Review into bias in algorithmic decision-making
Published 27 November 2020
Preface
Fairness is a highly prized human value. Societies in which individuals can flourish need to be held together by practices and institutions that are regarded as fair. What it means to be fair has been much debated throughout history, rarely more so than in recent months. Issues such as the global Black Lives Matter movement, the “levelling up” of regional inequalities within the UK, and the many complex questions of fairness raised by the COVID-19 pandemic have kept fairness and equality at the centre of public debate.
Inequality and unfairness have complex causes, but bias in the decisions that organisations make about individuals is often a key aspect. The impact of efforts to address unfair bias in decision-making have often either gone unmeasured or have been painfully slow to take effect. However, decision-making is currently going through a period of change. Use of data and automation has existed in some sectors for many years, but it is currently expanding rapidly due to an explosion in the volumes of available data, and the increasing sophistication and accessibility of machine learning algorithms. Data gives us a powerful weapon to see where bias is occurring and measure whether our efforts to combat it are effective; if an organisation has hard data about differences in how it treats people, it can build insight into what is driving those differences, and seek to address them.
However, data can also make things worse. New forms of decision-making have surfaced numerous examples where algorithms have entrenched or amplified historic biases; or even created new forms of bias or unfairness. Active steps to anticipate risks and measure outcomes are required to avoid this.
Concern about algorithmic bias was the starting point for this policy review. When we began the work this was an issue of concern to a growing, but relatively small, number of people. As we publish this report, the issue has exploded into mainstream attention in the context of exam results, with a strong narrative that algorithms are inherently problematic. This highlights the urgent need for the world to do better in using algorithms in the right way: to promote fairness, not undermine it. Algorithms, like all technology, should work for people, and not against them.
This is true in all sectors, but especially key in the public sector. When the state is making life-affecting decisions about individuals, that individual often can’t go elsewhere. Society may reasonably conclude that justice requires decision-making processes to be designed so that human judgement can intervene where needed to achieve fair and reasonable outcomes for each person, informed by individual evidence.
As our work has progressed it has become clear that we cannot separate the question of algorithmic bias from the question of biased decision-making more broadly. The approach we take to tackling biased algorithms in recruitment, for example, must form part of, and be consistent with, the way we understand and tackle discrimination in recruitment more generally.
A core theme of this report is that we now have the opportunity to adopt a more rigorous and proactive approach to identifying and mitigating bias in key areas of life, such as policing, social services, finance and recruitment. Good use of data can enable organisations to shine a light on existing practices and identify what is driving bias. There is an ethical obligation to act wherever there is a risk that bias is causing harm and instead make fairer, better choices.
The risk is growing as algorithms, and the datasets that feed them, become increasingly complex. Organisations often find it challenging to build the skills and capacity to understand bias, or to determine the most appropriate means of addressing it in a data-driven world. A cohort of people is needed with the skills to navigate between the analytical techniques that expose bias and the ethical and legal considerations that inform best responses. Some organisations may be able to create this internally, others will want to be able to call on external experts to advise them. Senior decision-makers in organisations need to engage with understanding the trade-offs inherent in introducing an algorithm. They should expect and demand sufficient explainability of how an algorithm works so that they can make informed decisions on how to balance risks and opportunities as they deploy it into a decision-making process.
Regulators and industry bodies need to work together with wider society to agree best practice within their industry and establish appropriate regulatory standards. Bias and discrimination are harmful in any context. But the specific forms they take, and the precise mechanisms needed to root them out, vary greatly between contexts. We recommend that there should be clear standards for anticipating and monitoring bias, for auditing algorithms and for addressing problems. There are some overarching principles, but the details of these standards need to be determined within each sector and use case. We hope that CDEI can play a key role in supporting organisations, regulators and government in getting this right.
Lastly, society as a whole will need to be engaged in this process. In the world before AI there were many different concepts of fairness. Once we introduce complex algorithms to decision-making systems, that range of definitions multiplies rapidly. These definitions are often contradictory with no formula for deciding which is correct. Technical expertise is needed to navigate these choices, but the fundamental decisions about what is fair cannot be left to data scientists alone. They are decisions that can only be truly legitimate if society agrees and accepts them. Our report sets out how organisations might tackle this challenge.
Transparency is key to helping organisations build and maintain public trust. There is a clear, and understandable, nervousness about the use and consequences of algorithms, exacerbated by the events of this summer. Being open about how and why algorithms are being used, and the checks and balances in place, is the best way to deal with this. Organisational leaders need to be clear that they retain accountability for decisions made by their organisations, regardless of whether an algorithm or a team of humans is making those decisions on a day-to-day basis.
In this report we set out some key next steps for the government and regulators to support organisations to get their use of algorithms right, whilst ensuring that the UK ecosystem is set up to support good ethical innovation. Our recommendations are designed to produce a step change in the behaviour of all organisations making life changing decisions on the basis of data, however limited, and regardless of whether they used complex algorithms or more traditional methods.
Enabling data to be used to drive better, fairer, more trusted decision-making is a challenge that countries face around the world. By taking a lead in this area, the UK, with its strong legal traditions and its centres of expertise in AI, can help to address bias and inequalities not only within our own borders but also across the globe.
The Board of the Centre for Data Ethics and Innovation
Executive summary
Unfair biases, whether conscious or unconscious, can be a problem in many decision-making processes. This review considers the impact that an increasing use of algorithmic tools is having on bias in decision-making, the steps that are required to manage risks, and the opportunities that better use of data offers to enhance fairness. We have focused on the use of algorithms in significant decisions about individuals, looking across four sectors (recruitment, financial services, policing and local government), and making cross-cutting recommendations that aim to help build the right systems so that algorithms improve, rather than worsen, decision-making.
It is well established that there is a risk that algorithmic systems can lead to biased decisions, with perhaps the largest underlying cause being the encoding of existing human biases into algorithmic systems. But the evidence is far less clear on whether algorithmic decision-making tools carry more or less risk of bias than previous human decision-making processes. Indeed, there are reasons to think that better use of data can have a role in making decisions fairer, if done with appropriate care.
When changing processes that make life-affecting decisions about individuals we should always proceed with caution. It is important to recognise that algorithms cannot do everything. There are some aspects of decision-making where human judgement, including the ability to be sensitive and flexible to the unique circumstances of an individual, will remain crucial.
Using data and algorithms in innovative ways can enable organisations to understand inequalities and to reduce bias in some aspects of decision-making. But there are also circumstances where using algorithms to make life-affecting decisions can be seen as unfair by failing to consider an individual’s circumstances, or depriving them of personal agency. We do not directly focus on this kind of unfairness in this report, but note that this argument can also apply to human decision-making, if the individual who is subject to the decision does not have a role in contributing to the decision.
History to date in the design and deployment of algorithmic tools has not been good enough. There are numerous examples worldwide of the introduction of algorithms persisting or amplifying historical biases, or introducing new ones. We must and can do better. Making fair and unbiased decisions is not only good for the individuals involved, but it is good for business and society. Successful and sustainable innovation is dependent on building and maintaining public trust. Polling undertaken for this review suggested that, prior to August’s controversy over exam results, 57% of people were aware of algorithmic systems being used to support decisions about them, with only 19% of those disagreeing in principle with the suggestion of a “fair and accurate” algorithm helping to make decisions about them. By October, we found that awareness had risen slightly (to 62%), as had disagreement in principle (to 23%). This doesn’t suggest a step change in public attitudes, but there is clearly still a long way to go to build trust in algorithmic systems. The obvious starting point for this is to ensure that algorithms are trustworthy.
The use of algorithms in decision-making is a complex area, with widely varying approaches and levels of maturity across different organisations and sectors. Ultimately, many of the steps needed to challenge bias will be context specific. But from our work, we have identified a number of concrete steps for industry, regulators and government to take that can support ethical innovation across a wide range of use cases. This report is not a guidance manual, but considers what guidance, support, regulation and incentives are needed to create the right conditions for fair innovation to flourish.
It is crucial to take a broad view of the whole decision-making process when considering the different ways bias can enter a system and how this might impact on fairness. The issue is not simply whether an algorithm is biased, but whether the overall decision-making processes are biased. Looking at algorithms in isolation cannot fully address this.
It is important to consider bias in algorithmic decision-making in the context of all decision-making systems. Even in human decision-making, there are differing views about what is and isn’t fair. But society has developed a range of standards and common practices for how to manage these issues, and legal frameworks to support this. Organisations have a level of understanding on what constitutes an appropriate level of due care for fairness. The challenge is to make sure that we can translate this understanding across to the algorithmic world, and apply a consistent bar of fairness whether decisions are made by humans, algorithms or a combination of the two. We must ensure decisions can be scrutinised, explained and challenged so that our current laws and frameworks do not lose effectiveness, and indeed can be made more effective over time.
Significant growth is happening both in data availability and use of algorithmic decision-making across many sectors; we have a window of opportunity to get this right and ensure that these changes serve to promote equality, not to entrench existing biases.
Sector reviews
The four sectors studied in Part II of this report are at different maturity levels in their use of algorithmic decision-making. Some of the issues they face are sector-specific, but we found common challenges that span these sectors and beyond.
In recruitment we saw a sector that is experiencing rapid growth in the use of algorithmic tools at all stages of the recruitment process, but also one that is relatively mature in collecting data to monitor outcomes. Human bias in traditional recruitment is well evidenced and therefore there is potential for data-driven tools to improve matters by standardising processes and using data to inform areas of discretion where human biases can creep in.
However, we also found that a clear and consistent understanding of how to do this well is lacking, leading to a risk that algorithmic technologies will entrench inequalities. More guidance is needed on how to ensure that these tools do not unintentionally discriminate against groups of people, particularly when trained on historic or current employment data. Organisations must be particularly mindful to ensure they are meeting the appropriate legislative responsibilities around automated decision-making and reasonable adjustments for candidates with disabilities.
The innovation in this space has real potential for making recruitment fairer. However, given the potential risks, further scrutiny of how these tools work, how they are used and the impact they have on different groups, is required, along with higher and clearer standards of good governance to ensure that ethical and legal risks are anticipated and managed.
In financial services, we saw a much more mature sector that has long used data to support decision-making. Finance relies on making accurate predictions about peoples’ behaviours, for example how likely they are to repay debts. However, specific groups are historically underrepresented in the financial system, and there is a risk that these historic biases could be entrenched further through algorithmic systems. We found financial service organisations ranged from being highly innovative to more risk averse in their use of new algorithmic approaches. They are keen to test their systems for bias, but there are mixed views and approaches regarding how this should be done. This was particularly evident around the collection and use of protected characteristic data, and therefore organisations’ ability to monitor outcomes.
Our main focus within financial services was on credit scoring decisions made about individuals by traditional banks. Our work found the key obstacles to further innovation in the sector included data availability, quality and how to source data ethically, available techniques with sufficient explainability, risk averse culture, in some parts, given the impacts of the financial crisis and difficulty in gauging consumer and wider public acceptance. The regulatory picture is clearer in financial services than in the other sectors we have looked at. The Financial Conduct Authority (FCA) is the main regulator and is showing leadership in prioritising work to understand the impact and opportunities of innovative uses of data and AI in the sector.
The use of data from non-traditional sources could enable population groups who have historically found it difficult to access credit, due to lower availability of data about them from traditional sources, to gain better access in future. At the same time, more data and more complex algorithms could increase the potential for the introduction of indirect bias via proxy as well as the ability to detect and mitigate it.
Adoption of algorithmic decision-making in the public sector is generally at an early stage. In policing, we found very few tools currently in operation in the UK, with a varied picture across different police forces, both on usage and approaches to managing ethical risks.
There have been notable government reviews into the issue of bias in policing, which is important context when considering the risks and opportunities around the use of technology in this sector. Again, we found potential for algorithms to support decision-making, but this introduces new issues around the balance between security, privacy and fairness, and there is a clear requirement for strong democratic oversight.
Police forces have access to more digital material than ever before, and are expected to use this data to identify connections and manage future risks. The £63.7 million funding for police technology programmes announced in January 2020 demonstrates the government’s drive for innovation. But clearer national leadership is needed. Though there is strong momentum in data ethics in policing at a national level, the picture is fragmented with multiple governance and regulatory actors, and no single body fully empowered or resourced to take ownership. The use of data analytics tools in policing carries significant risk. Without sufficient care, processes can lead to outcomes that are biased against particular groups, or systematically unfair. In many scenarios where these tools are helpful, there is still an important balance to be struck between automated decision-making and the application of professional judgement and discretion. Given the sensitivities in this area it is not sufficient for care to be taken internally to consider these issues; it is also critical that police forces are transparent in how such tools are being used to maintain public trust.
In local government, we found an increased use of data to inform decision-making across a wide range of services. Whilst most tools are still in the early phase of deployment, there is an increasing demand for sophisticated predictive technologies to support more efficient and targeted services.
By bringing together multiple data sources, or representing existing data in new forms, data-driven technologies can guide decision-makers by providing a more contextualised picture of an individual’s needs. Beyond decisions about individuals, these tools can help predict and map future service demands to ensure there is sufficient and sustainable resourcing for delivering important services.
However, these technologies also come with significant risks. Evidence has shown that certain people are more likely to be overrepresented in data held by local authorities and this can then lead to biases in predictions and interventions. A related problem occurs when the number of people within a subgroup is small. Data used to make generalisations can result in disproportionately high error rates amongst minority groups.
Data-driven tools present genuine opportunities for local government. However, tools should not be considered a silver bullet for funding challenges and in some cases additional investment will be required to realise their potential. Moreover, we found that data infrastructure and data quality were significant barriers to developing and deploying data-driven tools effectively and responsibly. Investment in this area is needed before developing more advanced systems.
Sector-specific recommendations to regulators and government
Most of the recommendations in this report are cross-cutting, but we identified the following recommendations specific to individual sectors. More details are given in sector chapters below.
Recruitment
Recommendation 1: The Equality and Human Rights Commission should update its guidance on the application of the Equality Act 2010 to recruitment, to reflect issues associated with the use of algorithms, in collaboration with consumer and industry bodies.
Recommendation 2: The Information Commissioner’s Office should work with industry to understand why current guidance is not being consistently applied, and consider updates to guidance (e.g. in the Employment Practices Code), greater promotion of existing guidance, or other action as appropriate.
Policing
Recommendation 3: The Home Office should define clear roles and responsibilities for national policing bodies with regards to data analytics and ensure they have access to appropriate expertise and are empowered to set guidance and standards. As a first step, the Home Office should ensure that work underway by the National Police Chiefs’ Council and other policing stakeholders to develop guidance and ensure ethical oversight of data analytics tools is appropriately supported.
Local government
Recommendation 4: Government should develop national guidance to support local authorities to legally and ethically procure or develop algorithmic decision-making tools in areas where significant decisions are made about individuals, and consider how compliance with this guidance should be monitored.
Addressing the challenges
We found underlying challenges across the four sectors, and indeed other sectors where algorithmic decision-making is happening. In Part III of this report, we focus on understanding these challenges, where the ecosystem has got to on addressing them, and the key next steps for organisations, regulators and government. The main areas considered are:
The enablers needed by organisations building and deploying algorithmic decision-making tools to help them do this in a fair way, see Chapter 7.
The regulatory levers, both formal and informal, needed to incentivise organisations to do this, and create a level playing field for ethical innovation see Chapter 8.
How the public sector, as a major developer and user of data-driven technology, can show leadership in this area through transparency see Chapter 9.
There are inherent links between these areas. Creating the right incentives can only succeed if the right enablers are in place to help organisations act fairly, but conversely, there is little incentive for organisations to invest in tools and approaches for fair decision-making if there is insufficient clarity on expected norms. We want a system that is fair and accountable; one that preserves, protects or improves fairness in decisions being made with the use of algorithms. We want to address the obstacles that organisations may face to innovate ethically, to ensure the same or increased levels of accountability for these decisions and how society can identify and respond to bias in algorithmic decision-making processes. We have considered the existing landscape of standards and laws in this area, and whether they are sufficient for our increasingly data-driven society.
To realise this vision we need clear mechanisms for safe access to data to test for bias; organisations that are able to make judgements based on data about bias; a skilled industry of third parties who can provide support and assurance, and regulators equipped to oversee and support their sectors and remits through this change.
Enabling fair innovation
We found that many organisations are aware of the risks of algorithmic bias, but are unsure how to address bias in practice.
There is no universal formulation or rule that can tell you an algorithm is fair. Organisations need to identify what fairness objectives they want to achieve and how they plan to do this. Sector bodies, regulators, standards bodies and the government have a key role in setting out clear guidelines on what is appropriate in different contexts; getting this right is essential not only for avoiding bad practice, but for giving the clarity that enables good innovation. However, all organisations need to be clear about their own accountability for getting it right. Whether an algorithm or a structured human process is being used to make a decision doesn’t change an organisation’s accountability.
Improving diversity across a range of roles involved in the development and deployment of algorithmic decision-making tools is an important part of protecting against bias. Government and industry efforts to improve this must continue, and need to show results.
Data is needed to monitor outcomes and identify bias, but data on protected characteristics is not available often enough. One reason for this is an incorrect belief that data protection law prevents collection or usage of this data. Indeed, there are a number of lawful bases in data protection legislation for using protected or special characteristic data when monitoring or addressing discrimination. But there are some other genuine challenges in collecting this data, and more innovative thinking is needed in this area; for example around the potential for trusted third party intermediaries.
The machine learning community has developed multiple techniques to measure and mitigate algorithmic bias. Organisations should be encouraged to deploy methods that address bias and discrimination. However, there is little guidance on how to choose the right methods, or how to embed them into development and operational processes. Bias mitigation cannot be treated as a purely technical issue; it requires careful consideration of the wider policy, operational and legal contexts. There is insufficient legal clarity concerning novel techniques in this area. Many can be used legitimately, but care is needed to ensure that the application of some techniques does not cross into unlawful positive discrimination.
Recommendations to government
Recommendation 5: Government should continue to support and invest in programmes that facilitate greater diversity within the technology sector, building on its current programmes and developing new initiatives where there are gaps.
Recommendation 6: Government should work with relevant regulators to provide clear guidance on the collection and use of protected characteristic data in outcome monitoring and decision-making processes. They should then encourage the use of that guidance and data to address current and historic bias in key sectors.
Recommendation 7: Government and the Office for National Statistics (ONS) should open the Secure Research Service more broadly, to a wider variety of organisations, for use in evaluation of bias and inequality across a greater range of activities.
Recommendation 8: Government should support the creation and development of data-focused public and private partnerships, especially those focused on the identification and reduction of biases and issues specific to under-represented groups. The Office for National Statistics (ONS) and Government Statistical Service should work with these partnerships and regulators to promote harmonised principles of data collection and use into the private sector, via shared data and standards development.
Recommendations to regulators
Recommendation 9: Sector regulators and industry bodies should help create oversight and technical guidance for responsible bias detection and mitigation in their individual secin individual sectors, adding context-specific detail to the existing cross-cutting guidance on data protection, and any new cross-cutting guidance on the Equality Act.
Good, anticipatory governance is crucial here. Many of the high profile cases of algorithmic bias could have been anticipated with careful evaluation and mitigation of the potential risks. Organisations need to make sure that the right capabilities and structures are in place to ensure that this happens both before algorithms are introduced into decision-making processes, and through their life. Doing this well requires understanding of, and empathy for, the expectations of those who are affected by decisions, which can often only be achieved through the right engagement with groups. Given the complexity of this area, we expect to see a growing role for expert professional services supporting organisations. Although the ecosystem needs to develop further, there is already plenty that organisations can and should be doing to get this right. Data Protection Impact Assessments and Equality Impact Assessments can help with structuring thinking and documenting the steps taken.
Guidance to organisation leaders and boards
Those responsible for governance of organisations deploying or using algorithmic decision-making tools to support significant decisions about individuals should ensure that leaders are in place with accountability for:
- Understanding the capabilities and limits of those tools
- Considering carefully whether individuals will be fairly treated by the decision-making process that the tool forms part of
- Making a conscious decision on appropriate levels of human involvement in the decision-making process
- Putting structures in place to gather data and monitor outcomes for fairness
- Understanding their legal obligations and having carried out appropriate impact assessments
This especially applies in the public sector when citizens often do not have a choice about whether to use a service, and decisions made about individuals can often be life-affecting.
The regulatory environment
Clear industry norms, and good, proportionate regulation, are key both for addressing risks of algorithmic bias, and for promoting a level playing field for ethical innovation to thrive.
The increased use of algorithmic decision-making presents genuinely new challenges for regulation, and brings into question whether existing legislation and regulatory approaches can address these challenges sufficiently well. There is currently limited case law or statutory guidance directly addressing discrimination in algorithmic decision-making, and the ecosystems of guidance and support are at different maturity levels in different sectors.
Though there is only a limited amount of case law, the recent judgement of the Court of Appeal in relation to the usage of live facial recognition technology by South Wales Police seems likely to be significant. One of the grounds for successful appeal was that South Wales Police failed to adequately consider whether their trial could have a discriminatory impact, and specifically that they did not take reasonable steps to establish whether their facial recognition software contained biases related to race or sex. In doing so, the court found that they did not meet their obligations under the Public Sector Equality Duty, even though there was no evidence that this specific algorithm was biased. This suggests a general duty for public sector organisations to take reasonable steps to consider any potential impact on equality upfront and to detect algorithmic bias on an ongoing basis. The current regulatory landscape for algorithmic decision-making consists of the Equality and Human Rights Commission (EHRC), the Information Commissioner’s Office (ICO) and sector regulators. At this stage, we do not believe that there is a need for a new specialised regulator or primary legislation to address algorithmic bias.
However, algorithmic bias means the overlap between discrimination law, data protection law and sector regulations is becoming increasingly important. We see this overlap playing out in a number of contexts, including discussions around the use of protected characteristics data to measure and mitigate algorithmic bias, the lawful use of bias mitigation techniques, identifying new forms of bias beyond existing protected characteristics. The first step in resolving these challenges should be to clarify the interpretation of the law as it stands, particularly the Equality Act 2010, both to give certainty to organisations deploying algorithms and to ensure that existing individual rights are not eroded, and wider equality duties are met. However, as use of algorithmic decision-making grows further, we do foresee a future need to look again at the legislation itself, which should be kept under consideration as guidance is developed and case law evolves.
Existing regulators need to adapt their enforcement to algorithmic decision-making, and provide guidance on how regulated bodies can maintain and demonstrate compliance in an algorithmic age. Some regulators require new capabilities to enable them to respond effectively to the challenges of algorithmic decision-making. While larger regulators with a greater digital remit may be able to grow these capabilities in-house, others will need external support. Many regulators are working hard to do this, and the ICO has shown leadership in this area both by starting to build a skills base to address these new challenges, and in convening other regulators to consider issues arising from AI. Deeper collaboration across the regulatory ecosystem is likely to be needed in future.
Outside of the formal regulatory environment, there is increasing awareness within the private sector of the demand for a broader ecosystem of industry standards and professional services to help organisations address algorithmic bias. There are a number of reasons for this: it is a highly specialised skill that not all organisations will be able to support, it will be important to have consistency in how the problem is addressed, and because regulatory standards in some sectors may require independent audit of systems. Elements of such an ecosystem might be licenced auditors or qualification standards for individuals with the necessary skills. Audit of bias is likely to form part of a broader approach to audit that might also cover issues such as robustness and explainability. Government, regulators, industry bodies and private industry will all play important roles in growing this ecosystem so that organisations are better equipped to make fair decisions.
Recommendations to government
Recommendation 10: Government should issue guidance that clarifies the Equality Act responsibilities of organisations using algorithmic decision-making. This should include guidance on the collection of protected characteristics data to measure bias and the lawfulness of bias mitigation techniques.
Recommendation 11: Though the development of this guidance and its implementation, government should assess whether it provides both sufficient clarity for organisations on meeting their obligations, and leaves sufficient scope for organisations to take actions to mitigate algorithmic bias. If not, government should consider new regulations or amendments to the Equality Act to address this.
Recommendations to regulators
Recommendation 12: The EHRC should ensure that it has the capacity and capability to investigate algorithmic discrimination. This may include EHRC reprioritising resources to this area, EHRC supporting other regulators to address algorithmic discrimination in their sector, and additional technical support to the EHRC.
Recommendation 13: Regulators should consider algorithmic discrimination in their supervision and enforcement activities, as part of their responsibilities under the Public Sector Equality Duty.
Recommendation 14: Regulators should develop compliance and enforcement tools to address algorithmic bias, such as impact assessments, audit standards, certification and/or regulatory sandboxes.
Recommendation 15: Regulators should coordinate their compliance and enforcement efforts to address algorithmic bias, aligning standards and tools where possible. This could include jointly issued guidance, collaboration in regulatory sandboxes, and joint investigations.
Public sector transparency
Making decisions about individuals is a core responsibility of many parts of the public sector, and there is increasing recognition of the opportunities offered through the use of data and algorithms in decision-making. The use of technology should never reduce real or perceived accountability of public institutions to citizens. In fact, it offers opportunities to improve accountability and transparency, especially where algorithms have significant effects on significant decisions about individuals.
A range of transparency measures already exist around current public sector decision-making processes; both proactive sharing of information about how decisions are made, and reactive rights for citizens to request information on how decisions were made about them. The UK government has shown leadership in setting out guidance on AI usage in the public sector, including a focus on techniques for explainability and transparency. However, more is needed to make transparency about public sector use of algorithmic decision-making the norm. There is a window of opportunity to ensure that we get this right as adoption starts to increase, but it is sometimes hard for individual government departments or other public sector organisations to be first in being transparent; a strong central drive for this is needed.
The development and delivery of an algorithmic decision-making tool will often include one or more suppliers, whether acting as technology suppliers or business process outsourcing providers. While the ultimate accountability for fair decision-making always sits with the public body, there is limited maturity or consistency in contractual mechanisms to place responsibilities in the right place in the supply chain. Procurement processes should be updated in line with wider transparency commitments to ensure standards are not lost along the supply chain.
Recommendations to government
Recommendation 16: Government should place a mandatory transparency obligation on all public sector organisations using algorithms that have a significant influence on significant decisions affecting individuals. Government should conduct a project to scope this obligation more precisely, and to pilot an approach to implement it, but it should require the proactive publication of information on how the decision to use an algorithm was made, the type of algorithm, how it is used in the overall decision-making process, and steps taken to ensure fair treatment of individuals.
Recommendation 17: Cabinet Office and the Crown Commercial Service should update model contracts and framework agreements for public sector procurement to incorporate a set of minimum standards around ethical use of AI, with particular focus on expected levels of transparency and explainability, and ongoing testing for fairness.
Next steps and future challenges
This review has considered a complex and rapidly evolving field. There is plenty to do across industry, regulators and government to manage the risks and maximise the benefits of algorithmic decision-making. Some of the next steps fall within CDEI’s remit, and we are happy to support industry, regulators and government in taking forward the practical delivery work to address the issues we have identified and future challenges which may arise. Outside of specific activities, and noting the complexity and range of the work needed across multiple sectors, we see a key need for national leadership and coordination to ensure continued focus and pace in addressing these challenges across sectors. This is a rapidly moving area. A level of coordination and monitoring will be needed to assess how organisations building and using algorithmic decision-making tools are responding to the challenges highlighted in this report, and to the proposed new guidance from regulators and government. Government should be clear on where it wants this coordination to sit; for example in central government directly, in a specific regulator or in CDEI.
In this review we have concluded that there is significant scope to address the risks posed by bias in algorithmic decision-making within the law as it stands, but if this does not succeed then there is a clear possibility that future legislation may be required. We encourage organisations to respond to this challenge; to innovate responsibly and think through the implications for individuals and society at large as they do so.
Part I: Introduction
1. Background and scope
1.1 About CDEI
The adoption of data-driven technology affects every aspect of our society and its use is creating opportunities as well as new ethical challenges.
The Centre for Data Ethics and Innovation (CDEI) is an independent expert committee, led by a board of specialists, set up and tasked by the UK government to investigate and advise on how we maximise the benefits of these technologies.
Our goal is to create the conditions in which ethical innovation can thrive: an environment in which the public are confident their values are reflected in the way data-driven technology is developed and deployed; where we can trust that decisions informed by algorithms are fair; and where risks posed by innovation are identified and addressed.
More information about CDEI can be found at www.gov.uk/cdei.
1.2 About this review
In the October 2018 Budget, the Chancellor announced that we would investigate the potential bias in decisions made by algorithms. This review formed a key part of our 2019/2020 work programme, though completion was delayed by the onset of COVID-19. This is the final report of CDEI’s review and includes a set of formal recommendations to the government.
Government tasked us to draw on expertise and perspectives from stakeholders across society to provide recommendations on how they should address this issue. We also provide advice for regulators and industry, aiming to support responsible innovation and help build a strong, trustworthy system of governance. The government has committed to consider and respond publicly to our recommendations.
1.3 Our focus
The use of algorithms in decision-making is increasing across multiple sectors of our society. Bias in algorithmic decision-making is a broad topic, so in this review, we have prioritised the types of decisions where potential bias seems to represent a significant and imminent ethical risk.
This has led us to focus on:
- Areas where algorithms have the potential to make or inform a decision that directly affects an individual human being (as opposed to other entities, such as companies). The significance of decisions of course varies, and we have typically focused on areas where individual decisions could have a considerable impact on a person’s life, i.e. decisions that are significant in the sense of the Data Protection Act 2018.
- The extent to which algorithmic decision-making is being used now, or is likely to be soon, in different sectors.
- Decisions made or supported by algorithms, and not wider ethical issues in the use of artificial intelligence.
- The changes in ethical risk in an algorithmic world as compared to an analogue world.
- Circumstances where decisions are biased (see Chapter 2 for a discussion of what this means), rather than other forms of unfairness such as arbitrariness or unreasonableness.
This scope is broad, but it doesn’t cover all possible areas where algorithmic bias can be an issue. For example, the CDEI Review of online targeting, published earlier this year, highlighted the risk of harm through bias in targeting within online platforms. These are decisions which are individually very small, for example on targeting an advert or recommending content to a user, but the overall impact of bias across many small decisions can still be problematic. This review did touch on these issues, but they fell outside of our core focus on significant decisions about individuals.
It is worth highlighting that the main work of this review was carried out before a number of highly relevant events in mid 2020; the COVID-19 pandemic, Black Lives Matter, the awarding of exam results without exams, and (with less widespread attention, but very specific relevance) the judgement of the Court of Appeal in Bridges v South Wales Police. We have considered links to these issues in our review, but have not been able to treat them in full depth.[footnote 1]
1.4 Our approach
Sector approach
The ethical questions in relation to bias in algorithmic decision-making vary depending on the context and sector. We chose four initial areas of focus to illustrate the range of issues. These were recruitment, financial services, policing and local government. Our rationale for choosing these sectors is set out in the introduction to Part II.
Cross-sector themes
From the work we carried out on the four sectors, as well as our engagement across government, civil society, academia and interested parties in other sectors, we were able to identify themes, issues and opportunities that went beyond the individual sectors.
We set out three key cross-cutting questions in our interim report, which we have sought to address on a cross-sector basis:
1. Data: Do organisations and regulators have access to the data they require to adequately identify and mitigate bias?
2. Tools and techniques: What statistical and technical solutions are available now or will be required in future to identify and mitigate bias and which represent best practice?
3. Governance: Who should be responsible for governing, auditing and assuring these algorithmic decision-making systems?
These questions have guided the review. While we have made sector-specific recommendations where appropriate, our recommendations focus more heavily on opportunities to address these questions (and others) across multiple sectors.
Evidence
Our evidence base for this final report is informed by a variety of work including:
-
A landscape summary led by Professor Michael Rovatsos of the University of Edinburgh, which assessed the current academic and policy literature.
-
An open call for evidence which received responses from a wide cross section of academic institutions and individuals, civil society, industry and the public sector.
-
A series of semi-structured interviews with companies in the financial services and recruitment sectors developing and using algorithmic tools.
-
Work with the Behavioural Insights Team on attitudes to the use of algorithms in personal banking.[footnote 2]
- Commissioned research from the Royal United Services Institute (RUSI) on data analytics in policing in England and Wales.[footnote 3]
- Contracted work by Faculty on technical bias mitigation techniques.[footnote 4]
-
Representative polling on public attitudes to a number of the issues raised in this report, conducted by Deltapoll as part of CDEI’s ongoing public engagement work.
-
Meetings with a variety of stakeholders including regulators, industry groups, civil society organisations, academics and government departments, as well as desk-based research to understand the existing technical and policy landscape.
2. The issue
Summary
-
Algorithms are structured processes, which have long been used to aid human decision-making. Recent developments in machine learning techniques and exponential growth in data has allowed for more sophisticated and complex algorithmic decisions, and there has been corresponding growth in usage of algorithm supported decision-making across many areas of society.
-
This growth has been accompanied by significant concerns about bias; that the use of algorithms can cause a systematic skew in decision-making that results in unfair outcomes. There is clear evidence that algorithmic bias can occur, whether through entrenching previous human biases or introducing new ones.
-
Some forms of bias constitute discrimination under the Equality Act 2010, namely when bias leads to unfair treatment based on certain protected characteristics. There are also other kinds of algorithmic bias that are non-discriminatory, but still lead to unfair outcomes.
-
There are multiple concepts of fairness, some of which are incompatible and many of which are ambiguous. In human decisions we can often accept this ambiguity and allow for human judgement to consider complex reasons for a decision. In contrast, algorithms are unambiguous.
-
Fairness is about much more than the absence of bias: fair decisions need to also be non-arbitrary, reasonable, consider equality implications and respect the circumstances and personal agency of the individuals concerned.
-
Despite concerns about ‘black box’ algorithms, in some ways algorithms can be more transparent than human decisions; unlike a human it is possible to reliably test how an algorithm responds to changes in parts of the input. There are opportunities to deploy algorithmic decision-making transparently, and enable the identification and mitigation of systematic bias in ways that are challenging with humans. Human developers and users of algorithms must decide the concepts of fairness that apply to their context, and ensure that algorithms deliver fair outcomes.
-
Fairness through unawareness is often not enough to prevent bias: ignoring protected characteristics is insufficient to prevent algorithmic bias and it can prevent organisations from identifying and addressing bias.
-
The need to address algorithmic bias goes beyond regulatory requirements under equality and data protection law. It is also critical for innovation that algorithms are used in a way that is both fair, and seen by the public to be fair.
2.1 Introduction
Human decision-making has always been flawed, shaped by individual or societal biases that are often unconscious. Over the years, society has identified ways of improving it, often by building processes and structures that encourage us to make decisions in a fairer and more objective way, from agreed social norms to equality legislation. However, new technology is introducing new complexities. The growing use of algorithms in decision-making has raised concerns around bias and fairness.
Even in this data-driven context, the challenges are not new. In 1988, the UK Commission for Racial Equality found a British medical school guilty of algorithmic discrimination when inviting applicants to interview.[footnote 5] The computer program they had used was determined to be biased against both women and applicants with non-European names.
The growth in this area has been driven by the availability and volume of (often personal) data that can be used to train machine learning models, or as inputs into decisions, as well as cheaper and easier availability of computing power, and innovations in tools and techniques. As usage of algorithmic tools grows, so does their complexity. Understanding the risks is therefore crucial to ensure that these tools have a positive impact and improve decision-making.
Algorithms have different but related vulnerabilities to human decision-making processes. They can be more able to explain themselves statistically, but less able to explain themselves in human terms. They are more consistent than humans but are less able to take nuanced contextual factors into account. They can be highly scalable and efficient, but consequently capable of consistently applying errors to very large populations. They can also act to obscure the accountabilities and liabilities that individual people or organisations have for making fair decisions.
2.2 The use of algorithms in decision-making
In simple terms, an algorithm is a structured process. Using structured processes to aid human decision-making is much older than computation. Over time, the tools and approaches available to deploy such decision-making have become more sophisticated. Many organisations responsible for making large numbers of structured decisions (for example, whether an individual qualifies for a welfare benefits payment, or whether a bank should offer a customer a loan), make these processes scalable and consistent by giving their staff well-structured processes and rules to follow. Initial computerisation of such decisions took a similar path, with humans designing structured processes (or algorithms) to be followed by a computer handling an application.
However, technology has reached a point where the specifics of those decision-making processes are not always explicitly manually designed. Machine learning tools often seek to find patterns in data without requiring the developer to specify which factors to use or how exactly to link them, before formalising relationships or extracting information that could be useful to make decisions. The results of these tools can be simple and intuitive for humans to understand and interpret, but they can also be highly complex.
Some sectors, such as credit scoring and insurance, have a long history of using statistical techniques to inform the design of automated processes based on historical data. An ecosystem has evolved that helps to manage some of the potential risks, for example credit reference agencies offer customers the ability to see their own credit history, and offer guidance on the factors that can affect credit scoring. In these cases, there are a range of UK regulations that govern the factors that can and cannot be used.
We are now seeing the application of data-driven decision-making in a much wider range of scenarios. There are a number of drivers for this increase, including:
- The exponential growth in the amount of data held by organisations, which makes more decision-making processes amenable to data-driven approaches.
- Improvements in the availability and cost of computing power and skills.
- Increased focus on cost saving, driven by fiscal constraints in the public sector, and competition from disruptive new entrants in many private sector markets.
- Advances in machine learning techniques, especially deep neural networks, that have rapidly brought many problems previously inaccessible to computers into routine everyday use (e.g. image and speech recognition). In simple terms, an algorithm is a set of instructions designed to perform a specific task. In algorithmic decision-making, the word is applied in two different contexts:
- A machine learning algorithm takes data as an input to create a model. This can be a one-off process, or something that happens continually as new data is gathered.
- Algorithm can also be used to describe a structured process for making a decision, whether followed by a human or computer, and possibly incorporating a machine learning model.
