planning, navigation, ‘smart infrastructure’, and ‘Mobility as a Service’ (MaaS) offerings.
- Managing transport networks - The use of algorithmic systems in some transport contexts such as rail network management has a long history[footnote 1], but more substantial automation at the system level is becoming increasingly commonplace, for example in traffic control systems that use video, GPS, phone tower and telematics data to manage traffic signals, as is the case in the development of the European Railway Traffic Management System. Machine vision is particularly useful for automating data collection on transport system use - e.g. the volume and types of traffic on roads, or detecting traffic accidents. Meanwhile, adding sensors to transport environments can enable monitoring of environmental impacts such as air quality. Some AI systems even focus on ‘human traffic’ or queue flow, for example in transport hubs.
- Smart infrastructure and freight management - AI systems have a broad range of uses in the tracking, picking, packing[footnote 2], routing and physical handling of freight/cargo (both at port and inside ships [footnote 3]), as well as baggage[footnote 4] at travel terminals. Capacity sensors can detect space in a warehouse, parking lot or container, allowing for efficient routing and use of space, as well as detecting unauthorised container opening. As well as optimising physical logistics, these technologies can support efficient customs systems, by automating tax and duty calculations, identifying documentation requirements, and spotting errors or potentially fraudulent behaviour in customs declarations. More broadly, some providers offer blockchain-powered supply chain management solutions to track freight across multi-le.g. journeys.[footnote 5]
Spotlight: Automating customs systems
Israeli start up AiDock has produced a customs declaration solution which automates paper-based tasks in the import and export process. The AI-based solution offers a number of functions; it verifies pre-alerts for shipments, analyses shipment data to identify the correct Harmonised Systems (HS) code (a requirement for international shipments) as well as identifying mistakes in previous declarations. The solution calculates tax and duties due as well as identifying what documentation is required to ensure compliance at the border. A mailbox service flags and prioritises urgent tasks and a separate route optimisation tool calculates the best routes and rates for shipments. It is unclear how many customers AiDock has.
TradeLens, a blockchain solution jointly developed by IBM, A.P Moller and Maersk enables customs authorities on the platform to reduce the risk of fraudulent documentation by requiring blockchain verification for authenticity control. Member authorities include Canada, China, Jordan, Singapore, Russia and many others.
- Planning - data-driven technologies can help understand demand, trends, and flow of transport infrastructure usage, using ticketing and geolocation data and predictive analytics to identify future system needs.[footnote 6] Digital twins (whereby real-world systems are modelled or simulated digitally) are increasingly being used for supply chain management, predicting transport system use, or providing training environments for AV AI systems.
- In-vehicle technologies - data-driven technologies perform a wide variety of functions in current generations of automated vehicles, including perceiving their environment, predicting the behaviour of other vehicles and pedestrians, and making decisions such as when to turn, brake, or hand over control to a human driver. AVs are highly sensorised and collect large volumes of data about their environment, often sharing it to improve AI system training, environment mapping, and to coordinate with other AVs.[footnote 7] The role of data-driven technologies such as machine vision and predictive analytics is only expected to increase in these contexts. AI systems can also be integrated into normal vehicles, for example using telematics and inferential biometrics to monitor drivers’ attention, wakefulness or the quality of their driving. Beyond road vehicles, AI systems are also being trialled to support safer assisted docking in ports, although fully unmanned ocean-going ships are not anticipated to be in commercial use until the 2030s.
-
Navigation - modern mapping tools have commoditised route optimisation, travel time prediction and access to real-time traffic information, making them readily accessible to both businesses and consumers for planning journeys. Many such products offer API access, allowing businesses to build their own apps on top of powerful existing map tools. Routing tools are also prevalent in shipping, where small adjustments can result in significant fuel efficiencies. Machine vision can also be used to classify geospatial data, for example, automatically identifying different types of land use or property, improving map data.
-
Mobility as a Service (MaaS) - data-driven technologies have enabled a growing market of MaaS providers, in particular on-demand transport and delivery services. Integrated MaaS, whereby different transport systems are linked up to enable journey and delivery planning over the system as a whole, has the potential to reduce carbon emissions by reducing reliance on private cars, as well as improve user experience. The advent of better drones and ground-based robotic delivery systems (which depend on similar technologies to larger automated vehicles) may further automate ‘last-mile delivery’, although such approaches are still in early trial stages.
-
Supporting technologies - AI-assisted industrial design is improving vehicle and civil engineering, for example in reducing vehicle air resistance or designing more energy efficient engines.