The usage is usually clear from context. In this review we are focused mainly on decision-making processes involving machine learning algorithms, although some of the content is also relevant to other structured decision-making processes. Note that there is no hard definition of exactly which statistical techniques and algorithms constitute novel machine learning. We have observed that many recent developments are associated with applying existing statistical techniques more widely in new sectors, not about novel techniques.
We interpret algorithmic decision-making to include any decision-making process where an algorithm makes, or meaningfully assists, the decision. This includes what is sometimes referred to as algorithmically-assisted decision-making. In this review we are focused mainly on decisions about individual people.
Figure 1 below shows an example of how a machine learning algorithm can be used within a decision-making process, such as a bank making a decision on whether to offer a loan to an individual.
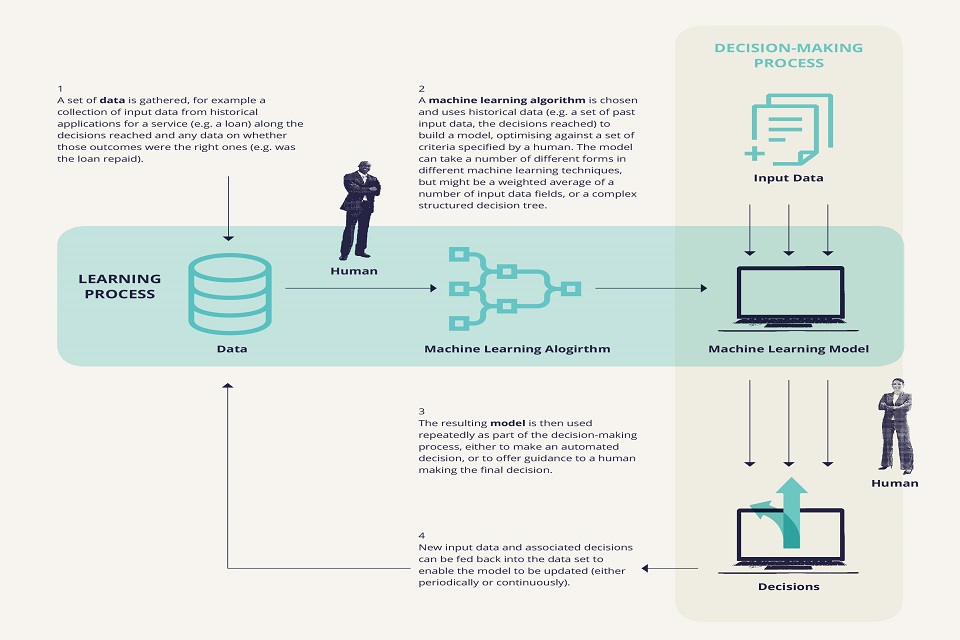
Machine-learning algorithms can be used within decision-making processes. First, a set of data is gathered, for example a collection of input data from historical applications for a service (e.g. a loan) along with the decisions reached, and any data on whether those outcomes were the right ones (e.g. was the loan repaid). A human decides what data to make available to the model. Second, A machine learning algorithm is chosen, and uses historical data (e.g. a set of past input data (e.g. a set of past input data, the decisions reached) to build a model, optimising against a set of criteria specified by a human. The model can take a number of different forms in different machine learning techniques, but might be a weighted average of a number of a number of input data fields, or a complex structured decision tree. Third, the resulting model is then used repeatedly as part of the decision-making process, either to make an automated decision, or to offer guidance to a human making the final decision. A human can be involved at this stage to vet the machine-learning model’s outputs and make judgements about how to incorporate this information into a final decision. Fourth, new input data and associated decisions can be fed back into the data set to enable the model to be updated (either periodically or continuously).
Figure 1: How data and algorithms come together to support decision-making
It is important to emphasise that algorithms often do not represent the complete decision-making process. There may be elements of human judgement, exceptions treated outside of the usual process and opportunities for appeal or reconsideration. In fact, for significant decisions, an appropriate provision for human review will usually be required to comply with data protection law. Even before an algorithm is deployed into a decision-making process, it is humans that decide on the objectives it is trying to meet, the data available to it, and how the output is used.
It is therefore critical to consider not only the algorithmic aspect, but the whole decision-making process that sits around it. Human intervention in these processes will vary, and in some cases may be absent entirely in fully automated systems. Ultimately the aim is not just to avoid bias in algorithmic aspects of a process, but that the process as a whole achieves fair decision-making.
2.3 Bias
As algorithmic decision-making grows in scale, increasing concerns are being raised around the risks of bias. Bias has a precise meaning in statistics, referring to a systematic skew in results, that is an output that is not correct on average with respect to the overall population being sampled.
However in general usage, and in this review, bias is used to refer to an output that is not only skewed, but skewed in a way that is unfair (see below for a discussion on what unfair might mean in this context).
Bias can enter algorithmic decision-making systems in a number of ways, including:
-
Historical bias: The data that the model is built, tested and operated on could introduce bias. This may be because of previously biased human decision-making or due to societal or historical inequalities. For example, if a company’s current workforce is predominantly male then the algorithm may reinforce this, whether the imbalance was originally caused by biased recruitment processes or other historical factors. If your criminal record is in part a result of how likely you are to be arrested (as compared to someone else with the same history of behaviour, but not arrests), an algorithm constructed to assess risk of reoffending is at risk of not reflecting the true likelihood of reoffending, but instead reflects the more biased likelihood of being caught reoffending.
-
Data selection bias: How the data is collected and selected could mean it is not representative. For example, over or under recording of particular groups could mean the algorithm was less accurate for some people, or gave a skewed picture of particular groups. This has been the main cause of some of the widely reported problems with accuracy of some facial recognition algorithms across different ethnic groups, with attempts to address this focusing on ensuring a better balance in training data.[footnote 6]
-
Algorithmic design bias: It may also be that the design of the algorithm leads to introduction of bias. For example, CDEI’s Review of online targeting noted examples of algorithms placing job advertisements online designed to optimise for engagement at a given cost, leading to such adverts being more frequently targeted at men because women are more costly to advertise to.
-
Human oversight is widely considered to be a good thing when algorithms are making decisions, and mitigates the risk that purely algorithmic processes cannot apply human judgement to deal with unfamiliar situations. However, depending on how humans interpret or use the outputs of an algorithm, there is also a risk that bias re-enters the process as the human applies their own conscious or unconscious biases to the final decision.
There is also risk that bias can be amplified over time by feedback loops, as models are incrementally re-trained on new data generated, either fully or partly, via use of earlier versions of the model in decision-making. For example, if a model predicting crime rates based on historical arrest data is used to prioritise police resources, then arrests in high risk areas could increase further, reinforcing the imbalance. CDEI’s Landscape summary discusses this issue in more detail.
2.4 Discrimination and equality
In this report we use the word discrimination in the sense defined in the Equality Act 2010, meaning unfavourable treatment on the basis of a protected characteristic.[footnote 7]
The Equality Act 2010[footnote 8] makes it unlawful to discriminate against someone on the basis of certain protected characteristics (for example age, race, sex, disability) in public functions, employment and the provision of goods and services.
The choice of these characteristics is a recognition that they have been used to treat people unfairly in the past and that, as a society, we have deemed this unfairness unacceptable. Many, albeit not all, of the concerns about algorithmic bias relate to situations where that bias may lead to discrimination in the sense set out in the Equality Act 2010.
The Equality Act 2010[footnote 9] defines two main categories of discrimination:[footnote 10]
-
Direct Discrimination: When a person is treated less favourably than another because of a protected characteristic.
-
Indirect Discrimination: When a wider policy or practice, even if it applies to everyone, disadvantages a group of people who share a protected characteristic (and there is not a legitimate reason for doing so).
Where this discrimination is direct, the interpretation of the law in an algorithmic decision-making process seems relatively clear. If an algorithmic model explicitly leads to someone being treated less favourably on the basis of a protected characteristic that would be unlawful. There are some very specific exceptions to this in the case of direct discrimination on the basis of age (where such discrimination could be lawful if a proportionate means to a proportionate aim, e.g. services targeted at a particular age range) or limited positive actions in favour of those with disabilities.
However, the increased use of data-driven technology has created new possibilities for indirect discrimination. For example, a model might consider an individual’s postcode. This is not a protected characteristic, but there is some correlation between postcode and race. Such a model, used in a decision-making process (perhaps in financial services or policing) could in principle cause indirect racial discrimination. Whether that is the case or not depends on a judgement about the extent to which such selection methods are a proportionate means of achieving a legitimate aim.[footnote 11] For example, an insurer might be able to provide good reasons why postcode is a relevant risk factor in a type of insurance. The level of clarity about what is and is not acceptable practice varies by sector, reflecting in part the maturity in using data in complex ways. As algorithmic decision-making spreads into more use cases and sectors, clear context-specific norms will need to be established. Indeed as the ability of algorithms to deduce protected characteristics with certainty from proxies continues to improve, it could even be argued that some examples could potentially cross into direct discrimination.
Unfair bias beyond discrimination
Discrimination is a narrower concept than bias. Protected characteristics have been included in law due to historical evidence of systematic unfair treatment, but individuals can also experience unfair treatment on the basis of other characteristics that are not protected.
There will always be grey areas where individuals experience systematic and unfair bias on the basis of characteristics that are not protected, for example accent, hairstyle, education or socio-economic status.[footnote 12] In some cases, these may be considered as indirect discrimination if they are connected with protected characteristics, but in other cases they may reflect unfair biases that are not protected by discrimination law.
However the increased use of algorithms may exacerbate this difficulty. The introduction of algorithms can encode existing biases into algorithms, if they are trained from existing decisions. This can reinforce and amplify existing unfair bias, whether on the basis of protected characteristics or not.
Algorithmic decision-making can also go beyond amplifying existing biases, to creating new biases that may be unfair, though difficult to address through discrimination law. This is because machine learning algorithms find new statistical relationships, without necessarily considering whether the basis for those relationships is fair, and then apply this systematically in large numbers of individual decisions.
2.5 Fairness
Overview
We defined bias as including an element of unfairness. This highlights challenges in defining what we mean by fairness, which is a complex and long debated topic. Notions of fairness are neither universal nor unambiguous, and they are often inconsistent with one another.
In human decision-making systems, it is possible to leave a degree of ambiguity about how fairness is defined. Humans may make decisions for complex reasons, and are not always able to articulate their full reasoning for making a decision, even to themselves. There are pros and cons to this. It allows for good fair-minded decision-makers to consider the specific individual circumstances, and human understanding of the reasons for why these circumstances might not conform to typical patterns. This is especially important in some of the most critical life-affecting decisions, such as those in policing or social services, where decisions often need to be made on the basis of limited or uncertain information; or where wider circumstances, beyond the scope of the specific decision, need to be taken into account. It is hard to imagine that automated decisions could ever fully replace human judgement in such cases. But human decisions are also open to the conscious or unconscious biases of the decision-makers, as well as variations in their competence, concentration levels or mood when specific decisions are made.
Algorithms, by contrast, are unambiguous. If we want a model to comply with a definition of fairness, we must tell it explicitly what that definition is. How significant a challenge that is depends on context. Sometimes the meaning of fairness is very clearly defined; to take an extreme example, a chess playing AI achieves fairness by following the rules of the game. Often though, existing rules or processes require a human decision-maker to exercise discretion or judgement, or to account for data that is difficult to include in a model (e.g. context around the decision that cannot be readily quantified). Existing decision-making processes must be fully understood in context in order to decide whether algorithmic decision-making is likely to be appropriate. For example, police officers are charged with enforcing the criminal law, but it is often necessary for officers to apply discretion on whether a breach of the letter of the law warrants action. This is broadly a good thing, but such discretion also allows an individual’s personal biases, whether conscious or unconscious, to affect decisions.
Even in cases where fairness can be more precisely defined, it can still be challenging to capture all relevant aspects of fairness in a mathematical definition. In fact, the trade-offs between mathematical definitions demonstrate that a model cannot conform to all possible fairness definitions at the same time. Humans must choose which notions of fairness are appropriate for a particular algorithm, and they need to be willing to do so upfront when a model is built and a process is designed.
The General Data Protection Regulation (GDPR) and Data Protection Act 2018 contain a requirement that organisations should use personal data in a way that is fair. The legislation does not elaborate further on the meaning of fairness, but the ICO guides organisations that “In general, fairness means that you should only handle personal data in ways that people would reasonably expect and not use it in ways that have unjustified adverse effects on them.”[footnote 13] Note that the discussion in this section is wider than the notion in GDPR, and does not attempt to define how the word fair should be interpreted in that context.
Notions of fairness
Notions of fair decision-making (whether human or algorithmic) are typically gathered into two broad categories:
-
procedural fairness is concerned with ‘fair treatment’ of people, i.e. equal treatment within the process of how a decision is made. It might include, for example, defining an objective set of criteria for decisions, and enabling individuals to understand and challenge decisions about them.
-
outcome fairness is concerned with what decisions are made i.e. measuring average outcomes of a decision-making process and assessing how they compare to an expected baseline. The concept of what a fair outcome means is of course highly subjective; there are multiple different definitions of outcome fairness.
Some of these definitions are complementary to each other, and none alone can capture all notions of fairness. A ‘fair’ process may still produce ‘unfair’ results, and vice versa, depending on your perspective. Even within outcome fairness there are many mutually incompatible definitions for a fair outcome. Consider for example a bank making a decision on whether an applicant should be eligible for a given loan, and the role of an applicant’s sex in this decision. Two possible definitions of outcome fairness in this example are:
A. The probability of getting a loan should be the same for men and women.
B. The probability of getting a loan should be the same for men and women who earn the same income. Taken individually, either of these might seem like an acceptable definition of fair. But they are incompatible. In the real world sex and income are not independent of each other; the UK has a gender pay gap meaning that, on average, men earn more than women.[footnote 14] Given that gap, it is mathematically impossible to achieve both A and B simultaneously.
This example is by no means exhaustive in highlighting the possible conflicting definitions that can be made, with a large collection of possible definitions identified in the machine learning literature.[footnote 15]
In human decision-making we can often accept ambiguity around this type of issue, but when determining if an algorithmic decision-making process is fair, we have to be able to explicitly determine what notion of fairness we are trying to optimise for. It is a human judgement call whether the variable (in this case salary) acting as a proxy for a protected characteristic (in this case sex) is seen as reasonable and proportionate in the context. We investigated public reactions to a similar example to this in work with the Behavioural Insights Team (see further detail in Chapter 4.
Addressing fairness
Even when we can agree what constitutes fairness, it is not always clear how to respond. Conflicting views about the value of fairness definitions arise when the application of a process intended to be fair produces outcomes regarded as unfair. This can be explained in several ways, for example:
- Differences in outcomes are evidence that the process is not fair. If in principle, there is no good reason why there should be differences on average in the ability of men and women to do a particular job, differences in the outcomes between male and female applicants may be evidence that a process is biased and failing to accurately identify those most able. By correcting this, the process is both fairer and more efficient.
- Differences in outcomes are the consequence of past injustices. For example, a particular set of previous experience might be regarded as a necessary requirement for a role, but might be more common among certain socio-economic backgrounds due to past differences in access to employment and educational opportunities. Sometimes it might be appropriate for an employer to be more flexible on requirements to enable them to get the benefits of a more diverse workforce (perhaps bearing a cost of additional training); but sometimes this may not be possible for an individual employer to resolve in their recruitment, especially for highly specialist roles.
The first argument implies greater outcome fairness is consistent with more accurate and fair decision-making. The second argues that different groups ought to be treated differently to correct for historical wrongs and is the argument associated with quota regimes. It is not possible to reach a general opinion on which argument is correct, this is highly dependent on the context (and there are also other possible explanations).
In decision-making processes based on human judgement it is rarely possible to fully separate the causes of differences in outcomes. Human recruiters may believe they are accurately assessing capabilities, but if the outcomes seem skewed it is not always possible to determine the extent to which this in fact reflects bias in methods of assessing capabilities.
How do we handle this in the human world? There are a variety of techniques, for example steps to ensure fairness in an interview-based recruitment process might include:
- Training interviewers to recognise and challenge their own individual unconscious biases.
- Policies on the composition of interview panels.
- Designing assessment processes that score candidates against objective criteria.
- Applying formal or informal quotas (though a quota based on protected characteristics would usually be unlawful in the UK).
Why algorithms are different
The increased use of more complex algorithmic approaches in decision-making introduces a number of new challenges and opportunities.
The need for conscious decisions about fairness: In data-driven systems, organisations need to address more of these issues at the point a model is built, rather than relying on human decision-makers to interpret guidance appropriately (an algorithm can’t apply “common sense” on a case-by-case basis). Humans are able to balance things implicitly, machines will optimise without any balance if asked to do so.
Explainability: Data-driven systems allow for a degree of explainability about the factors causing variation in the outcomes of decision-making systems between different groups and to assess whether or not this is regarded as fair. For example, it is possible to examine more directly the degree to which relevant characteristics are acting as a proxy for other characteristics, and causing differences in outcomes between different groups. If a recruitment process included requirements for length of service and qualification, it would be possible to see whether, for example, length of service was generally lower for women due to career breaks and that this was causing an imbalance.
The extent to which this is possible depends on the complexity of the algorithm used. Dynamic algorithms drawing on large datasets may not allow for a precise attribution of the extent to which the outcome of the process for an individual woman was attributable to a particular characteristic and its association with gender. However, it is possible to assess the degree to which over a time period, different characteristics are influencing recruitment decisions and how they correlate with characteristics during that time.
The term ‘black box’ is often used to describe situations where, for a variety of different reasons, an explanation for a decision is unobtainable. This can include commercial issues (e.g. the decision-making organisation does not understand the details of the algorithm which their supplier considers their own intellectual property) or technical reasons (e.g. machine learning techniques that are less accessible for easy human explanation of individual decisions). The Information Commissioner’s Office and the Alan Turing Institute have recently published detailed joint advice on how organisations can overcome some of these challenges and provide a level of explanation of decisions.[footnote 16]
Scale of impact: The potential breadth of impact of an algorithm links to the market dynamics. Many algorithmic software tools are developed as platforms and sold across many companies. It is therefore possible, for example, that individuals applying to multiple jobs could be rejected at sift by the same algorithm (perhaps sold to a large number of companies recruiting for the same skill sets in the same industry). If the algorithm does this for reasons irrelevant to their actual performance, but on the basis of a set of characteristics that are not protected, then this feels very much like systematic discrimination against a group of individuals, but the Equality Act provides no obvious protection against this.
Algorithmic decision-making will inevitably increase over time; the aim should be to ensure that this happens in a way that acts to challenge bias, increase fairness and promote equality, rather than entrenching existing problems. The recommendations of this review are targeted at making this happen.
Case study: Exam results in August 2020
Due to COVID-19, governments across the UK decided to cancel school examinations in summer 2020, and find an alternative approach to awarding grades. All four nations of the UK attempted to implement similar processes to deliver this; combining teacher assessments with a statistical moderation process that attempted to achieve a similar distribution of grades to previous years. The approaches were changed in response to public concerns, and significant criticism about both individual fairness and concerns that grades were biased.
How should fairness have been interpreted in this case? There were a number of different notions of fairness to consider, including:
-
Fairness between year groups: Achieve a similar distribution of grades to previous and future year groups.
-
Group fairness between different schools: Attempt to standardise teacher assessed grades, given the different levels of strictness/optimism in grading between different schools to be fair to individual students from different schools.
-
Group fairness and discrimination: Avoid exacerbating differences in outcomes correlated with protected characteristics; particularly sex and race. This did not include addressing any systematic bias in results based on inequality of opportunity; this was seen as outside the mandate of an exam body.
-
Avoid any bias based on socio-economic status.
-
A fair process for allocating grades to individual students, i.e. allocating them a grade that was seen to be a fair representation of their own individual capabilities and efforts.
The main work of this review was complete prior to the release of summer 2020 exam results, but there are some clear links between the issues raised and the contents of this review, including issues of public trust, transparency and governance.
2.6 Applying ethical principles
The way decisions are made, the potential biases which they are subject to, and the impact these decisions have on individuals, are highly context dependent. It is unlikely that all forms of bias can be entirely eliminated. This is also true in human decision-making; it is important to understand the status quo prior to the introduction of data-driven technology in any given scenario. Decisions may need to be made about what kinds and degrees of bias are tolerable in certain contexts and the ethical questions will vary depending on the sector.
We want to help create the conditions where ethical innovation using data-driven technology can thrive. It is therefore essential to ensure our approach is grounded in robust ethical principles.
The UK government, along with 41 other countries, has signed up to the OECD Principles on Artificial Intelligence[footnote 18]. They provide a good starting point for considering our approach to dealing with bias, as follows:[footnote 19]
1: AI should benefit people and the planet by driving inclusive growth, sustainable development and well-being.
There are many potential advantages of algorithmic decision-making tools when used appropriately, such as the potential efficiency and accuracy of predictions. There is also the opportunity for these tools to support good decision-making by reducing human error and combating existing bias. When designed correctly, they can offer a more objective alternative (or supplement) to human subjective interpretation. It is core to this review, and the wider purpose of CDEI, to identify how we can collectively ensure that these opportunities outweigh the risks.
2: AI systems should be designed in a way that respects the rule of law, human rights, democratic values and diversity, and they should include appropriate safeguards – for example, enabling human intervention where necessary – to ensure a fair and just society.
This principle sets out some core terms for what we mean by fairness in an algorithmic decision-making process. We cover a number of aspects of it throughout the review.
Our focus in this review on significant decisions means that we have been largely considering decisions where the algorithm forms only part of an overall decision-making process, and hence there is a level of direct human oversight of individual decisions. However, consideration is always needed on whether the role of the human remains meaningful; does the human understand the algorithm (and its limitations) sufficiently well to exercise that oversight effectively? Does the organisational environment that they are working within empower them to do so? Is there a risk that human biases could be reintroduced through this oversight?
In Chapter 8 below we consider the ability of existing UK legal and regulatory structures to ensure fairness in this area, especially data protection and equality legislation, and how they will need to evolve to adapt to an algorithmic world.
3: There should be transparency and responsible disclosure around AI systems to ensure that people understand AI-based outcomes and can challenge them.
Our sector-led work has identified variable levels of transparency on the usage of algorithms. A variety of other recent reviews have called for increased levels of transparency across the public sector.
It is clear that more work is needed to achieve this level of transparency in a consistent way across the economy, and especially in the public sector where many of the highest stakes decisions are made. We discuss how this can be achieved in Chapter 9.
4: AI systems must function in a robust, secure and safe way throughout their life cycles and potential risks should be continually assessed and managed.
In Chapter 7 we identify approaches taken to mitigate the risk of bias through the development lifecycle of an algorithmic decision-making system, and suggest action that the government can take to support development teams in taking a fair approach.
5: Organisations and individuals developing, deploying or operating AI systems should be held accountable for their proper functioning in line with the above principles.
The use of algorithmic decision-making tools within decisions can have a significant impact on individuals or society, raising a requirement for clear lines of accountability in their use and impact.
When decisions are made by humans in large organisations, we don’t generally consider it possible to get it right every time. Instead, we expect organisations to have appropriate structures, policies and procedures to anticipate and address potential bias, offer redress when it occurs, and set clear governance processes and lines of accountability for decisions.
Organisations that are introducing algorithms into decisions that were previously purely made by humans should be looking to achieve at least equivalent standards of fairness, accountability and transparency, and in many cases should look to do better. Defining equivalence is not always easy of course, there may be occasions where these standards have to be achieved in a different way in an algorithmic world. We discuss this issue in more detail in Part III of the report.
For all of these issues, it is important to remember that we are not just interested in the output of an algorithm, but the overall decision-making process that sits around it. Organisations have existing accountability processes and standards, and the use of algorithms in decision-making needs to sit within existing accountability processes to ensure that they are used intentionally and effectively, and therefore that the organisation is as accountable for the outcome as they are for traditional human decision-making.
We must decide how far to mitigate bias and how we should govern our approach to doing so. These decisions require value judgements and trade-offs between competing values. Humans are often trusted to make these trade-offs without having to explicitly state how much weight they have put on different considerations. Algorithms are different. They are programmed to make trade-offs according to rules and their decisions can be interrogated and made explicit.
2.7 The opportunity
The OECD principles are clearly high level, and only take us so far when making difficult ethical balances for individual decision-making systems. The work in this review suggests that as algorithmic decision-making continues to grow in scale, we should be ambitious in aiming not only to avoid new bias, but to use this as an opportunity to address historical unfairness.
Organisations responsible for using algorithms require more specific guidance on how principles apply in their circumstances. The principles are often context specific and are discussed in more detail in the sector sections below. However, we can start to outline some rules of thumb that can guide all organisations using algorithms to support significant decision-making processes:
- History shows that most decision-making processes are biased, often unintentionally. If you want to make fairer decisions, then using data to measure this is the best approach; certainly assuming the non-existence of bias in a process is a highly unreliable approach.
- If your data shows historical patterns of bias, this does not mean that algorithms should not be considered. The bias should be addressed, and the evidence from the data should help inform that approach. Algorithms designed to mitigate bias may be part of the solution.
- If an algorithm is introduced to replace a human decision system, the bias mitigation strategy should be designed to result in fairer outcomes and a reduction in unwarranted differences between groups.
- While it is important to test the outputs of algorithms and assess their fairness, the key measure of the fairness of an algorithm is the impact it has on the whole decision process. In some cases, resolving fairness issues may only be possible outside of the actual decision-making process, e.g. by addressing wider systemic issues in society.
- Putting a ‘human in the loop’ is a way of addressing concern about the ‘unforgiving nature’ of algorithms (as they can bring perspectives or contextual information not available to the algorithm) but can also introduce human bias into the system. Humans ‘over the loop’ monitoring the fairness of the whole decision process are also needed, with responsibility for the whole process.
- Humans over the loop need to understand how the machine learning model works, and the limitations and trade-offs that it is making, to a great enough extent to make informed judgements on whether it is performing effectively and fairly.
Part II: Sector reviews
The ethical questions in relation to bias in algorithmic decision-making vary depending on the context and sector. We therefore chose four initial areas of focus to illustrate the range of issues. These were recruitment, financial services, policing and local government.
All of these sectors have the following in common:
- They involve making decisions at scale about individuals which involve significant potential impacts on those individuals’ lives.
- There is a growing interest in the use of algorithmic decision-making tools in these sectors, including those involving machine learning in particular.
- There is evidence of historic bias in decision-making within these sectors, leading to risks of this being perpetuated by the introduction of algorithms.
There are of course other sectors that we could have considered; these were chosen as a representative sample across the public and private sector, not because we have judged that the risk of bias is most acute in these specific cases.
In this part of the review, we focus on the sector-specific issues, and reach a number of recommendations specific to individual sectors. The sector studies then inform the cross-cutting findings and recommendations in Part III below.
3. Recruitment
Summary
Overview of findings:
-
The use of algorithms in recruitment has increased in recent years, in all stages of the recruitment process. Trends suggest these tools will become more widespread, meaning that clear guidance and a robust regulatory framework are essential.
-
When developed responsibly, data-driven tools have the potential to improve recruitment by standardising processes and removing discretion where human biases can creep in, however if using historical data, these human biases are highly likely to be replicated.
-
Rigorous testing of new technologies is necessary to ensure platforms do not unintentionally discriminate against groups of people, and the only way to do this is to collect demographic data on applicants and use this data to monitor how the model performs. Currently, there is little standardised guidance for how to do this testing, meaning companies are largely self-regulated.
-
Algorithmic decision-making in recruitment is currently governed primarily by the Equality Act 2010 and the Data Protection Act 2018, however we found in both cases there is confusion regarding how organisations should enact their legislative responsibilities.
Recommendations to regulators:
-
Recommendation 1: The Equality and Human Rights Commission should update its guidance on the application of the Equality Act 2010 to recruitment, to reflect issues associated with the use of algorithms, in collaboration with consumer and industry bodies.
-
Recommendation 2: The Information Commissioner’s Office should work with industry to understand why current guidance is not being consistently applied, and consider updates to guidance (e.g. in the Employment Practices Code), greater promotion of existing guidance, or other action as appropriate.
Advice to industry
- Organisations should carry out Equality Impact Assessments to understand how their models perform for candidates with different protected characteristics, including intersectional analysis for those with multiple protected characteristics.
Future CDEI work
- CDEI will consider how it can work with relevant organisations to assist with developing guidance on applying the Equality Act 2010 to algorithms in recruitment.
3.1 Background
Decisions about who to shortlist, interview and employ have significant effects on the lives of individuals and society. When certain groups are disadvantaged either directly or indirectly from the recruitment process, social inequalities are broadened and embedded.
The existence of human bias in traditional recruitment is well-evidenced.[footnote 20] A famous study found that when orchestral players were kept behind a screen for their audition, there was a significant increase in the number of women who were successful.[footnote 21] Research in the UK found that candidates with ethnic minority backgrounds have to send as many of 60% more applications than white candidates to receive a positive response.[footnote 22] Even more concerning is the fact that there has been very little historical improvement in these figures over the last few decades.[footnote 23] Recruitment is also considered a barrier to employment for people with disabilities.[footnote 24] A range of factors from affinity biases, where recruiters tend to prefer people similar to them, to informal processes that recruit candidates already known to the organisation all amplify these biases, and some people believe technology could play a role in helping to standardise processes and make them fairer.[footnote 25]
The internet has also meant that candidates are able to apply for a much larger number of jobs, thus creating a new problem for organisations needing to review hundreds, sometimes thousands, of applications. These factors have led to an increase in new data-driven tools, promising greater efficiency, standardisation and objectivity. There is a consistent upwards trend in adoption, with around 40% of HR functions in international companies now using AI.[footnote 26] It is however important to distinguish between new technologies and algorithmic decision-making. Whilst new technology is increasingly being applied across the board in recruitment, our research was focused on tools that utilise algorithmic decision-making systems, training on data to predict a candidate’s future success.
There are concerns about the potential negative impacts of algorithmic decision-making in recruitment. There are also concerns about the effectiveness of technologies to be able to predict good job performance given the relative inflexibility of systems and the challenge of conducting a thorough assessment using automated processes at scale. For the purpose of this report, our focus is on bias rather than effectiveness.
How we approached our work
Our work on recruitment as a sector began with a call for evidence and the landscape summary. This evidence gathering provided a broad overview of the challenges and opportunities presented by using algorithmic tools in hiring.
In addition to desk-based research, we conducted a series of semi-structured interviews with a broad range of software providers and recruiters. In these conversations we focused on how providers currently test and mitigate bias in their tools. We also spoke with a range of other relevant organisations and individuals including think tanks, academics, government departments, regulators and civil society groups.
3.2 Findings
Tools are being created and used for every stage of the recruitment process
There are many stages in a recruitment process and algorithms are increasingly being used throughout.[footnote 27] Starting with the sourcing of applicants via targeting online advertisements[footnote 28] through to CV screening, then interview and selection phases. Data-driven tools are sold as a more efficient, accurate and objective way of assisting with recruiting decisions.
Figure 2: Examples of algorithmic tools used through the sourcing, screening, interview and selection stages of the recruitment process
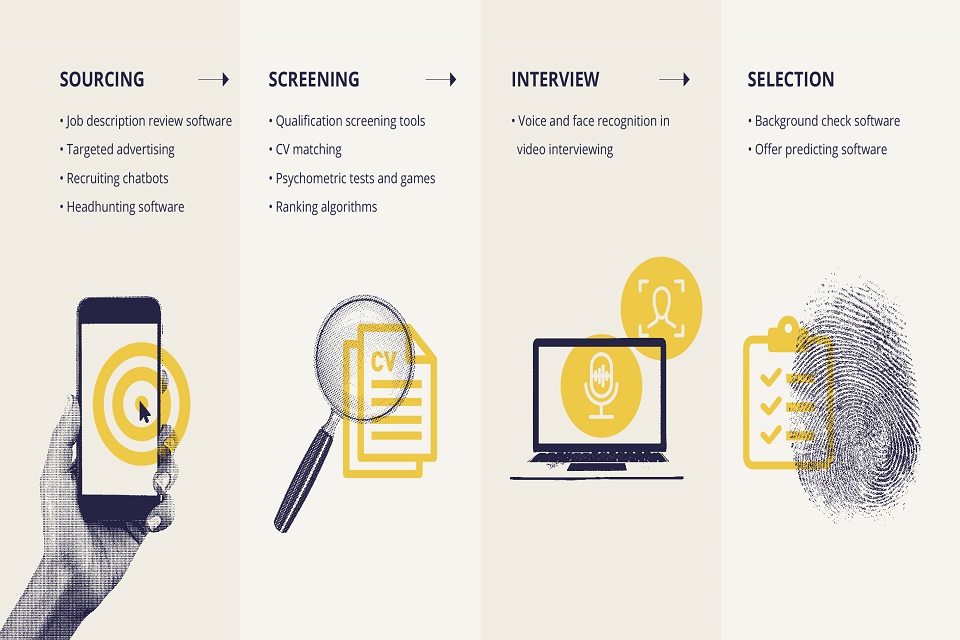
In recruitment, AI tools are available to support all stages of the hiring process: sourcing, screening, interview and selection. At the sourcing stage, tools are available to review job descriptions, target job advertising to potential candidates, power recruiting chatbots and ‘headhunt’ for high-performing candidates. At the screening stage, AI tools can be used to screen qualifications, match CVs to specific job roles, run psychometric and game-based tests to assess cognitive skills, and rank applications. At the interview stage, voice and face recognition technology can be used in video interviewing. At the selection stage, AI software can be used to perform background checks and predict offers.
Organisations may use different providers for the stages of the recruitment process and there are increasing options to integrate different types of tools.
Algorithms trained on historic data carry significant risks for bias
There are many ways bias can be introduced into the recruiting process when using data-driven technology. Decisions such as how data is collected, which variables to collect, how the variables are weighted, and the data the algorithm is trained on all have an impact and will vary depending on the context. However one theme that arises consistently is the risk of training algorithms on biased historical data.
High profile cases of biased recruiting algorithms include those trained using historical data on current and past employees within an organisation, which is then used to try and predict the performance of future candidates.[footnote 29] Similar systems are used for video interviewing software where existing employees or prospective applicants undertake the assessment and this is assessed and correlated in line with a performance benchmark.[footnote 30] The model is then trained on this data to understand the traits of people who are considered high performers.
Without rigorous testing, these kinds of predictive systems can pull out characteristics that have no relevance to job performance but are rather descriptive features that correlate with current employees. For example, one company developed a predictive model trained on their company data that found having the name “Jared” was a key indicator of a successful applicant.[footnote 31] This is an example where a machine learning process has picked up a very explicit bias, others are often more subtle but can be still as damaging. In the high profile case of Amazon, an application system trained on existing employees never made it past the development phase when testing showed that women’s CVs were consistently rated worse.[footnote 32] Pattern detection of this type is likely to identify various factors that correspond with protected characteristics if development goes unchecked, so it is essential that organisations interrogate their models to identify proxies or risk indirectly discriminating against protected groups.
Another way bias can arise is through having a dataset that is limited in respect to candidates with certain characteristics. For example, if the training set was from a company that had never hired a woman, the algorithm would be far less accurate in respect to female candidates. This type of bias arises from imbalance, and can easily be replicated across other demographic groups. Industry should therefore be careful about the datasets used to develop these systems both with respect to biases arising through historical prejudice, but also from unbalanced data.
Whilst most companies we spoke to evaluated their models to check that the patterns being detected did not correlate with protected characteristics, there is very little guidance or standards companies have to meet so it is difficult to evaluate the robustness of these processes.[footnote 33] Further detail can be found in Section 7.4 on the challenges and limitations of bias mitigation approaches.
Recruiting tool providers are largely self-regulated but tend to follow international standards
Currently guidance on discrimination within recruitment sits with the Equality and Human Rights Commission who oversee compliance with the Equality Act (2010) through the Employment Statutory Code of Practice setting out what fair recruitment looks like under the Equality Act. They also provide detailed guidance to employers on how to interpret and apply the Equality Act.[footnote 34] However there is not currently any guidance on how the Equality Act extends to algorithmic recruitment. This means providers of recruiting tools are largely self-regulating, and often base their systems on equality law in other jurisdictions, especially the US (where there have been some high profile legal cases in this area).[footnote 35]
Our research found that most companies test their tools internally and only some independently validate results. This has led to researchers and civil society groups calling for greater transparency around bias testing in recruiting algorithms as a way of assuring the public that appropriate steps have been taken to minimise the risk of bias.[footnote 36] We are now seeing some companies publish information on how their tools are validated and tested for bias.[footnote 37] However, researchers and civil society groups believe this has not gone far enough, calling for recruiting algorithms to be independently audited.[footnote 38] Further discussion of the regulatory landscape and auditing can be found in Chapter 8.
Recommendations to regulators
Recommendation 1: The Equality and Human Rights Commission should update its guidance on the application of the Equality Act 2010 to recruitment, to reflect issues associated with the use of algorithms, in collaboration with relevant industry and consumer bodies.
CDEI is happy to support this work if this would be helpful.
Collecting demographic data for monitoring purposes is increasingly widespread and helps to test models for biases and proxies
The only way to be sure a model is not directly or indirectly discriminating against a protected group is to check, and doing so requires having the necessary data. The practice of collecting data on protected characteristics is becoming increasingly common in recruitment as part of the wider drive to monitor and improve recruiting for underrepresented groups. This then allows vendors or recruiting organisations to test their models for proxies and monitor the drop-out rate of groups across the recruitment process. Compared to the other sectors we studied, recruitment is more advanced in the practice of collecting equality data for monitoring purposes. We found in our interviews that it is now standard practice to collect this data and provide applicants with disclaimers highlighting that the data will not be used as part of the process.
One challenge that was raised in our interviews was that some applicants may not want to provide this data as part of a job application, which is within their rights to withhold. We consider this issue in detail in Section 7.3 below, and conclude that clearer national guidance is needed to support organisations in doing this. Organisations should also be encouraged to monitor the overlap for people with multiple protected characteristics, as this may not be picked up through monitoring that only reviews data through a one-dimensional lens. This form of intersectional analysis is essential for ensuring people are not missed as a result of having multiple protected characteristics.[footnote 39]
Advice to employers and industry: Organisations should carry out equality impact assessments to understand how their models perform for candidates with different protected characteristics, including intersectional analysis for those with multiple protected characteristics.
In the US there is specific guidance setting the minimum level of drop-off allowed for applicants from protected groups before a recruitment process could be considered discriminatory. This is known as the “four-fifths rule” and was introduced as a mechanism to adjudicate on whether a recruitment process was considered to have had a disparate impact on certain groups of people.[footnote 40] We found in our research that many third-party software providers use these standards and some tools offer this feature as part of their platforms to assess the proportion of applicants that are moving through the process. However, the four-fifths rule is not part of UK law, and not a meaningful test of whether a system might lead to discrimination under UK law. It is therefore important for the EHRC to provide guidance on how the Equality Act 2010 applies (see Chapter 7 and Chapter 8 below for further discussion in this area).
Many tools are developed and used with fairness in mind
Although the most frequently cited reason for adopting data-driven technologies is efficiency, we found a genuine desire to use tools to make processes fairer. Where historically decisions about who to hire were made through referrals or unconscious biases. Recruiters also often do not have the relevant demographic data on applicants to know whether they are being fair in the criteria they are applying when looking for candidates. Many companies developing these tools want to provide less biased assessments of candidates by standardising processes and using more accurate assessment for candidates’ potential to succeed in a job. For example, one provider offers machine learning software that redacts parts of CVs associated with protected characteristics so those assessing the application can make a fairer judgement. Others try to equalise the playing field by developing games that assess core skills rather than relying on CVs which place weight on socio-demographic markers like educational institutions.
The innovation in this space has real potential for making recruitment less biased if developed and deployed responsibly.[footnote 41] However, the risks if they go wrong are significant because the tools are incorporating and replicating biases on a larger scale. Given the potential risks, there is a need for scrutiny in how these tools work, how they are used and the impact they have on different groups.
More needs to be done to ensure that data-driven tools can support reasonable adjustments for those who need them, or that alternative routes are available
One area where there is particular concern is how certain tools may work for those with disabilities. AI often identifies patterns related to a defined norm, however those with disabilities often require more bespoke arrangements because their requirements will likely differ from the majority, which may lead to indirect discrimination.[footnote 42] For example, someone with a speech impediment may be at a disadvantage in an AI assessed video interview, or someone with a particular cognitive disability may not perform as well in a gamified recruitment exercise. In the same way that reasonable adjustments are made for in-person interviews, companies should consider how any algorithmic recruitment process takes these factors into account, meeting their obligations under the Equality Act 2010.
Organisations should start by building inclusive design into their processes and include explicit steps for considering how certain tools may impact those with disabilities. This may include increasing the number of people with disabilities hired in development and design teams or offering candidates with disabilities the option of a human-assessed alternative route where appropriate. It is worth noting that some reports have found that AI recruitment could improve the experience for disabled applicants by reducing biases, however this will likely vary depending on the tools and the wide spectrum of barriers to progression faced by candidates with disabilities. A one size fits all approach is unlikely to be successful.
Automated rejections are governed by data protection legislation but compliance with guidance seems mixed
Most algorithmic tools in recruitment are designed to assist people with decision-making, however some fully automate elements of the process. This appears particularly common around automated rejections for candidates at application stage that do not meet certain requirements. Fully automated decision-making is regulated under Article 22 of the General Data Protection Regulation (GDPR), overseen by the Information Commissioner’s Office (ICO). The ICO have set out how this requirement should be operationalised for automated decision-making, with guidance that states organisations should be:
- giving individuals information about the processing;
- introducing simple ways for them to request human intervention or challenge a decision;
- carrying out regular checks to make sure that your systems are working as intended[footnote 43]
It is not clear how organisations screening many thousands of candidates should make provisions for the second of these suggestions, and indeed this is often not common practice for large scale sifts carried out by either algorithmic or non-algorithmic methods. Our research suggested the guidance was rarely applied in the way outlined above, particularly on introducing ways to request human intervention or review. We therefore think there would be value in the ICO working with employers to understand how this guidance (and the more detailed guidance set out in the Employment Practices Code) is being interpreted and applied, and consider how to ensure greater consistency in the application of the law so individuals are sufficiently able to exercise their rights under data protection.
Recommendations to regulators:
Recommendation 2: The Information Commissioner’s Office should work with industry to understand why current guidance is not being consistently applied, and consider updates to guidance (e.g. in the Employment Practices Code), greater promotion of existing guidance, or other action as appropriate.
Clearly it would be most helpful for the EHRC and ICO to coordinate their work to ensure that organisations have clarity on how data protection and equality law requirements interact; they may even wish to consider joint guidance addressing recommendations 1 and 2. Topics where there may be relevant overlaps include levels of transparency, auditing and recording recommended to improve standards of practice and ensure legal compliance. CDEI is happy to support this collaboration.
4. Financial services
Summary
Overview of findings:
-
Financial services organisations have long used data to support their decision-making. They range from being highly innovative to more risk averse in their use of new algorithmic approaches. For example, when it comes to credit scoring decisions, most banks are using logistic regression models rather than more advanced machine learning algorithms.
-
There are mixed views and approaches amongst financial organisations on the collection and use of protected characteristics data and this affects the ability of organisations to check for bias.
-
Explainability of models used in financial services, in particular in customer-facing decisions, is key for organisations and regulators to identify and mitigate discriminatory outcomes and for fostering customer trust in the use of algorithms.
-
The regulatory picture is clearer in financial services than in the other sectors we have looked at. The Financial Conduct Authority (FCA) is the lead regulator and is conducting work to understand the impact and opportunities of innovative uses of data and AI in the sector.
Future CDEI work:
- CDEI will be an observer on the Financial Conduct Authority and Bank of England’s AI Public Private Forum which will explore means to support the safe adoption of machine learning and artificial intelligence within financial services.
4.1 Background
Financial services organisations make decisions which have a significant impact on our lives, such as the amount of credit we can be offered or the price our insurance premium is set at. Algorithms have long been used in this sector but more recent technological advances have seen the application of machine learning techniques to inform these decisions.[footnote 4] Given the historical use of algorithms, the financial services industry is well-placed to embrace the most advanced data-driven technology to make better decisions about which products to offer to which customers.
However, these decisions are being made in the context of a socio-economic environment where financial resources are not spread evenly between different groups. For example, there is established evidence documenting the inequalities experienced by ethnic minorities and women in accessing credit, either as business owners or individuals, though these are generally attributed to wider societal and structural inequalities, rather than to the direct actions of lenders.[footnote 45] If financial organisations rely on making accurate predictions about peoples’ behaviours, for example how likely they are to repay debts like mortgages, and specific individuals or groups are historically underrepresented in the financial system, there is a risk that these historic biases could be entrenched further through algorithmic systems.[footnote 46]
In theory, using more data and better algorithms could help make these predictions more accurate. For example, incorporating non-traditional data sources could enable groups who have historically found it difficult to access credit, because of a paucity of data about them from traditional sources, to gain better access in future. At the same time, more complex algorithms could increase the potential of indirect bias via proxy as we become less able to understand how an algorithm is reaching its output and what assumptions it is making about an individual in doing so.
Difficulty in assessing credit discrimination by gender
New York’s Department of Financial Services’ investigated Goldman Sachs for potential credit discrimination by gender. This came from the web entrepreneur David Heinemeier Hansson who tweeted that the Apple Card, which Goldman manages, had given him a credit limit 20 times that extended to his wife, though the two filed joint tax returns and she had a better credit score. Goldman Sachs’ response was that it did not consider gender when determining creditworthiness, as this would be illegal in the US, and therefore there was no way they could discriminate on the basis of gender. The full facts around this case are not yet public, as the regulatory investigation is ongoing. Nonetheless, there is evidence that considering gender could help mitigate gender bias or at least test the algorithm to better understand whether it is biased. This example brings up a key challenge for financial organisations in terms of testing for bias which we will explore later in this chapter.
Current landscape
Despite plenty of anecdotal evidence, there has previously been a lack of structured evidence about the adoption of machine learning (ML) in UK financial services. In 2018, a Financial Times global survey of banks provided evidence of a cautious approach being taken by firms.[footnote 48] However, in 2019, the Bank of England and FCA conducted a joint survey into the use of ML in financial services, which was the first systematic survey of its kind. The survey found that ML algorithms were increasingly being used in UK financial services, with two thirds of respondents[footnote 49] reporting its use in some form and the average firm using ML applications in two business areas.[footnote 50] It also found that development is entering the more mature stages of deployment, in particular in the banking and insurance sectors.
The survey focused on ML algorithms in financial services, rather than rules-based algorithms. The key difference being that a human does not explicitly programme an ML algorithm, but instead computer programmes fit a model or recognise patterns from data. Many ML algorithms constitute an incremental, rather than fundamental, change in statistical methods used in financial services. They also provide more flexibility as they are not constrained by the linear relationships often imposed in traditional economic and financial analysis and can often make better predictions than traditional models or find patterns in large amounts of data from increasingly diverse sources.
The key uses of ML algorithms in financial services are to inform back-office functions, such as risk management and compliance. This can include identifying third parties who are trying to damage customers, or the bank itself, through fraud, identity theft and money laundering. This is the area where ML algorithms find the highest extent of application due to their ability to connect large datasets and pattern detection. However, ML algorithms are also increasingly being applied to front-office areas, such as credit scoring, where ML applications are used in granting access to credit products such as credit cards, loans and mortgages.
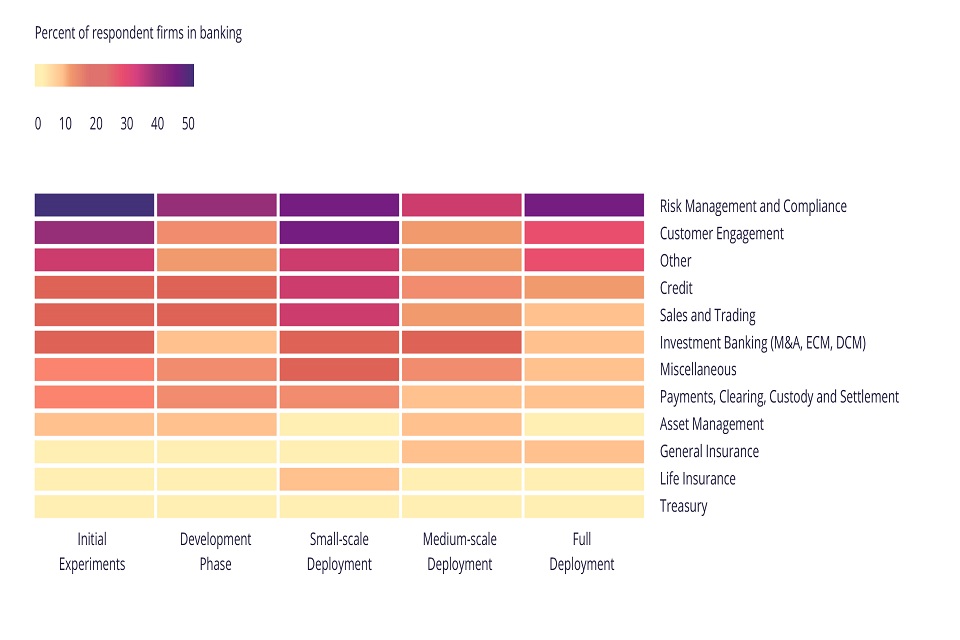
The Financial Conduct Authority and the Bank of England conducted a survey about the maturity of machine learning tools, by business area within financial services. The survey results are organised by the following levels of deployment: “initial experiments”, “development phase”, “small-scale deployment”, “medium-scale deployment” and “full deployment.” Roughly 30% to 50% of respondents reported “risk management”, “customer engagement” and “other” use cases were in “initial experiments”, “small-scale deployment” and “full deployment”. Approximately 20% to 30% of respondents reported that “credit” and “sales and trading” were in “small-scale deployment”. Around 10% to 20% of respondents reported that “customer engagement”, “other”, “credit”, “sales and trading”, “investment banking (M&A, ECM, DCM)” and “miscellaneous” were being deployed at all levels. Between 0% and 10% of respondents stated that “asset management’, “general insurance”, “life insurance” and “treasury” uses were present at all levels of deployment.
Figure 3: Machine learning maturity of different business areas in financial services, as surveyed by the FCA and Bank of England[footnote 51]
The underlying methodology behind these different applications varies from more traditional methods such as logistic regression and random forest models, to more advanced machine learning and natural language processing. There are varying reports on how widely the most advanced tools are being used. For example, the FCA and Bank of England report highlights how many cases of ML development have entered into the more advanced stages of deployment, in particular in the banking and insurance sectors.[footnote 52]
We have seen a real acceleration in the last five years with machine learning and deep learning being more widely adopted in financial services. We do not see this slowing down. - CDEI interview with leading credit reference agency
The Bank of England has identified the following potential benefits of increased use of algorithmic decision-making in financial services: improved customer choice, services and more accurate pricing; increased access to credit for households and SMEs; substantially lower cross border transaction costs; and improved diversity and resilience of the system.[^53] However, there are obstacles to the adoption of algorithmic decision-making in financial services. Organisations report these to be mainly internal to firms themselves, rather than stemming from regulation, and range from lack of data accessibility, legacy systems, and challenges integrating ML into existing business processes.[footnote 54]
The FCA is the lead sector regulator for financial services and regulates 59,000 financial services firms and financial markets in the UK and is the prudential regulator for over 18,000 of those firms. The FCA and Bank of England recently jointly announced that they would be establishing the Financial Services Artificial Intelligence Public-Private Forum (AIPPF).[footnote 55] The Forum was set up in recognition that work is needed to better understand how the pursuit of algorithmic decision-making and increasing data availability are driving change in financial markets and consumer engagement, and a wide range of views need to be gathered on the potential areas where principles, guidance or good practice examples could support the safe adoption of these technologies.
4.2 Findings
Our main focus within financial services has been on credit scoring decisions made about individuals by traditional banks. We did not look in detail at how algorithms are being used by fintech companies and in the insurance industry, but do incorporate key trends and findings from these areas in our Review. We also separately conducted a short piece of research on AI in personal insurance.[footnote 56] In order to understand the key opportunities and risks with regards to the use of algorithms in the financial sector we conducted semi-structured interviews with financial services organisations, predominantly traditional banks and credit reference agencies. We also ran an online experiment with the Behavioural Insights Team to understand people’s perceptions of the use of algorithms in credit scoring and how fair they view the use of data which could act as a proxy for sex or ethnicity, particularly newer forms of data, such as social media, in informing these algorithms.
On the whole, the financial organisations we interviewed range from being very innovative to more risk averse with regards to the models they are building and the data sources they are drawing on. However, they agreed that the key obstacles to further innovation in the sector were as follows:
- Data availability, quality and how to source data ethically
- Available techniques with sufficient explainability
- A risk averse culture, in some parts, given the impacts of the financial crisis
- Difficulty in gauging consumer and wider public acceptance
Algorithms are mainly trained using historical data, with financial organisations being hesitant to incorporate newer, non-traditional, data-sets
In our interviews, organisations argued that financial data would be biased due to the fact that, in the past, mainly men have participated in the financial system. One could also see another data-related risk in the fact that there are fewer training datasets for minority communities might result in the reduced performance of investment advice algorithms for these communities.
A key challenge is posed by data… the output of a model is only as good as the quality of data fed into it – the so-called “rubbish in, rubbish out”[footnote 57] problem… AI/ML is underpinned by the huge expansion in the availability and sources of data: as the amount of data used grows, so the scale of managing this problem will increase.[footnote 58] - James Proudman, Bank of England
On the whole, financial organisations train their algorithms on historical data. The amount of data that a bank or credit reference agency has at its disposal varies. We know from our interview with one of the major banks that they use data on the location of transactions made, along with data they share with other companies to identify existing credit relationships between banks and consumers. In the case of a credit reference agency we spoke to, models are built on historical data, but are trained on a variety of public sources including applications made on the credit market, the electoral registry, public data, such as filing for bankruptcy, data provided by the clients themselves, and behavioural information such as: turnover, returned items, rental data.
In terms of using non-traditional forms of data, the phenomenon of credit-worthiness by association[footnote 59] describes the move from credit scoring algorithms just using data from an individual’s credit history, to drawing on additional data about an individual for example their rent repayment history or their wider social network. Of the companies we spoke to, most were not using social media data and were sceptical of its value. For example, a credit reference agency and major bank we interviewed had explored using social media data a few years ago, but decided against it as they did not believe it would sufficiently improve the accuracy of the algorithm to justify its use.
The use of more data from non-traditional sources could enable population groups who have historically found it difficult to access credit, due to there being less data about them from traditional sources, to gain better access in future. For example in our interview with a credit reference agency they spoke of customers who are referred to as “thin files”, as there is little data available about them, which could be a source of financial exclusion. Their approach with these customers is to ensure decisions about them are subjected to manual review. In order to address the problem of “thin files”, Experian added rent repayments to the credit reports of more than 1.2 million tenants in the UK with the intention of making it easier for renters to access finance deals.[footnote 60]
While having more data could improve inclusiveness and improve the representativeness of datasets, more data and more complex algorithms could also increase the potential for the introduction of indirect bias via proxy as well as the ability to detect and mitigate it.
Although there is a general standard not to collect protected characteristics data, financial organisations are developing approaches to testing their algorithms for bias
It is common practice to avoid using data on protected characteristics, or proxies for those characteristics, as inputs into decision-making algorithms, as to do so is likely to be unlawful or discriminatory. However, understanding the distribution of protected characteristics among the individuals affected by a decision is necessary to identify biased outcomes. For example, it is difficult to establish the existence of a gender pay gap at a company without knowing whether each employee is a man or woman. This tension between the need to create algorithms which are blind to protected characteristics while also checking for bias against those same characteristics creates a challenge for organisations seeking to use data responsibly. This means that whilst organisations will go to lengths to ensure they are not breaking the law or being discriminatory, their ability to test how the outcomes of their decisions affect different population groups is limited by the lack of demographic data. Instead organisations test their model’s accuracy through validation techniques[footnote 61] and ensuring sufficient human oversight of the process as a way of managing bias in the development of the model.
Case study - London fintech company
We spoke to a London fintech company which uses supervised ML in order to predict whether people are able to repay personal loans and to detect fraud. In line with legislation, they do not include protected characteristics in their models, but to check for bias they adopt a ‘fairness through unawareness’ approach involving ongoing monitoring and human judgement. The ongoing monitoring includes checking for sufficiency across the model performance, business optimisation and building test models to counteract the model. The human judgement involves interpreting the direction in which their models are going and if a variable does not fit the pattern rejecting or transforming it. This approach requires significant oversight to ensure fair operation and to effectively mitigate bias.
Some organisations do hold some protected characteristic data, which they do not use in their models. For example, a major bank we interviewed has access to sex, age and postcode data on their customers, and can test for bias on the basis of sex and age. Moreover, banks advise that parameters that they consider to strongly correlate with protected characteristics are usually removed from the models. Given there is no defined threshold for bias imposed by the FCA or any standards body, organisations manage risks around algorithmic bias using their own judgement and by managing data quality. A small proportion of companies analyse model predictions on test data, such as representative synthetic data or anonymised public data.
The extent to which a problem of algorithmic bias exists in financial services is still relatively unclear given that decisions around finance and credit are often highly opaque for reasons of commercial sensitivity and competitiveness. Even where it is apparent that there are differences in outcomes for different demographic groups, without extensive access to the models used by companies in their assessments of individuals, and access to protected characteristic data, it is very difficult to determine whether these differences are due to biased algorithms or to underlying societal, economic or structural causes.
Insights from our work in financial services have fed into our wider recommendation around collecting protected characteristics data which is set out in Chapter 7.
Case study: Bias in insurance algorithms
A Propublica investigation in the US found that people in minority neighbourhoods on average paid higher car insurance premiums than residents of majority-white neighbourhoods, despite having similar accident costs. While the journalists could not confirm the cause of these differences, they suggest biased algorithms may be to blame.
Like any organisation using algorithms to make significant decisions, insurers must be mindful of the risks of bias in their AI systems and take steps to mitigate unwarranted discrimination. However, there may be some instances where using proxy data may be justified. For example, while car engine size may be a proxy for sex, it is also a material factor in determining damage costs, giving insurers more cause to collect and process information related to it. Another complication is that insurers often lack the data to identify where proxies exist. Proxies can in theory be located by checking for correlations between different data points and the protected characteristic in question (e.g. between the colour of a car and ethnicity). Yet insurers are reluctant to collect this sensitive information for fear of customers believing the data will be used to directly discriminate against them.
The Financial Conduct Authority conducted research in 2018 on the pricing practices of household insurance firms. One of the key findings was the risk that firms could discriminate against consumers by using rating factors in pricing based either directly or indirectly on data relating to or derived from protected characteristics. The FCA has since done further work, including a market study and initiating a public debate, on fair pricing and related possible harms in the insurance industry.
Ensuring explainability of all models used in financial services is key
Explainability refers to the ability to understand and summarise the inner workings of a model, including the factors that have gone into the model. As set out in Section 2.5, explainability is key to understanding the factors causing variation in outcomes of decision-making systems between different groups and to assess whether or not this is regarded as fair. In polling undertaken for this review, of the possible safeguards which could be put in place to ensure an algorithmic decision-making process was as fair as possible, an easy to understand explanation came in second in a list of six options, after only human oversight.
In the context of financial services, the explainability of an algorithm is important for regulators, banks and customers. For banks, when developing their own algorithms, explainability should be a key requirement in order to have better oversight of what their systems do and why, so they can identify and mitigate discriminatory outcomes. For example when giving loans, using an explainable algorithm makes it possible to examine more directly the degree to which relevant characteristics are acting as a proxy for other characteristics, and causing differences in outcomes between different groups. This means that where there may be valid reasons for loans to be disproportionately given to one group over another this can be properly understood and explained.
For customers, explainability is crucial so that they can understand the role the model has played in the decision being made about them. For regulators, understanding how an algorithmically-assisted decision was reached is vital to knowing whether an organisation has met legal requirements and treated people fairly in the process. Indeed, the expert panel we convened for our AI Barometer discussions, viewed a lack of explainability for regulators as a significantly greater risk than a lack of transparency for consumers of algorithmic decision-making in financial services.[footnote 65]
The lack of explainability of machine learning models was highlighted as one of the top risks by respondents to the Bank of England and FCA’s survey. The survey highlighted that in use cases such as credit scoring where explainability was a priority, banks were opting for logistic regression techniques, with ML elements, to ensure decisions could be explained to customers where required. However, research by the ICO[footnote 66] has shown that while some organisations in banking and insurance are continuing to select interpretable models in their customer-facing AI decision-support applications, they are increasingly using more opaque ‘challenger’ models alongside these, for the purposes of feature engineering or selection, comparison, and insight.
In our interviews with banks they reported using tree-based models, such as ‘random forests’, as they claim they generate the most accurate predictions. However, they acknowledged that the size and complexity of the models made it difficult to explain exactly how they work and the key variables that drive predictions. As a result, logistic regression techniques with ML elements continue to be popular in this type of use case, and provide a higher degree of apparent explainability.
There are approaches to breaking down the procedures of neural networks in order to justify why a decision is made about a particular customer or transaction. In our interview with a credit reference agency they described an example in which their US team had calculated the impact that every input parameter had on the final score and then used this information to return the factors that had the biggest impact, in a format that was customer-specific but still general enough to work across the entire population. This then means a low credit score could be explained with a simple statement such as “rent not paid on time”. Nonetheless, even if there are approaches to explain models at a system level and understand why credit has been denied, these are not always directly available as individual level explanations to customers and it may be difficult to assign it to one factor, rather than a combination.
In other cases, firms are required to provide information about individual decisions. This includes under the GDPR (Articles 13, 14, 15 and 22 in particular), under FCA rules for lenders and under industry standards such as the Standards of Lending Practice.
The risks to explainability may not always come from the type of model being used, but from other considerations for example commercial sensitivities or concerns that people may game or exploit a model if they know too much about how it works. Interestingly, public attitudes research conducted by the RSA suggested that customers could consider some circumstances in which commercial interests could supersede individuals’ rights, for example when making financial decisions, in recognition that providing a detailed explanation could backfire by helping fraudsters outwit the system, and where such interests should be overruled. The firms we interviewed reported mostly designing and developing tools in-house, apart from sometimes procuring from third-parties for the underlying platforms and infrastructure such as cloud computing, which should mean that intellectual property considerations do not impinge on explainability standards. Nonetheless, where there may be commercial sensitivities, concerns around gaming, or other risks these should be clearly documented from the outset and justified in the necessary documentation.
There are clear benefits to organisations, individuals and society in explaining algorithmic decision-making in financial services. Providing explanations to individuals affected by a decision can help organisations ensure more fairness in the outcomes for different groups across society. Moreover, for organisations it makes business sense as a way of building trust with your customers by empowering them to understand the process and providing them the opportunity to challenge where needed.
ICO and the Alan Turing Institute’s Explainability Guidance
In May 2020, the ICO and the Alan Turing Institute published their guidance to organisations on how to explain decisions made with AI, to the individuals affected by them. The guidance sets out key concepts and different types of explanations, along with more tailored support to senior management teams on policies and procedures organisations should put in place to ensure they provide meaningful explanations to affected individuals. The FCA has fed into this guidance to ensure it takes into account the opportunities and challenges facing banks in explaining AI-assisted decisions to customers.
Public acceptability of the use of algorithms in financial services is higher than in other sectors, but can be difficult to gauge
In polling undertaken for this review, when asked how aware they were of algorithms being used to support decisions in the context of the four sectors we have looked at in this report, financial services was the only option selected by a majority of people (around 54-57%). This was in contrast to only 29-30% of people being aware of their use in local government.
In our interviews with financial companies, it was evident they were making efforts to understand public acceptability, mainly in the context of their customers. For example, financial organisations we interviewed had conducted consumer polling and focus groups to understand how the public felt about the use of payment data. In another interview, we learnt that a bank gauged public acceptability with a focus on customer vulnerability, by conducting surveys and interviews, but also by considering the impact of a new product on customers through their risk management framework. Moreover, each product goes through an annual review, which takes into account if there have been any problems, for example customer complaints.
In order to better understand public attitudes we conducted a public engagement exercise with the Behavioural Insights Team (BIT)[footnote 69] through their online platform, Predictiv. We measured participants’ perceptions of fairness of banks’ use of algorithms in loan decisions. In particular we wanted to understand how people’s fairness perceptions of banking practices varied depending on the type of information an algorithm used in a loan decision, for example the use of a variable which could serve as a proxy for a protected characteristic such as sex or ethnicity.
We found that, on average, people moved twice as much money away from banks that use algorithms in loan application decisions, when told that the algorithms draw on proxy data for protected characteristics or social media data. Not surprisingly, those historically most at risk of being discriminated against in society feel most strongly that it is unfair for a bank to use proxy information for protected characteristics. For example, directionally, women punish the bank that used information which could act as a proxy for sex more strongly than men. However, some people thought it was fair to use the proxy variable if it produced a more accurate result. This brings into question whether there are legitimate proxies, for example salary, which although could function as proxies for sex and ethnicity, may also accurately assist a bank in making decisions around loan eligibility. The experiment also found that people are less concerned about the use of social media data than about data that relates to sex and ethnicity. However, the frequency with which an individual uses social media does not have an impact on how concerned they are about its use in informing loan decisions.
This experiment highlighted the challenges in framing questions about a bank’s use of algorithms in an unbiased and nuanced way. More research is needed into the use of proxies and non-traditional forms of data in financial services to give financial organisations the confidence that they are innovating in a way that is deemed acceptable by the public.
Regulation on bias and fairness in financial services is currently not seen as an unjustified barrier to innovation, but additional guidance and support would be beneficial
The majority (75%) of respondents to the FCA and the Bank of England’s survey, said that they did not consider Prudential Regulation Authority[footnote 70]/ FCA regulations an unjustified barrier to deploying ML algorithms. This view was supported by organisations we interviewed. This may be because the FCA has responded constructively to the increased use of ML algorithms and is proactively finding ways to support ethical innovation. However, there were respondents in the survey who noted challenges of meeting regulatory requirements to explain decision-making when using more advanced, complex algorithms. Moreover, firms also highlighted that they would benefit from additional guidance from regulators on how to apply existing regulations to ML.
Whilst it is positive that the FCA is seen as a constructive, innovation-enabling regulator, future regulation may need to be adapted or adjusted to account for developments in ML algorithms in order to protect consumers. The AIPPF will be well-placed to identify where this may be the case whilst also identifying good practice examples.
Future CDEI work: CDEI will be an observer on the Financial Conduct Authority and Bank of England’s AI Public Private Forum which will explore means to support the safe adoption of machine learning and artificial intelligence within financial services.
5. Policing
Summary
Overview of findings:
-
Adoption of algorithmic decision-making is at an early stage, with very few tools currently in operation in the UK. There is a varied picture across different police forces, both on levels of usage and approaches to managing ethical risks.
-
Police forces have identified opportunities to use data analytics and AI at scale to better allocate resources, but there is a significant risk that without sufficient care systematically unfair outcomes could occur.
-
The use of algorithms to support decision-making introduces new issues around the balance between security, privacy and fairness. There is a clear requirement for strong democratic oversight of this and meaningful engagement with the public is needed on which uses of police technology are acceptable.
-
Clearer national leadership is needed around the ethical use of data analytics in policing. Though there is strong momentum in data ethics in policing at a national level, the picture is fragmented with multiple governance and regulatory actors and no one body fully empowered or resourced to take ownership. A clearer steer is required from the Home Office.
Recommendation to government:
- Recommendation 3: The Home Office should define clear roles and responsibilities for national policing bodies with regards to data analytics and ensure they have access to appropriate expertise and are empowered to set guidance and standards. As a first step, the Home Office should ensure that work underway by the National Police Chiefs’ Council and other policing stakeholders to develop guidance and ensure ethical oversight of data analytics tools is appropriately supported.
Advice to police forces/ suppliers:
-
Police forces should conduct an integrated impact assessment before investing in new data analytics software as a full operational capability, to establish a clear legal basis and operational guidelines for use of the tool. Further details of what the integrated impact assessment should include are set out in the report we commissioned from RUSI.
-
Police forces should classify the output of statistical algorithms as a form of police intelligence, alongside a confidence rating indicating the level of uncertainty associated with the prediction.
-
Police forces should ensure that they have appropriate rights of access to algorithmic software and national regulators should be able to audit the underlying statistical models if needed (for instance, to assess risk of bias and error rates). Intellectual property rights must not be a restriction on this scrutiny.
Future CDEI work:
- CDEI will be applying and testing its draft ethics framework for police use of data analytics with police partners on real-life projects and developing underlying governance structures to make the framework operational.
5.1 Background
There have been notable government reviews into the issue of bias in policing which are important when considering the risks and opportunities around the use of technology in policing. For example, the 2017 Lammy Review[footnote 71] found that BAME individuals faced bias, including overt discrimination, in parts of the justice system. And prior to the Lammy Review, the 1999 public inquiry into the fatal stabbing of Black teenager Stephen Lawrence branded the Metropolitan Police force “institutionally racist”[footnote 72].More recently, the 2017 Race Disparity Audit[footnote 73] highlighted important disparities in treatment and outcomes for BAME communities along with lower levels of confidence in the police among younger Black adults. With these findings in mind, it is vital to consider historic and current disparities and inequalities when looking at how algorithms are incorporated into decision-making in policing. Whilst there is no current evidence of police algorithms in the UK being racially biased, one can certainly see the risks of algorithms entrenching and amplifying widely documented human biases and prejudices, in particular against BAME individuals, in the criminal justice system.
The police have long been under significant pressure and scrutiny to predict, prevent and reduce crime. But as Martin Hewitt QPM, Chair of the National Police Chiefs’ Council (NPCC) and other senior police leaders, have highlighted that “the policing environment has changed profoundly in many ways and the policing mission has expanded in both volume and complexity. This has taken place against a backdrop of diminishing resources”.[footnote 74]
Prime Minister Boris Johnson’s announcement to recruit 20,000 new police officers, as one of his headline policy pledges[footnote 75], signals a government commitment to respond to mounting public unease about local visibility of police officers. But the decentralised nature of policing in England and Wales means that each force is developing their own plan for how to respond to these new pressures and challenges.
Police forces have access to more digital material than ever before[footnote 76], and are expected to use this data to identify connections and manage future risks. Indeed, the £63.7 million ministerial funding announcement [footnote 77] in January 2020 for police technology programmes, amongst other infrastructure and national priorities, demonstrates the government’s commitment to police innovation.
In response to these incentives to innovate, some police forces are looking to data analytics tools to derive insight, inform resource allocation and generate predictions. But drawing insights and predictions from data requires careful consideration, independent oversight and the right expertise to ensure it is done legally, ethically and in line with existing policing codes[footnote 78]. Despite multiple legal frameworks and codes setting out clear duties, the police are facing new challenges in adhering to the law and following these codes in their development and use of data analytics.
Case study: Innovation in Avon and Somerset Constabulary
Avon and Somerset Constabulary have been successful in building in-house data science expertise through their Office for Data Analytics. One of their tools is Qlik Sense, a software product that connects the force’s own internal databases and other local authority datasets. It applies predictive modelling to produce individual risk-assessment and intelligence profiles, to assist the force in triaging offenders according to their perceived level of risk.
Although Avon and Somerset Constabulary do not operate a data ethics committee model, like West Midlands Police, they do have governance and oversight processes in place. Moreover, their predictive models are subject to ongoing empirical validation, which involves revisiting models on a quarterly basis to ensure they are accurate and adding value.
In theory, tools which help spot patterns of activity and potential crime, should lead to more effective prioritisation and allocation of scarce police resources. A range of data driven tools are being developed and deployed by police forces including tools which help police better integrate and visualise their data, tools which help guide resource allocation decisions and those that inform decisions about individuals such as someone’s likelihood to reoffend. However, there is a limited evidence base regarding the claimed benefits, scientific validity or cost effectiveness of police use of algorithms[footnote 79]. For example, there is empirical evidence around the effectiveness of actuarial tools to predict reoffending. However, experts disagree over the statistical and theoretical validity of individual risk-assessment tools. More needs to be done to establish benefits of this technology. In order to do this the technology must be tested in a controlled, proportionate manner, following national guidelines.
The use of data-driven tools in policing also carries significant risk. The Met Police’s Gangs Matrix[footnote 80] is an example of a highly controversial intelligence and prioritisation tool in use since 2011. The tool intends to identify those at risk of committing, or being a victim of, gang-related violence in London. Amnesty International raised serious concerns with the Gangs Matrix in 2018, in particular that it featured a disproportionate number of Black boys and young men and people were being kept on the database despite a lack of evidence and a reliance on out-of-date information[footnote 81]. In addition, the Gangs Matrix was found by the Information Commissioner’s Office to have breached data protection laws and an enforcement notice was issued to the Met Police[footnote 82]. Since, the Mayor of London, Sadiq Khan announced an overhaul[footnote 83] of the Gangs Matrix highlighting that the number of people of a Black African Caribbean background added to the database had dropped from 82.8 per cent in 2018 to 66 per cent in 2019. The Gangs Matrix is likely to continue to be closely scrutinised by civil society, regulators and policymakers.
It is evident that without sufficient care, the use of intelligence and prioritisation tools in policing can lead to outcomes that are biased against particular groups, or systematically unfair in other regards. In many scenarios where these tools are helpful, there is still an important balance to be struck between automated decision-making and the application of professional judgement and discretion. Where appropriate care has been taken internally to consider these issues fully, it is critical for public trust in policing that police forces are transparent in how such tools are being used.
Our approach
Given the breadth of applications and areas where technology is being used in law enforcement we chose to focus on the use of data analytics in policing to derive insights, inform operational decision-making or make predictions. This does not include biometric identification, automated facial recognition[footnote 84], digital forensics or intrusive surveillance. However, some of the opportunities, risks and potential approaches that we discuss remain relevant to other data-driven technology issues in policing.
To build on and strengthen existing research and publications on these issues[footnote 85] we commissioned new, independent research from the Royal United Services Institute (RUSI).[footnote 86] The aim of this research was to identify the key ethical concerns, in particular on the issue of bias, and propose future policy to address these issues. We incorporated the findings in RUSI’s Report[footnote 87] into this chapter and, where relevant, throughout this report.
We also issued a call for evidence on the use of algorithmic tools, efforts to mitigate bias, engagement with the public on these issues, and governance and regulation gaps across the four sectors addressed in this report, including policing, receiving a diverse range of responses.
We have conducted extensive stakeholder engagement over the last year to understand the key challenges and concerns about the development and use of data analytics tools in this sector. For example, we have spoken to local police forces, including Avon and Somerset, Durham, Essex, Hampshire, Police Scotland and South Wales.
Working with West Midlands Police
West Midlands Police are one of the leading forces in England and Wales in the development of data analytics. They have an in-house data analytics lab and are the lead force on the National Data Analytics Solution. Their PCC has also set up an Ethics Committee to review data science projects developed by the lab and advise the PCC and Chief Constable on whether the proposed project has sufficiently addressed legal and ethical considerations. We have met with representatives at West Midlands Police and the PCC’s Office multiple times throughout this project and we were invited to observe a meeting of their ethics committee. They were also interviewed for and contributed to the RUSI report and development of our policing framework. We are interested in seeing, going forward, to what extent other forces follow the West Midlands PCC Ethics Committee model and hope to continue working closely with West Midlands on future policing work.