Spotlight: Port automation
Hamburg Port Authority’s smartPORT solution aggregates data about all participants in the port in a single data system, creating efficiencies in delivery journeys. The port is also developing mobile GPS sensors to transmit data back to the central system on crane locations, locations of incident response vehicles, fleet management, temperature, wind speed and direction, air pollution and the flow of the Elbe.
The Port of Rotterdam claims to have two of the world’s most automated container terminals. Ships are loaded and unloaded by automatic cranes which lift containers and load them onto fully automated vehicles. Other applications include the Boxinsider app to provide shippers and forwarding companies with real-time status information on containers they import, and predicts vessel arrival times in the port to reduce congestion and enable more efficient use of terminals.
The Port of Tyne launched its Maritime 2050 Innovation Hub in July 2019, which forms part of the UK Government’s Maritime 2050 Strategy. The Hub hosts innovation sprints and workshops to develop operational efficiency through technology and automation, improve supply chain integration and hinterland connectivity, and to work towards full decarbonisation. Examples of the developing technologies that Port of Tyne are testing include an AI application to predict how weather patterns could impact quayside operations, and predictive maintenance technology with smart sensors located on key equipment parts.
3. Opportunities
-
The capacity for data-driven technologies to reduce the environmental impact of transport and logistics activity was seen by our panel as one of the highest benefits and hardest to achieve opportunities, particularly within the three-year time horizon our research examines. Our panel noted that the uptake required to achieve these benefits would be significant. For example, many of the environmental benefits of AVs may only be felt once they reach a sufficient proportion of road traffic and may require substantial development of supporting infrastructure beforehand. Transport management systems and vehicle fleets tend to have large sums of capital invested in them already, meaning integration of new technologies tends to be slow and incremental.
-
Accounting for the environmental benefits of improved mobility technologies must consider rebound effects, whereby improvements such as lower journey times and increased convenience lead to greater demand and use over time, potentially cancelling out lower environmental impacts. This means that behaviour change is likely to have an important role in securing the environmental benefits of new technologies, for example, decisions around where people choose to live, whether business is conducted remotely or face-to-face, and what modes of transport people choose to use, or how efficiently goods are stored and transported. AI and data can play an important role in understanding behaviour (e.g. via monitoring demand and usage at the system and vehicle levels) but our panel indicated that these may need to be combined with other incentives or interventions to drive change.
Spotlight: Environmental opportunities
Discounting the considerable reduction in direct emissions that a shift to electric vehicles will cause, technologies integrated into autonomous vehicles are expected to provide additional mechanisms for energy efficiency, from fleet management to better routing and braking, that in aggregate may reduce congestion.
Similar technologies in other transport contexts such as shipping are able to improve fuel efficiency and reduce emissions, and predictive maintenance can help preserve the longevity of carbon-intensive infrastructure investments like road networks, reducing their lifetime carbon footprint. Perhaps more significantly in the near term, traffic flow management systems can reduce journey times and consequent emissions. These technologies can also have similarly beneficial effects across other measures of environmental impact, such as air quality and noise pollution.
- Certain technologies in this sector are expected to carry significant economic benefits with their growth and development. The Connected Places Catapult’s Market Forecast suggests that the UK market for road vehicles with CAV technology will be worth £41.7bn by 2035, capturing 6.4% of the £650bn global market, creating 49,000 UK jobs in manufacturing & assembly (replacing equivalent jobs in non-CAV manufacturing), 23,400 UK jobs in CAV technology production, and 14,600 indirect jobs in the supply chain.
Spotlight: Last-mile delivery
A report released by Connected Places Catapult in January 2021 explores how the use of new technologies in the transport and logistics sectors could help the UK deliver on ‘net zero’ by 2050 pledge, with a focus on the potential impact of changes in heavy freight decarbonisation, drones and electric aircraft, and last-mile home delivery services. Among many non-data-driven technologies (e.g. new vehicle energy sources), it suggests that drones could fundamentally alter certain spaces with use cases across media, parcel delivery, search and rescue, agriculture, wildlife protection, environmental monitoring, infrastructure maintenance, and tree planting. Less optimistically, the report points to the potential impact increasing demand for home delivery has had, further accelerated by the pandemic. It argues that it is hard to measure the total carbon emissions from e-commerce but notes last-minute delivery and packaging are generally considered to be two key sources of carbon emissions.