We established a partnership with the Cabinet Office’s Race Disparity Unit (RDU), a UK Government unit which collates, analyses and publishes government data on the experiences of people from different ethnic backgrounds in order to drive policy change where disparities are found. We have drawn on their expertise to better understand how algorithmic decision-making could disproportionately impact ethnic minorities. Our partnership has included jointly meeting with police forces and local authorities, along with the RDU and their Advisory Group contributing to our roundtables with RUSI and reviewing our report and recommendations.
We have met with senior representatives from policing bodies including the National Police Chiefs’ Council (NPCC), Her Majesty’s Inspectorate of Constabulary and Fire Rescue Services (HMICFRS), the Police ICT Company, the College of Policing, the Association of Police and Crime Commissioners (APCC), and regulators with an interest in this sector, including the Information Commissioner’s Office. We have also engaged with teams across the Home Office, with an interest in police technology.
A draft framework to support police to develop data analytics ethically
CDEI has been developing a Draft Framework to support police in innovating ethically with data. It is intended for police project teams developing or planning to develop data analytics tools. It should also help senior decision-makers in the police identify the problems best addressed using data analytics along with those not suited to a technological solution. The Framework is structured around the agile delivery cycle and sets out the key questions that should be asked at each stage. We have tested the Framework with a small group of stakeholders from police forces, academics and civil society and plan to release it more widely following the publication of this review. The feedback we have received to date has also highlighted that a well-informed public debate around AI in policing is missing. These are complex issues where current public commentary is polarised. But without building a common consensus on where and how it is acceptable for police to use AI, the police risk moving ahead without public buy-in. CDEI will be exploring options for facilitating that public conversation going forward and testing the Framework with police forces.
Future CDEI work: CDEI will be applying and testing its draft ethics framework for police use of data analytics with police partners on real-life projects and developing underlying governance structures to make the framework operational.
5.2 Findings
Algorithms are in development and use across some police forces in England and Wales but the picture is varied
From the responses we received to our call for evidence and wider research, we know there are challenges in defining what is meant by an algorithmic tool and consequently understanding the extent and scale of adoption. In line with this, it is difficult to say with certainty how many police forces in England and Wales are currently developing, trialling or using algorithms due in part to different definitions and also a lack of information sharing between forces.
The RUSI research surfaced different terms being used to refer to data analytics tools used by police forces. For example, several interviewees considered the term ‘predictive policing’ problematic. Given that many advanced analytics tools are used to ‘classify’ and ‘categorise’ entities into different groups, it would be more accurate to describe them as tools for ‘prioritisation’ rather than ‘prediction’. For instance, ‘risk scoring’ offenders according to their perceived likelihood of reoffending by comparing selected characteristics within a specified group does not necessarily imply that an individual is expected to commit a crime. Rather, it suggests that a higher level of risk management is required than the level assigned to other individuals within the same cohort.
RUSI sorted the application of data analytics tools being developed by the police into the following categories:
- Predictive mapping: the use of statistical forecasting applied to crime data to identify locations where crime may be most likely to happen in the near future. Recent data suggests that 12 of 43 police forces in England and Wales are currently using or developing such systems.[footnote 89]
- Individual risk assessment: the use of algorithms applied to individual-level personal data to assess risk of future offending. For example, the Offender Assessment System (OASys) and the Offender Group Reconviction Scale (OGRS), routinely used by HM Prison and Probation Service (HMPPS) to measure individuals’ likelihood of reoffending and to develop individual risk management plans.[footnote 90]
-
Data scoring tools: the use of advanced machine learning algorithms applied to police data to generate ‘risk’ scores of known offenders.
-
Other: complex algorithms used to forecast demand in control centres, or triage crimes for investigation according to their predicted ‘solvability’.
Examples of the data scoring tools include:
-
A Harm Assessment Risk Tool (HART), developed and being deployed by Durham police. It uses supervised machine learning to classify individuals in terms of their likelihood of committing a violent or non-violent offence within the next two years.
-
Use of Qlik Sense (a COTS analytics platform) by Avon and Somerset to link data from separate police databases to generate new insights into crime patterns.
-
The Integrated Offender Management Model, in development but not currently deployed by West Midlands Police. It makes predictions as to the probability that an individual will move from committing low / middling levels of harm, via criminal activity, to perpetrating the most harmful offending.
There have also been reports of individual forces buying similar technology, for example the Origins software which is reportedly currently being used by the Metropolitan Police Service and has previously been used by several forces including Norfolk, Suffolk, West Midlands and Bedfordshire police forces[footnote 91]. The software intends to help identify whether different ethnic groups “specialise” in particular types of crime and has come under strong critique from equality and race relations campaigners who argue that it is a clear example of police forces racial profiling at a particularly fraught time between police and the Black community.
In England and Wales, police forces are currently taking a variety of different approaches to their development of algorithmic systems, ethical safeguards, community engagement and data science expertise.
Mitigating bias and ensuring fairness requires looking at the entire decision-making process
As set out earlier in the report, we think it is crucial to take a broad view of the whole decision-making process when considering the different ways bias can enter a system and how this might impact on fairness. In the context of policing, this means not only looking at the development of an algorithm, but also the context in which it is deployed operationally.
At the design and testing stage, there is a significant risk of bias entering the system due to the nature of the police data which the algorithms are trained on. Police data can be biased due to it either being unrepresentative of how crime is distributed or in more serious cases reflecting unlawful policing practices. It is well-documented[footnote 92] that certain communities are over or under-policed and certain crimes are over or under-reported. For example, a police officer interviewed in our RUSI research, highlighted that ‘young Black men are more likely to be stopped and searched than young white men, and that’s purely down to human bias’. Indeed this is backed by Home Office data released last year stating that those who identify as Black or Black British are 9.7 times more likely to be stopped and searched by an officer than a white person[footnote 93]. Another way police data can provide a misrepresentative picture is that individuals from disadvantaged socio demographic backgrounds are likely to engage with public services more frequently, which means that more data is held on them. Algorithms could then risk calculating groups with more data held on them by the police as posing a greater risk. Further empirical research is needed to assess the level of bias in police data and the impact of that potential bias.
A further challenge to be considered at this stage is the use of sensitive personal data to develop data analytics tools. Whilst models may not include a variable for race, in some areas postcode can function as a proxy variable for race or community deprivation, thereby having an indirect and undue influence on the outcome prediction. If these biases in the data are not understood and managed early on this could lead to the creation of a feedback loop whereby future policing, not crime, is predicted. It could also influence how high or low risk certain crimes or areas are deemed by a data analytics tool and potentially perpetuate or exacerbate biased criminal justice outcomes for certain groups or individuals.
At the deployment stage, bias may occur in the way the human decision-maker receiving the output of the algorithm responds. One possibility is that the decision-maker over-relies on the automated output, without applying their professional judgement to the information. The opposite is also possible, where the human decision-maker feels inherently uncomfortable with taking insights from an algorithm to the point where they are nervous to use it at all[footnote 94], or simply ignores it in cases where their own human bias suggests a different risk level. A balance is important to ensure due regard is paid to the insights derived, whilst ensuring the professional applies their expertise and understanding of the wider context and relevant factors. It has been argued, for example by Dame Cressida Dick in her keynote address at the launch event of the CDEI/RUSI report on data analytics in policing, that police officers may be better equipped than many professionals to apply a suitable level of scepticism to the outcome of an algorithm, given that weighing the reliability of evidence is so fundamental to their general professional practice.
Without sufficient care of the multiple ways bias can enter the system, outcomes can be systematically unfair and lead to bias and discrimination against individuals or those within particular groups.
There is a need for strong national leadership on the ethical use of data analytics tools
The key finding from the RUSI research was a “widespread concern across the UK law enforcement community regarding the lack of any official national guidance for the use of algorithms in policing, with respondents suggesting that this gap should be addressed as a matter of urgency.”[footnote 95] Without any national guidance, initiatives are being developed to different standards and to varying levels of oversight and scrutiny.
For example, while all police forces in England and Wales have established local ethics committees, these are not currently resourced to look at digital projects. Instead, some Police and Crime Commissioners, like West Midlands, have established data ethics committees to provide independent ethical oversight to police data analytics projects. However, given the absence of guidance it is unclear whether each force should be setting up data ethics committees, upskilling existing ones, or whether regional or a centralised national structure should be set up to provide digital ethical oversight to all police forces. A review of existing police ethics committees would be useful in order to develop proposals for ethical oversight of data analytics projects.
Similarly, the lack of national coordination and oversight means that data initiatives are developed at a local level. This can lead to pockets of innovation and experimentation. However, it also risks meaning that efforts are duplicated, knowledge and lessons are not transferred across forces and systems are not made interoperable. As described by a senior officer interviewed as part of the RUSI project, “it’s a patchwork quilt, uncoordinated, and delivered to different standards in different settings and for different outcomes”.[footnote 96]
There is work underway at a national level, led by the National Police Chiefs’ Council, in order to develop a coordinated approach to data analytics in policing. This is reflected in the National Digital Policing Strategy[footnote 97], which sets out an intention to develop a National Data Ethics Governance model, and to provide clear lines of accountability on data and algorithm use at the top of all policing organisations. This should continue to be supported to ensure a more consistent approach across police forces. Moreover, HMICFRS should be included in national work in this area for example by establishing an External Reference Group for police use of data analytics, with a view to incorporating use of data analytics and its effectiveness into future crime data integrity inspections.
The RUSI report sets out in detail what a policy framework for data analytics in policing should involve and at CDEI we have been developing a draft Framework to support police project teams in addressing the legal and ethical considerations when developing data analytics tools. Without clear, consistent national guidance, coordination and oversight, we strongly believe that the potential benefits of these tools may not be fully realised, and the risks will materialise.
Recommendations to government:
Recommendation 3: The Home Office should define clear roles and responsibilities for national policing bodies with regards to data analytics and ensure they have access to appropriate expertise and are empowered to set guidance and standards. As a first step, the Home Office should ensure that work underway by the National Police Chiefs’ Council and other policing stakeholders to develop guidance and ensure ethical oversight of data analytics tools is appropriately supported.
Significant investment is needed in police project teams to address new challenges
Whilst it is crucial that a national policy framework is developed, without significant investment in skills and expertise in police forces, no framework will be implemented effectively. If police forces are expected to be accountable for these systems,engage with developers and make ethical decisions including trade-off considerations, significant investment is needed.
The announcement to recruit 20,000 new police officers provides an opportunity to bring in a diverse set of skills, however work is needed to ensure existing police officers are equipped to use data analytics tools. We also welcome the announcement in January 2020 of a Police Chief Scientific Advisor and dedicated funding for investment in Science, Technology and Research[footnote 98] as first steps in addressing this skills gap.
Based on the RUSI research and our engagement with police stakeholders, we know that a wide range of skills are required, ranging from, and not limited to legal, data science, and evaluation. In particular, our research with RUSI highlighted insufficient expert legal consultation at the development phase of data analytics projects, leading to a problematic delay in adhering to legal requirements. Developing a mechanism by which specialist expertise, such as legal, can be accessed by forces would help ensure this expertise is incorporated from the outset of developing a tool.
Moreover, there have been examples where the police force’s Data Protection Officer was not involved in discussions at the beginning of the project and has not been able to highlight where the project may interact with GDPR and support with the completion of a Data Protection Impact Assessment. Similarly, upskilling is needed of police ethics committees if they are to provide ethical oversight of data analytics projects.
Public deliberation on police use of data-driven technology is urgently needed
The decisions police make on a daily basis about which neighbourhoods or individuals to prioritise monitoring affect us all. The data and techniques used to inform these decisions are of great interest and significance to society at large. Moreover, due to wider public sector funding cuts, police are increasingly required to respond to non-crime problems[footnote 99]. For example, evidence suggests that police are spending less time dealing with theft and burglary and more time investigating sexual crime and responding to mental health incidents.[footnote 100]
The longstanding Peelian Principles, which define the British approach of policing by consent, are central to how a police force should behave and their legitimacy in the eyes of the public. And the values at the core of the Peelian Principles, integrity, transparency and accountability, continue to be as relevant today, in particular in light of the ethical considerations brought up by new technologies.
Research by the RSA and DeepMind[footnote 101] highlights that people feel more strongly against the use of automated decision systems in the criminal justice system (60 percent of people oppose or strongly oppose its use in this domain) than other sectors such as financial services. Moreover, people are least familiar with the use of automated decision-making systems in the criminal justice system; 83 percent were either not very familiar or not at all familiar with its use. These findings suggest a risk that if police forces move too quickly in developing these tools, without engaging meaningfully with the public, there could be significant public backlash and a loss of trust in the police’s use of data. A failure to engage effectively with the public is therefore not only an ethical risk, but a risk to the speed of innovation.
Police have many existing ways of engaging with the public through relationships with community groups and through Police and Crime Commissioners (PCC). West Midlands PCC have introduced a community representative role to their Ethics Committee to increase accountability for their use of data analytics tools. However, a civil society representative interviewed by RUSI highlighted that ethics committees could “act as a fig leaf over wider discussions” which the police should be having with the public.
We should take the steady increase in public trust in police to tell the truth since the 1980s[footnote 102] as a promising overarching trend. This signals an opportunity for police, policymakers, technologists, and regulators, to ensure data analytics tools in policing are designed and used in a way that builds legitimacy and is trustworthy in the eyes of the public.
6. Local government
Summary
Overview of findings:
-
Local authorities are increasingly using data to inform decision-making across a wide range of services. Whilst most tools are still in the early phase of deployment, there is an increasing demand for sophisticated predictive technologies to support more efficient and targeted services.
-
Data-driven tools present genuine opportunities for local government when used to support decisions. However, tools should not be considered a silver bullet for funding challenges and in some cases will require significant additional investment to fulfil their potential and possible increase in demand for services.
-
Data infrastructure and data quality are significant barriers to developing and deploying data-driven tools; investing in these is necessary before developing more advanced systems.
-
National guidance is needed as a priority to support local authorities in developing and using data-driven tools ethically, with specific guidance addressing how to identify and mitigate biases. There is also a need for wider sharing of best practice between local authorities.
Recommendation to government:
- Recommendation 4: Government should develop national guidance to support local authorities to legally and ethically procure or develop algorithmic decision-making tools in areas where significant decisions are made about individuals, and consider how compliance with this guidance should be monitored.
Future CDEI work:
- CDEI is exploring how best to support local authorities to responsibly and ethically develop data-driven technologies, including possible partnerships with both central and local government.
6.1 Background
Local authorities are responsible for making significant decisions about individuals on a daily basis. The individuals making these decisions are required to draw on complex sources of evidence, as well as their professional judgement. There is also increasing pressure to target resources and services effectively following a reduction of £16 billion in local authority funding over the last decade.[footnote 103] These competing pressures have created an environment where local authorities are looking to digital transformation as a way to improve efficiency and service quality.[footnote 104]
Whilst most research has found machine learning approaches and predictive technologies in local government to be in a nascent stage, there is growing interest in AI as a way to maximise service delivery and target early intervention as a way of saving resources further down the line when a citizen’s needs are more complex.[footnote 105] By bringing together multiple data sources, or representing existing data in new forms, data-driven technologies can guide decision-makers by providing a more contextualised picture of an individual’s needs. Beyond decisions about individuals, these tools can help predict and map future service demands to ensure there is sufficient and sustainable resourcing for delivering important services.
However, these technologies also come with significant risks. Evidence has shown that certain people are more likely to be overrepresented in data held by local authorities and this can then lead to biases in predictions and interventions.[footnote 106] A related problem is when the number of people within a subgroup is small, data used to make generalisations can result in disproportionately high error rates amongst minority groups. In many applications of predictive technologies, false positives may have limited impact on the individual. However in particularly sensitive areas, such as when deciding if and how to intervene in a case where a child may be at risk, false negatives and positives both carry significant consequences, and biases may mean certain people are more likely to experience these negative effects. Because the risks are more acute when using these technologies to support individual decision-making in areas such as adult and children’s services, it is for this reason that we have focused predominantly on these use cases.[footnote 107]
Where is data science in local government most being used?
-
Welfare and social care
-
Healthcare
-
Transportation
-
Housing and planning
-
Environment and sustainability
-
Waste management
-
Education
Policing and public safety
6.2 Findings
Our work on local government as a sector began with desk based research facilitated through our call for evidence and the landscape summary we commissioned. This evidence gathering provided a broad overview of the challenges and opportunities presented by predictive tools in local government.
We wanted to ensure our research was informed by those with first-hand accounts of the challenges of implementing and using these technologies, so we met and spoke with a broad range of people and organisations. This included researchers based in academic and policy organisations, third-party tool providers, local authorities, industry bodies and associations, and relevant government departments.
It is difficult to map how widespread algorithmic decision-making is in local government
There have been multiple attempts to map the usage of algorithmic decision-making tools across local authorities but many researchers have found this challenging.[footnote 109] An investigation by The Guardian found that, at a minimum, 140 councils out of 408 have invested in software contracts that cover identifying benefit fraud, identifying children at risk and allocating school places.[footnote 110] However this did not include additional use cases found in a report by the Data Justice Lab, a research group based in Cardiff University. The Data Justice Lab used Freedom of Information requests to learn which tools are being used and how frequently. However, there were many challenges with this approach with one fifth of requests being delayed or never receiving a response.[footnote 111] On the part of the local authorities, we have heard that there is often a significant challenge presented by the inconsistent terminology being used to describe algorithmic decision-making systems leading to varied reporting across local authorities using similar technologies. It is also sometimes difficult to coordinate activities across the whole authority because service delivery areas can operate relatively independently.
Given the rising interest in the use of predictive tools in local government, local authorities are keen to emphasise that their algorithms support rather than replace decision-makers, particularly in sensitive areas such as children’s social services. Our interviews found that local authorities were concerned that the current narrative focused heavily on automation rather than their focus which is more towards using data to make more evidence based decisions.
There is a risk that concerns around public reaction or media reporting on this topic will disincentivize transparency in the short-term. However, this is likely to cause further suspicion if data-driven technologies in local authorities appear opaque to the public. This may go on to harm trust if citizens do not have a way to understand how their data is being used to deliver public services. We believe that introducing requirements to promote transparency across the public sector will help standardise reporting, support researchers and build public trust (see Chapter 9 below for further discussion).
Comparison: Bias in local government and policing
There are many overlaps in the risks and challenges of data-driven technologies in both policing and local government. In both cases, public sector organisations are developing tools with data that may not be high quality and where certain populations are more likely to be represented, which could lead to unintentional discrimination. Both sectors often rely on procuring third-party software and may not have the necessary capacity and capability to question providers over risks around bias and discrimination.
There is scope for greater sharing and learning between these two sectors, and the wider public sector, around how to tackle these challenges, as well as considering adopting practices that have worked well in other places. For example, local authorities may want to look to certain police forces that have set up ethics committees as a way of providing external oversight of their data projects. Similarly, initiatives to develop integrated impact assessments, taking into account both data protection and equality legislation, may be applicable in both contexts.
Tool development approaches
Some local authorities have developed algorithmic decision-making tools in-house, others have tools procured from third-parties.
A. In-house approaches
Some local authorities have developed their own tools in-house, such as the Integrated Analytical Hub used by Bristol City Council. Bristol developed the hub in response to the Government’s Troubled Families programme, which provided financial incentives to local authorities who could successfully identify and support families at risk.[footnote 112] The hub brings together 35 datasets covering a wide range of topics including school attendance, crime statistics, children’s care data, domestic abuse records and health problem data such as adult involvement in alcohol and drug programmes. The datasets are then used to develop predictive modelling with targeted interventions then offered to families who are identified as most at risk.[footnote 113]
One of the benefits of using in-house approaches is that they offer local authorities greater control over the data being used. They also require a fuller understanding of the organisation’s data quality and infrastructure, which is useful when monitoring the system. However, building tools in-house often require significant investment in internal expertise, which may not be feasible for many local authorities. They also carry significant risks if an in-house project ultimately does not work.
B. Third-party software
There is an increasing number of third-party providers offering predictive analytics and data analysis software to support decision-making. Software to support detecting fraudulent benefit claims which is reportedly used by around 70 local councils.[footnote 114]
Other third-party providers offer predictive software that brings together different data sources and uses them to develop models to identify and target services. The use cases are varied and include identifying children at risk, adults requiring social care, or those at risk of homelessness. Software that helps with earlier interventions has the potential to bring costs down in the longer-term, however this relies on the tools being accurate and precise and so far there has been limited evaluation on the efficacy of these interventions.
Third-party providers offer specialist data science expertise that is likely not available to most local authorities and are likely to have valuable experience from previous work with other local authorities. However there are also risks around the costs of procuring the technologies. Transparency and accountability are also particularly important when procuring third-party tools, because commercial sensitivities may prevent providers wanting to share information to explain how a model is developed. Local authorities have a responsibility to understand how decisions are made regardless of whether they are using a third-party or developing an in-house approach, and third-parties should not be seen as a way to outsource these complex decisions. Local authorities should also consider how they will manage risks around bias, that may be outside the scope of the provider’s service (see Section 9.3 for further discussion of public sector procurement and transparency).
Local authorities struggle with data quality and data sharing when implementing data-driven tools
There are multiple challenges local authorities face when introducing new data-driven technologies. Due to legacy systems, local authorities often struggle with maintaining their data infrastructure and developing standardised processes for data sharing. Many of the conversations we had with companies who partnered with local authorities found that the set-up phase took a lot longer than expected due to these challenges which led to costly delays and a need to reprioritise resources. Local authorities should be wary of introducing data-driven tools as a quick-fix, particularly in cases where data infrastructure requires significant investment. For many local authorities, investing in more basic data requirements is likely to reap higher rewards than introducing more advanced technologies at this stage.
There is also an associated risk that legacy systems will have poor data quality. Poor data quality creates significant challenges because without a good quality, representative dataset, the algorithm will face the challenge of “rubbish in, rubbish out”, where poor quality training data results in a poor quality algorithm. One of the challenges identified by data scientists is that because data was being pulled from different sources, data scientists did not always have the necessary access to correct data errors.[footnote 115] As algorithms are only as good as their training data, interrogating the data quality of all data sources being used to develop a new predictive tool should be a top priority prior to procuring any new software.
CDEI’s work on data sharing
One of the challenges most frequently mentioned by local authorities wanting to explore the opportunities presented by data-driven technologies are concerns around data sharing. Often decision-support systems require bringing together different datasets, but physical barriers, such as poor infrastructure, and cultural barriers, such as insufficient knowledge of how and when to share data in line with data protection legislation, often mean that innovation is slow, even in cases where there are clear benefits.
For example, we often hear that in children’s social services, social workers do not always have access to the data they need to assess whether a child is at risk. Whilst the data may be held within the local authority and there is a clear legal basis for social workers to have access, local authorities experience various challenges in facilitating sharing. For data sharing to be effective, there also needs to be consideration of how to share data whilst retaining trust between individuals and organisations. Our recent report on data sharing explores these challenges and potential solutions in more detail.
National guidance is needed to govern the use of algorithms in the delivery of public services
There is currently little guidance for local authorities wanting to use algorithms to assist decision-making. We found that whilst many local authorities are confident in understanding the data protection risks, they are less clear on how legislation such as the Equality Act 2010 and Human Rights Act 1998 should be applied. There is a risk that without understanding and applying these frameworks, some tools may be in breach of the law.
The What Works Centre for Children’s Social Care recently commissioned a review of the ethics of machine learning approaches to children’s social care, conducted by the Alan Turing Institute and University of Oxford’s Rees Centre. They also found that national guidance should be a priority to ensure the ethical development and deployment of new data-driven approaches.[footnote 116] The review concludes that a “cautious, thoughtful and inclusive approach to using machine learning in children’s social care” is needed, but that this will only be facilitated through a series of recommendations, including nationally mandated standards. The research echoed what we have found in our work, that stakeholders felt strongly that national guidance was needed to protect vulnerable groups against the misuse of their data, including reducing the risk of unintentional biases.
Whilst most research has looked at the need for guidance in children’s social care, similar challenges are likely to arise across a range of services within local government that make important decisions about individuals, such as housing, adult social care, education, public health. We therefore think that guidance should be applicable across a range of areas, recognising there are likely to be places where supplementary detailed guidance is necessary, particularly where regulatory frameworks differ.
Taken together, there is a strong case for national guidelines setting out how to responsibly develop and introduce decision-supporting algorithms in local government. Government departments such as the Department for Education, the Ministry of Housing, Communities, and Local Government (MHCLG) and Department of Health and Social Care are best placed to support and coordinate the development of national guidance. The Local Government Association has also started a project bringing local authorities together to understand the challenges and opportunities with the aim of bringing this expertise together to develop guidance. National guidelines should look to build upon this work.
Recommendations to government
Recommendation 4: Government should develop national guidance to support local authorities to legally and ethically procure or develop algorithmic decision-making tools in areas where significant decisions are made about individuals, and consider how compliance with this guidance should be monitored.
Introducing data-driven technologies to save money may result in significant challenges
Local authorities have a variety of motivations for introducing algorithmic tools, with many focused on wanting to improve decision-making. However, given the significant reduction in income over the last decade, there is a drive towards using technology to improve efficiencies in service delivery within local government.
In their research exploring the uptake of AI across local government, the Oxford Internet Institute found that deploying tools with cost-saving as a primary motivation was unlikely to yield the results as expected. They state: “The case for many such projects is often built around the idea that they will save money. In the current climate of intense financial difficulty this is understandable. But we also believe this is fundamentally the wrong way to conceive data science in a government context: many useful projects will not, in the short term at least, save money.”[footnote 117] The focus of predictive tools is often grounded in the idea of early intervention. If there is a way to identify someone who is at risk and put assistive measures in place early, then the situation is managed prior to escalation, thus reducing overall resources. This longer-term way of thinking may result in less demand overall, however in the short-term it is likely to lead to increased workload and investment in preventative services.
There is a challenging ethical issue around the follow-up required once someone is identified. We heard examples of local authorities who held off adopting new tools because it would cost too much to follow up on the intelligence provided. Due to the duty of care placed on local authorities, there is also a concern that staff may be blamed for not following up leads if a case later develops. Therefore councils need to carefully plan how they will deploy resources in response to a potential increase in demands for services and should be wary of viewing these tools as a silver bullet for solving resourcing needs.
There should be greater support for sharing lessons, best practice and joint working between local authorities
Local authorities often experience similar challenges, but the networks to share lessons learned are often ad hoc and informal and rely on local authorities knowing which authorities have used similar tools. The Local Government Association’s work has started bringing this knowledge and experience together which is an important first step. There should also be opportunities for central government to learn from the work undertaken within local government, so as not to miss out on the innovation taking place and the lessons learned from challenges that are similar in both sectors.
The Local Digital Collaboration Unit within the Ministry of Housing, Communities and Local Government has also been set up to provide support and training to local authorities undertaking digital innovation projects. The Local Digital Collaboration Unit oversees the Local Digital Fund that provides financial support for digital innovation projects in local government. Greater support for this fund, particularly for projects looking at case studies for identifying and mitigating bias in local government algorithms, evaluating the effectiveness of algorithmic tools, public engagement and sharing best practice, would all add significant value. Our research found local authorities thought this fund was a very helpful initiative, however felt that greater investment would improve access to the benefits and be more cost effective over the long term.
Part III: Addressing the challenges
In Part I we surveyed the issue of bias in algorithmic decision-making, and in Part II we studied the current state in more detail across four sectors. Here, we move on to identify how some of the challenges we identified can be addressed, the progress made so far, and what needs to happen next.
There are three main areas to consider:
-
The enablers needed by organisations building and deploying algorithmic decision-making tools to help them do so in a fair way (see Chapter 7).
-
The regulatory levers, both formal and informal, needed to incentivise organisations to do this, and create a level playing field for ethical innovation (see Chapter 8).
-
How the public sector, as a major developer and user of data-driven technology, can show leadership through transparency (see Chapter 9).
There are inherent links between these areas. Creating the right incentives can only succeed if the right enablers are in place to help organisations act fairly, but conversely there is little incentive for organisations to invest in tools and approaches for fair decision-making if there is insufficient clarity on the expected norms.
Lots of good work is happening to try to make decision-making fair, but there remains a long way to go. We see the status quo as follows:
Impact on bias: Algorithms could help to address bias |
but | Building algorithms that replicate existing biased mechanisms will embed or even exacerbate existing inequalities. |
---|---|---|
Measurement of bias: More data available than ever before to help organisations understand the impacts of decision-making. |
but | Collection of protected characteristic data is very patchy, with significant perceived uncertainty about ethics, legality, and the willingness of individuals to provide data (see Section 7.3). Uncertainties concerning the legality and ethics of inferring protected characteristics. Most decision processes (whether using algorithms or not) exhibit bias in some form and will fail certain tests of fairness. The law offers limited guidance to organisations on adequate ways to address this. |
Mitigating bias: Lots of academic study and open source tooling available to support bias mitigation. |
but | Relatively limited understanding of how to use these tools in practice to support fair, end-to-end, decision-making. A US-centric ecosystem where many tools do not align with UK equality law. Uncertainty about usage of tools, and issues on legality of some approaches under UK law. Perceived trade-offs with accuracy (though often this may suggest an incomplete notion of accuracy) (see Section 7.4). |
Expert support: A range of consultancy services are available to help with these issues. |
but | An immature ecosystem, with no clear industry norms around these services, the relevant professional skills, or important legal clarity (see Section 8.5). |
Workforce diversity: Strong stated commitment from government and industry to improving diversity. |
but | Still far too little diversity in the tech sector (see Section 7.2). |
Leadership and governance: Many organisations understand the strategic drivers to act fairly and proactively in complying with data protection obligations and anticipating ethical risks. |
but | Recent focus on data protection (due to the arrival of GDPR), and especially privacy and security aspects of this, risks de-prioritisation of fairness and equality issues (even though these are also required in GDPR). Identifying historical or current bias in decision-making is not a comfortable thing for organisations to do. There is a risk that public opinion will penalise those who proactively identify and address bias. Governance needs to be more than compliance with current regulations; it needs to consider the possible wider implications of the introduction of algorithms, and anticipate future ethical problems that may emerge (see Section 7.5). |
Transparency: Transparency about the use and impact of algorithmic decision-making would help to drive greater consistency. |
but | There are insufficient incentives for organisations to be more transparent and risks to going alone. There is a danger of creating requirements that create public perception risks for organisations even if they would help reduce risks of biased decision-making. The UK public sector has identified this issue, but could do more to lead through its own development and use of algorithmic decision-making (see Chapter 9). |
Regulation: Good regulation can support ethical innovation. |
but | Not all regulators are currently equipped to deal with the challenges posed by algorithms. There is continued nervousness in industry around the implications of GDPR. The ICO has worked hard to address this, and recent guidance will help, but there remains a way to go to build confidence on how to interpret GDPR in this context (see Chapter 8). |
Governance is a key theme throughout this part of the review; how should organisations and regulators ensure that risks of bias are being anticipated and managed effectively? This is not trivial to get right, but there is clear scope for organisations to do better in considering potential impacts of algorithmic decision-making tools, and anticipating risks in advance.
The terms anticipatory governance and anticipatory regulation are sometimes used to describe this approach; though arguably anticipatory governance or regulation is simply part of any good governance or regulation. In Chapter 7 we consider how organisations should approach this, in Chapter 8 the role of regulators and the law in doing so, and in Chapter 9 how a habit of increased transparency in the public sector’s use of such tools could encourage this.
7. Enabling fair innovation
Summary
Overview of findings:
-
Many organisations are unsure how to address bias in practice. Support is needed to help them consider, measure, and mitigate unfairness.
-
Improving diversity across a range of roles involved in technology development is an important part of protecting against certain forms of bias. Government and industry efforts to improve this must continue, and need to show results.
-
Data is needed to monitor outcomes and identify bias, but data on protected characteristics is not available often enough. One cause is an incorrect belief that data protection law prevents collection or usage; but there are a number of lawful bases in data protection legislation for using protected or special characteristic data for monitoring or addressing discrimination. There are some other genuine challenges in collecting this data, and more innovative thinking is needed in this area; for example, the potential for trusted third party intermediaries.
-
The machine learning community has developed multiple techniques to measure and mitigate algorithmic bias. Organisations should be encouraged to deploy methods that address bias and discrimination. However, there is little guidance on how to choose the right methods, or how to embed them into development and operational processes. Bias mitigation cannot be treated as a purely technical issue; it requires careful consideration of the wider policy, operational and legal context. There is insufficient legal clarity concerning novel techniques in this area; some may not be compatible with equality law.
Recommendations to government:
-
Recommendation 5: Government should continue to support and invest in programmes that facilitate greater diversity within the technology sector, building on its current programmes and developing new initiatives where there are gaps.
-
Recommendation 6: Government should work with relevant regulators to provide clear guidance on the collection and use of protected characteristic data in outcome monitoring and decision-making processes. They should then encourage the use of that guidance and data to address current and historic bias in key sectors.
-
Recommendation 7: Government and the ONS should open the Secure Research Service more broadly, to a wider variety of organisations, for use in evaluation of bias and inequality across a greater range of activities.
-
Recommendation 8: Government should support the creation and development of data-focused public and private partnerships, especially those focused on the identification and reduction of biases and issues specific to under-represented groups. The ONS and Government Statistical Service should work with these partnerships and regulators to promote harmonised principles of data collection and use into the private sector, via shared data and standards development.
Recommendations to regulators:
- Recommendation 9: Sector regulators and industry bodies should help create oversight and technical guidance for responsible bias detection and mitigation in their individual sectors, adding context-specific detail to the existing cross-cutting guidance on data protection, and any new cross-cutting guidance on the Equality Act.
Advice to industry:
-
Organisations building and deploying algorithmic decision-making tools should make increased diversity in their workforce a priority. This applies not just to data science roles, but also to wider operational, management and oversight roles. Proactive gathering and use of data in the industry is required to identify and challenge barriers for increased diversity in recruitment and progression, including into senior leadership roles.
-
Where organisations operating within the UK deploy bias detection or mitigation tools developed in the US, they must be mindful that relevant equality law (along with that across much of Europe) is different.
-
Where organisations face historical issues, attract significant societal concern, or otherwise believe bias is a risk, they will need to measure outcomes by relevant protected characteristics to detect biases in their decision-making, algorithmic or otherwise. They must then address any uncovered direct discrimination, indirect discrimination, or outcome differences by protected characteristics that lack objective justification.
-
In doing so, organisations should ensure that their mitigation efforts do not produce new forms of bias or discrimination. Many bias mitigation techniques, especially those focused on representation and inclusion, can legitimately and lawfully address algorithmic bias when used responsibly. However, some risk introducing positive discrimination, which is illegal under the Equality Act. Organisations should consider the legal implications of their mitigation tools, drawing on industry guidance and legal advice.
Guidance to organisation leaders and boards:
Those responsible for governance of organisations deploying or using algorithmic decision-making tools to support significant decisions about individuals should ensure that leaders are in place with accountability for:
-
Understanding the capabilities and limits of those tools.
-
Considering carefully whether individuals will be fairly treated by the decision-making process that the tool forms part of.
-
Making a conscious decision on appropriate levels of human involvement in the decision-making process.
-
Putting structures in place to gather data and monitor outcomes for fairness.
-
Understanding their legal obligations and having carried out appropriate impact assessments.
This especially applies in the public sector when citizens often do not have a choice about whether to use a service, and decisions made about individuals can often be life-affecting.
7.1 Introduction
There is clear evidence, both from wider public commentary and our research, that many organisations are aware of potential bias issues and are keen to take steps to address them.
However, the picture is variable across different sectors and organisations, and many do not feel that they have the right enablers in place to take action. Some organisations are uncertain of how they should approach issues of fairness, including associated reputational, legal and commercial issues. To improve fairness in decision-making, it needs to be as easy as possible for organisations to identify and address bias. A number of factors are required to help build algorithmic decision-making tools and machine learning models with fairness in mind:
-
Sufficient diversity in the workforce to understand potential issues of bias and the problems they cause.
-
Availability of the right data to understand bias in data and models.
-
Access to the right tools and approaches to help identify and mitigate bias.
-
An ecosystem of expert individuals and organisations able to support them.
-
Governance structures that anticipate risks, and build in opportunities to consider the wider impact of an algorithmic tool with those affected.
-
Confidence that efforts to behave ethically (by challenging bias) and lawfully (by eliminating discrimination) will attract the support of organisational leadership and the relevant regulatory bodies.
Some of these strategies can only be achieved by individual organisations, but the wider ecosystem needs to enable them to act in a way that is both effective and commercially viable.
It is always better to acknowledge biases, understand underlying causes, and address them as far as possible, but the ‘correct’ approach for ensuring fairness in an algorithmic decision-making tool will depend strongly on use case and context. The real-world notion of what is considered ‘fair’ is as much a legal, ethical or philosophical idea as a mathematical one, which can never be as holistic, or as applicable across cases. What good practice should a team then follow when seeking to ensure fairness in an algorithmic decision-making tool? We investigate the issue further in this chapter.
7.2 Workforce diversity
There is increasing recognition that it is not algorithms that cause bias alone, but rather that technology may encode and amplify human biases. One of the strongest themes in responses to our Call for Evidence, and our wider research and engagement, was the need to have a diverse technology workforce; better able to interrogate biases that may arise throughout the process of developing, deploying and operating an algorithmic decision-making tool. By having more diverse teams, biases are more likely to be identified and less likely to be replicated in these systems.
There is a lot to do to make the technology sector more diverse. A report from Tech Nation found that only 19% of tech workers are women.[footnote 117] What is perhaps more worrying is how little this has changed over the last 10 years, compared with sectors such as engineering, which have seen a significant increase in the proportion of women becoming engineers. This gender gap is similarly represented at senior levels of tech companies.
Although the representation of people with BAME backgrounds is proportionate to the UK population (15%), when this is broken down by ethnicity we see that Black people are underrepresented by some margin. It should be a priority to improve this representation. Organisations should also undertake research to understand how ethnicity intersects with other characteristics, as well as whether this representation is mirrored at more senior levels.[footnote 118]
There is less data on other forms of diversity, which has spurred calls for greater focus on disability inclusion within the tech sector.[footnote 119] Similarly, more work needs to be done in terms of age, socio-economic background, and geographic spread across the UK. It is important to note that the technology sector is doing well in some areas. For example, the tech workforce is much more international than many others.[footnote 120] The workforce relevant to algorithmic decision-making is, of course, not limited to technology professionals; a diverse range of skills is necessary within teams and organisations to properly experience the benefits of diversity and equality. Beyond training and recruitment, technology companies need to support workers by building inclusive workplaces, which are key to retaining, as well as attracting, talented staff from different backgrounds.
There are a lot of activities aimed at improving the current landscape. The Government is providing financial support to a variety of initiatives including the Tech Talent Charter[footnote 121], founded by a group of organisations wanting to work together to create meaningful change for diversity in tech. Currently, the charter has around 500 signatories ranging from small start-ups to big businesses and is intending to grow to 600 by the end of 2020. In 2018 the Government also launched a £1 million “Digital Skills Innovation Fund”, specifically for helping underrepresented groups develop skills to move into digital jobs. The Government’s Office for AI and AI Council is conducting a wide range of work in this area, including helping to drive diversity in the tech workforce, as well as recently securing £10 million in funding for students from underrepresented backgrounds to study AI related courses.[footnote 122]
Recommendations to government
Recommendation 5: Government should continue to support and invest in programmes that facilitate greater diversity within the technology sector, building on its current programmes and developing new initiatives where there are gaps.
There are also a huge number of industry initiatives and nonprofits aimed at encouraging and supporting underrepresented groups in the technology sector.[footnote 123] They are wide-ranging in both their approaches and the people they are supporting. These efforts are already helping to raise the profile of tech’s diversity problem, as well as supporting people who want to either move into the tech sector or develop further within it. The more government and industry can do to support this work the better.
Advice to industry
Organisations building and deploying algorithmic decision-making tools should make increased diversity in their workforce a priority. This applies not just to data science roles, but also to wider operational, management and oversight roles. Proactive gathering and use of data in the industry is required to identify and challenge barriers for increased diversity in recruitment and progression, including into senior leadership roles.
Given the increasing momentum around a wide-range of initiatives springing up both within government and from grassroots campaigns, we hope to soon see a measurable improvement in data on diversity in tech.
7.3 Protected characteristic data and monitoring outcomes
The issue
A key part of understanding whether a decision-making process is achieving fair outcomes is measurement. Organisations may need to compare outcomes across different demographic groups to assess whether they match expectations. To do this, organisations must have some data on the demographic characteristics of groups they are making decisions about. In recruitment, especially in the public sector, the collection of some ‘protected characteristic’ data (defined under the Equality Act, 2010) for monitoring purposes has become common-place, but this is less common in other sectors.
Removing or not collecting protected characteristic data does not by itself ensure fair data-driven outcomes. Although this removes the possibility of direct discrimination, it may make it impossible to evaluate whether indirect discrimination is taking place. This highlights an important tension: to avoid direct discrimination as part of the decision-making process, protected characteristic attributes should not be considered by an algorithm. But, in order to assess the overall outcome (and hence assess the risk of indirect discrimination), data on protected characteristics is required.[footnote 124]
There have been calls for wider data collection, reflecting an acceptance that doing so helps promote fairness and equality in areas where bias could occur.[footnote 125] CDEI supports these calls; we think that greater collection of protected characteristic data would allow for fairer algorithmic decision-making in many circumstances. In this section we explore why that is, and the issues that need to be overcome to make this happen more often.
The need to monitor outcomes is important even when no algorithm is involved in a decision, but the introduction of algorithms makes this more pressing. Machine learning detects patterns and can find relationships in data that humans may not see or be able to fully understand. Although machine learning models optimise against objectives they have been given by a human, if data being analysed reflects historical or subconscious bias, then imposed blindness will not prevent models from finding other, perhaps more obscure, relationships. These could then lead to similarly biased outcomes, encoding them into future decisions in a repeatable way. This is therefore the right time to investigate organisational biases and take the actions required to address them.
There are a number of reasons why organisations are not currently collecting protected characteristic data, including concerns or perceptions that:
-
Collecting protected characteristic data is not permitted by data protection law. This is incorrect in the UK, but seems to be a common perception (see below for further discussion).
-
It may be difficult to justify collection in data protection law, and then store and use that data in an appropriate way (i.e. separate to the main decision-making process).
-
Service users and customers will not want to share the data, and may be concerned about why they are being asked for it. Our own survey work suggests that this is not necessarily true for recruitment[footnote 126], although it may be elsewhere.
- Data could provide an evidence base that organisational outcomes were biased; whether in a new algorithmic decision-making process, or historically.
In this section, we consider what is needed to overcome these barriers, so that organisations in the public and private sector can collect and use data more often, in a responsible way. Not all organisations will need to collect or use protected characteristic data. Services may not require it, or an assessment may find that its inclusion does more harm than good. However, many more should engage in collection than do so at present.
Data protection concerns
Our research suggests a degree of confusion about how data protection law affects the collection, retention and use of protected characteristic data.
Data protection law sets additional conditions for processing special category data. This includes many of the protected characteristics in the Equality Act 2010 (discussed in this chapter), as well as other forms of sensitive data that are not currently protected characteristics, such as biometric data.
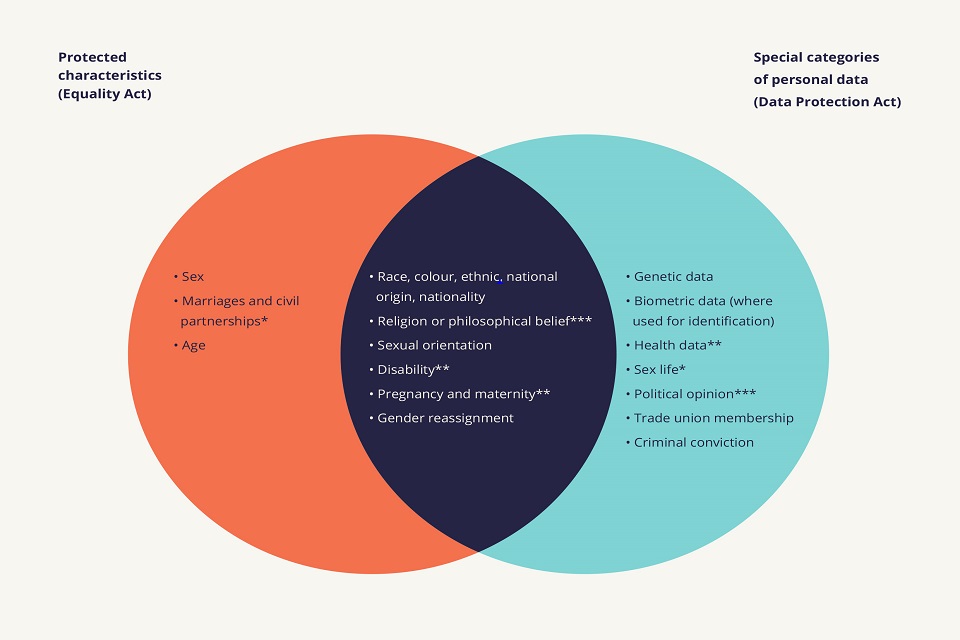
There are overlaps between the protected characteristics covered by the Equality Act, and the special categories of personal data under data protection law. Protected characteristics solely covered under the Equality Act are “sex”, “marriage & civil partnership” and “age”. Special categories of personal data under Data Protection law include “genetic data”, “biometric data (where used for identification”, “health data”, “sex life”, “political opinion”, “trade union membership” and “criminal convictions”. The overlapping characteristics are: “race, colour, ethnic origin & nationality”, “religion or philosophical belief”, “sexual orientation”, “disability”, “pregnancy & maternity” and “gender reassignment.”
Figure 4: Overlap between the Protected Characteristics of equality law and Special Categories of personal data under data protection law
Several organisations we spoke to believed that data protection requirements prevent the collection, processing or use of special category data to test for algorithmic bias and discrimination. This is not the case: data protection law sets out specific conditions and safeguards for the processing of special category data, but explicitly includes use for monitoring equality.
The collection, processing and use of special category data is allowed if it is for “substantial public interest”, among other specific purposes set out in data protection law. In Schedule 1, the Data Protection Act sets out specific public interest conditions that meet this requirement, including ‘equality of opportunity or treatment’ where the Act allows processing of special category data where it “is necessary for the purposes of identifying or keeping under review the existence or absence of equality of opportunity or treatment between groups of people specified in relation to that category with a view to enabling such equality to be promoted or maintained,” (Schedule 1, 8.1(b)). Notably, this provision also specifically mentions equality rather than discrimination, which allows for this data to address broader fairness and equality considerations rather than just discrimination as defined by equality or human rights law.
However, this provision of the Data Protection Act clarifies that it does not allow for using special category data for individual decisions (Schedule 1, 8.3), or if it causes substantial damage or distress to an individual (Schedule 1, 8.4). In addition to collection, data retention also needs some thought. Organisations may want to monitor outcomes for historically disadvantaged groups over time, which would require longer data retention periods than needed for individual use cases. This may lead to a tension between monitoring equality and data protection in practice, but these restrictions are much less onerous than sometimes described by organisations. The recently published ICO guidance on AI and data protection[footnote 127] sets out some approaches to assessing these issues, including guidance on special category data.
Section 8.3 of this report sets out further details of how equality and data protection law apply to algorithmic decision-making.
The need for guidance
As with diversity monitoring in recruitment, pockets of the public sector are increasingly viewing the collection of data on protected characteristics as essential to the monitoring of unintentional discrimination in their services. The Open Data Institute recently explored how public sector organisations should consider collecting protected characteristic data to help fulfil their responsibilities under the Public Sector Equality Duty[footnote 128]. They recognise that:
there is no accepted practice for collecting and publishing data about who uses digital services, which makes it hard to tell whether they discriminate or not.[footnote 129]
The EHRC provides guidance[footnote 130] on how to deal with data protection issues when collecting data in support of obligations in the Public Sector Equality Duty, but is yet to update it for the significant changes to data protection law through GDPR and the Data Protection Act 2018, or to consider the implications of algorithmic decision-making on data collection. This needs to be addressed. There should also be consistent guidance for public sector organisations wanting to collect protected characteristic data specifically for equality monitoring purposes, which should become standard practice. Such practice is essential for testing algorithmic discrimination against protected groups. Organisations need to be assured that by following guidance, they are not just making their systems more fair and reducing their legal risk, but also minimising any unintended consequences of personal data collection and use, and thus helping to maintain public trust.
The picture is more complicated in the private sector because organisations do not have the same legal responsibility under the Public Sector Equality Duty[footnote 131]. Equalities law requires that all organisations avoid discrimination, but there is little guidance on how they should practically identify it in algorithmic contexts. Without guidance or the PSED, private sector organisations have to manage different expectations from customers, employees, investors and the public about how to measure and manage the risks of algorithmic bias.
There are also concerns about balancing the trade-off between fairness and privacy. In our interviews with financial institutions, many focused on principles such as data minimisation within data protection legislation. In some cases it was felt that collecting this data at all may be inappropriate, even if the data does not touch upon decision-making tools and models. In insurance, for example, there are concerns around public trust in whether providing this information could affect an individual’s insurance premium. Organisations should think carefully about how to introduce processes that secure trust, such as being as transparent as possible about the data being collected, why and how it is used and stored, and how people can access and control their data. Building public trust is difficult, especially when looking to assess historic practices which may hide potential liabilities. Organisations may fear that by collecting data, they identify and expose patterns of historic bad practice. However, data provides a key means of addressing issues of bias and discrimination, and therefore reducing risk in the long term.
Although public services normally sit within a single national jurisdiction, private organisations may be international. Different jurisdictions have different requirements for the collection of protected characteristic data, which may even be prohibited. The French Constitutional Council, for example, prohibits data collection or processing regarding race or religion. International organisations may need help to satisfy UK specific or nationally devolved regulation.
There will be exceptions to the general principle that collection of protected or special characteristic data is a good thing. In cases where action is not needed, or urgently required, due to context or entirely obvious pre-existing biases, collecting protected characteristic data will be unnecessary. In others it may be seen as disproportionately difficult to gather the relevant data to identify bias. In others still, it may be impossible to provide privacy for very small groups, where only a very small number of service users or customers have a particular characteristic. Overcoming and navigating such barriers and concerns will require a combination of effective guidance, strong promotion of new norms from a centralised authority, or even regulatory compulsion.
Recommendations to government
Recommendation 6: Government should work with relevant regulators to provide clear guidance on the collection and use of protected characteristic data in outcome monitoring and decision-making processes. They should then encourage the use of that guidance and data to address current and historic bias in key sectors.
Alternative approaches
Guidance is a first step, but more innovative thinking may be needed on new models for collecting, protecting or inferring protected characteristic data.
Such models include a safe public third-party, collecting protected characteristic data on behalf of organisations, and securely testing their algorithms and decision-making processes without ever providing data to companies themselves.[footnote 132] This could be a responsibility of the relevant sector regulator or a government organisation such as the Office for National Statistics. There are also models where a private sector company could collect and store data securely, offering individuals guarantees on privacy and purpose, but then carrying out testing on behalf of other companies as a third party service.
Where organisations do not collect protected characteristic data explicitly, they can sometimes infer it from other data; for example by extracting the likely ethnicity of an individual from their name and postcode. If used within an actual decision-making process, such proxies present some of the key bias risks, and using this information in relation to any individual presents substantial issues for transparency, accuracy, appropriateness and agency. In cases where collecting protected characteristic data is unfeasible, identifying proxies for protected characteristics purely for monitoring purposes may be a better option than keeping processes blind. However, there are clear risks around the potential for this type of monitoring to undermine trust, so organisations need to think carefully about how to proceed ethically, legally and responsibly. Inferred personal data (under data protection law) is still, legally, personal data, and thus subject to the relevant laws and issues described above.[footnote 133] A right to reasonable inference is under current academic discussion.[footnote 134]
Further development is needed around all of these concepts, and few models exist of how they would work in practice. As above, legal clarity is a necessary first step, followed by economic viability, technical capability, security, and public trust. However, there are some models of success to work from, such as the ONS Secure Research Service, described below, and the NHS Data Safe Havens[footnote 135], as well as ongoing research projects in the field[footnote 136]. If a successful model could be developed, private sector companies would be able to audit their algorithms for bias without individuals being required to hand over their sensitive data to multiple organisations. We believe further research is needed to develop a longer-term proposal for the role of third-parties in such auditing, and will consider future CDEI work in this area.
Access to baseline data
Where organisations determine that collecting protected characteristics is appropriate for assessing bias, they will often need to collect information about their service users or customers, and compare it with relevant wider (often national) demographic data. It is hard to tell if a decision is having a negative effect on a group without some sense of what should be considered normal. A lack of relevant and representative wider data can make it difficult for both public and private organisations to tell if their processes are biased, and then to develop responsible algorithmic tools in response.
Relevant data is already made available publicly, including UK Census and survey data published by the ONS. The devolved administrations have also made significant volumes of data widely accessible (through StatsWales, Statistics.gov.scot, and NISRA), as have a number of Government departments and programmes. Sector specific datasets and portals add to this landscape in policing, finance, and others.
More detailed population data can be accessed through the ONS’ Secure Research Service which provides a wide variety of national scale information, including pre-existing survey and administrative data resources.
Usage of this service is managed through its “5 Safes” (safe projects, people, settings, data and outputs) framework, and restricted to the purposes of research, evaluation and analysis. This often restricts access to academic research groups, but there may be opportunities to widen the service to support evaluation of diversity outcomes by regulators and delivery organisations.
Regulators can help by promoting key public datasets of specific value to their sector, along with guidance material accessible for their industry. Wider availability of aggregate demographic information for business use would also allow for better data gathering, or better synthetic data generation. Publicly available, synthetically augmented, and plausible versions of more surveys (beyond the Labour Force Survey[footnote 137]) would help more users find and develop use cases.
Government announcements in 2020 included £6.8 million (over three years) to help the ONS share more, higher-quality data across government, and to link and combine datasets in new ways (for example, to inform policy or evaluate interventions).
Recommendations to government
Recommendation 7: Government and the ONS should open the Secure Research Service more broadly, to a wider variety of organisations, for use in evaluation of bias and inequality across a greater range of activities.
In the short term, organisations who find publicly held data insufficient will need to engage in partnership with their peers, or bodies that hold additional representative or demographic information, to create new resources. In the private sphere these approaches include industry specific data sharing initiatives (Open Banking in finance, Presumed Open in energy, and more under discussion by the Better Regulation Executive), and trusted sector-specific data intermediaries.
Recommendations to government
Recommendation 8: Government should support the creation and development of data-focused public and private partnerships, especially those focused on the identification and reduction of biases and issues specific to under-represented groups. The Office for National Statistics and Government Statistical Service should work with these partnerships and regulators to promote harmonised principles of data collection and use into the private sector, via shared data and standards development.
Case Study: Open Banking
In 2016 the Competition and Markets Authority (CMA) intervened in the UK ecosystem to require that nine of the largest UK banks grant direct, transaction level, data access to licensed startups. Although compliance and enforcement sits with the CMA, Open Banking represents a regulatory partnership as much as a data partnership, with the FCA providing financial oversight, and the ICO providing data protection. Open Banking has led to over 200 regulated companies providing new services, including financial management and credit scoring. As a result access to credit, debt advice and financial advice is likely to widen, which in turn is expected to allow for better service provision for under-represented groups. This provides an opportunity to address unfairness and systemic biases, but new forms of (digital) exclusion and bias may yet appear.
Examples of wider partnerships include projects within the Administrative Data Research UK programme (bringing together government, academia and public bodies) and the increasing number of developmental sandboxes aimed at industry or government support (see Section 8.5). Where new data ecosystems are created around service-user data, organisations like the new Global Open Finance Centre of Excellence can then provide coordination and research support. Empowering organisations to share their own data with trusted bodies will enable industry wide implementation of simple but specific common data regimes. Relatively quick wins are achievable in sectors that have open data standards in active development such as Open Banking and Open Energy.
Case Study: Monitoring for bias in digital transformation of the courts
Accessing protected characteristic data to monitor outcomes is not only necessary when introducing algorithmic decision-making, but also when making other major changes to significant decision-making processes.
Her Majesty’s Courts and Tribunal Service (HMCTS) is currently undergoing a large-scale digital transformation process aimed largely at making the court system more affordable and fair, including online dispute resolution and opt-in automated fixed penalties for minor offences where there is a guilty plea. As part of this transformation, they have recognised a need for more information about people entering and exiting the judicial process. More protected characteristic data would allow HMCTS to assess the effectiveness of different interventions and the level of dependency on, and uptake of, different parts of the judicial system within different groups. Senior justices would largely prefer to see a general reduction in the number of people going through criminal courts and greater diversity in use of civil courts. It is hard to objectively measure these outcomes, or whether courts are acting fairly and without bias, without data.
In order to achieve these goals, HMCTS have focused on access to protected characteristic data, predominantly through data linkage and inference from wider administrative data. They have worked with the Government Statistical Service’s Harmonisation Team and academic researchers to rebuild their data architecture to support this. The resulting information is intended to both be valuable to the Ministry of Justice for designing fair interventions in the functioning of the courts, but also eventually to be made available for independent academic research (via Administrative Data Research UK and the Office for National Statistics).
This is just one example of a drive toward new forms of data collection, designed to test and assure fair processes and services within public bodies. It is also illustrative of a project navigating the Data Protection Act 2018 and the ‘substantial public interest’ provision of the GDPR to assess risks around legal exposure. It is essential for public bodies to establish whether or not their digital services involve personal data, are classed as statistical research, or sit within other legislative ‘carve-outs’. This is especially true when dealing with data that is not necessarily accompanied by end-user consent.
7.4 Detecting and mitigating bias
In the previous section we argue that it is preferable to seek to identify bias and to address it, rather than hope to avoid it by unawareness. There is a high level of focus on this area in the academic literature, and an increasing number of practical algorithmic fairness tools have appeared in the last three years.
Approaches for detecting bias include:
-
Comparing training with population datasets to see if they are representative.
-
Analysing the drivers of differences in outcomes that are likely to cause bias. For example, if it could be shown that certain recruiters in an organisation held measurable biases compared to other recruiters (after controlling for other characteristics), it would be possible to train an algorithm with a less biased subset of the data (e.g. by excluding the biased group).
-
Analysing how and where relevant model variables correlate with different groups. For example, if qualifications are a factor in a model for recommending recruitment, analysis can show the extent to which this results in more job offers being made to particular groups.
Different approaches are necessary in different contexts. For most organisations, bias monitoring and analysis are a necessary part of their decision-making (whether algorithmic or not). Where that monitoring suggests a biased process, the question is then how to address it. Ensuring that the data being collected (Section 7.3) is both necessary and sufficient is an important first step. Further methods (detailed below) will need to be proportionate to organisational needs.
Case study: Bias detection in 1988
The 1988 medical school case mentioned in Section 2.1 is an interesting example of bias detection. Their program was developed to match human admissions decisions, doing so with 90-95% accuracy. Despite bias against them, the school still had a higher proportion of non-European students admitted than most other London medical schools. The human admissions officers’ biases would probably never have been demonstrated, but for the use of their program. Had that medical school been equipped with a current understanding of how to assess an algorithm for bias, and been motivated to do so, perhaps they would have been able to use their algorithm to reduce bias rather than propagate it.
Organisations that need to directly mitigate bias in their models now have a number of interventions at their disposal. This is a generally positive development but the ecosystem is complex. Organisations see a need for clarity on which mitigation tools and techniques are appropriate and legal in which circumstances. Crucially, what is missing is practical guidance about how to create, deploy, monitor, audit, and adjust fairer algorithms, using the most effective tools and techniques available. It is important to recognise that the growing literature and toolsets on algorithmic fairness often only address part of the issue (that which can be quantified), and wider interventions to promote fairness and equality remain key to success.
As part of this review, we contracted[footnote 143] Faculty to analyse, assess and compare the various technical approaches to bias mitigation. This section is informed by their technical work. The outputs from that work are being made available elsewhere.
Statistical definitions of fairness
If we want model development to include a definition of fairness, we must tell the relevant model what that definition is, and then measure it. There is, however, no single mathematical definition of fairness that can apply to all contexts[footnote 144]. As a result, the academic literature has seen dozens of competing notions of fairness introduced, each with their own merits and drawbacks, and many different terminologies for categorising these notions, none of which are complete. Ultimately, humans must choose which notions of fairness an algorithm will work to, taking wider notions and considerations into account, and recognising that there will always be aspects of fairness outside of any statistical definition.
Fairness definitions can be grouped by notion of fairness sought and stage of development involved. In the first instance, these fall into the broad categories of procedural and outcome fairness discussed in Section 2.5. Within the technical aspects of machine learning, procedural fairness approaches often concern the information used by a system, and thus include “Fairness Through Unawareness”, which is rarely an effective strategy. The statistical concept of fairness as applied to algorithms is then focused on achieving unbiased outcomes, rather than other concepts of fairness. Explicit measurement of equality across results for different groups is necessary for most of these approaches.
Within Outcome Fairness we can make additional distinctions, between Causal and Observational notions of fairness, as well as Individual and Group notions.
-
Individual notions compare outcomes for individuals to see if they are treated differently. However, circumstances are generally highly specific to individuals, making them difficult to compare without common features.
-
Group notions aggregate individual outcomes by a common feature into a group, then compare aggregated outcomes to each other.
Group and individual notions are not mutually exclusive: an idealised ‘fair’ algorithm could achieve both simultaneously.
-
Observational approaches then deal entirely with the measurable facts of a system, whether outcomes, decisions, data, mathematical definitions, or types of model.
-
Causal approaches can consider ‘what if?’ effects of different choices or interventions. This typically requires a deeper understanding of the real-world system that the algorithm interacts with.
In their technical review of this area, Faculty describe a way to categorise different bias mitigation strategies within these notions of fairness (see table below). They also identified that the four Group Observational notions (highlighted) are currently the most practical approaches to implement for developers: being relatively easy to compute and providing meaningful measures for simple differences between groups (this does not necessarily mean they are an appropriate choice of fairness definition in all contexts). The majority of existing bias mitigation tools available to developers address (Conditional) Demographic Parity, or Equalised Odds, or are focused on removing sensitive attributes from data. The table below shows how the most commonly used approaches (and other examples) sit within wider definitions as described above. Visual demonstrations of these notions are shown in a web app that accompanies this review.[footnote 145]
Observational | Causal | |
Group |
Demographic Parity (‘Independence’) Conditional Demographic Parity Equalised Odds (‘Separation’) Calibration (‘Sufficiency’) Sub-Group Fairness |
Unresolved Discrimination Proxy Discrimination |
---|---|---|
Individual | Individual Fairness | Meritocratic Fairness Counterfactual Fairness |
* Demographic Parity - outcomes for different protected groups are equally distributed, and statistically independent. Members of one group are as likely to achieve a given outcome as those in a different group, and successes in one group do not imply successes (or failures) in another. At a decision level, Demographic Parity might mean that the same proportion of men and women applying for loans or insurance are successful, but this kind of fairness can also be applied when assigning risk scores, regardless of where a success threshold is applied. * Conditional Demographic Parity - as above, but “legitimate risk factors” might mean that we consider it fair to discriminate for certain groups, such as by age in car insurance. The difficulty then sits in deciding which factors qualify as legitimate, and which may be perpetuating historical biases. * Equalised Odds (separation) - qualified and unqualified candidates are treated the same, regardless of their protected attributes. True positive rates are the same for all protected groups, as are false positive rates: the chance that a qualified individual is overlooked, or that an unqualified individual is approved, is the same across all protected groups. However, if different groups have different rates of education, or claim/repayment risk, or some other qualifier, Equalised Odds can result in different groups being held to different standards. This means that Equalised Odds is capable of entrenching systematic bias, rather than addressing it. * Calibration - outcomes for each protected group are predicted with equal reliability. If outcomes are found to be consistently under or overpredicted for a group (possibly due to a lack of representative data), then an adjustment/calibration needs to be made. Calibration is also capable of perpetuating pre-existing biases. |
An example of how these different definitions play out in practice can be seen in the US criminal justice system, as per the box below.
Case study: COMPAS
For a given risk score in the US COMPAS criminal recidivism model, the proportion of defendants who reoffend is roughly the same independent of a protected attribute, including ethnicity (Calibration). Otherwise, a risk score of 8 for a white person would mean something different for a Black person. Propublica’s criticism of this model highlighted that Black defendants who didn’t reoffend were roughly twice as likely to be given scores indicating a medium/high risk of recidivism as white defendants. However, ensuring equal risk scores among defendants who didn’t offend or re-offend (Equalised Odds) would result in losing Calibration at least to some degree. Fully satisfying both measures proves impossible.
If attributes of individuals (protected or otherwise) are apparently linked, such as recidivism and race, then generally equality of opportunity (the generalised form of Equalised Odds) and Calibration cannot be reconciled. If a model satisfies Calibration, then in each risk category, the proportion of defendants who reoffend is the same, regardless of race. The only way of achieving this if the recidivism rate is higher for one group, is if more individuals from that group are predicted to be high-risk. Consequently, this means that the model will make more false positives for that group than others, meaning Equalised Odds cannot be satisfied.
Similarly, if a recidivism model satisfies Demographic Parity, then the chance a defendant ends up in any particular risk category is the same, regardless of their race. If one group has a higher recidivism rate than the others, that means models must make more false negatives for that group to maintain Demographic Parity, which (again) means Equalised Odds cannot be satisfied. Similar arguments apply for other notions of fairness.
It is worth noting that none of these fairness metrics take into account whether or not a given group is more likely to be arrested than another, or treated differently by a given prosecution service. This example serves to illustrate both the mutual incompatibility of many metrics, and their distinct limitations in context.
Mitigating bias
Once an organisation has understood how different statistical definitions of fairness are relevant to their context, and relate to institutional goals, they can then be used to detect, and potentially mitigate, bias in their statistical approaches. Detection protocols and interventions can take place before, during, or after an algorithm is deployed.
Pre-processing protocols and interventions generally concern training data, aiming to detect and remove sources of unfairness before a model is built. Modified data can then be used for any algorithmic approach. Fairness-focused changes in decision-making then exist at the most fundamental level. However, the nature of a given application should inform data and definitions of fairness used. If an organisation seeks to equalise the odds of particular outcomes for different groups (an Equalised Odds approach), pre-processing needs to be informed by those outcomes, and a prior round of model output. Most of the pre-processing interventions present in the machine learning literature do not incorporate model outcomes, only inputs and the use of protected attributes. Some pre-processing methods only require access to the protected attributes in the training data, and not in the test data.[footnote 151] In finance it is clear that companies place more emphasis on detecting and mitigating bias in the pre-processing stages (by carefully selecting variables and involving human judgement in the loop) than in- or post-processing.
In-processing methods are applied during model training and analyse or affect the way a model operates. This typically involves a model’s architecture or training objectives, including potential fairness metrics. Modification (and often retraining) of a model can be an intensive process but the resulting high level of specification to a particular problem can allow models to retain a higher level of performance against their (sometimes new) goals. Methods such as constrained optimisation have been used to address both Demographic Parity and Equalised Odds requirements[footnote 152]. In-processing is a rapidly evolving field and often highly model dependent. It is also the biggest opportunity in terms of systemic fairness, but many approaches need to be formalised, incorporated into commonly used toolsets and, most importantly, be accompanied with legal certainty (see below).
Post-processing approaches concern a model’s outputs, seeking to detect and correct unfairness in its decisions. This approach only requires scores or decisions from the original model and corresponding protected attributes or labels that otherwise describe the data used. Post-processing approaches are usually model-agnostic, without model modification or retraining. However, they effectively flag and treat symptoms of bias, not original causes. They are also often disconnected from model development, and are relatively easy to distort, making them risky if not deployed as part of a broader oversight process.
Different interventions at different stages can sometimes be combined. Striving to achieve a baseline level of fairness in a model via pre-processing, but then looking for bias in particularly sensitive or important decisions during post-processing is an attractive approach. Care must be taken, however, that combinations of interventions do not hinder each other.
Bias mitigation methods by stage of intervention and notion of fairness are shown in Appendix A. Detailed references can then be found in Faculty’s “Bias identification and mitigation in decision-making algorithms”, published separately.
Practical challenges
There are a number of challenges facing organisations attempting to apply some of these statistical notions of fairness in practical situations:
Using statistical notions of fairness appropriately
Statistical definitions of fairness deliver specific results to specific constraints. They struggle to encompass wider characteristics that do not lend themselves to mathematical formulation.
There is no clear decision-making framework (logistical or legal) for selecting between definitions. Decisions over which measure of fairness to impose need extensive contextual understanding and domain knowledge beyond issues of data science. In the first instance, organisations should strive to understand stakeholder and end-user expectations around fairness, scale of impact and retention of agency, and consider these when setting desired outcomes.
Any given practitioner is then forced, to some extent, to choose or mathematically trade off between different definitions. Techniques to inform this trade off are currently limited. Although exceptions exist[footnote 153] there seems to be a gap in the literature regarding trade-offs between different notions of fairness, and in the general maturity of the industry in making such decisions in a holistic way (i.e. not relying on data science teams to make them in isolation).
Compatibility with accuracy
A large part of the machine learning literature on fairness is concerned with the trade-off between fairness and accuracy, i.e. ensuring that the introduction of fairness metrics has minimal impacts on model accuracy. In a pure statistical sense there is often a genuine trade-off here; imposing a constraint on fairness may lower the statistical accuracy rate. But this is often a false trade-off when thinking more holistically. Applying a fairness constraint to a recruitment algorithm sifting CVs might lower accuracy measured by a loss function over a large dataset, but doesn’t necessarily mean that company recruiting is sifting in worse candidates, or that the company’s sense of accuracy is free from historic bias.
The effects of accuracy are relevant even when models attempt to satisfy fairness metrics in ways that run counter to wider notions of fairness. Random allocation of positions in a company would likely satisfy Demographic Parity, but would not generally be considered fair. Implementing any specific fairness measure also fundamentally changes the nature of what a model is trying to achieve. Doing so may make models ‘less accurate’ when compared to prior versions. This apparent incompatibility can lead to models being seen as less desirable because they are less effective at making choices that replicate those of the past. Debiasing credit models might require accepting ‘higher risk’ loans, and thus greater capital reserves, but (as mentioned below) these choices do not exist in isolation.
Accuracy can in itself be a fairness issue. Notions of accuracy that are based on average outcomes, or swayed by outcomes for specific (usually large) demographic groups, may miss or conceal substantial biases in unexpected or less evident parts of a model’s output. Accuracy for one individual does not always mean accuracy for another.
Organisations need to consider these trade-offs in the round, and understand the limitations of purely statistical notions of both fairness and accuracy when doing so.
Understanding causality and reasons for unfairness
Causes of unfairness are not part of these definitions and must be assessed on an organisational level. Most techniques look at outcomes, and can’t understand how they come to be, or why biases may exist (except in specific circumstances). Defining fairness based on causal inference[footnote 154] has only been developed to a limited extent[footnote 155] due to the difficulty of validating underlying (apparent) causal factors. Real-world definition of these factors can introduce further bias, especially for less well understood groups with less data.
Static measurement and unintended consequences
Definitions of fairness are “static”, in the sense that we measure them on a snapshot of the population at a particular moment in time. However, a static view of fairness neglects that most decisions in the real world are taken in sequence. Making any intervention into model predictions, their results, or the way decisions are implemented will cause that population to change over time. Failing to account for this risks leading to interventions that are actively counter-productive, and there are cases where a supposedly fair intervention could lead to greater unfairness.[footnote 156] There is the scope for unintended consequence here, and strategic manipulation on the part of individuals. However, the cost of manipulation will typically be higher for any disadvantaged group. Differing costs of manipulation can result in disparities between protected groups being exaggerated.[footnote 157] In implementing a process to tackle unfairness, organisations must deploy sufficient context-aware oversight, and development teams must ask themselves if they have inadvertently created the potential for new kinds of bias. Checking back against reference data is especially useful over longer time periods.
Legal and policy issues
Although these bias mitigation techniques can seem complex and mathematical, they are encoding fundamental policy choices concerning organisational aims around fairness and equality, and there are legal risks. Organisations must bring a wide range of expertise into making these decisions. A better set of common language and understanding between the machine learning and equality law communities would assist this.
Seeking to detect bias in decision-making processes, and to address it, is a good thing. However, there is a need for care in how some of the bias mitigation techniques listed above are applied. Interventions can affect the outcomes of decisions about individuals, and even if the intent is to improve fairness, this must be done in a way that is compatible with data protection and equality law.
Many of the algorithmic fairness tools currently in use have been developed under the US regulatory regime, which is based on a different set of principles to those in the UK and includes different ideas of fairness that rely on threshold levels (most notably the “4/5ths” rule), and enable affirmative action to address imbalances. Tools developed in the US may not be fit for purpose in other legal jurisdictions.
Advice to industry
Where organisations operating within the UK deploy tools developed in the US, they must be mindful that relevant equality law (along with that across much of Europe) is different.
This uncertainty presents a challenge to organisations seeking to ensure their use of algorithms is fair and legally compliant. Further guidance is needed in this area (an example of the general need for clarity on interpretation discussed in Chapter 9); our understanding of the current position is as follows.
Data Protection law
The bias mitigation interventions discussed involve the processing of personal data, and therefore must have a lawful basis under data protection law. Broadly speaking the same considerations apply as for any other use of data; it must be collected, processed and stored in a lawful, fair and transparent manner, for specific, explicit and legitimate purposes. Its terms of use must be adequately communicated to the people it describes.
The ICO has provided guidance on how to ensure that processing of this type complies with data protection law, along with some examples, in their recently published guidance on AI and data protection.[footnote 158] Processing data to support the development of fair algorithms is a legitimate objective (provided it is lawful under the Equality Act, see below), and broadly speaking if the right controls are put in place, data protection law does not seem to present a barrier to these techniques.
There are some nuances to consider, especially for pre-processing interventions involving modification of labels on training data. In usual circumstances modifying personal data to be inaccurate would be inappropriate. However, where alterations made to training data are anonymised and not used outside of model development contexts, this can be justified under data protection legislation if care is taken. Required care might include ensuring that model features cannot be related back to an individual, the information that is stored is never used directly to make decisions about an individual, and that there is a lawful basis for processing the data in this way to support training a model (whether consent or another basis).
Particular care is needed when dealing with Special Category data, which requires additional protections under data protection law.[footnote 159] While special category data is allowed to be used for measuring bias, this explicitly excludes decisions about individuals: which would include many mitigation techniques, particularly in post-processing. Instead, automated processing of special category data would need to rely on explicit consent from its subjects, or one of a small number of explicit exceptions. It is not enough to rest on the proportionate means to legitimate ends provision (in this case, fairer models) that otherwise applies.
Equality law
The position regarding the Equality Act 2010 is less clear.