- The difficulty of leveraging system-level technologies may vary by the process they seek to improve. For example, integrating system-level technologies into existing infrastructure such as traffic flow management systems was generally seen as easier to achieve than informing transport system planning (e.g. via predicting future mobility needs or predictive maintenance of infrastructure). This may reflect differences in the time and investment necessary to achieve planning benefits, the time periods over which predictive technologies remain accurate, and the different intended outputs of management and planning respectively. For example, it may be easier to integrate data-driven technology into systems where the input and outputs are both digital and there is a rapid feedback loop (e.g. detecting congestion through traffic cameras and using the data to automate traffic light signalling) as opposed to feeding data-driven insights into human decision-making systems such as long term infrastructure planning decisions. However, system-level applications such as traffic management were generally seen as being of very high benefit where they are able to be implemented in the near-term, as they are able to contribute environmental benefits before longer-term net zero infrastructure changes (such as a shift to electric vehicles) come online.
Spotlight: Reducing road congestion
The Chinese city of Xi’an is trialling intelligent traffic management services, using a network of surveillance cameras to monitor of traffic violations, provide a command and dispatching platform for police using aggregated camera data, predictively identify potential traffic events before they happen using large volumes of road condition and camera data, and dynamically alter the timing of signal lights to optimise the flow of vehicles.
- The use of AI-enabled robotics in both vehicular and logistics contexts to improve safety was also seen as a high benefit but hard to achieve opportunity, primarily reflecting the technical challenges that developing truly autonomous road vehicles present, and in making robotics systems sufficiently resistant to cyberattack. Our panel was more optimistic about the capacity for robotics to improve safety in more controlled environments in the near term, for example in dock or warehouse environments, particularly where they can automate dangerous tasks that would otherwise expose people to hazards or be prone to human error.
Opportunities quadrant
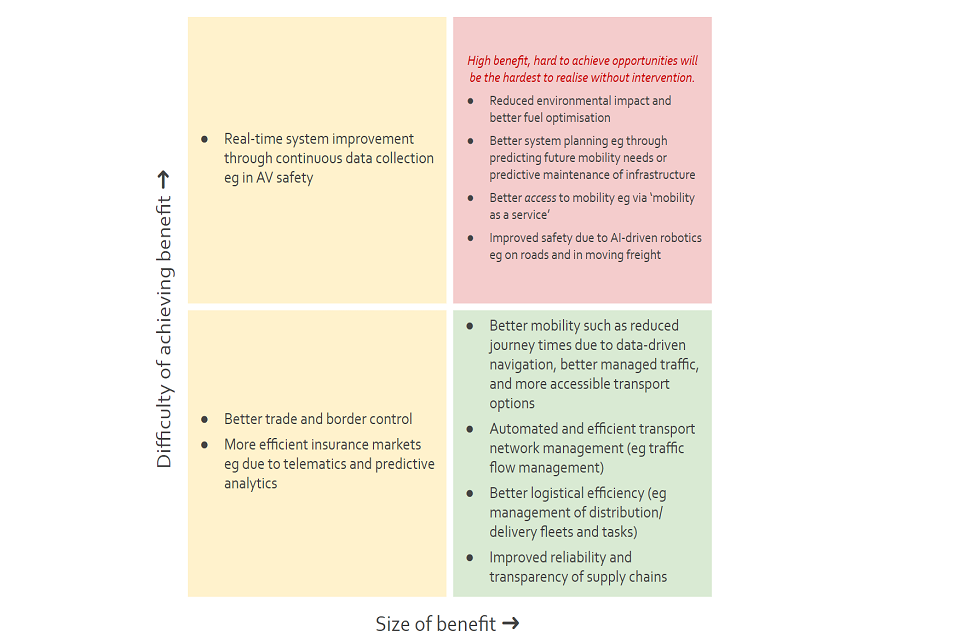
This quadrant is based on a panel survey rating the major opportunities in this sector over the next three years. This diagram is not exhaustive and reflects a review of existing policy literature, workshop discussion, further socialising and additional research and analysis. See our methodology for further detail.
Spotlight: Digital Twins
Japanese firms Toshiba and Mitsui are supporting Greater Anglia to optimise its rolling stock and timetabling through the use of digital twins - creating digital models of real-world systems to help rapidly iterate how different approaches will work in practice. The approach has already successfully improved punctuality of train services in Japan. Greater Anglia ran a trial on the route from Cambridge to London Liverpool Street and are now rolling out the solution across the network.