All of the mitigation approaches discussed in this section are intended to reduce bias, including indirect discrimination. However, there is a risk that some of the techniques used to do this could themselves be a cause of new direct discrimination. Even if “positive”, i.e. discrimination to promote equality for a disadvantaged group, this is generally illegal under the Equality Act.
It is not yet possible to give definitive general guidance on exactly which techniques would or would not be legal in a given situation; organisations will need to think this through on a case-by-case basis. Issues to consider might include:
-
Explicit use of a protected characteristic (or relevant proxies) to reweight models to achieve a fairness metric (e.g. in some applications of Feature Modification, or Decision Threshold Modification) carries risk. Organisations need to think through whether the consequence of using such a technique could disadvantage an individual explicitly on the basis of a protected characteristic (which is direct discrimination) or otherwise place those individuals at a disadvantage (which can lead to indirect discrimination).
-
Resampling data to ensure a representative set of inputs is likely to be acceptable; even if it did have a disparate impact across different groups any potential discrimination would be indirect, and likely justifiable as a proportionate means to a legitimate end.
Though there is a need for caution here, the legal risk of attempting to mitigate bias should not be overplayed. If an organisation’s aim is legitimate, and decisions on how to address this are taken carefully with due regard to the requirements of the Equality Act, then the law will generally be supportive. Involving a broad team in these decisions, and documenting them (e.g. in an Equality Impact Assessment) is good practice.
If bias exists, and an organisation can identify a non-discriminatory approach to mitigate that, then there seems to be an ethical responsibility to do so. If this can’t be done at the level of a machine learning model itself, then wider action may be required. Organisations developing and deploying algorithmic decision-making should ensure that their mitigation efforts do not lead to direct discrimination, or outcome differences without objective justification.
Despite the complexity here, algorithmic fairness approaches will be essential to facilitate widespread adoption of algorithmic decision-making.
Advice to industry
Where organisations face historical issues, attract significant societal concern, or otherwise believe bias is a risk, they will need to measure outcomes by relevant protected characteristics to detect biases in their decision-making, algorithmic or otherwise. They must then address any uncovered direct discrimination, indirect discrimination, or outcome differences by protected characteristics that lack objective justification.
In doing so, organisations should ensure that their mitigation efforts do not produce new forms of bias or discrimination. Many bias mitigation techniques, especially those focused on representation and inclusion, can legitimately and lawfully address algorithmic bias when used responsibly. However, some risk introducing positive discrimination, which is illegal under the Equality Act. Organisations should consider the legal implications of their mitigation tools, drawing on industry guidance and legal advice.
The best approach depends strongly on the use case and context. Interviews with organisations in the finance sector did not reveal a commonly used approach; companies use a mix of in-house and external tools. There is a general appetite for adapting open-source tools to internal uses, and among the companies consulted, none had developed in-house tools from scratch. In recruitment, we found that vendors of machine learning tools had established processes for examining their models, both off-the-shelf and bespoke tools. The most elaborate processes had three stages: pre-deployment checks with dummy data or sampled real-world data on models prior to deployment; post deployment checks where anonymised data from customers was used for further adjustments and correction of over-fitting; and third-party audits conducted by academic institutions particularly focused on identifying sources of bias. Firms used a mixture of proprietary techniques and open-source software to test their models.
In terms of mitigation, there is a lot that can be done within the current legislative framework, but regulators will need to keep an eye on the way the law is applied, what guidance is needed to guide ethical innovation and whether the law might need to change in the future. Engagement with the public and industry will be required in many sectors to identify which notions of fairness and bias mitigation approaches are acceptable and desirable.
Recommendations to regulators
Recommendation 9: Sector regulators and industry bodies should help create oversight and technical guidance for responsible bias detection and mitigation in their individual sectors, adding context-specific detail to the existing cross-cutting guidance on data protection, and any new cross-cutting guidance on the Equality Act.
We think it is likely that a significant industry and ecosystem will need to develop with the skills to audit systems for bias, in part because this is a highly specialised skill that not all organisations will be able to support; in part because it will be important to have consistency in how the problem is addressed; and in part because regulatory standards in some sectors may require independent audit of systems. Elements of such an ecosystem might be licenced auditors or qualification standards for individuals with the necessary skills. Audit of bias is likely to form part of a broader approach to audit that might also cover issues such as robustness and explainability.
7.5 Anticipatory Governance
Within an organisation, especially a large one, good intentions in individual teams are often insufficient to ensure that the organisation as a whole achieves the desired outcome. A proportionate level of governance is usually required to enable this. What does this look like in this context?
There is no one-size-fits-all approach, and unlike in some other areas (e.g. health and safety or security management), not yet an agreed standard on what such an approach should include. However, there is an increasing range of tools and approaches available. What is clear is that, given the pace of change, and the wide range of potential impacts, governance in this space must be anticipatory.
Anticipatory Governance aims to foresee potential issues with new technology, and intervene before they occur, minimising the need for advisory or adaptive approaches, responding to new technologies after their deployment. Tools, ways of working, and organisations already exist to help proactively and iteratively test approaches to emerging challenges while they are still in active development. The goal is to reduce the amount of individual regulatory or corrective action and replace it with more collaborative solutions to reduce costs, and develop best practice, good standards, policy and practice.
In practical terms, assessment of impacts and risks, and consultation with affected parties, are core to doing this within individual organisations. However, it is critical that they aren’t simply followed as tick box procedures. Organisations need to show genuine curiosity about the short, medium and long term impacts of increasingly automated decision-making, and ensure that they have considered the views of a wide range of impacted parties both within their organisation and in wider society. Assessments must not only consider the detail of how an algorithm is implemented, but whether it is appropriate at all in the circumstances, and how and where it interacts with human decision-makers.
There are many published frameworks and sets of guidance offering approaches to structuring governance processes[footnote 160], including guidance from GDS and the Alan Turing Institute targeted primarily at the UK public sector. [footnote 161] Different approaches will be appropriate to different organisations, but some key questions that should be covered include the following.
Guidance to organisation leaders and boards
Those responsible for governance of organisations deploying or using algorithmic decision-making tools to support significant decisions about individuals should ensure that leaders are in place with accountability for:
-
Understanding the capabilities and limits of those tools.
-
Considering carefully whether individuals will be fairly treated by the decision-making process that the tool forms part of.
-
Making a conscious decision on appropriate levels of human involvement in the decision-making process.
-
Putting structures in place to gather data and monitor outcomes for fairness.
-
Understanding their legal obligations and having carried out appropriate impact assessments.
This especially applies in the public sector when citizens often do not have a choice about whether to use a service, and decisions made about individuals can often be life-affecting.
The list above is far from exhaustive, but organisations that consider these factors early on, and as part of their governance process, will be better placed to form a robust strategy for fair algorithmic deployment. In Chapters 8 and 9 below we discuss some of the more specific assessment processes (e.g. Data Protection Impact Assessments, Equality Impact Assessments, Human Rights Impact Assessments) which can provide useful structures for doing this.
8. The regulatory environment
Summary
Overview of findings:
-
Regulation can help to address algorithmic bias by setting minimum standards, providing clear guidance that supports organisations to meet their obligations, and enforcement to ensure minimum standards are met.
-
AI presents genuinely new challenges for regulation, and brings into question whether existing legislation and regulatory approaches can address these challenges sufficiently well. There is currently little case law or statutory guidance directly addressing discrimination in algorithmic decision-making.
-
The current regulatory landscape for algorithmic decision-making consists of the Equality and Human Rights Commission (EHRC), the Information Commissioner’s Office (ICO) , and sector regulators and non-government industry bodies. At this stage, we do not believe that there is a need for a new specialised regulator or primary legislation to address algorithmic bias.
-
However, algorithmic bias means that the overlap between discrimination law, data protection law and sector regulations is becoming increasingly important. This is particularly relevant for the use of protected characteristics data to measure and mitigate algorithmic bias, the lawful use of bias mitigation techniques, identifying new forms of bias beyond existing protected characteristics, and for sector-specific measures of algorithmic fairness beyond discrimination.
-
Existing regulators need to adapt their enforcement to algorithmic decision-making, and provide guidance on how regulated bodies can maintain and demonstrate compliance in an algorithmic age. Some regulators require new capabilities to enable them to respond effectively to the challenges of algorithmic decision-making. While larger regulators with a greater digital remit may be able to grow these capabilities in-house, others will need external support.
Recommendations to government:
-
Recommendation 10: Government should issue guidance that clarifies the Equality Act responsibilities of organisations using algorithmic decision-making. This should include guidance on the collection of protected characteristics data to measure bias and the lawfulness of bias mitigation techniques.
-
Recommendation 11: Through the development of this guidance and its implementation, government should assess whether it provides both sufficient clarity for organisations on their obligations, and leaves sufficient scope for organisations to take actions to mitigate algorithmic bias. If not, government should consider new regulations or amendments to the Equality Act to address this.
Recommendations to regulators:
-
Recommendation 12: The EHRC should ensure that it has the capacity and capability to investigate algorithmic discrimination. This may include EHRC reprioritising resources to this area, EHRC supporting other regulators to address algorithmic discrimination in their sector, and additional technical support to the EHRC.
-
Recommendation 13: Regulators should consider algorithmic discrimination in their supervision and enforcement activities, as part of their responsibilities under the Public Sector Equality Duty.
-
Recommendation 14: Regulators should develop compliance and enforcement tools to address algorithmic bias, such as impact assessments, audit standards, certification and/or regulatory sandboxes.
-
Recommendation 15: Regulators should coordinate their compliance and enforcement efforts to address algorithmic bias, aligning standards and tools where possible. This could include jointly issued guidance, collaboration in regulatory sandboxes, and joint investigations.
Advice to industry:
- Industry bodies and standards organisations should develop the ecosystem of tools and services to enable organisations to address algorithmic bias, including sector- specific standards, auditing and certification services for both algorithmic systems and the organisations and developers who create them.
Future CDEI work:
-
CDEI plans to grow its ability to provide expert advice and support to regulators, in line with our existing terms of reference. This will include supporting regulators to coordinate efforts to address algorithmic bias and to share best practice.
-
CDEI will monitor the development of algorithmic decision-making and the extent to which new forms of discrimination or bias emerge. This will include referring issues to relevant regulators, and working with government if issues are not covered by existing regulations.
8.1 Introduction
This report has shown the problem of algorithmic bias, and ways that organisations can try to address the problem. There are good reasons for organisations to address algorithmic bias, ranging from ethical responsibility through to pressure from customers and employees. These are useful incentives for companies to try to do the right thing, and can extend beyond minimum standards to creating a competitive advantage for firms that earn public trust.
However, the regulatory environment can help organisations to address algorithmic bias in three ways. Government can set clear minimum standards through legislation that prohibits unacceptable behaviour. Government, regulators and industry bodies can provide guidance and assurance services to help organisations correctly interpret the law and meet their obligations. Finally, regulators can enforce these minimum standards to create meaningful disincentives for organisations who fail to meet these obligations.
Alternatively, a regulatory environment with unclear requirements and weak enforcement creates the risk that organisations inadvertently break the law, or alternatively that this risk prevents organisations from adopting beneficial technologies. Both of these situations are barriers to ethical innovation, which can be addressed through clear and supportive regulation.
Data-driven technologies and AI present a range of new challenges for regulators. The rapid development of new algorithmic systems means they now interact with many aspects of our daily lives. These technologies have the power to transform the relationship between people and services across most industries by introducing the ability to segment populations using algorithms trained on larger and richer datasets. However, as we have seen in our sector-focused work, there are risks of these approaches reinforcing old biases, or introducing new ones, by treating citizens differently due to features beyond their control, and in ways they may not be aware of. The regulatory approach of every sector where decision-making takes place about individuals will need to adapt and respond to these new practices that algorithmic decision-making brings.
Given this widespread shift, it is necessary to reflect both on whether the existing regulatory and legislative frameworks are sufficient to deal with these novel challenges, as well as how compliance and enforcement may operate in an increasingly data-driven world. For example, regulatory approaches that rely on individual complaints may not be sufficient in a time where people are not always aware of how an algorithm has impacted their life. Similarly, the pace of change in the development of decision-making technologies may mean that certain approaches are too slow to respond to the new ways algorithms are already impacting people’s lives. Regulators will need to be ambitious in their thinking, considering the ways algorithms are already transforming their sectors, and what the future may require.
The government and some regulators have already recognised the need for anticipatory regulation to respond to these challenges. Regulation For The Fourth Industrial Revolution[footnote 162] lays out the challenge as a need for proactive, flexible, outcome-focused regulation, enabling greater experimentation under appropriate supervision, and supporting innovators to actively seek compliance. It also details the need for regulators to build dialogue across society and industry, and to engage in global partnerships. NESTA adds[footnote 163] that such regulation should be inclusive and collaborative, future-facing, iterative, and experimental, with methods including “sandboxes: experimental testbeds; use of open data; interaction between regulators and innovators; and, in some cases, active engagement of the public”. In this section we look at both the current landscape, and the steps required to go further.
8.2 Current landscape
The UK’s regulatory environment is made up of multiple regulators, enforcement agencies, inspectorates and ombudsmen (which this report will call ‘regulators’ for simplicity) with a range of responsibilities, powers and accountabilities. These regulators are typically granted powers by the primary legislation that established them, although some ‘private regulators’ may be set up through industry self-regulation.
Some regulators have an explicit remit to address bias and discrimination in their enabling legislation, while others may need to consider bias and discrimination in decision-making when regulating their sectors. In practice, however, there is a mixed picture of responsibility and prioritisation of the issue.
Data-driven algorithms do not necessarily replace other decision-making mechanisms wholesale, but instead fit into existing decision-making processes. Therefore, rather than a new algorithmic regulatory system, the existing regulatory environment needs to evolve in order to address bias and discrimination in an increasingly data-driven world.
The key piece of legislation that governs discrimination is the Equality Act 2010. The Act provides a legal framework to protect the rights of individuals and provides discrimination law to protect individuals from unfair treatment, including through algorithmic discrimination. Underlying anti-discrimination rights are also set out in the Human Rights Act 1998 (which establishes the European Convention on Human Rights in UK law). When a decision is made by an organisation on the basis of recorded information (which is the case for most significant decisions), the Data Protection Act 2018 and the General Data Protection Regulation (GDPR) are also relevant. This legislation controls how personal information is used by organisations, businesses or the government and sets out data protection principles which includes ensuring that personal information is used lawfully, fairly and transparently. Data protection law takes on a higher level of relevance in the case of algorithmic decision-making, where decisions are inherently data-driven, and specific clauses related to automated processing and profiling apply (see below for more details).
In support of this legislation, there are two primary cross-cutting regulators: the Equality and Human Rights Commission (EHRC, for the Equality Act and Human Rights Act) and the Information Commissioner’s Office (ICO, for the Data Protection Act and GDPR).
However, given the range of types of decisions that are being made with the use of algorithmic tools, there is clearly a limit in how far cross-cutting regulators can define and oversee what is acceptable practice. Many sectors where significant decisions are made about individuals have their own specific regulatory framework with oversight on how those decisions are made.
These sector regulators have a clear role to play: algorithmic bias is ultimately an issue of how decisions are made by organisations, and decision-making is inherently sector-specific. In sectors where algorithmic decision-making is already significant, the relevant enforcement bodies are already considering the issues raised by algorithmic decision-making tools, carrying out dedicated sector-specific research and increasing their internal skills and capability to respond.
Overall, the picture is complex, reflecting the overlapping regulatory environment of different types of decisions. Some have called for a new cross-cutting algorithms regulator, for example Lord Sales of the UK Supreme Court.[footnote 164] We do not believe that this is the best response to the issue of bias, given that many of the regulatory challenges raised are inevitably sector-specific, and typically algorithms only form part of an overall decision-making process regulated at sector level. However, more coordinated support for and alignment between regulators may be required (see below) to address the challenge across the regulatory landscape.
8.3 Legal background
Equality Act
The Equality Act 2010 (the Act) legally protects people from discrimination and sets out nine ‘protected characteristics’ which it is unlawful to discriminate on the basis of:
- age
- disability
- gender reassignment
- marriage and civil partnership
- pregnancy and maternity
- race
- religion or belief
- sex
- sexual orientation
The Act prohibits direct discrimination, indirect discrimination, victimisation and harassment based on these characteristics.[footnote 165] It also establishes a requirement to make reasonable adjustments for people with disabilities, and allows for, but does not require, ‘positive action’ to enable or encourage the participation of disadvantaged groups. The Act also establishes the Public Sector Equality Duty[footnote 166] which requires all public sector bodies to address inequality through their day-to-day activities.
The Equality Act has effect in England, Wales and Scotland. Although Northern Ireland has similar anti-discrimination principles, they are covered in different legislation. There are some legal differences in the scope of protected characteristics (e.g. political opinions are protected in Northern Ireland), thresholds for indirect discrimination, and some practical differences in the Public Sector Equality Duty. However, for the purpose of this report, we will use the language of the Equality Act.
Section 1 of the Equality Act requires public bodies to actively consider the socio-economic outcomes of any given policy. It is currently in effect in Scotland, and will commence in Wales next year. Increasingly large parts of the public sector (and those contracted by it) must show that they have given due diligence to such issues ahead of time, as part of their development and oversight chain. Recent controversies over exam results have highlighted broad public concern about socio-economic disparities.
Each of these provisions apply to any area where individuals are treated differently, regardless of whether an algorithm was involved in the decision.
Human Rights Act
The UK also protects against discrimination in the Human Rights Act 1998, which establishes the European Convention on Human Rights in UK domestic law. This Act explicitly prohibits discrimination in Article 14: “The enjoyment of the rights and freedoms set forth in this Convention shall be secured without discrimination on any ground such as sex, race, colour, language, religion, political or other opinion, national or social origin, association with a national minority, property, birth or other status.”
This is a broader set of characteristics, notably preventing discrimination based on language, political opinion and property. However, this also provides narrower protection than the Equality Act, as it applies specifically to realising the other human rights in the Act. This means that government bodies cannot discriminate based on these characteristics when granting or protecting rights such as the right to a fair trial (Article 6), freedom of expression (Article 10), or freedom of assembly (Article 11).
The Council of Europe has recently established an Ad-hoc Committee on AI (CAHAI)[footnote 167] to consider a potential legal framework to support the application of AI based on human rights, democracy and the rule of law.
Data Protection Law
The Data Protection Act 2018 alongside the EU General Data Protection Regulation (GDPR) regulates how personal information is processed[footnote 168] by organisations, businesses or the government. The Data Protection Act supplements and tailors the GDPR in UK domestic law. Under data protection law, organisations processing personal data must follow data protection principles, which includes ensuring that information is used lawfully, fairly and transparently.
Data protection law gives individuals (“data subjects” in GDPR language) a number of rights that are relevant to algorithmic decision-making, for example the right to find out what information organisations store about them, including how their data is being used. There are additional specific rights when an organisation is using personal data for fully automated decision-making processes and profiling which have legal or other significant effects on individuals. The introduction of the Data Protection Act and the GDPR, which make organisations liable for significant financial penalties for serious breaches, has led to a strong focus on data protection issues at the top level of organisations, and a significant supporting ecosystem of guidance and consultancy helping organisations to comply.
A wide range of data protection provisions are highly relevant to AI generally, and automated decision-making, and there has been widespread public commentary (both positive and negative) on approaches to training and deploying AI tools compliant with them.[footnote 169] The GDPR sets out other provisions relating to algorithmic bias and discrimination, including:
- Principle for data processing to be lawful and fair. In Article 5(1), there is the general principle that personal data must be “processed lawfully, fairly and in a transparent manner”.[footnote 170] The lawfulness requirement means that data processing must be compliant with other laws, including the Equality Act. The fairness requirement means that the processing is not “unduly detrimental, unexpected, or misleading” to data subjects.
-
Provisions around the illegality of discriminatory profiling. In Recital 71, the GDPR advises that organisations should avoid any form of profiling that results in “discriminatory effects on natural persons on the basis of racial or ethnic origin, political opinion, religion or beliefs, trade union membership, genetic or health status or sexual orientation, or processing that results in measures having such an effect.”
-
Data subjects have a right to not be subject to a solely automated decision-making process with significant effects. Article 22(1) states that “The data subject shall have the right not to be subject to a decision based solely on automated processing, including profiling, which produces legal effects concerning him or her or similarly significantly affects him or her.” The ICO specifies[footnote 171] that organisations have proactive obligations to bring details of these rights to the attention of individuals.
- Under Article 7.3, the rights of data subjects to withdraw their consent for processing of their data at any time, and under Article 21 the right to object to data processing carried out under a legal basis other than consent.
Data protection legislation provides several strong levers to ensure procedural fairness. However, there are some inherent limitations in thinking about fair decisions purely through the lens of data protection; processing of personal data processing is a significant contributor to algorithmic decisions, but is not the decision itself, and other considerations less directly relevant to data may apply. Data protection should therefore not be seen as the entirety of regulation applying to algorithmic decisions. Efforts to comply with data protection law must not distract organisations from considering other ethical and legal obligations, for example those defined in the Equality Act.
Consumer protection and sector-specific legislation
Beyond the three cross-cutting Acts above, additional laws establish fair or unfair conduct in a specific area of decision-making. These laws also apply in principle where this conduct is made or supported by an algorithm, although this is often untested in case law.
Consumer Protection law such as the Consumer Rights Act 2015 sets out consumer rights around misleading sales practices, unfair contract terms, and defective products and services. This law sets out cross-sector standards for commercial behaviour, but is typically enforced through sector-specific Ombudsmen.
Some regulated sectors, particularly those that are consumer facing, set out additional requirements for fair treatment, notably the Financial Conduct Authority’s principles[footnote 172] for fair treatment of customers, or Ofcom’s framework for assessing fairness in telecommunications services.[footnote 173] Again, algorithmic decisions would still remain subject to these rules, though it is not always clear how algorithmic decision-making could meet them in practice. The requirement for consumers to be “provided with clear information” and to be “kept appropriately informed before, during and after the point of sale” is straightforward to apply to algorithmic processes, but ‘consumers can be confident they are dealing with firms where the fair treatment of customers is central to the corporate culture’ is less clear.
Limitations of current legislation
As previously discussed, the Equality Act defines a list of protected characteristics which it is unlawful to use as the basis for less favourable treatment. These characteristics reflect the evidence of systematic discrimination at a point in time, and can (and should) evolve as new forms of discrimination emerge and are recognised by society and the legal system.
There are also multiple situations where algorithms could potentially lead to unfair bias that does not amount to discrimination, such as bias based on non-protected characteristics.[footnote 174] In some cases we may expect the emergence of new protected characteristics to cover these issues, but this will reflect society recognising new forms of discrimination that have been amplified by algorithms, rather than the use of algorithms themselves creating a new type of discrimination.
While these situations challenge current equality legislation, they do not imply that an entirely new framework is required for algorithmic decision-making. In these examples, data protection legislation would offer affected people some levers to understand and challenge the process by which these decisions had been reached. Furthermore, the requirement for ‘fair’ data processing under GDPR could mean that this kind of bias is non-compliant with data protection law, but this is legally untested.
In the public sector, bias based on arbitrary characteristics could also be challenged under the Human Rights Act where Article 14 prohibits discrimination based on ‘other status’, although any specific type of arbitrary bias would also need to be tested by the courts.
Therefore, we do not believe there is evidence to justify an entirely new legislative or regulatory regime for algorithmic bias. Furthermore, a specific regulatory regime for algorithmic bias would risk inconsistent standards for bias and discrimination across algorithmic and non-algorithmic decisions, which we believe would be unworkable.
Instead, the current focus should be on clarifying how existing legislation applies to algorithmic decision-making, ensuring that organisations know how to comply in an algorithmic context, alongside effective enforcement of these laws to algorithmic decision-making.
This is a matter of some urgency; as we have set out in this report, there are clearly risks that algorithmic decision-making can lead to discrimination. This is unlawful and the application of current legislation must be clear and enforced accordingly to ensure bad practice is reduced as much as possible.
Case law on the Equality Act
While legislation sets out the principles and minimum requirements for behaviour, these principles need to be interpreted in order to be applied in practice. This interpretation can occur by individual decision makers and/or regulators, but this interpretation is only definitive when tested by the courts.
While there is a growing body of case law that addresses algorithms in data protection law, there have been very few examples of litigation in which algorithmic or algorithm supported decisions have been challenged under the Equality Act. In the absence of such case law, such interpretations are inherently somewhat speculative.
One of the few examples was on the use of facial recognition technology by South Wales Police, which was recently challenged via a judicial review, both on data protection and Equality Act grounds:
Case study: Facial recognition technology
One of the few legal cases to test the regulatory environment of algorithmic bias was on the use of live facial recognition technology by police forces, following concerns around violations of privacy and potential biases within the system. Facial recognition technology has been frequently criticised for performing differently against people with different skin tones, meaning accuracy of many systems is often higher for white men compared to people with other ethnicities.
South Wales Police have trialled the use of live facial recognition in public spaces on several occasions since 2017. These trials were challenged through judicial review, and were found unlawful in the Court of Appeal on 11 August 2020.
One of the grounds for successful appeal was that South Wales Police failed to adequately consider whether their trial could have a discriminatory impact, and specifically that they did not take reasonable steps to establish whether their facial recognition software contained biases related to race or sex. In doing so, the court found that they did not meet their obligations under the Public Sector Equality Duty.
Note that in this case there was no evidence that this specific algorithm was biased in this way, but that South West Police failed to take reasonable steps to consider this. This judgement is very new as this report goes to press, but it seems likely that this could have significant legal implications for public sector use of algorithmic decision-making, suggesting that the Public Sector Equality Duty requires public sector organisations to take reasonable steps to consider potential bias when deploying algorithmic systems, and to detect algorithmic bias on an ongoing basis.
Beyond this, we are not aware of any other litigation in which the use of AI in decision-making has been challenged under the Equality Act. This means there is little understanding of what the Equality Act requires in relation to data-driven technology and AI. Whilst it is clear that if an algorithm was using a protected characteristic as input into a model and was making decisions on this basis, that would likely constitute discrimination, it is less clear in what circumstances use of variables that correlate with protected characteristics would be considered (indirectly) discriminatory.
The Equality Act states that in some cases, apparent bias may not constitute indirect discrimination if it involves proportionate means of achieving a legitimate aim. There is guidance and case law to help organisations understand how to interpret this in a non-algorithmic context.
However in algorithmic decision-making this is perhaps less clear. For example, the ruling by the European Court of Justice in the Test-Achats case made it unlawful for insurers to charge different rates based on sex or gender.[footnote 177] UK car insurance providers had routinely charged higher premiums for men, based on their higher expected claims profile. These insurers responded by pricing insurance with more opaque algorithms based on other observable characteristics such as occupation, car model and size of engine, or even telematics that tracked individual driver behaviour. This change eliminated direct discrimination by sex and arguably shifted pricing towards more ‘objective’ measures of insurance risk. However, auto insurance prices remain significantly higher for men, and it is unclear and legally untested where these algorithms cross from legitimate pricing based on risk, to indirect discrimination based on proxies for sex, such as occupation.
The lack of case law has meant organisations are often left to figure out the appropriate balance for themselves or look to international standards that do not necessarily reflect the equality framework in the UK. The uncertainty in this area is both a risk to fairness and a constraint on innovation. Guidance on appropriate good practice would help organisations navigate some of these challenges, as well as help understand the parameters of what is considered acceptable within the law.
Regulations and guidance
Government and regulators have several ways to provide clearer guidance on how to interpret the law. These types of guidance and regulations differ in their legal status and their audience.
Statutory Codes of Practice are provided by regulators to clarify how existing law applies to a particular context. These are typically prepared by a regulator but presented by a minister in parliament. These codes and guidelines are legal in nature, and are targeted at courts, lawyers and other specialists such as HR professionals. Technical guidelines are similar to statutory codes, but are prepared by a regulator without statutory backing. Courts are not required to follow them, but will generally consider them (and whether an organisation followed them) as evidence. They must draw from existing statute and case law, and focus on how to apply the existing law to particular situations.
Regulators can also issue guidance as information and advice for particular audiences, e.g. for employers or service providers. This could extend beyond current statute and case law, but must be compatible with the existing law. EHRC guidance is harmonised with statutory codes, and is focused on making the existing legal rights and obligations accessible to different audiences such as employers or affected individuals. ICO guidance often takes a similar approach, though some ICO guidance (such as that on AI) offers additional best practice recommendations which organisations are not required to follow if they can find another way to meet their legal obligations.
The issues of algorithmic bias raised in this report require both clarification of the existing law, and more practical guidance that supports different stakeholders to understand and meet their obligations. In particular, organisations need clarity on the lawfulness of bias mitigation techniques, so that they can understand what they can do to address bias
This clarification of existing law requires detailed knowledge of both employment law and how bias mitigation techniques work. This cross-functional effort should be led by government in order to provide official sanction as government policy, but draw on relevant expertise across the broader public sector, including from EHRC and CDEI.
Recommendations to government
Recommendation 10: Government should issue guidance that clarifies the Equality Act responsibilities of organisations using algorithmic decision-making. This should include guidance on the collection of protected characteristics data to measure bias and the lawfulness of bias mitigation techniques.
It is possible that the work to clarify existing legal obligations could still leave specific areas of uncertainty on how organisations can lawfully mitigate algorithmic bias while avoiding direct positive discrimination, or highlight undesirable constraints in what is possible. We believe this situation would be unacceptable, as it could leave organisations with an ethical, and often a legal, obligation to monitor algorithmic bias risks, but make them unable to deploy proportionate methods to address the bias they find.
In this case, further clarity or amendments to equality law could be required, for example to help to clarify what lawful positive action means in the context of mitigating algorithmic bias, and where this might cross a line into unlawful (positive) discrimination.
Government can clarify or amend existing law by issuing supplementary regulations or statutory instruments. These regulations are usually implemented by a minister presenting a statutory instrument in parliament. In some areas, a regulator is specifically authorised to issue rules or regulations that are also legally enforceable, such as the Financial Conduct Authority Handbook. However, under the Equality Act, the EHRC and other regulators do not have this power, and any regulations would need to be issued by a minister. If current law is unable to provide enough clarity to allow organisations to address algorithmic bias, government should issue regulations to help clarify the law.
Recommendations to government
Recommendation 11: Through the development of this guidance and its implementation, government should assess whether it provides both sufficient clarity for organisations on their obligations, and leaves sufficient scope for organisations to take actions to mitigate algorithmic bias. If not, government should consider new regulations or amendments to the Equality Act to address this.
Beyond clarifying existing obligations, organisations need practical guidance that helps them meet their obligations. This should include their obligations under equality law, but also includes sector-specific concepts of fairness, and best practices and advice that go beyond minimum standards. As described in Recommendation 9 above, we believe that many of the specific issues and methods are likely to be sector-specific. Private sector industry bodies can also play a leadership role to facilitate best practice sharing and guidance within their industry.
8.4 The role of regulators
The use of algorithms to make decisions will develop and be deployed differently depending on the context and sector. Algorithmic decision-making is taking place increasingly across sectors and industries, and in novel ways. For algorithmic bias, both the EHRC and ICO have explicit responsibilities to regulate, while there are also responsibilities within the mandate of each sector regulator.
The Equality and Human Rights Commission
The Equality and Human Rights Commission (EHRC) is a statutory body responsible for enforcing the Equality Act 2010, as well as responsibilities as a National Human Rights Institution. Their duties include reducing inequality, eliminating discrimination and promoting and protecting human rights.
The EHRC carries out its functions through a variety of means, including providing advice and issuing guidance to ensure compliance with the law. They also take on investigations where substantial breaches of the law are suspected, however these resource intensive investigations are limited to a few high priority areas. In addition to investigations, the EHRC uses an approach of strategic litigation where they pursue legal test cases in areas where the law is unclear.[footnote 178] The EHRC is less likely to be involved in individual cases, and rather directs people to the Equality Advisory Support Service.
Given its broad mandate, the EHRC leverages its limited resources by working collaboratively with other regulators to promote compliance with the Equality Act 2010, for example by incorporating equality and human rights in sector-specific standards, compliance and enforcement. They also produce joint guidance in collaboration with sector regulators.
Within their 2019-22 strategic plan, the EHRC highlights that technology affects many equality and human rights concerns but does not currently have a strand of work specifically addressing the risks of data-driven technologies. Instead, the implications of new technologies for the justice system, transport provision and decision-making in the workplace are captured within those specific programmes.
In March 2020, the EHRC called for the suspension of the use of automated facial recognition and predictive algorithms in policing in England and Wales, until their impact has been independently scrutinised and laws are improved. However this was a specific response to a UN report and does not yet appear to be part of a wider strand of work.[footnote 179] The EHRC continues to monitor the development and implementation of such tools across policy areas to identify opportunities for strategic litigation to clarify privacy and equality implications. It also recently completed an inquiry into the experiences of people with disabilities in the criminal justice system, including the challenges arising from a move towards digital justice, and has undertaken research into the potential for discrimination in using AI in recruitment.
Due to the importance of the Equality Act in governing bias and discrimination, the EHRC has a key role to play in supporting the application and enforcement of the Equality Act to algorithmic decision-making. While the EHRC has shown some interest in these issues, we believe they should further prioritise the enforcement of the Equality Act in relation to algorithmic decision-making. This will partly involve a re-prioritisation of the EHRC’s own enforcement, but there is also room to leverage the reach of sector regulators, by ensuring they have the necessary capability to carry out investigations and provide guidance for specific contexts. Data-driven technologies present a genuine shift in how discrimination operates in the 21st Century, so the EHRC will also need to consider whether they have sufficient technical skills in this area to carry out investigations and enforcement work, and how they might build up that expertise.
Recommendations to regulators
Recommendation 12: The EHRC should ensure that it has the capacity and capability to investigate algorithmic discrimination. This may include EHRC reprioritising resources to this area, EHRC supporting other regulators to address algorithmic discrimination in their sector, and additional technical support to the EHRC.
Equalities bodies across Europe are facing similar challenges in addressing these new issues, and others have previously identified the need for additional resourcing.[footnote 180]
The Information Commissioner’s Office
The Information Commissioner’s Office (ICO) is the UK’s independent regulator for information rights. It is responsible for the implementation and enforcement of a number of pieces of legislation, including the Data Protection Act 2018 and GDPR.
The ICO has a range of powers to carry out its work:
-
It can require organisations to provide information.
-
It can issue assessment notices that enable it to assess whether an organisation is complying with data protection regulation.
-
Where it finds a breach of data protection regulation, it can issue an enforcement notice telling the organisation what it needs to do to bring itself into compliance (including the power to instruct an organisation to stop processing).
-
It can impose significant financial penalties for breaches: up to €20m or 4% of annual total worldwide turnover.
The ICO has a broad, cross-sectoral remit. It is focused on the challenge of overseeing new legislation: the interpretation and application of the GDPR is still evolving; case law under this legislation remains limited; and organisations and the public are still adapting to the new regime. The ICO has played a prominent role both in the UK and internationally in thinking about regulatory approaches to AI. Relevant activities have included:
-
Leading a Regulators and AI Working Group providing a forum for regulators, and other relevant organisations (including CDEI) to share best practice and collaborate effectively.
-
Developing, at the request of the government, detailed guidance on explainability, in partnership with the Alan Turing Institute.[footnote 181]
- Publishing guidance on AI and data protection that aims to help organisations consider their legal obligations under data protection as they develop data-driven tools. This guidance is not a statutory code, but contains advice on how to interpret relevant data protection law as it applies to AI, and recommendations on good practice for organisational and technical measures to mitigate the risks to individuals that AI may cause or exacerbate.
This activity is, in part, a reflection of the increased scope of responsibilities placed on organisations within the Data Protection Act 2018, but also reflects gradual growth in the importance of data-driven technologies over several decades. These efforts have been useful in pushing forward activity in this space.
The ICO has recently stated that bias in algorithms may fall under data protection law via the Equality Act: “The DPA 2018 requires that any processing is lawful, so compliance with the Equality Act 2010 is also a requirement of data protection law.”[footnote 182] The ICO also makes clear in its guidance that data protection also includes broader fairness requirements, for example: “Fairness, in a data protection context, generally means that you should handle personal data in ways that people would reasonably expect and not use it in ways that have unjustified adverse effects on them.”[footnote 183]
Sector and specialist regulators
In the sectors we studied in this review, relevant bodies include the Financial Conduct Authority (FCA) for financial services, Ofsted for children’s social care and HM Inspectorate of Constabulary and Fire and Rescue Services in policing. Recruitment does not fall under the remit of a specific sector regulator, although it is an area that has been a focus for the EHRC.
There are other sector regulators in areas not studied in detail in this review, e.g. Ofgem for energy services. For all consumer-facing services, the remit of the Competition and Markets Authority (CMA) is also relevant, with obligations within consumer protection legislation for consumers to be treated fairly.
Public Sector Equality Duty
Whilst the Equality Act applies to both the public and private sector, there are further provisions for the public sector under the Public Sector Equality Duty (PSED). This duty sets out a legal mandate for public authorities to undertake activity to promote equality.
A public authority must, in the exercise of its functions, have due regard to the need to:
-
eliminate discrimination, harassment, victimisation and any other conduct that is prohibited by or under the Act;
-
advance equality of opportunity between persons who share a relevant protected characteristic * and persons who do not share it;
-
foster good relations between persons who share a relevant protected characteristic and persons who do not share it.
Public authorities include sector regulators who should therefore deliver the commitments set out above. These obligations under the Equality Act provide the necessary mandate for regulators to work towards eliminating risks of discrimination from algorithmic decision-making within their sectors.
There is a mixed picture of how well enforcement bodies are equipped to respond to bias in algorithmic decision-making. There are regulators such as the FCA who have explored specific research and have been proactive in understanding and addressing these concerns through regulatory guidance such as the Draft Guidance on Fair Treatment of Vulnerable Customers.[footnote 184] The FCA has also deployed innovations such as the regulatory sandbox, which temporarily reduces regulatory requirements for selected products and services, in exchange for more direct supervision and guidance from the FCA.[footnote 185] Some other regulators, for example the CMA, are taking action to build their expertise and activities in this area. However, many others are not as well resourced, do not have the relevant expertise to develop guidance in these areas, or are not treating this issue as a priority. There are particular challenges for enforcement bodies in sectors where these tools are particularly novel.
Case study: The Financial Conduct Authority
As we set out in Chapter 4, the financial services sector is one where the use of algorithmic decision-making tools are growing in development and deployment. One of the key enforcement bodies in this sector is the FCA, who have a responsibility for consumer protection.
The FCA has focused a lot of attention on the sector’s use of technology, big data and AI, and identified this as a key research priority. They have spoken publicly about how the use of big data and algorithmic approaches could raise ethical issues, including concerns of algorithmic bias, and committed to further work to investigate issues in financial markets and present strategies for reducing potential harm.
The FCA’s joint survey with the Bank of England of the use of ML by financial institutions demonstrates their focus on this area. Following this study, they have established a public-private working group on AI to further address some of the issues.
The FCA sees its role to support the safe, beneficial, ethical and resilient deployment of these technologies across the UK financial sector. It acknowledges that firms are best placed to make decisions on which technologies to use and how to integrate them into their business, but that regulators will seek to ensure that firms identify, understand and manage the risks surrounding the use of new technologies, and apply the existing regulatory framework in a way that supports good outcomes for consumers.
As algorithmic decision-making grows, we expect to see similar responses from sector bodies in areas where high stakes decisions are being made about people’s lives. This might involve developing technical standards on how these tools can be assessed for fairness and appropriate routes for challenge and redress for individuals. We believe there is a role for support from both the EHRC, within their regulatory remit, to work with other regulators, as well as CDEI for advice and coordination.
This demonstrates the need for regulators to be sufficiently resourced to deal with equality issues related to the use of AI and data-driven technology in their sectors. It also raises the question of how the equality legislation is applied, regardless of the use of algorithms. This concern was also raised by the Women and Equalities Committee in their report “Enforcing the Equality Act: the law and the role of the Equality and Human Rights”, which stated:
As public bodies all enforcement bodies should be using their powers to secure compliance with the Equality Act 2010 in the areas for which they are responsible. Such bodies are far better placed than the Equality and Human Rights Commission could ever be to combat the kind of routine, systemic, discrimination matters where the legal requirements are clear and employers, service providers and public authorities are simply ignoring them because there is no realistic expectation of sanction.[footnote 186]
Consumer facing regulators such as the FCA, Ofgem and CMA also need to ensure fair treatment for vulnerable customers within their remit. While not an issue of discrimination, regulators set out guidelines for unfair treatment and monitor outcomes for this group. This regulatory activity is conducted separately for each sector, and there is scope for greater collaboration between enforcement bodies to share best practice and develop guidance, as well as being sufficiently skilled and resourced to carry out this work. CDEI can play a key role in providing advice to regulators as well as coordinating activities.
Recommendation to regulators
Recommendation 13: Regulators should consider algorithmic discrimination in their supervision and enforcement activities, as part of their responsibilities under the Public Sector Equality Duty.
8.5 Regulatory tools
Beyond enforcement and guidance, there are a range of tools that can help organisations to meet their regulatory requirements. These range from more proactive supervision models to methods that assure whether organisations have compliant processes and suitably skilled staff. All of these complementary tools should be considered by regulators and industry as they attempt to address algorithmic bias.
Regulatory sandboxes
A regulatory sandbox is a differentiated regulatory approach where a regulator provides more direct supervision for new products and services in a controlled environment. This supervision can range from advice whether new practices are compliant, through to limited exemptions from existing regulatory requirements. A number of regulators currently offer sandbox-based support for their sector, such as the FCA, Ofgem and the ICO.
The main focus of these initiatives is to help organisations understand how they can operate effectively within regulatory frameworks, and help regulators understand how innovative products and services interact with existing regulations. However, this service is most useful to those organisations adopting new business models or innovative approaches to persistent problems that may not fit existing regulations. Examples include new applications of blockchain technology in the FCA sandbox, peer-to-peer energy trading in the Ofgem sandbox, and the use of health and social care data to reduce violence in London in the ICO sandbox.
Addressing algorithmic bias is an important area of regulatory complexity where closer regulatory supervision may be helpful, particularly when new innovations are being adopted that do not easily fit the existing regulatory model.
Regulators with existing sandboxes should consider applications where algorithmic bias is a serious risk, potentially with additional engagement from the EHRC. Regulators in sectors that are seeing accelerated deployment of algorithmic decision-making could consider the regulatory sandbox approach to provide greater support and supervision for innovations that may need new ways of addressing algorithmic bias.
Impact assessments
In the UK, organisations are already required to produce Data Protection Impact Assessments (DPIAs) when processing personal data that is high risk to individual rights and freedoms. These assessments must consider ‘risks to the rights and freedoms of natural persons’ more generally including the ‘impact on society as a whole’.[footnote 187] As a consequence, issues like discrimination may be considered within the remit of data protection impact assessments. However our sector work suggests that in practice, bias and discrimination are not often considered within DPIAs.
Public sector organisations are also required to have due regard to a number of equality considerations when exercising their functions, which are focused on addressing the obligations organisations have under the Equality Act 2010.[footnote 188] Equality Impact Assessments are often carried out by public sector organisations prior to implementing a policy, ascertaining its potential impact on equality. Though not required by law, they are considered good practice as a way of facilitating and evidencing compliance with the Public Sector Equality Duty. There have been efforts to extend the role of Equality Impact Assessments more broadly to assess the risks to fairness raised by AI,[footnote 189] particularly in areas like recruiting.[footnote 190]
Algorithmic bias and discrimination should be incorporated into existing Equality and Data Protection Impact Assessments as part of their internal governance and quality assurance processes. However, our research has indicated that there are a variety of challenges with using impact assessments for addressing algorithmic bias as a regulatory approach. There is limited evidence regarding the effectiveness of impact assessments for providing useful course correction in the development and implementation of new technologies. While the impact assessment process can usefully uncover and resolve compliance issues throughout the development and use of algorithms, we found that in practice[footnote 191] impact assessments are usually treated as a static document, completed either at the very beginning or very end of a development process and therefore do not capture the dynamic nature of machine learning algorithms, which is where algorithmic bias issues are likely to occur. It is therefore hard to regulate only against an impact assessment as it only shows one point in time; they should be seen as one tool complemented by others.
There have also been efforts to combine equality and data protection concerns into a combined Algorithmic Impact Assessment[footnote 192] or Integrated Impact Assessment.[footnote 193] This could be an effective way to remove duplication and support a more consistent way of managing the regulatory and ethical risks raised by these technologies, including fairness. It may also help to highlight to regulators and organisations any tensions between different aspects of current law or guidance.
Audit and certification
One of the frequently cited challenges with the governance of algorithmic decision-making is around how organisations demonstrate compliance with equality legislation. For individuals who are the subject of algorithmic decision-making, the systems can appear opaque and commentators often refer to fears around the risk of “black-boxes” that hide the variables making the decisions. These concerns have led to calls for ways to assure that algorithmic systems have met a particular standard of fairness. These calls are often framed in terms of auditing, certification or impact assessments, which could also be used to assess other measures of algorithmic appropriateness, such as privacy or safety.
In algorithmic bias, this lack of explainability also raises challenges for the burden of proof. In discrimination cases, the Equality Act (Section 136) reverses the burden of proof, meaning that if outcomes data suggest algorithmic discrimination has occurred, courts will assume this has occurred, unless the accused discriminating organisation can prove otherwise. That is, it is not enough for an organisation to say that it does not believe the discrimination has occurred, it needs to explicitly demonstrate that it doesn’t. It is therefore essential for organisations to know what would constitute a proportionate level of proof that their AI systems are not unintentionally discriminating against protected groups.[footnote 194]
There are many contexts where organisations are required to meet standards or regulations, including health and safety, cyber security and financial standards. Each of these systems have evolved into ecosystems of services that allow organisations to prove to themselves, their customers and regulators, that they have met the standard. These ecosystems include auditing, professional accreditation, and product certification.
There are some parts of the ‘AI assurance’ ecosystem that are starting to emerge, such as firms offering ‘AI ethics’ consultancy and calls for ‘AI auditing’ or ‘AI certification’. However, these efforts tend to be focused on data protection and accuracy, rather than fairness and discrimination.
The ICO has recently published “Guidance on AI and data protection”, which sets out a set of key considerations for development of an AI system. It is focused largely on compliance with data protection principles, but it also touches on the areas of data protection that relate to discrimination, including discussion on the legal basis upon which to collect sensitive data for testing for bias. However, this guidance does not directly address compliance with equality law, including the lawfulness of mitigation. The ICO has also announced a process for assessing GDPR certification schemes[footnote 195] which could be used to show that algorithmic decision-making is GDPR compliant. These steps reflect real progress in the governance of algorithms, but algorithmic bias and discrimination would inevitably be a secondary concern in a data protection centred framework.
ICO’s Guidance on AI and Data Protection
The ICO published its guidance on AI and data protection in July 2020. This guidance is aimed at two audiences:
-
those with a compliance focus, such as data protection officers (DPOs), general counsel, risk managers, senior management and the ICO’s own auditors; and
-
technology specialists, including machine learning experts, data scientists, software developers and engineers, and cybersecurity and IT risk managers.
This guidance does not provide ethical or design principles for the use of AI, but corresponds to application of data protection principles.
There is currently no equivalent assurance ecosystem for bias and discrimination in algorithmic decision-making. We see this as a gap that will need to be filled over time, but will require increasing standardisation and guidance in the steps to prevent, measure and mitigate algorithmic bias.
In the US, the National Institute of Standards and Technology (NIST), a non-regulatory agency of the United States Department of Commerce, provides a model for how external auditing of algorithms could emerge. The NIST developed the Facial Recognition Vendor Tests which requested access to commonly used facial recognition algorithms and to then test them under ‘black box’ conditions, by subjecting them all to the same set of validated test images. It initially started these efforts by benchmarking false positive and false negative rates of these algorithms, allowing them to be compared based on their accuracy.
In 2019 this test was extended to examine racial bias, and found that many of these algorithms had much higher error rates, particularly false positives for women and minority ethnic groups. It also found that some algorithms had much lower demographic bias, and were often the algorithms that were the most accurate in general. This analysis has allowed benchmarking and standards based on accuracy to evolve into performance comparisons of algorithmic bias.
Importantly for this role, NIST is seen as a trusted, independent, third party standards body by algorithm developers. However, this function does not necessarily need to be conducted by the government or regulators. Given sufficient expertise and commonly agreed standards, testing and certification against these standards could just as easily be provided by industry bodies or trusted intermediaries.
As well as testing and certification of algorithmic systems themselves, there is a need for good practice standards for organisations and individuals developing these systems, and a relevant ecosystem of training and certification. This ecosystem of private or third sector services to support organisations to address algorithmic bias should be encouraged and is a growth opportunity for the UK. Professional services are a strong and growing area of the UK economy, including those providing audit and related professional services in a number of areas. Many companies are already looking at services that they can provide to help others build fair algorithms. By showing leadership in this area the UK can both ensure fairness for UK citizens, but also unlock an opportunity for growth.
Recommendations to regulators
Recommendation 14: Regulators should develop compliance and enforcement tools to address algorithmic bias, such as impact assessments, audit standards, certification and/or regulatory sandboxes.
Advice to Industry
Industry bodies and standards organisations should develop the ecosystem of tools and services to enable organisations to address algorithmic bias, including sector-specific standards, auditing and certification services for both algorithmic systems and the organisations and developers who create them.
Regulatory coordination and alignment
Algorithmic bias is likely to grow in importance, and this report shows that regulators will need to update regulatory guidance and enforcement to respond to this challenge. Given the overlapping nature of equality, data protection and sector-specific regulations, there is a risk that this could lead to a more fragmented and complex environment. Regulators will need to coordinate their efforts to support regulated organisations through guidance and enforcement tools. This will need to go further than cross-regulator forums, through to practical collaboration in their supervision and enforcement activities. Ideally, regulators should avoid duplicative compliance efforts by aligning regulatory requirements, or jointly issue guidance. Regulators should also pursue joint enforcement activities, where sector regulators pursue non-compliant organisations in their sector, with the support of cross-cutting regulators like the EHRC[footnote 197] and ICO.
This will require additional dedicated work to coordinate efforts between regulators, who have traditionally focused on their regulatory responsibility. However, there has been an increasing effort for regulatory collaboration in other areas such as the UK Regulators Network which has more formally brought together economic sector regulators for collaboration and joint projects. Similar efforts to collaborate should be explored by sector regulators when addressing algorithmic bias.
Recommendations to regulators
Recommendation 15: Regulators should coordinate their compliance and enforcement efforts to address algorithmic bias, aligning standards and tools where possible. This could include jointly issued guidance, collaboration in regulatory sandboxes, and joint investigations.
Future CDEI work
-
CDEI plans to grow its ability to provide expert advice and support to regulators, in line with our existing terms of reference. This will include supporting regulators to coordinate efforts to address algorithmic bias and to share best practice.
-
CDEI will monitor the development of algorithmic decision-making and the extent to which new forms of discrimination or bias emerge. This will include referring issues to relevant regulators, and working with government if issues are not covered by existing laws and regulations.
9. Transparency in the public sector
Summary
Overview of findings:
-
Making decisions about individuals is a core responsibility of many parts of the public sector, and there is increasing recognition of the opportunities offered through the use of data and algorithms in decision-making.
-
The use of technology should never reduce real or perceived accountability of public institutions to citizens. In fact, it offers opportunities to improve accountability and transparency, especially where algorithms have significant effects on significant decisions about individuals.
-
A range of transparency measures already exist around current public sector decision-making processes. There is a window of opportunity to ensure that we get transparency right for algorithmic decision-making as adoption starts to increase.
-
The supply chain that delivers an algorithmic decision-making tool will often include one or more suppliers external to the public body ultimately responsible for the decision-making itself. While the ultimate accountability for fair decision-making always sits with the public body, there is limited maturity or consistency in contractual mechanisms to place responsibilities in the right place in the supply chain.
Recommendations to government:
-
Recommendation 16: Government should place a mandatory transparency obligation on all public sector organisations using algorithms that have a significant influence on significant decisions affecting individuals. Government should conduct a project to scope this obligation more precisely, and to pilot an approach to implement it, but it should require the proactive publication of information on how the decision to use an algorithm was made, the type of algorithm, how it is used in the overall decision-making process, and steps taken to ensure fair treatment of individuals.
-
Recommendation 17: Cabinet Office and the Crown Commercial Service should update model contracts and framework agreements for public sector procurement to incorporate a set of minimum standards around ethical use of AI, with particular focus on expected levels transparency and explainability, and ongoing testing for fairness.
Advice to industry:
- Industry should follow existing public sector guidance on transparency, principally within the Understanding AI Ethics and Safety guidance developed by the Office for AI, the Alan Turing Institute and the Government Digital Service, which sets out a process-based governance framework for responsible AI innovation projects in the UK public sector
9.1 Identifying the issue
Why the public sector?
Ensuring fairness in how the public sector uses algorithms in decision-making is crucial. The public sector makes many of the highest impact decisions affecting individuals, for example related to individual liberty or entitlement to essential public services. There is also precedent of failures in large scale, but not necessarily algorithmic, decision-making processes causing impacts on a large number of individuals, for example fitness-to-work assessments for disability benefits[footnote 198] or immigration case-working.[footnote 199] These examples demonstrate the significant impact that decisions made at scale by public sector organisations can have if they go wrong and why we should expect the highest standards of transparency and accountability.
The lines of accountability are different between the public and private sectors. Democratically-elected governments bear special duties of accountability to citizens.[footnote 200] We expect the public sector to be able to justify and evidence its decisions. Moreover, an individual has the option to opt-out of using a commercial service whose approach to data they do not agree with, but they do not have the same option with essential services provided by the state.
There are already specific transparency obligations and measures relevant to fair decision-making in the public sector in the UK, for example:
- Publication of internal process documentation for large scale decision-making processes such as those within Home Office[footnote 201], Department of Work and Pensions[footnote 202] and HMRC.[footnote 203]
-
The Freedom of Information Act offers citizens the ability to access a wide range of information about the internal workings of public sector organisations.
-
Subject Access Requests under the Data Protection Act enable individuals to request and challenge information held about them (also applicable to the private sector). Some organisations publish Personal Information Charters describing how they manage personal information in line with the Data Protection Act.[footnote 204]
-
Publication of Equality Impact Assessments for decision-making practices (which is not strictly required by the Equality Act 2010, but is often conducted as part of organisations demonstrating compliance with the Public Sector Equality Duty).
-
Various other existing public sector transparency policies enable an understanding of some of the wider structures around decision-making, for example the publication of spending[footnote 205] and workforce data.[footnote 206]
-
Parliamentary questions and other representation by MPs.
-
Disclosure related to legal challenges to decision-making, e.g. judicial review.
-
Inquiries and investigations by some statutory bodies and commissioners on behalf of individuals, e.g. the EHRC.
There is also an opportunity for the government to set an example for the highest levels of transparency. Government can do this through the strong levers it has at its disposal to affect behaviour, either through direct management control over the use of algorithmic decision-making, or strategic oversight of arms-length delivery bodies, for example in policing or the NHS.
Setting high ethical standards in how it manages private sector service delivery also offers a potential lever for strong standards of transparency in the public sector to raise standards in the private sector. For example, in a different context, mandation in 2016 of Cyber Essentials certification for all new public sector contracts not only improved public sector cyber security, but also cyber security in a marketplace of service providers who supply both public and private sector organisations.[footnote 207]
The public is right to expect services to be delivered responsibly and ethically, regardless of how they are being delivered, or who is providing those services. - The Committee on Standards in Public life (2018)[footnote 208]
Public bodies have a duty to use public money responsibly[footnote 209] and in a way that is “conducive to efficiency”. Given that a potential benefit of the use of algorithms to support decision-making, if done well, is optimising the deployment of scarce resources,[footnote 210] it could be argued that the public sector has a responsibility to trial new technological approaches. Nonetheless, this must be done in a way that manages potential risks, builds clear evidence of impact, and upholds the highest standards of transparency and accountability.
What is the problem?
Currently, it is difficult to find out what algorithmic systems the UK public sector is using and where.[footnote 211] This is a problem because it makes it impossible to get a true sense of the scale of algorithmic adoption in the UK public sector and therefore to understand the potential harms, risks and opportunities with regard to public sector innovation.
The recent report by the Committee on Standards in Public Life on ‘AI and Public Standards’ noted that adoption of AI in the UK public sector remains limited, with most examples being under development or at a proof-of-concept stage.[footnote 212] This is consistent with what CDEI has observed in the sectors we have looked at in this Review. Nonetheless, these varying accounts could lead to a perception of intended opacity from government by citizens.
Government is increasingly automating itself with the use of data and new technology tools, including AI. Evidence shows that the human rights of the poorest and most vulnerable are especially at risk in such contexts. A major issue with the development of new technologies by the UK government is a lack of transparency. - Philip Alston, The UN Special Rapporteur on Extreme Poverty and Human Rights [footnote 213]
What is the value of transparency?
The case for transparency has been made in multiple contexts, including for government policy[footnote 214] and algorithms.[footnote 215] Yet the term ‘transparency’ can be ambiguous, mean different things in different contexts, and should not in itself be considered a universal good.[footnote 216] For example, publishing all details of an algorithm could lead to the gaming of rules through people understanding how the algorithm works or disincentivise the development of relevant intellectual property. Another risk is that actors with misaligned interests could abuse transparency as a way of sharing selective pieces of information to serve communication objectives or purposefully manipulating an audience. However, we should be able to mitigate these risks if we consider transparency within the context of decisions being made by the public sector and if it is not seen as an end in itself, but alongside other principles of good governance[footnote 217] including accountability.
We should also not assume that greater transparency from public sector organisations will inevitably lead to greater trust in the public sector. In fact, just providing information, if not intelligible to the public could fail to inform the public and even foster concern. Baroness Onora O’Neill established the principle of “intelligent accountability”[footnote 218] in her 2002 Reith Lecture and has since spoken of the need for “intelligent transparency” summarised below.
According to Onora O’Neill’s principle of “intelligent transparency” information should be:
-
Accessible: interested people should be able to find it easily.
-
Intelligible: they should be able to understand it.
-
Useable: it should address their concerns.
-
Assessable: if requested, the basis for any claims should be available.
These are useful requirements to bear in mind when considering what type of transparency is desirable given that simply providing more information just for the sake of it will not automatically build trust.
Trust requires an intelligent judgement of trustworthiness. So those who want others’ trust have to do two things. First, they have to be trustworthy, which requires competence, honesty and reliability. Second, they have to provide intelligible evidence that they are trustworthy, enabling others to judge intelligently where they should place or refuse their trust. - Onora O’Neill, ‘How to trust intelligently’[footnote 220]
Sir David Spiegelhalter has built on Onora O’Neill’s work by articulating the need to be able to interrogate the trustworthiness of claims made about an algorithm, and those made by an algorithm. This led him to produce the following set of questions that we should expect to be able to answer about an algorithm:[footnote 221]
-
Is it any good (when tried in new parts of the real world)?
-
Would something simpler, and more transparent and robust, be just as good?
-
Could I explain how it works (in general) to anyone who is interested?
-
Could I explain to an individual how it reached its conclusion in their particular case?
-
Does it know when it is on shaky ground, and can it acknowledge uncertainty?
-
Do people use it appropriately, with the right level of scepticism?
-
Does it actually help in practice?
These questions are a helpful starting point for public sector organisations when evaluating an algorithm they are developing or using and considering the sort of information they need to know and share in order to ensure it is meaningful in the public’s eyes.
9.2 Delivering public sector transparency
Based on the discussion above, we believe that more concrete action is needed to ensure a consistent standard of transparency across the public sector related to the use of algorithmic decision-making.
In this section, we assess in some detail how this could work, the key conclusion of which is the following:
Recommendations to government
Recommendation 16: Government should place a mandatory transparency obligation on all public sector organisations using algorithms that have a significant influence on significant decisions affecting individuals. Government should conduct a project to scope this obligation more precisely, and to pilot an approach to implement it, but it should require the proactive publication of information on how the decision to use an algorithm was made, the type of algorithm, how it is used in the overall decision-making process, and steps taken to ensure fair treatment of individuals.
Below we discuss in more detail where this recommendation comes from. Further work is needed to precisely scope this, and define what is meant by transparency. But rooting this thinking in O’Neill’s principle of “intelligent transparency” and Spiegelhalter’s questions of what we should expect from a trustworthy algorithm provide a solid basis to ensure there is careful thinking about the algorithm itself and the information that is published.
What is in scope?
The use of the word significant clearly requires more careful definition:
-
Significant influence means that the output of the machine learning model is likely to meaningful affect the overall decision made about an individual, i.e. not just providing automation of a routine process but informing decision-making in a more meaningful way e.g. by assessing risk or categorising applications in a way that influences the outcome.
-
Significant decision means that the decision has a direct impact on the life of an individual or group of individuals. In the Data Protection Act 2018, a decision is a “significant decision” if it produces an adverse legal effect concerning an individual or otherwise significantly affects them. Although according to the Data Protection Act this applies specifically to fully automated significant decisions, we would suggest a similar interpretation here which includes decisions made with human input.
Some potential examples of algorithmic decision-making that would be in or out of scope are shown in Figure 5.
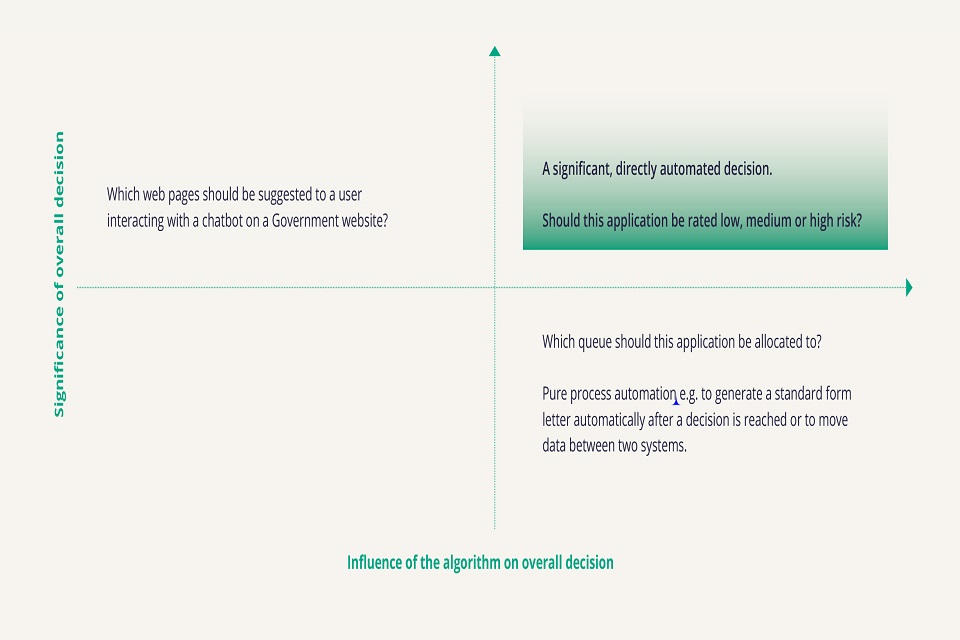
Our scope is defined by the significance of the “effect” of the algorithm on the overall outcome of a decision-making process, and the significance of the “impact” of the overall decision on the life of the person(s) affected. A low impact decision can involve an algorithm which has a significant effect on the overall outcome of the decision-making process. An example of this would be a chatbot suggesting a webpage to a user on a government website. A decision which is only marginally affected by an algorithm can have a high impact on a person’s life. For example, assigning applications to a given queue, pure process automation (generating a standard form letter after a decision has been made), or moving data between two systems. Finally, a decision can have a high impact on a person’s life and be significantly affected by an algorithm during the decision-making process. An example of this would be risk allocation - high, medium or low. Decisions that fall into this category are in scope for the purposes of this review.
Figure 5: Decisions can be differentiated by the influence of algorithms over the decision, and the significance of the overall decision
When defining impactful or significant decisions, due consideration should be paid to where decisions relate to potentially sensitive areas of government policy, or where there may be low levels of trust in public sector institutions. These could include social care, criminal justice or benefits allocation.
The definition of public sector in this context could be sensibly aligned with that used in the Equality Act 2010 or Freedom of Information Act 2000.
Some exemptions to this general scoping statement will clearly be needed, which will require careful consideration. Potential reasons for exemption are:
-
Transparency risks compromising outcomes: e.g. Where publication of too many details could undermine the use of the algorithm by enabling malicious outsiders to game it, such as in a fraud detection use case.
-
Intellectual Property: In some cases the full details of an algorithm or model will be proprietary to an organisation that is selling it. We believe that it is possible to achieve a balance, and achieve a level of transparency that is compatible with intellectual property concerns of suppliers to the public sector. This is already achieved in other areas where suppliers accept standard terms around public sector spending data etc. There is some detailed thinking around this area that needs to be worked through as part of government’s detailed design of these transparency processes.
-
National Security and Defence: e.g. there may be occasional cases where the existence of work in this area cannot be placed in the public domain.
In general, our view is that risks in areas 1 and 2 should be managed by being careful about the actual information that is being published (i.e. keeping details at a sufficiently high level), while area 3 is likely to require a more general exemption scoped under the same principles as those under Freedom of Information legislation.
What information should be published?
Defining the precise details of what should be published is a complex task, and will require extensive further consultation across government and elsewhere. This section sets out a proposed draft scope, which will need to be refined as the government considers its response to this recommendation.
A number of views on this have been expressed previously. For example, the Committee on Standards in Public Life report defines openness, which they use as interchangeable with transparency in their report, as: “fundamental information about the purpose of the technology, how it is being used, and how it affects the lives of citizens must be disclosed to the public.”[footnote 222]
As a starting point, we would anticipate a mandatory transparency publication to include:
A. Overall details of the decision-making process in which an algorithm/model is used.
B. A description of how the algorithm/model is used within this process (including how humans provide oversight of decisions and the overall operation of the decision-making process).
C. An overview of the algorithm/model itself and how it was developed, covering for example:
-
The type of machine learning technique used to generate the model.
-
A description of the data on which it was trained, an assessment of the known limitations of the data and any steps taken to address or mitigate these.
-
The steps taken to consider and monitor fairness.
D. An explanation of the rationale for why the overall decision-making process was designed in this way, including impact assessments covering data protection, equalities, human rights, carried out in line with relevant legislation. It is important to emphasise that this cannot be limited to the detailed design of the algorithm itself, but also needs to consider the impact of automation within the overall process, circumstances where the algorithm isn’t applicable, and indeed whether the use of an algorithm is appropriate at all in the context.
Much of this is already common practice for public sector decision-making. However, identifying the right level of information on the algorithm is the most novel aspect. There are examples elsewhere that can help guide this. For example:
- Google’s model cards[footnote 223] aim to provide an explanation of how a model works to experts and non-experts alike. The model cards can assist in exploring limitations and bias risk, by asking questions such as: ‘does a model perform consistently across a diverse range of people, or does it vary in unintended ways as characteristics like skin colour or region change?’
- The Government of Canada’s Algorithmic Impact Assessment which is a questionnaire designed to help organisations assess and mitigate the risks associated with deploying an automated decision system as part of wider efforts to ensure the responsible use of AI.[footnote 224]
- New York City Council passed the algorithmic accountability law in 2019 which has resulted in the setting up of a task force that will monitor the fairness and validity of algorithms used by municipal agencies, whilst ensuring they are transparent and accountable to the public.[footnote 225]
- The United Kingdom’s Departmental Returns prepared by different parts of government as part of the MacPherson review of government modelling in 2013.[footnote 226]
The Office for AI, Turing Institute and Government Digital Service’s Understanding AI Ethics and Safety guidance[footnote 227] have set out a process-based governance framework for responsible AI innovation projects in the UK public sector. Within this guidance document they provide a definition of transparency within AI ethics as including both the interpretability of an AI system and the justifiability of its processes and outcome. This Guidance should be the starting point, along with the ideas and other examples set out in this report, for the UK government when considering precisely what set of information makes sense in the UK public sector. CDEI is happy to provide independent input into this work if required.
How does this fit with existing transparency measures?
We listed above a variety of existing public sector transparency measures related to decision-making. A theme of public commentary on the use of algorithms is that they can potentially undermine this transparency and accountability. Government should seek to demonstrate that this is not the case.
In fact, existing FOI and DPA obligations arguably already give individuals the right to request access to all of the information listed in the scope above. Moreover, initiatives like the local government transparency code[footnote 228] which sets out the minimum data that local authorities should be publishing, the frequency it should be published and how it should be published are good examples to build on. In some regards, we are not proposing more transparency but more effective transparency. Whilst there are obligations for proactive disclosure under FOI and the DPA, these are not always effective as a transparency tool in practice and are often more reactive. By making publication of information a truly proactive process it can help government:
-
Build in expectations of what will eventually have to be published at the early stages of projects.
-
Structure releases in a consistent way which hopefully helps external groups (e.g. journalists, academia and civil society) engage with the data being published in an effective way, i.e. over time fewer genuine misunderstandings in the communication.
-
Manage the overhead of responding to large numbers of similar reactive requests.
Managing the process of transparency
The House of Lords Science and Technology Select Committee and the Law Society have both recently recommended that parts of the public sector should maintain a register of algorithms in development or use.
…the Government should produce, publish, and maintain a list of where algorithms with significant impacts are being used within Central Government, along with projects underway or planned for public service algorithms, to aid not just private sector involvement but also transparency. - House of Lords Science and Technology Select Committee[footnote 229]
A National Register of Algorithmic Systems should be created as a crucial initial scaffold for further openness, cross-sector learning and scrutiny. - The Law Society, ‘Algorithms in the Criminal Justice System’[footnote 230]
CDEI agrees that there are some significant advantages both to government and citizens in some central coordination around this transparency. For example it would enable easier comparisons across different organisations, e.g. by promoting consistent style of transparency. Moreover, there are delivery and innovation benefits in allowing public sector organisations themselves to see what their peers are doing.
However, implementing this transparency process in a coordinated way across the entire public sector is a challenging task, much greater in extent than either of the proposals quoted above (e.g. the use by local government in social care settings that we discussed in Chapter 6 would not be included in either of those examples).
There are a number of comparators to consider in levels of coordination:
-
Centralised for central government only: GDS Spend Controls
-
Devolved to individual organisations: Publication of transparency data
-
Central publication across public and private sector: Gender pay gap reporting[footnote 231]
We suspect that there is a sensible middle ground in this case. The complexities of coordinating such a register across the entire public sector would be high, and subtle differences in what is published in transparency data might well apply in different sectors. We therefore conclude that the starting point here is to set an overall transparency obligation, and for the government to decide on the best way to coordinate this as it considers implementation.
The natural approach to such an implementation is to pilot in a specific part of the public sector. For example, it could be done for services run directly by central government departments (or some subset of them), making use of existing coordination mechanisms managed by the Government Digital Service.
It is likely that a collection of sector-specific registers might be the best approach, with any public sector organisations out of scope of any sector register remaining responsible for publishing equivalent transparency data themselves.
The relationship between transparency and explainability
To uphold accountability, public sector organisations should be able to provide some kind of explanation of how an algorithm operates and reaches its conclusion. As David Spiegelhalter says “a trustworthy algorithm should be able to ‘show its working’ to those who want to understand how it came to its conclusions”.[footnote 232] Crucially, the working needs to be intelligible to a non-expert audience and therefore focusing on publishing the algorithm’s source code or technical details as a demonstration of transparency can be a red herring.
An area of explainability which previous reports and research have focused on is the black box. Indeed, the House of Lords Select Committee on AI expressed that it was unacceptable to deploy any AI system that could have a substantial impact on an individuals’ life, unless it can generate “a full and satisfactory explanation” for the decisions it will take and that this was extremely difficult to do with a black box algorithm.[footnote 233] In the case of many key administrative decisions, often based on well structured data, there may not be a need to develop highly sophisticated, black box algorithms to inform decisions; often simpler statistical techniques may perform as well. Where an algorithm is proposed that does have limitations in its explainability (i.e. a black box) the organisation should be able to satisfactorily answer Spiegelhalter’s questions in particular around whether something simpler would be just as good and whether you can explain how it works and how it reaches its conclusion.
As mentioned in Chapter 4 the ICO and ATI have jointly developed guidance for organisations on how to explain decisions made with AI. The guidance offers several types of examples of explanations for different contexts and decisions, along with advice on the practicalities of explaining these decisions to internal teams and individuals. Whilst the guidance is not directed exclusively at the public sector, it contains valuable information for public sector organisations who are using AI to make decisions. There is also the potential for public sector organisations to publish case studies and examples of where they are applying the guidance to explain decisions made with AI.
Ultimately, the algorithmic element of the decision-making process should not be so unexplainable and untransparent that it undermines the extent to which the public sector organisation is able to publish intelligent and intelligible information about the whole decision-making process.
9.3 Public sector procurement
Introduction
The development and delivery of an algorithmic decision-making tool will often include one or more suppliers, whether acting as technology suppliers or business process outsourcing providers. Even where development and delivery of an algorithmic decision-making tool is purely internal, there is always reliance on externally developed tools and libraries, e.g. open source machine learning libraries in Python or R.
In such supply chain models, the ultimate accountability for good decision-making always sits with the public body. Ministers are still held to account by Parliament and the public for the overall quality and fairness of decisions made (along with locally elected councillors or Police and Crime Commissioners where relevant). The Committee on Standards in Public Life noted in 2018 that the public is right to expect services to be delivered responsibly and ethically, regardless of how they are being delivered, or who is providing those services.[footnote 234]
The transparency mechanisms discussed in the section above form part of this overall accountability, and therefore need to be practical in all of these different potential supply chain models.
Supply chain models
Some examples of possible models for outsourcing a decision-making process are as follows.
Public Body in house | IT partner | Business process outsourcing | |
Policy and accountability for decision-making | Public body | Public body | Public body |
---|---|---|---|
Operational decision-making | Public body | Public body | Supplier |
Model and tool development | Public body | Supplier | Supplier (or subcontractor) |
Underlying algorithms and libraries | Mostly open source, potentially some 3rd party proprietary | Mostly open source, potentially some 3rd party proprietary | Mostly open source, potentially some 3rd party proprietary |
Training data | Public body and/or 3rd party | Public body and/or 3rd party | Public body and/or 3rd party |
Many of the issues around defining and managing such a supply chain in a sensible way are common with any government procurement of services dependent on technology. But the source and ownership of the data on which a machine learning model is trained can make the interdependency between customer and supplier more complex in this context than in many others. Where a model is trained on data provided by the customer, it’s not straightforward to flow down requirements on fairness in a supplier contract, as the ability to meet those requirements will be dependent in part on the customer’s data.
This is not just a public sector issue. In the wider marketplace, the ecosystem around contracting for AI is not fully developed. There is a natural desire from those at the top of the tree to push some of the responsibilities for ethics and legal compliance of AI systems down their supply chain. This is common practice in a number of other areas, e.g. TUPE regulations create obligations on organisations involved in the transfer of services between suppliers, related to the employees providing those services. There are commonly understood standard clauses included in contracts that make it clear where those any financial liabilities associated with this sit. A similar notion of commonly understood contractual wording does not exist in this case.