4. Risks
-
Safety risks were understandably among the highest-impact risks identified by our panel, with contributing factors such as low explainability, and appropriate integration of human decision-making into automated systems also ranking highly. Generally, our panel were more concerned about the use of robotics by autonomous road vehicles than in more controlled or predictable environments such as rail or ports, and noted the difficulty in safely achieving higher levels of road vehicle automation, particularly in hybrid contexts (ie around human drivers). The increasing integration of different digital systems can give rise to emergent interdependencies and risks, and our panel noted the particular impact that problematic performance of data-driven technology in this sector might have on public trust in AI technologies generally, due to the emblematic nature of use cases such as autonomous vehicles. This could be exacerbated by real or perceived bias in safety outcomes (for example, with autonomous vehicles being less capable of detecting some groups, such as wheelchair users).
-
With a few notable exceptions, data-driven systems in this sector tend not to involve the processing of large volumes of personal data, so privacy risks manifest differently. Geolocation data is typically one of the most identifiable forms of data and can reveal sensitive information about an individual’s lifestyle, although presently there are few contexts in which this data is collected by transport systems and then used in ways that may affect an individual’s privacy (e.g. for online profiling/targeting). However, our panel expressed concern about the large volumes of data that autonomous vehicles and traffic management systems need to collect in order to operate effectively, and how these give rise to a range of ethical questions around retention and access to such data for other purposes, citing international examples where such systems are also being used for surveillance purposes (see spotlight on reducing road congestion, above).
-
Our panel also identified a number of market risks, most notably the likelihood of data monopolies, where a single actor within a system holds an unparalleled market advantage due to the data they hold and are able to systematically collect. This was most notable with respect to autonomous vehicles due to the large volumes of real-world training data needed to develop them, where some market leaders already hold considerable advantages in environmental and vehicle performance data collection. In other contexts such as rail, panellists highlighted that contractual arrangements have sometimes resulted in public bodies missing out on the benefits that analysis of their datasets has provided contractors (e.g. around optimising scheduling).
-
In the longer term, our panel indicated that increased automation may give rise to additional public trust issues around job displacement, and also around cultural relationships between certain forms of transport such as cars and individual autonomy. For example, tension may arise between system efficiency (e.g. autonomous car ‘fleeting’) and an individual’s choice around their journey, as illustrated by some public reaction to an EU MCAP proposal on automated control of vehicle speed on new vehicles.
Risks quadrant
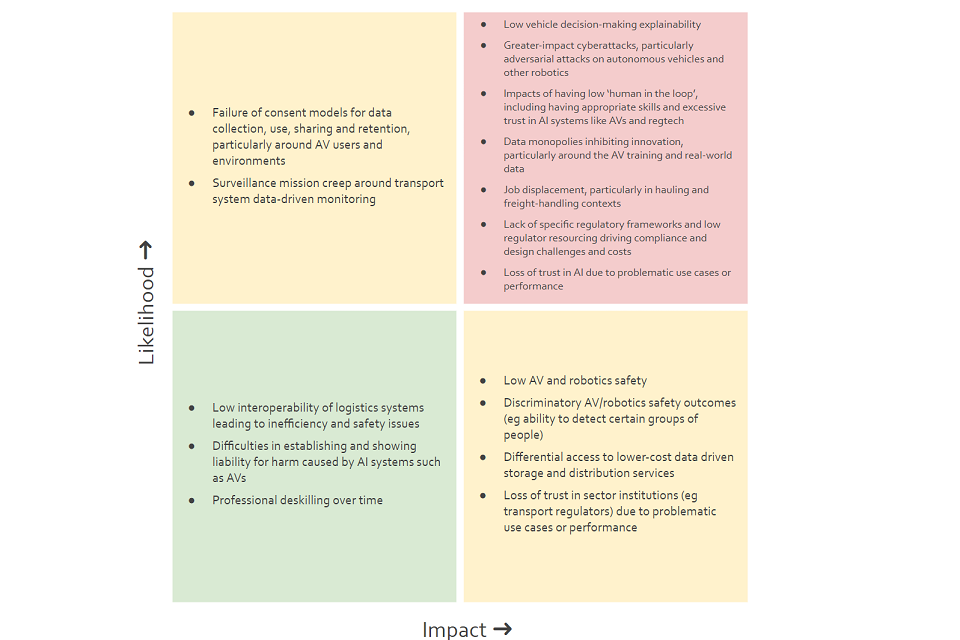
This quadrant is based on a panel survey rating the major risks in this sector over the next three years. This diagram is not exhaustive and reflects a review of existing policy literature, workshop discussion, further socialising and additional research and analysis. See our methodology for further detail.