There are pros and cons of this position. On the positive side, it ensures that organisations with responsibility for the overall decision-making process cannot attempt to pass this off onto their suppliers without properly considering the end-to-end picture. But conversely, it means that there may be limited commercial incentive for suppliers further down the supply chain to really focus on how their products and services can support ethical and legally compliant practices.
Addressing the issue
The Office for AI, working in partnership with the World Economic Forum, has developed detailed draft guidance[footnote 235] on effective procurement of AI in the public sector, which includes useful consideration of how ethics issues can be handled in procurement. This is a helpful step forward, and it is encouraging that the UK government is taking a leading role in getting this right globally.
The recent Committee on Standards in Public Life report on AI and Public Standards[footnote 236] noted that “…firms did not feel that the public sector often had the capability to make their products and services more explainable, but that they were rarely asked to do so by those procuring technology for the public sector.” This guidance aims to help address this, but there is clearly more to do to implement this effectively across the UK public sector.
The guidance as drafted is focused on projects that are primarily focused on buying AI solutions. This is a relevant situation, but as AI increasingly becomes a generic technology present in a whole variety of use cases, much public sector procurement of AI will be implicitly within wider contracts. It is unlikely (and not necessarily desirable) that procurement teams across all areas will focus specifically on AI procurement amongst a range of other guidance and best practice.
Similar issues occur for other common underlying requirements, such as those around data protection, cyber security and open book accounting. Part of the approach taken for these is to include standard terms with model contracts and framework agreements used across the public sector that capture a minimum set of core principles. These can never achieve as much as careful thought about how to contract for the right outcome in a specific context, but help establish a minimum common standard.
A similar approach should be taken for AI ethics. For procurement activity where AI is a specific focus then procurement teams need to be designing specific requirements applicable to the use case, drawing on the Office for AI and World Economic Forum guidelines. But where use of algorithmic decision-making is not specifically expected, but could form part of possible supplier solutions to an output based requirement, a common baseline requirement is needed to give the contracting authority the ability to manage that risk in life.
Given the range of different possible use cases it is difficult to place highly specific requirements in a model contract. The focus should be on enabling the contracting authority to have an appropriate level of oversight on the development and deployment of an algorithmic decision-making tool to oversee whether fairness considerations have been taken into account, along with rights to reject or request changes if they are not.
Helpfully, in central government, and to some extent in the wider public sector, there is a centrally managed set of procurement policies, model contracts and framework agreements which underpin the majority of procurement processes. These are mainly managed by Cabinet Office’s Government Commercial Function (policy and model contracts), and the Crown Commercial Service (framework agreements). Work is already underway by these bodies to incorporate findings from the Office for AI/WEF procurement guidelines into AI-specific procurement activities, and the new AI framework RM6200.[footnote 237] However, there is scope to go further than this to cover all procurement activity which could potentially result in purchasing an AI-reliant service:
Recommendations to government
Recommendation 17: Cabinet Office and the Crown Commercial Service should update model contracts and framework agreements for public sector procurement to incorporate a set of minimum standards around ethical use of AI, with particular focus on expected levels of transparency and explainability, and ongoing testing for fairness.
In developing the details of such terms, the government will need to consult with the marketplace to ensure that eventual terms are commercially palatable. The intention of this recommendation is to find a balance that gives commercial mechanisms for public bodies to manage concerns about bias in algorithmic decision-making (and indeed other ethical concerns around AI), but does not impose a burden on the market that is disproportionate to the risk or to other common terms within public sector procurement.
In developing such standard terms, the government may want to draw on support from the Office for AI and CDEI.
10. Next steps and future challenges
This review has considered a complex and rapidly evolving field. Recognising the breadth of the challenge, we have focused heavily on surveying the maturity of the landscape, identifying the gaps, and setting out some concrete next steps. There is plenty to do across industry, regulators and government to manage the risks and maximise the benefits of algorithmic decision-making.
Some of the next steps fall within CDEI’s remit, and we are keen to help industry, regulators and government in taking forward the practical delivery work to address the issues we have identified and future challenges which may arise.
Government, industry bodies and regulators need to give more help to organisations building and deploying algorithmic decision-making tools on how to interpret the Equality Act in this context. Drawing on the understanding built up through this review, CDEI is happy to support several aspects of the work in this space by, for example:
-
Supporting the development of any guidance on the application of the Equality Act to algorithmic decision-making.
-
Supporting government on developing guidance on collection and use of protected characteristics to meet responsibilities under the Equality Act, and in identifying any potential future need for a change in the law, with an intent to reduce barriers to innovation.
-
Drawing on the draft technical standards work produced in the course of this review and other inputs to help industry bodies, sector regulators and government departments in defining norms for bias detection and mitigation.
-
Supporting the Government Digital Service as they seek to scope and pilot an approach to transparency.
-
Growing our ability to provide expert advice and support to regulators, in line with our terms of reference, including supporting regulators to coordinate efforts to address algorithmic bias and to share best practice. As an example, we have been invited to take an observer role on the Financial Conduct Authority and Bank of England’s AI Public Private Forum which will explore means to support the safe adoption of machine learning and artificial intelligence within financial services, with an intent to both support that work, and draw lessons from a relatively mature sector to share with others.
We have noted the need for an ecosystem of skilled professionals and expert supporting services to help organisations in getting fairness right, and provide assurance. Some of the development needs to happen organically, but we believe that action may be needed to catalyse this. CDEI plans to bring together a diverse range of organisations with interest in this area, and identifying what would be needed to foster and develop a strong AI accountability ecosystem in the UK. This is both an opportunity to manage ethical risks for AI in the UK, but also to support innovation in an area where there is potential for UK companies to offer audit services worldwide.
Through the course of the review, a number of public sector organisations have expressed interest in working further with us to apply the general lessons learnt in specific projects. For example, we will be supporting a police force and a local authority as they develop practical governance structures to support responsible and trustworthy data innovation.
Looking across the work listed above, and the future challenges that will undoubtedly arise, we see a key need for national leadership and coordination to ensure continued focus and pace in addressing these challenges across sectors.
Government should be clear on where it wants this coordination to sit. There are a number of possible locations; for example in central government directly, in a regulator or in CDEI. Government should be clear on where responsibilities sit for tracking progress across sectors in this area, and driving the pace of change. As CDEI agrees our future priorities with government, we hope to be able to support them in this area.
This review has been, by necessity, a partial look at a very wide field. Indeed, some of the most prominent concerns around algorithmic bias to have emerged in recent months have unfortunately been outside of our core scope, including facial recognition and the impact of bias within how platforms target content (considered in CDEI’s Review of online targeting).
Our AI Monitoring function will continue to monitor the development of algorithmic decision-making and the extent to which new forms of discrimination or bias emerge. This will include referring issues to relevant regulators, and working with government if issues are not covered by existing regulations.
Experience from this review suggests that many of the steps needed to address the risk of bias overlap with those for tackling other ethical challenges, for example structures for good governance, appropriate data sharing, and explainability of models. We anticipate that we will return to issues of bias, fairness and equality through much of our future work, though likely as one cross-cutting ethical issue in wider projects.
If you are interested in knowing more about the projects listed above, or CDEI’s future work, please get in touch via bias@cdei.gov.uk.
Appendix A
Bias mitigation methods by stage of intervention and notion of fairness. Detailed references for each of these techniques can be found in Faculty’s “Bias identification and mitigation in decision-making algorithms”, published separately.
Pre-processing | In-processing | Post-processing |
Demographic Parity | ||
Data reweighting / resampling: * (Calders, Kamiran, and Pechenizkiy 2009) * (Faisal Kamiran and Calders 2012) Label modification: * (Calders, Kamiran, and Pechenizkiy 2009) * (Faisal Kamiran and Calders 2012) * (Luong, Ruggieri, and Turini 2011) Feature modification: * (Feldman et al. 2015) Optimal clustering / constrained optimisation: * (Zemel et al. 2013) * (Calmon et al. 2017) Auto-encoding: * (Louizos et al. 2016) |
Constrained optimisation: * (Corbett-Davies et al. 2017) * (Agarwal et al. 2018) * (Zafar, Valera, Rodriguez, et al. 2017) Regularisation: * (Kamishima et al. 2012) Naive Bayes/Balance models for each group: * (Calders and Verwer 2010) Naive Bayes/Training via modified labels: * (Calders and Verwer 2010) Tree-based splits adaptation: * (F. Kamiran, Calders, and Pechenizkiy 2010) Adversarial debiasing: * (Zhang et al. 2018) * (Adel et al. 2019) |
Naive Bayes/Modification of model probabilities: * (Calders and Verwer 2010) Tree-based leaves relabelling: * (F. Kamiran, Calders, and Pechenizkiy 2010) Label modification: * (Lohia et al. 2019) * (F. Kamiran, Karim, and Zhang 2012) |
Conditional Demographic Parity | ||
Constrained optimisation: * (Corbett-Davies et al. 2017) Adversarial debiasing: * (Zhang et al. 2018) * (Adel et al. 2019) - by passing cond. variable to adversarial |
||
Equalised Odds | ||
Constrained optimisation: * (Corbett-Davies et al. 2017) (predictive equality) * (Agarwal et al. 2018) * (Zafar, Valera, Gomez Rodriguez, et al. 2017) * (Woodworth et al. 2017) Adversarial debiasing: * (Zhang et al. 2018) * (Adel et al. 2019) |
Decision threshold modification (ROC curve)/ constrained optimisation: * (Hardt, Price, and Srebro 2016; Woodworth et al. 2017) |
|
Calibration | ||
Unconstrained optimisation: * (Corbett-Davies et al. 2017) |
Information Withholding: * (Pleiss et al. 2017) - achieves simultaneously a relaxation of Equalised Odds |
|
Individual Fairness | ||
Optimal clustering / constrained: * (Zemel et al. 2013) |
Constrained optimisation: * (Dwork et al. 2012) * (Biega, Gummadi, and Weikum 2018) |
Label modification: * (Lohia et al. 2019) |
Counterfactual Fairness | ||
Prediction via non-descendants in causal graph: * (Kusner et al. 2017) |
||
Subgroup Fairness | ||
Two-player zero-sum game: * (Kearns et al. 2018) * (Kearns et al. 2019) |
-
Note that Roger Taylor, the chair of the CDEI Board, is also the chair of Ofqual, the English exams regulator. Following the controversy around August 2020 exam results, Roger has stepped away from involvement in any changes made to the final version of the review. CDEI has not had any direct role in assessing Ofqual’s approach, at the time of writing we understand a number of regulators are looking into the issues raised in detail. ↩
-
The Behavioural Insights Team, ‘The perceptions of fairness of algorithms and proxy information in financial services’, 2019 ↩
-
Royal United Services Institute, Briefing Paper: ‘Data Analytics and Algorithmic Bias in Policing’, 2019; and Royal United Services Institute, Occasional Paper: ‘Data Analytics and Algorithms in Policing in England and Wales’, 2019 ↩
-
Lowry, Stella; Macpherson, Gordon; ‘A Blot on the Profession’, British Medical Journal 1988, pp.657–8 ↩
-
For avoidance of confusion, in place of the more neutral meaning often used in machine learning or other scientific literature (e.g. “to discriminate between”) we use “distinguish”. ↩
-
Note that in addition to discrimination the Equality Act also forbids victimisation and harassment, and places a requirement on organisations to make reasonable adjustments for people with disabilities, see Section 8.3 for more details. ↩
-
Equality and Human Rights Commission, ‘Words and terms used in the Equality Act’ ↩
-
Note that public sector bodies in Scotland must address socio-economic inequalities in their decision-making under the Fairer Scotland Duty. ↩
-
ICO, ‘Guide to the General Data Protection Regulation (GDPR) - Principles ↩
-
A comprehensive review of different possibilities is given in, for example, Mehrabi, Ninareh; Morstatter, Fred; Saxena, Nripsuta; Lerman, Kristina; Galstyan, Aram; ‘A Survey on Bias and Fairness in Machine Learning’, 2019; or Chouldechova, Alexandra; Roth, Aaron; ‘The Frontiers of Fairness in Machine Learning’, 2018 ↩
-
There are of course many other sets of ethical principles and frameworks for AI from a variety of organisations, including various non-profit organisations, consultancies and the Council of Europe. ↩
-
Correll, Shelley J., and Stephen Benard. ‘Gender and racial bias in hiring. Memorandum report for University of Pennsylvania’, 2006 ↩
-
Gender Action Portal, ‘Orchestrating Impartiality: The Impact of “Blind” Auditions on Female Musicians’ ↩
-
Nuffield College Oxford, Centre for Social Investigation, ‘New CSI Research reveals high levels of job discrimination faced by ethnic minorities in Britain’, 2019 ↩
-
Applied, ‘ “It’s a pandemic of racism”: the failure of data, implicit bias and systemic discrimiation’, 2020 ↩
-
Trades Union Congress, ‘Disability employment and pay gaps 2018’ ↩
-
Chartered Institute of Personnel and Development, ‘A head for hiring: the behavioural science of recruitment’, 2015 ↩
-
For a comprehensive analysis of different tools and the associated risks see Bogen, Miranda and Aaron Rieke, ‘Help wanted: an examination of hiring algorithms, equity, and bias.’, Upturn, 2018 ↩
-
CDEI’s recent review of online targeting covers this in more detail ↩
-
BBC News, ‘Amazon scrapped ‘sexist AI’ tool’, 2018 ↩
-
HireVue, ‘Train, Validate, Re-Train: How We Build HireVue Assessments Models’, 2018 ↩
-
Quartz, ‘Companies are on the hook if their hiring algorithms are biased’, 2018 ↩
-
BBC News, ‘Amazon scrapped ‘sexist AI’ tool’, 2018 ↩
-
For an detailed report on the challenges and gaps related to auditing AI in recruitment, see the report from the Institute for the Future of Work, ‘Artificial intelligence in hiring: Assessing impacts on equality’, 2020 ↩
-
See: Equality and Human Rights Commission, ‘What equality law means for you as an employer: when you recruit someone to work for you’ ↩
-
Sanchez-Monedero, Javier; Dencik, Lina; Edwards, Lilian; ‘What Does It Mean to ‘Solve’ the Problem of Discrimination in Hiring?’, 2019 ↩
-
Ibid. ↩
-
HireVue, ‘Train, Validate, Re-Train: How We Build HireVue Assessments Models’, 2018 and ScienceDaily, ‘Are hiring algorithms fair? They’re too opaque to tell, study finds’, 2019 ↩
-
Sanchez-Monedero, Javier; Dencik, Lina; Edwards, Lilian. ‘What Does It Mean to ‘Solve’ the Problem of Discrimination in Hiring?’, 2019 ↩
-
Crenshaw, Kimberlé. ‘Demarginalizing the intersection of race and sex: A black feminist critique of antidiscrimination doctrine, feminist theory and antiracist politics.’ in University of Chicago Legal Forum, Volume 1989, Issue 1, pp.139-167 ↩
-
U.S. Equal Employment Opportunity Commission, Questions and Answers on EEOC Final Rule on Disparate Impact and “Reasonable Factors Other Than Age” Under the Age Discrimination in Employment Act of 1967 ↩
-
Due to the focus of our review being bias, we were less concerned in our research with the accuracy of the tools involved. This is clearly an important question because if tools are ineffective, they are also arguably unethical however this sits outside the scope of this report. ↩
-
Financial Times, ‘How to design AI that eliminates disability bias’ and Wired, ‘An AI to stop hiring bias could be bad news for disabled people’, 2019, 2020 ↩
-
ICO, Guide to General Data Protection Regulation (GDPR) - Rights related to automated decision making including profiling ↩
-
Carter, S., Mwaura, S., Ram, M., Trehan, K., and Jones, T., ‘Barriers to ethnic minority and women’s enterprise: Existing evidence, policy tensions and unsettled questions’, in International Small Business Journal, no.33, 2015, pp.49-69 ↩
-
In the case of credit scoring, credit reference agency data tends to only go back six years, and lenders generally only look at the last few years, which should provide some mitigation against discriminatory lending practices from decades ago. ↩
-
Financial Times, ‘AI in banking reality behind the hype’, 2018 ↩
-
The survey was sent to almost 300 firms and a total of 106 responses were received. ↩
-
Financial Conduct Authority and Bank of England, ‘Machine learning in UK financial services’, 2019 ↩
-
Ibid. ↩
-
Ibid. ↩
-
McKinsey and Company, ‘Adoption of AI advances, but foundational barriers remain’, 2018 ↩
-
Financial Conduct Authority, ‘Financial Services AI Public Private Forum’, 2020 ↩
-
Ibid. ↩
-
The term was coined in the early days of computing to describe the concept that nonsense input data produces nonsense output. ↩
-
Bank of England, Speech by James Proudman, ‘Managing machines: the governance of artificial intelligence’, 2019 ↩
-
Hurley, M., and Adebayo, J.; ‘Credit scoring in the era of big data.’, in Yale Journal of Law and Technology, Volume 18, Issue 1, 2016; pp.148-216 ↩
-
Which?, ‘Experian credit reports to include rent payments for the first time’, 2018 ↩
-
Validation techniques including detecting errors and risks in the data. ↩
-
CDEI, ‘AI Barometer’, 2020 ↩
-
ICO and The Alan Turing Institute, ‘Guidance: Part 2 Explaining decisions made with AI’ ↩
-
The Behavioural Insights Team, ‘The perceptions of fairness of algorithms and proxy information in financial services’, 2019 ↩
-
The Bank of England prudentially regulates and supervises financial services firms through the Prudential Regulation Authority ↩
-
The Lammy Review, 2017 ↩
-
Institutional Racism was defined in The Stephen Lawrence Inquiry as: “the collective failure of an organisation to provide an appropriate and professional service to people because of their colour, culture or ethnic origin” - The Stephen Lawrence Inquiry, 1999 ↩
-
GOV.UK, ‘Ethnicity facts and figures’ ↩
-
Strategic Review of Policing in England and Wales, ‘Sir Michael Barber to head major review of the police service’, 2019 ↩
-
GOV.UK, ‘Prime Minister launches police recruitment drive’, 2019 ↩
-
Police Foundation, Data-Driven Policing and Public Value, 2019 ↩
-
Kit Malthouse MP, ‘Police Funding 2020/21: Written statement HCWS51’, 2020; ↩
-
Policing codes, such as the College of Policing’s National Decision Model and Code of Ethics are key reference points for decision-making in policing. ↩
-
Babuta, Alexander and Oswald, Marion; ‘Analytics and Algorithms in Policing in England and Wales Towards A New Policy Framework’, Royal United Services Institute, 2020 ↩
-
Now referred to as the Gangs Violence Matrix. ↩
-
Amnesty International, ‘Trapped in the Matrix’, 2020 ↩
-
ICO, ‘ICO finds Metropolitan Police Service’s Gangs Matrix breached data protection laws’, 2018 ↩
-
Mayor of London, ‘Mayor’s intervention results in overhaul of Met’s Gangs Matrix’, 2020 ↩
-
CDEI have published a research paper on facial recognition technology, which covers police use of live facial recognition technology, along with other uses. ↩
-
See for example: Richardson, Rashida and Schultz, Jason and Crawford, Kate, ‘Dirty Data, Bad Predictions: How Civil Rights Violations Impact Police Data, Predictive Policing Systems, and Justice’, AI Now Institute; Kearns, Ian and Rick Muir, ‘Data Driven Policing and Public Value’, The Police Foundation, 2019; ‘Policing By Machine’, Liberty, 2019 ↩
-
RUSI sent Freedom of Information requests to all police forces in England and Wales, interviewed over 60 people from police forces, technology providers, academia, civil society, government, and regulation, and ran roundtables, jointly with CDEI and TechUK. ↩
-
Babuta, Alexander and Oswald, Marion; ‘Analytics and Algorithms in Policing in England and Wales Towards A New Policy Framework’, RUSI, 2020 ↩
-
Couchman, Hannah; ‘Policing by machine’, Liberty, 2019 ↩
-
Robin Moore, ‘A Compendium of Research and Analysis on the Offender Assessment System (OASys) 2009–2013’, National Offender Management Service, Ministry of Justice Analytical Series, 2015 ↩
-
The Guardian, ‘Met uses software that can be deployed to see if ethnic groups ‘specialise’ in areas of crime’, 2020 ↩
-
See for example: Richardson, Rashida and Schultz, Jason and Crawford, Kate, ‘Dirty Data, Bad Predictions: How Civil Rights Violations Impact Police Data, Predictive Policing Systems, and Justice’, AI Now Institute ↩
-
Home Office, ‘Police powers and procedures, England and Wales, year ending 31 March 2019’ ↩
-
Nesta, ‘Decision-making in the Age of the Algorithm’, 2019 ↩
-
Babuta, Alexander and Oswald, Marion; ‘Analytics and Algorithms in Policing in England and Wales Towards A New Policy Framework’, RUSI, 2020 ↩
-
Ibid. ↩
-
NPCC and APCC, ‘National Policing Digital Strategy, Digital, Data and Technology Strategy 2020 - 2030’ ↩
-
TechUK, ‘Police funding settlement and tech’, 2020 ↩
-
Police Foundation, ‘Data-Driven Policing and Public Value’, 2019 ↩
-
Quote from Rick Muir, Director of Police Foundation, in, Strategic View of Policing, ‘Sir Michael Barber to head major review of the police service’, 2019 ↩
-
Royal Society for the encouragement of Arts, Manufactures and Commerce, ‘Artificial Intelligence: Real Public Engagement’, 2018 ↩
-
‘Ipsos MORI Veracity Index 2019’; 76% survey respondents trust the police to tell the truth - an increase of 15ppt since 1983. ↩
-
Local Government Association, ‘Local government funding - Moving the conversation on’, 2018 ↩
-
Open Access Government, ‘Changing the face of local government with digital transformation’, 2019 ↩
-
Oxford Internet Institute, University of Oxford, ‘Data Science in Local Government’, 2019 ↩
-
Eubanks, Virginia. Automating inequality: How high-tech tools profile, police, and punish the poor. St. Martin’s Press, 2018. ↩
-
As outlined in Chapter 5 on the policing sector, we are considering the use of tools as part of the full decision-making process. ↩
-
The Guardian, ‘One in three councils using algorithms to make welfare decisions’, 2019; and, Dencik, L. et al., ‘Data Scores as Governance: Investigating uses of citizen scoring in public services’, Data Justice Lab, Cardiff University, 2018 ↩
-
The Guardian, ‘One in three councils using algorithms to make welfare decisions’, 2019 ↩
-
Ibid. ↩
-
House of Commons Library, ‘The Troubled Families programme (England)’, 2020 ↩
-
Dencik, L. et al., ‘Data Scores as Governance: Investigating uses of citizen scoring in public services’, Data Justice Lab, Cardiff University, 2018 ↩
-
The Guardian, ‘One in three councils using algorithms to make welfare decisions’, 2019; and, Dencik, L. et al., ‘Data Scores as Governance: Investigating uses of citizen scoring in public services’, Data Justice Lab, Cardiff University, 2018 ↩
-
Denick, L. et al., ‘Data Scores as Governance: Investigating uses of citizen scoring in public services’, Data Justice Lab, Cardiff University, 2018, p.34 ↩
-
What Works for Children’s Social Care, ‘Ethics Review of Machine Learning in Children’s Social Care’, 2020 ↩
-
Tech Nation, ‘Diversity and inclusion in UK tech companies’ ↩ ↩2
-
Ibid. ↩
-
New Statesman Tech, ‘London Tech Week’s missing voice’, 2019 ↩
-
Tech Nation, ‘Diversity and inclusion in UK tech companies’ ↩
-
See Tech Talent Charter ↩
-
GOV.UK, ‘£18.5 million to boost diversity in AI tech roles and innovation in online training for adults’, 2019 ↩
-
Kilbertus, N.; Gascon, A.; Kusner, M.; Veale, M.; Gummadi, K. P.; and Weller, A.; ‘Blind Justice: Fairness with Encrypted Sensitive Attributes’. In the International Conference on Machine Learning (ICML), 2018 ↩
-
With over 75% of respondents comfortable sharing information on age and ethnicity, and over 65% sharing disability, religious belief or sex information with new employers in order to test for and prevent unintentional bias in their algorithms. ↩
-
Open Data Institute, ‘Monitoring Equality in Digital Public Services’, 2020 ↩
-
Open Data Institute, ‘Protected Characteristics in Practice’, 2019 ↩
-
Equality and Human Rights Commission, ‘The Public Sector Equality Duty and Data Protection’, 2015 ↩
-
Equality and Human Rights Commission, ‘Public Sector Equality Duty’ ↩
-
Kilbertus, N., Gascon, A., Kusner, M., Veale, M., Gummadi, K. P., and Weller, A., ‘Blind Justice: Fairness with Encrypted Sensitive Attributes’. In the International Conference on Machine Learning (ICML), 2018 ↩
-
ICO, ‘What do we need to do to ensure lawfulness, fairness, and transparency in AI systems’ ↩
-
Wachter, Sandra, and Mittelstadt, Brent; ‘A right to reasonable inferences: Re-thinking data protection law in the age of big data and AI’, Columbia Business Law Review, 2019, p.494 ↩
-
NHS Research Scotland, ‘Data Safe Haven’ ↩
-
The Alan Turing Institute, ‘Enabling trust models for differential privacy’, ongoing ↩
-
Office for National Statistics, ‘ONS methodology working paper series number 16 - Synthetic data pilot’, 2019 ↩
-
Note that in the machine learning literature on fairness, some terms used throughout this report take on specific, often narrower, definitions. Discrimination is sometimes used to refer to both different outcomes for different groups, and the statistical ability to distinguish between them. Bias is both favourable or unfavourable treatment of a group, and the statistical over or under-estimation of their quantitative properties. The field of study of how to create a mathematical system that is unbiased, is called “algorithmic fairness”. In this report we use “discrimination” and “bias” in the common language sense as defined in Chapter 2 (rather than their statistical meanings), and note that the concept of “fairness” discussed in this section is narrower than that described above. ↩
-
CDEI, ‘Training a biased model’, 2020 ↩
-
Calders, Toon, Kamiran, Faisal; and Pechenizkiy, Mykola; ‘Building Classifiers with Independency Constraints’, ICDMW ‘09: Proceedings of the 2009 IEEE International Conference on Data Mining Workshops, 2009; pp.13–18; and Zemel, Rich; Wu, Yu; Swersky, Kevin; Pitassi, Toni; and Dwork, Cynthia, ‘Learning Fair Representations, in International Conference on Machine Learning/’, 2013, pp.325–33 ↩
-
Larson, Jeff; Mattu, Surya; Kirchner, Lauren; and Angwin, Julia; ‘How We Analyzed the COMPAS Recidivism Algorithm’ 2016; and Corbett-Davies, Sam; Pierson, Emma; Feller, Avi; Goel, Sharad; and Huq, Aziz; ‘Algorithmic decision-making and the Cost of Fairness’, 2017 ↩
-
Kleinberg, Jon; Mullainathan, Sendhil; and Raghavan, Manis, ‘Inherent Trade-Offs in the Fair Determination of Risk Scores’, 2016 ↩
-
Kusner, Matt J.; Joshua, Loftus R.; , Russell, Chris and Silva, Ricardo; ‘Counterfactual Fairness’, in Advances in Neural Information Processing Systems, 2017; and Kilbertus, Niki; Rojas-Carulla, Mateo; Parascandolo, Giambattista; Hardt, Moritz; Janzing, Dominik; and Schölkopf, Bernhard, ‘Avoiding Discrimination through Causal Reasoning’, in Advances in Neural Information Processing Systems, 2018 ↩
-
Garg, Sahaj; Perot, Vincent; Limtiaco, Nicole; Taly, Ankur; Chi, Ed., and Beutel, Alex; ‘Counterfactual Fairness in Text Classification through Robustness’, in AAAI/ACM Conference on AI, Ethics, and Society, 2019; and Chiappa, Silvia and Gillam, Thomas P. S.; ‘Path-Specific Counterfactual Fairness’, in AAAI Conference on Artificial Intelligence, 2018; and Russell, Chris; Kusner, Matt J.; Loftus, Joshua and Ricardo Silva, ‘When Worlds Collide: Integrating Different Counterfactual Assumptions in Fairness’, In Advances in Neural Information Processing Systems, edited by Guyon, I.; Luxburg, U. V.; Bengio, S.; Wallach, H.; Fergus, R.; Vishwanathan, S. and Garnett, R.; Curran Associates, Inc., 2017 ↩
-
Kusner, Matt J.; Joshua, Loftus R.; , Russell, Chris and Silva, Ricardo; ‘Counterfactual Fairness’, in Advances in Neural Information Processing Systems, 2017; and Liu, Lydia T.; Dean, Sarah; Rolf, Esther; Simchowitz, Max and Hardt, Moritz; ‘Delayed Impact of Fair Machine Learning’, in International Conference on Machine Learning, 2018 ↩
-
Hu, Lily; Immorlica, Nicole; Wortman Vaughan, Jennifer, ‘The Disparate Effects of Strategic Manipulation’, in ACM Conference on Fairness, Accountability, and Transparency, 2018 ↩
-
ICO, ‘Guide to the General Data Protection Regulation (GDPR) - Special category data’ ↩
-
See, for example, many of those highlighted in the open source list curated by the Institute for Ethical AI and Machine Learning here ↩
-
Leslie, David; ‘Understanding artificial intelligence ethics and safety’, The Alan Turing Institute, (2019) ↩
-
Regulation for the Fourth Industrial Revolution, Department for Business, Energy and Industrial Strategy, 2019 ↩
-
Renewing regulation: Anticipatory regulation in an age of disruption, Nesta, 2019 ↩
-
Lord Sales, Justice of the UK Supreme Court, ‘Algorithms, Artificial Intelligence and the Law’, The Sir Henry Brooke Lecture for BAILI, 2019 ↩
-
For a more detailed discussion on direct and indirect discrimination, see Section 2.4 ↩
-
Equality and Human Rights Commission, ‘Public Sector Equality Duty’ ↩
-
See https://www.coe.int/en/web/artificial-intelligence/cahai, CDEI is providing expert input into this work. ↩
-
GDPR defines data processing broadly in Article 4(2): collection, recording, organisation, structuring, storage, adaptation or alteration, retrieval, consultation, use, disclosure by transmission, dissemination or otherwise making available, alignment or combination, restriction, erasure or destruction ↩
-
See, for example, https://techgdpr.com/blog/develop-artificial-intelligence-ai-gdpr-friendly/ https://iapp.org/news/a/want-europe-to-have-the-best-ai-reform-the-gdpr/ ↩
-
ICO, ‘Guide to the General Data Protection Regulation (GDPR) - Principle (a): Lawfulness, fairness and transparency’ ↩
-
ICO, ‘Guide to the General Data Protection Regulation (GDPR) - Rights related to automated decision making including profiling’ ↩
-
Financial Conduct Authority, ‘Fair treatment of customers’ ↩
-
Ofcom, ‘Statement: Making communications markets work for customers - a framework for assessing fairness in broadband, , mobile, home phone and pay TV’, 2019 ↩
-
See Section 2.4 for detailed discussion on these types of unfair bias. In other cases, algorithms could lead to bias based on arbitrary characteristics. It would not be practical to address these issues through discrimination law, as these biases are based on characteristics that differ by algorithm, and may not be identified in advance. ↩
-
Case C 236/09 Test-Achats ECLI:EU:C:2011:100, see summary here ↩
-
Equality and Human Rights Commission, ‘Our powers’ ↩
-
Equality and Human Rights Commission, Civil and political rights in Great Britain: Submission to the UN’, 2020 ↩
-
Equinet (European Network of Equalities Bodies): Meeting the new challenges to equality and non-discrimination from increased digitisation and the use of Artificial Intelligence (by Robin Allen QC and Dee Masters, June 2020) ↩
-
ICO, ‘ICO and the Turing consultation on Explaining AI decisions guidance’, 2020 ↩
-
ICO, ‘ICO investigation into how the police use facial recognition technology in public places’, 2019 ↩
-
ICO, ‘What do we need to do to ensure lawfulness, fairness and transparency in AI systems’, 2020 ↩
-
Financial Conduct Authority, ‘GC20/3: Guidance from firms on the fair treatment of vulnerable customers’, 2020 ↩
-
Financial Conduct Authority, ‘Regulatory Sandbox’ ↩
-
https://old.parliament.uk/business/committees/committees-a-z/commons-select/women-and-equalities-committee/inquiries/parliament-2017/enforcing-the-equality-act-17-19/ ↩
-
ICO, ‘Guide to the General Data Protection Regulation (GDPR) - What is a DPIA?’, Retrieved March 30, 2019 ↩
-
Equality and Human Rights Commission, ‘Equality impact assessments’ ↩
-
https://www.ifow.org/publications/artificial-intelligence-in-hiring-assessing-impacts-on-equality ↩
-
It is of course good practice to update impact assessments over time, and indeed GDPR requires DPIAs to be revisited when there is a change in the risk profile (see GDPR Article 35(11)), but there is not always a clear trigger point for an organisation to invest the time to do this. ↩
-
https://www.ifow.org/publications/mind-the-gap-the-final-report-of-the-equality-task-force ↩
-
https://rusi.org/sites/default/files/rusi_pub_165_2020_01_algorithmic_policing_babuta_final_web_copy.pdf ↩
-
For further discussion of this issue, see Allen, R and Masters, D, 2020. Cloisters, September 2019, The Legal Education Foundation, In the matter of Automated Data Processing in Government Decision Making, available here ↩
-
ICO, ‘Guide to the General Data Protection Regulator (GDPR) - Certification’ ↩
-
The Guardian, ‘Government to review 1.6m disability benefit claims after U-turn’, 2018; and BBC, ‘Personal independence payments: All 1.6m claims to be reviewed’, 2018; and Government update on progress of this review ↩
-
National Audit Office, ‘Reforming the UK border and immigration system’, 2014 ↩
-
Brauneis, Robert and Goodman, Ellen P., ‘Algorithmic Transparency for the Smart City ‘(August 2, 2017). 20 Yale J. of Law and Tech. p.103, 2018 ↩
-
See, for example, GOV.UK, Department of Health and Social Care, ‘Personal information charter’; and GOV.UK, Department for Work and Pensions, ‘Personal information charter; and GOV.UK, Home Office, ‘Personal information charter’ ↩
-
FOI release, ‘Publication of spending data by local authorities’ ↩
-
Transparency data, ‘HMRC’s headcount and payroll data for January 2020’ ↩
-
The Committee on Standards in Public Life, ‘The Continuing Importance of Ethical Standards for Public Service Providers’, 2018 ↩
-
Guidance, ‘Managing public money ↩
-
Oxford Internet Institute, University of Oxford, ‘Data Science in Local Government’, 2019 ↩
-
There are differing accounts. For example, an investigation by The Guardian last year showed some 140 of 408 councils in the UK are using privately-developed algorithmic ‘risk assessment’ tools, particularly to determine eligibility for benefits and to calculate entitlements; the New Statesman revealed that Experian secured £2m from British councils in 2018; and Data Justice Lab research in late 2018 showed 53 out of 96 local authorities and about a quarter of police authorities are now using algorithms for prediction, risk assessment and assistance in decision-making. ↩
-
Committee on Standards in Public Life, ‘Artificial Intelligence and Public Standards’, 2020 ↩
-
United Nations Human Rights, Office of the High Commissioner, 2019 ↩
-
Vishwanath, T., Kaufmann, D.: Toward transparency: New approaches and their application to financial markets. The World Bank Research Observer 16(1), 2001; 41–57 ↩
-
Mortier, R.; Haddadi, H.; Henderson, T.; McAuley, D.; Crowcroft, J.; ‘Human-data interaction: the human face of the data-driven society’, 2014 ↩
-
Weller, A.; ‘Transparency: Motivations and Challenges’, 2019 ↩
-
The Centre for Data Ethics and Innovation’s approach to the governance of data-driven technology ↩
-
O’Neill, Onora, ‘Reith 2002: A Question of Trust’, Open University, 2002 ↩
-
Ted Blog, ‘How to trust intelligently’, 2013 ↩
-
Spiegelhalter, David - ‘Should we trust algorithms?’, Harvard Data Science Review, 2020 ↩
-
Committee on Standards in Public Life, ‘Artificial Intelligence and Public Standards’, 2020 ↩
-
Mitchell, Margaret et al., ‘Model Cards for Model Reporting’, 2018 ↩
-
Government of Canada’s ‘Algorithmic Impact Assessment’ ↩
-
ProPublica, ‘New York City Moves to Create Accountability for Algorithms’, 2017 ↩
-
GOV.UK, HM Treasury, ‘Review of quality assurance of government of government models’, 2013 ↩
-
Leslie, David; ‘Understanding artificial intelligence ethics and safety’, The Alan Turing Institute, 2019 ↩
-
House of Commons Science and Technology Committee, ‘Algorithms in decision-making, Fourth Report of Session 2017-19’ ↩
-
The Law Society, ‘Algorithms in the criminal justice system’, 201 ↩
-
See reference from Spiegelhalter, D.; ‘Should We Trust Algorithms?’ Harvard Data Science Review, 2(1). (2020) ↩
-
House of Lords Select Committee on Artificial Intelligence, ‘AI in the UK:, ready, willing and able? Report of session 2017-19’ ↩
-
The Committee on Standards in Public Life, ‘The Continuing Importance of Ethical Standards for Public Service Providers’, 2018 ↩
-
GOV.UK, ‘Guidelines for AI procurement’, 2020; and World Economic Forum, ‘UK Government First to Pilot AI Procurement Guidelines Co-Designed with World Economic Forum’, 2019 ↩
-
Committee on Standards in Public Life, ‘Artificial Intelligence and Public Standards’, 2020 ↩
-
Crown Commercial Service, ‘Artificial Intelligence (AI)’ ↩