Major risk theme - increased cybersecurity risks
-
The increased use of data-driven technology in any context presents novel cybersecurity risks beyond the mere digitisation of individual systems. The data collected by such systems represents an attractive target for thieves, and the use of machine learning introduces new ‘attack surfaces’ by virtue of their vulnerability to ‘adversarial attacks’ that take advantage of flaws in a system’s training, and the low explainability of many such systems making disguising such attacks easier.
-
Transport and logistics systems involve the individual or system level control (or signalling) for physical infrastructure, such as vehicles, traffic management systems, or cranes that can cause direct physical harms if mishandled.Their increasing centrality in critical national infrastructure makes them a bigger target for actors seeking to cause economic disruption; for example, ports tend to represent natural bottlenecks in supply chains, which can have considerable knock on effects if disrupted, or enable criminal activity such as smuggling. Autonomous vehicles and road monitoring infrastructure also collect large volumes of data about their environments, which may be of interest to some malicious actors.
-
System developers increasingly consider cybersecurity issues from the outset (e.g. autonomous vehicles being subject to cybersecurity checks before being permitted on the roads).However, there is still much the transport sector can learn from the practices employed in other sectors to understand risks. For example, in aviation, crash data is shared among manufacturers to improve safety for the industry as a whole, a practice not currently reflected in the autonomous vehicle sector.
-
Automation in transport and logistics is one of the most visible across sectors, with a large number of robotics-based applications; this may mean that the perceived safety and security of data-driven technology in this sector will have significant effects on public perception of AI generally.
Spotlight: NotPetya’s impact on international shipping
The NotPetya cyberattack occurred in June 2017 shortly after the WannaCry ransomware attack in May of the same year, encrypting data across a huge range of networks around the world, causing billions of dollars of damage. The shipping company Maersk was one of the worst affected organisations, with almost 50,000 infected endpoints, leaving only one undamaged copy of Maersk’s Active Directory that contained data on its entire distribution network and the contents of all containers. Maersk were able to rebuild their IT infrastructure over ten days thanks to the directory copy that had survived due to a power outage in Lagos that had taken its host server offline while the virus was spreading. While not the result of exposure due to the use of AI, the NotPetya attacks demonstrate the scale of impact that cyberattacks can have on critical logistics infrastructure.
Major risk theme - humans in the loop
-
While robotics have been commonplace in manufacturing contexts for many years, automation in safety-critical systems such as roads, rail, and loading environments are still in relative infancy. While greater safety is one of the most enticing promises of data-driven technologies, ensuring the right level of human control when systems malfunction or don’t understand how to behave holds a particular set of challenges, which are exacerbated in ‘hybrid’ systems where both human and machine decision-making takes place, such as CAVs on public roads. These technologies are often in place because they permit faster operation (e.g. trains packed more closely on a line), but this then creates challenges in switching decision-making over to slower human processes, in some instances making it impractical for a human operator to meaningfully intervene in time.
-
In general, tasks that are highly standardised, occur in controlled environments, and have a low risk of going wrong can be automated relatively easily and safely. While some logistics contexts like warehouses meet most of these criteria, others such as points of transfer (i.e. where loading/unloading takes place) and road environments are less predictable, meaning AI systems and human oversight must be much more resilient.
-
This challenge becomes harder the greater the degree of automation, and is reflected in the current state of automated vehicle development; ‘level 3’ automated cars that still require a human to be alert and ready to take control if needed are now commercially available in some regions, but by contrast, ‘level 4’ vehicles that only require human intervention in extreme circumstances are largely still in testing, and are presently planned for use in more restricted ‘geofenced’ contexts.
-
One way the ‘handover’ challenge can be addressed is through regular training. In some contexts, professionals periodically ensure they refresh their skills in managing technology manually - for example, on the normally-automated London Underground Victoria Line, train drivers will revert to manual control on Sundays, lowering the capacity of the line, but allowing them to maintain their skills. Consultation with staff on how to implement handover processes can also improve the resilience of such processes. However, achieving this outside of work contexts (e.g. with individual road users) is more challenging.
-
The collection of real-world data on how people are able to react in oversight roles or during handover events (e.g. in CAVs on public roads) will be crucial for designing more resilient systems that are able to call on human intervention appropriately and meaningfully.
5. Barriers
Barriers to responsible innovation are the issues that prevent us from maximising the benefits of data-driven technologies, and which prevent harms from being appropriately mitigated.
Safety-critical contexts
-
Many transport and logistic contexts such as road transport and rail system management are safety-critical, and also involve critical infrastructure that must be resilient to disruption. This has implications for the standards technologies must meet for implementation (e.g. around explainability) or whether they can be used at all - for example, it is currently not possible to use AI in safety critical applications in rail, as safety requirements demand deterministic systems.
-
The prevalence of ‘hybrid’ environments (such as roads with both human and AI-assisted drivers) and cyberattack vulnerabilities in many machine vision implementations further exacerbate this challenge.
-
Panellists observed that data monopolies and protection of intellectual property may inhibit the improvement of safety, particularly with respect to AVs, and pointed to alternative contexts such as aviation where governance strongly encourages data sharing for this purpose.
Complex governance challenges
-
Data-driven technologies present novel problems for regulators, such as the lack of clear liability and accountability frameworks for decisions made by autonomous agents or the ethics of ‘fleeting’ AVs.
-
Limited international regulatory development means there are not clear best practices to follow, although panellists noted that transport modes are regulated in very different ways and lessons could be learnt across transport contexts (e.g. the UK has had autonomous trains since 1966).
-
Many regulators in this sector have typically worked on a model of regulating products as a ‘one off’, in contrast to the real-time and ongoing nature of data-driven product development, with our panel reporting some having relatively low capacity to adapt. Panellists also noted that the lack of standardisation around data may lead to interoperability, data sharing and cybersecurity issues.
Data skills shortages
-
Our panel reported well-documented data and AI skill shortages across the transport and logistics sector, with some subsectors such as freight being particularly badly affected. While there are some skills programmes attempting to address this, competition for workers with expertise in both data and sectoral skillsets such as hardware production and manufacturing is high.
-
These shortages are also apparent in the regulatory space, where the lack of staff with technical expertise may be a further obstacle to developing effective governance.
-
Panellists cited specific procurement challenges for local authorities in making the business case for investment in data-driven technologies (e.g. to support environmental or traffic management outcomes) without technical expertise.
Market and investment incentives
-
Many of the major benefits offered by technology in this sector are spread diffusely across many others (e.g. improving air quality), meaning incentives may not be aligned between developers and users of technology, and bodies responsible for regulatory or environmental outcomes.
-
This can also mean sharing of learning does not take place - for example, data-driven business logistics approaches that can reduce the amount of travel required are very promising for environmental outcomes, but will typically not be shared by organisations for competitive advantage.
-
Panellists noted that collaboration between different public and private actors was necessary for achieving many of the highest-benefit opportunities, which will rely on high levels of interoperability and standardisation of data between systems, and observed that while some projects like the UKRI Trustworthy Autonomous Systems Programme are seeking to address this need for cross-sectoral multidisciplinary approaches, mechanisms for such coordination were generally lacking.
Sensitivity to organisational and public trust
-
The adoption of data-driven technologies can be highly influenced by organisational and public trust. Use cases in this sector are particularly sensitive to concerns around safety, fairness and privacy, and the emblematic nature of autonomous vehicles for AI can magnify these effects.
-
Beyond general safety concerns described above, skewed datasets used to train autonomous vehicle AI models may mean that this technology performs unevenly for different sections of the population (e.g. people using mobility equipment) - with a consequent additional impact on public trust.
-
The need for many use cases in this sector to collect large amounts of data on their environment (e.g. smart city infrastructure, AVs recording in public places or sharing data with each other) also raises privacy questions around data retention, access, and consent for collection - that again may impact public trust if not managed by governance or using tools like privacy enhancing technologies.
-
For example, the London Underground Victoria Line, which opened in 1968 with an Automatic Train Operation system in operation ↩
-
For example, Maersk have trialled an AI solution to optimise container stuffing (taking into account origin, destination, or cargo-specific regulations, client determined rule restrictions, optimum patterns for loading and unloading) ↩
-
For example, the ScanReach wireless IoT platform for complex and confined steel environments ↩
-
For example, Undagrid’s GSEtrack system that tracks baggage carts and other equipment through a ‘mesh network’ of mounted sensors, providing data on: location, movement, maintenance, battery and temperature ↩
-
The role and ethics of blockchain technologies in supply chain management has been extensively explored in Digital Catapult’s KnowRisk Report, 2021 ↩
-
For example, GrowthPlanner tool, based on Transport for London’s Public Transport Access Level ↩
-
MuCCA (Multi-Car Collision Avoidance): ~3 year, £4.5m collaboration between CCAV, Innovate UK & 5 automotive industry partners. ↩